11am - 12 noon
Tuesday 23 January 2024
Shape and appearance super-resolution for 3D humans
PhD Viva Open presentation by Marco Pesavento. All welcome!
Free
University of Surrey
Guildford
Surrey
GU2 7XH
This event has passed
You can join us either in person or online.
Abstract
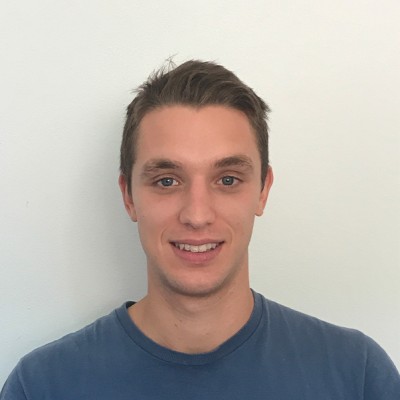
The surge in interest towards immersive applications in entertainment and in industries has influenced research, prompting an increasing emphasis for digital avatar creation. A pivotal requirement in immersive applications is the attainment for a high-level of fidelity for these digital human avatars, mirroring real world characteristics. The realism of the avatar contributes to the comfort of the users in a virtual environment, which should closely emulate reality. The focus of this thesis is on reconstructing highly realistic human avatars from input data where details of humans may not be distinctly visible due to factors such as low-resolution images of the subject, large capture volume or noise introduced by the capture system. In addition, this thesis focuses on improving the reproducibility of reconstruction techniques by using only a minimal number of consumer-grade sensors to reconstruct digital humans. This objective underscores the importance of making high-quality reconstruction techniques more accessible and feasible, irrespective of the equipment used.
The first contribution of the thesis addresses the problem of low-quality texture appearance when capturing in a large volume. Typically, the requirement to frame cameras to capture the volume in a large space (>50m^3) results in the person occupying only a small proportion of the field of view ($<$ 10\%), resulting in low-quality rendering of the captured subject. The quality of the appearance of the large-volume capture is improved through super-resolution appearance transfer from a static high-resolution appearance capture rig that involves high-resolution digital cameras (> 8k) to capture the person in a small volume (<8m^3). The proposed approach reproduces the high-resolution detail of the static capture whilst maintaining the appearance of the captured video.
The second contribution is an Attention-based Multi-Reference Super-resolution network (AMRSR) that, given a low-resolution image, learns to adaptively transfer the most similar texture from multiple reference images to the super-resolution output whilst maintaining spatial coherence. The concept of reference super-resolution is extended to multi-reference super-resolution by providing a more diverse pool of image features to overcome the inherent information deficit while maintaining memory efficiency. With this approach, images showing all the sides of a human model can be leveraged as references to super-resolve the texture map of the model. Using just a single reference deteriorates performance because it does not contain all the information illustrated in a texture map. With multiple images as references, the network can learn relevant features from all the regions of the body.
The third contribution consists of a novel super-resolution human shape introduced to represent high-quality details in the 3D shape reconstructed from a single low-resolution image or from an image captured in a large volume, where the human occupies only a small fraction of the image. A novel framework learns a high-detail implicit function to represent the reconstructed shape.
The proposed method overcomes limitations of existing approaches, which require high-resolution images in conjunction with auxiliary data, such as surface normals or parametric models, to reconstruct a high-quality 3D shape from a single image.
The fourth contribution is a new method that reconstructs accurate full-body human shapes from single-view RGB-D images. The previous contribution demonstrated that neural implicit models are beneficial for the generation of high-quality and accurate surfaces. However, they are easily prone to depth ambiguities with only front-facing appearance observations, which result in distorted shapes along the camera depth axis. The benefit of depth observations is investigated with an approach that considers a single RGB-D image as input. The introduced framework is build on neural implicit representation and proposes a data-driven strategy to learn accurate geometric details from both multi-resolution pixel-aligned and voxel-aligned features.
The final contribution of this thesis introduces a novel framework for reconstructing a complete, high-quality 3D human shape from a single image by leveraging a collection of monocular unconstrained images. Using a single image alone does not provide sufficient information to accurately reproduce details in body regions that are not visible in the input, leading to excessively smoothed reconstructions. Rather than using inaccessible multi-view capture systems to acquire multiple views of an individual, uncalibrated and unregistered images of the subject are leveraged. A novel module processes these reference images to simulate a multi-view scenario by generating 2D normal maps of the individual in the same pose as the target input. A multi-view transformer-based neural implicit model estimates the implicit representation of the complete, high-quality 3D human shape from the generated normal maps.
Featured Academics
Visitor information
Find out how to get to the University, make your way around campus and see what you can do when you get here.