11am - 12 noon
Tuesday 24 May 2022
Neural Reconstruction and Rendering of 3D Humans from Video
PhD Viva Open Presentation by Akin Caliskan - All Welcome!
Free
CVSSP Seminar Room
University of Surrey
Guildford
Surrey
GU2 7XH
This event has passed
Speakers
Neural Reconstruction and Rendering of 3D Humans from Video
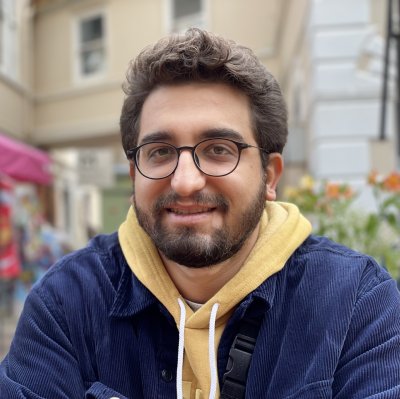
Abstract:
Learning to reconstruct and render humans from unconstrained video sequences captured by a few cameras is extremely challenging due to the complex shape and articulated motion of the human body. This thesis explores neural architectures and representations and examines possible solutions to tackle these challenges.
Conventional 3D dynamic human reconstruction builds upon the video sequences captured by advanced cameras systems in controlled environments. Stereo image pairs from the capture are processed to compute depth images. For wide baseline separated camera configurations, an initial model of the human is used as a proxy to compute stereo reconstruction since the conventional dense stereo matching methods are prone to fail. To mitigate this problem, the first contribution of the thesis explores a neural network architecture to learn dense stereo reconstruction for people without any proxy 3D model required. We also introduce a new stereo dataset for humans to learn generalizable neural features and stereo matching networks.
The outcome of this research outperforms the baseline methods for quantitative and qualitative experiments, showing improved stereo depth estimation for people.
The conventional 3D dynamic human reconstruction methods are heavily dependent on data captured by a significant number of cameras in a controlled environment. This causes issues with the democratization of 3D human reconstruction for emerging technologies that required digital virtual humans. To address this, the second contribution of the thesis explores 3D human reconstruction from a single image. Previous approaches have limited performance on clothing and hair reconstruction and consistent reconstruction for different views. So, this part of the thesis addresses a novel multi-view loss function and a new dataset consisting of realistic image-3D human model pairs with clothing and hair details. The outcome of this research outperforms the state-of-the-art methods on both synthetic and real datasets and shows the possibility of 3D human avatar generation from a single image.
Rapid development in deep learning research shows the immediate need for datasets to train generalizable networks for real-life problems. Previous methods approach this need by proposing annotated data for 2D image applications; however, it is difficult and expensive to annotate 3D data. In the first two chapters of this thesis, we showed the utilization of synthetic data to train deep neural networks for 3D computer vision tasks. However, the need for synthetic data for human-related computer vision problems requires a rendering of realistic 3D human models with the subject, pose, clothing, appearance, scene, and illumination variation. To address this, the third contribution of the thesis is a synthetic human dataset generation framework for human reconstruction and human tracking tasks. The dataset framework is designed and developed in the scope of this thesis and multiple datasets are generated to address computer vision challenges of 3D human reconstruction and rendering.
Significant effort has previously been devoted to single-image 3D human reconstruction; however, real humans are dynamic and state-of-the-art approaches often fail to achieve temporally consistent and high-resolution 3D human reconstruction from an unconstrained monocular video. To address this problem, the fourth contribution of the thesis explores a novel temporal consistency function and a hybrid neural feature embedding. The output of this research outperforms state-of-the-art methods enabling temporally consistent 3D human reconstruction.
Conventional dynamic human character generation methods consist of two main parts, namely reconstruction, and rendering of 3D humans. In this case, reconstruction has to be as accurate as possible so that texture rendering can be applied using traditional computer graphics methods. In the majority of this thesis, the task is to replace the reconstruction part of the conventional capturing method with deep learning-based techniques that require only one camera. However, the fifth contribution of the thesis explores the possibility of realistic human avatar rendering with a coarse geometry estimation using a neural rendering module instead of a traditional rendering pipeline. For this purpose, a coarse geometry of the subject is estimated from a monocular video and the final rendering of a person in an arbitrary pose is predicted using a neural rendering module. Furthermore, in the final chapter, a novel weakly-supervised training methodology is proposed which requires only a few frames of the subject in natural poses.
Taken together, these contributions address the creation of digital virtual humans from a video. The presented research advances the field of 3D human reconstruction and rendering from unconstrained videos. Finally, the outcome of the thesis is an important step towards creating realistic, animatable human avatars from unconstrained videos.
Attend the Event
This is a free hybrid event open to everyone.