11am - 12 noon
Monday 22 May 2023
Machine learning methods to analyse dynamic and incomplete data
PhD Open Viva Presentation by Narges Pourshahrokhi.
Free
University of Surrey
Guildford
Surrey
GU2 7XH
This event has passed
Abstract
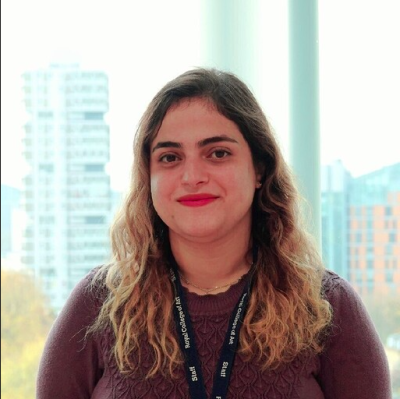
The rapid advancements in sensor networks, cyber-physical systems, and the widespread adoption of the Internet of Things (IoT) have led to a vast collection of data from various domains, such as health care, agriculture, finance, education, and more. However, the collected data is often of low quality due to noise, incompleteness, and inconsistencies caused by human error and machine failure, leading to missing values in datasets.
These missing values pose significant challenges for data analysis and machine learning, as they can reduce the reliability of the dataset and result in biased or misleading results.
Additionally, some data is not publicly available due to privacy concerns or difficulties in collecting it, making it difficult for researchers and scientists to access and analyse it. To overcome these challenges, researchers often use synthetic data generation techniques to generate new samples while preserving the characteristics of the original data to respect privacy concerns.
Moreover, high-dimensionality and multi-modality increase the complexity of mentioned challenges.
The aim of this thesis is to address the challenges posed by high-dimensional, multi-modal data with missing or lower numbers of records. A thorough review of related work reveals that while data imputation and synthetic data generation methods have been extensively studied, they are not effective for high-dimensional, multi-modal data. To resolve these issues, the thesis proposes a generalisable model called Folded Hamiltonian Monte Carlo (F-HMC).
The F-HMC model is presented in several stages of development throughout this thesis. The third chapter introduces F-HMC as a sampling-based method. The following chapter improves the model by integrating it with a Generative Adversarial Network (GAN) within a Bayesian framework, enhancing its capabilities in generating synthetic data for complex targets. In chapter 5, the model is further improved to handle time series data.
Measuring the effectiveness of the generation or imputation procedure is another problem when working with generated or imputed data. Because it can be challenging to employ a metric that assesses the quality of generated data since it can be subjective. On the other hand, when the value that is missing is unknown, determining the quality of data that has been imputed might be difficult. This thesis focuses on this issue and provides potential solutions for it.
The evaluation method needs to be reasonable in terms of distance, performance, and similarity metrics. To evaluate the effectiveness of the F-HMC model, three main evaluation metrics are used: Normalised Root Mean Square Error (NRMSE) as distance, classification prediction as performance, and propensity as similarity metric. The results show that the F-HMC model outperforms the baseline in the suggested metrics, making it a promising solution for handling missing or low-quality data in high-dimensional, multi-modal scenarios.
Visitor information
Find out how to get to the University, make your way around campus and see what you can do when you get here.