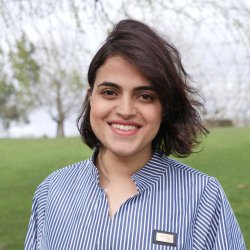
Dr Narges Pourshahrokhi
Academic and research departments
Faculty of Engineering and Physical Sciences, Centre for Vision, Speech and Signal Processing (CVSSP).Publications
The rapid growth in collecting and sharing sensory observation form the urban environments provides opportunities to plan and manage the services in the cities better and allows citizens to also observe and understand the changes in their surrounding in a better way. The new urban sensory data also creates opportunities for further application and service development by creative industries and start-ups. However, as the size and diversity of this data increase, finding and accessing the right set of data in a timely manner is becoming more challenging. This paper describes a search engine designed for indexing, searching and accessing urban sensory data. We present the key feature and architecture of the system and demonstrate some of the functionalities that are provided by searching for raw sensory observations and also pattern search functions that are enabled by a pattern analysis algorithm, supported by monitoring of data streams for changes in quality of information and remediation.
Traditional authentication mechanisms use passwords, Personal Identification Numbers (PINs) and biometrics, but these only authenticate at the point of entry. Continuous authentication schemes instead allow systems to verify identity and mitigate unauthorised access continuously. However, recent developments in generative modelling can significantly threaten continuous authentication systems, allowing attackers to craft adversarial examples to gain unauthorised access and may even limit a legitimate user from accessing protected data in the network. The research available on the use of generative models for attacking continuous authentication is relatively scarce. This paper explores the feasibility of bypassing continuous authentication using generative models, measuring the impact of the damage, and advising the usage of metrics to compare the various advertised attacks in such a system. Our empirical results demonstrate that generative models cause a higher Equal Error Rate and misclassification error in attack scenarios. At the same time, training and detection time during attack scenarios is increased compared to perturbation models. The results prove that data samples crafted by generative models can be a severe threat to continuous authentication schemes using motion sensor data.
Due to the rapid development of the Internet of Things (IoT) and consequently, the availability of more and more IoT data sources, mechanisms for searching and integrating IoT data sources become essential to leverage all relevant data for improving processes and services. This paper presents the IoT search framework IoTCrawler. The IoTCrawler framework is not only another IoT framework, it is a system of systems which connects existing solutions to offer interoperability and to overcome data fragmentation. In addition to its domain-independent design, IoTCrawler features a layered approach, offering solutions for crawling, indexing and searching IoT data sources, while ensuring privacy and security, adaptivity and reliability. The concept is proven by addressing a list of requirements defined for searching the IoT and an extensive evaluation. In addition, real world use cases showcase the applicability of the framework and provide examples of how it can be instantiated for new scenarios.
Missing values exist in nearly all clinical studies because data for a variable or question are not collected or not available. Imputing missing values and augmenting data can significantly improve generalisation and avoid bias in machine learning models. We propose a Hybrid Bayesian inference using Hamiltonian Monte Carlo (F-HMC) as a more practical approach to process cross-dimensional relations by applying a random walk and Hamiltonian dynamics to adapt posterior distribution and generate large-scale samples. The proposed method is applied to cancer symptom assessment, and MNIST datasets confirmed to enrich data quality in precision, accuracy, recall, F1-score, and propensity metric.