10am - 11am
Tuesday 17 September 2024
Unveiling the Potential of Synthetic Data for Animal Pose Estimation
PhD Viva Open Presentation - Moira Shooter
Hybrid event - All Welcome!
Free
Arthur C. Clarke building
University of Surrey
Guildford
Surrey
GU2 7XH
This event has passed
Unveiling the Potential of Synthetic Data for Animal Pose Estimation
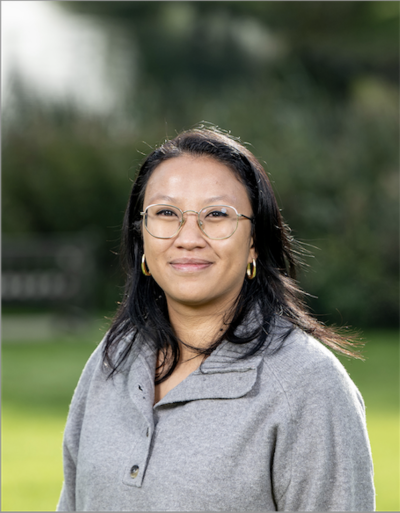
Abstract:
This thesis explores the changing impact of synthetic data on advancing the field of animal pose estimation, focusing specifically on dogs, but extendable to other species. The research addresses critical challenges inherent in animal pose estimation, particularly those arising from data scarcity, aiming to enhance the accuracy and temporal consistency of animal pose estimation models.
The first contribution involves the creation of a comprehensive 2D synthetic dog dataset called SyDog using the game engine Unity3D. This dataset serves as a valuable resource for refining the accuracy of 2D dog pose estimation models, laying the groundwork for improved accuracy and robustness in estimating the 2D skeletal pose from images of canines. Despite limitations in achieving photorealism, the dataset significantly enhances pose estimation accuracy.
The second contribution extends into the temporal domain by generating a synthetic video dog dataset called SyDog-Video. This approach is motivated by two factors. The absence of real-world dog video pose datasets and the advantages associated with generating synthetic datasets, in contrast to the complexities involved in manually labelling real-world videos. The pre-training of a video-based pose estimation model with the SyDog-Video dataset consistently demonstrates superior performance when compared to models pre-trained on existing real-world animal datasets. Additionally, the versatility exhibited by the network pre-trained on SyDog-Video becomes apparent in its adept tackling of challenging scenarios such as handling temporal occlusion. This approach ensures temporally consistent 2D pose predictions for dynamic sequences and challenging scenarios of dog movements, presenting a novel solution for video-based applications.
The third contribution addresses the domain gap and the scarcity of 3D pose datasets by proposing a novel pose estimation network (D-Pose), including two novel synthetic datasets named DigiDogs and 3DDogs. Additionally, a 3D pose benchmark is established. It is demonstrated through extensive experiments that it is possible to extract plausible 3D poses from in-the-wild monocular images by leveraging the synergy between synthetic data and the proposed pose estimation network, D-Pose.
In conclusion, this thesis not only advances the capabilities of pose estimation models for dogs but also establishes synthetic data as an essential tool in overcoming challenges associated with data scarcity including acquiring data. The findings contribute significantly to the broader field of computer vision, with implications for applications ranging from wildlife conservation efforts and ecological research to applications in agriculture, robotics, animal sports and entertainment.