11am - 12 noon
Tuesday 13 May 2025
Unsupervised Anomaly Detection in Sensor-based Remote Healthcare Monitoring
PhD Viva Open Presentation - Nivedita Bijlani
Online event - All Welcome!
Free
University of Surrey
Guildford
Surrey
GU2 7XH
Unsupervised Anomaly Detection in Sensor-based Remote Healthcare Monitoring
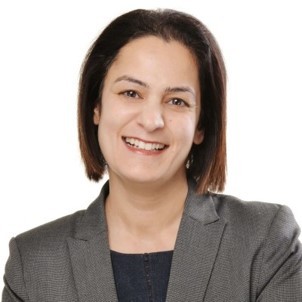
Abstract:
Sensor-based remote healthcare monitoring offers valuable insights into daily living patterns for people with long-term conditions, with minimal intrusion. Detecting anomalies in sensor data is critical for identifying adverse health events early, enabling timely intervention, preventing hospitalisations, and easing the caregiving burden. An effective anomaly detection model should be fast, personalised, noise-robust, low-maintenance, and explainable to clinicians and carers. Existing statistical methods rely on predefined rules or distributions and struggle with noise and evolving patterns, while many deep learning models lack adaptability, personalisation, explainability, or efficiency. This research addresses these gaps by developing unsupervised AI methods for detecting anomalies and identifying adverse events in home sensor data in a lightweight, explainable, and personalised yet generalisable manner for people living with dementia.
Our first contribution addresses anomaly detection through outlier and pattern discovery, using the high-speed Matrix Profile to identify significant discords in patient activity timelines. We introduce the Multidimensional Contextual Matrix Profile (M-CMP) to detect anomalies in noisy multivariate sensor data while reducing noise. To enhance explainability, we also develop a method for identifying patient-specific and cohort-wide anomaly biomarkers for clinical interpretation. Finally, we propose "generalisability" as a metric to capture sensitivity across individual patient households.
Our second contribution builds on the M-CMP to train a patient-agnostic, self-supervised GNN that encodes distance information from multi-channel CMPs as graphs. We reframe anomaly detection as a graph problem, identifying significant changes between successive graphs. This first learned Matrix Profile-based model enables cross-patient training without the need for retraining during operation.
Our third contribution introduces a Graph Barlow Twins (G-BT) contrastive learning approach to create noise-robust graph representations from daily activity, improving anomaly detection in noisy sensor data. Moving beyond CMP-based preprocessing, we directly use granular daily sensor data to extract richer features and reduce latency. We also develop a data-driven system for generating household-personalised, periodically refreshed alert thresholds aligned with clinician-defined target alert rates. Graph attention-based explainability is used to identify digital biomarkers of anomalies.
Our fourth contribution adapts contrastive learning frameworks from computer vision for representation learning in sensor-based health monitoring. The proposed adaptive contrast model automatically learns the aggregation factor during training and outperforms fixed-contrast models and G-BT across three test cohorts of 65 people with dementia from the Minder study by the UK Dementia Research Institute. We also introduce a novel "spatiotemporal attention map", combining temporal attention with spatial relevance to identify the most influential times and sensors for daily anomaly detection.
Finally, we demonstrate the real-world effectiveness of the adaptive contrast model through operational validation on a cohort of 90 patients from the Minder study (May 2022–February 2024). This confirms its readiness for deployment, supporting integration into the production Minder system and expansion to over 260 households. Offering both qualitative and quantitative insights, this work establishes a framework for AI-driven anomaly detection in real-world healthcare.
The methods developed in this research are domain-agnostic and applicable to other healthcare, urban, and industrial sensor environments that share similar operational challenges - including small signal-to-noise ratios, irregular activity patterns, scarce reliable annotations, need for explainability in decision support, and efficacy across diverse profiles and environments.