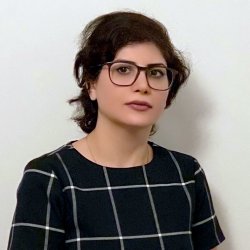
Dr Samaneh Kouchaki
Academic and research departments
Centre for Vision, Speech and Signal Processing (CVSSP), School of Computer Science and Electronic Engineering.About
Biography
I joined CVSSP in July 2020 to lead research and teaching in machine learning for health and dementia care in collaboration with the UK Dementia Research Institute (UK DRI) Care Research & Technology Centre (a joint initiative between CVSSP, the Surrey Sleep Research Centre and Department of Mathematics at Surrey, and Imperial College London).
I previously spent three years as a postdoctoral researcher within the Institute of Biomedical Engineering at the University of Oxford. I was the senior machine learning researcher for the ‘100,000 Genomes Project for Tuberculosis’, an international consortium involving the Centres for Disease Control of most major nations (including the USA, UK and China), jointly funded by the Gates Foundation and the Wellcome Trust. My research focus was on the prediction of antibiotic resistance in pathogens such as those that cause tuberculosis.
Prior to this, I was at the University of Manchester within the Division of Evolutionary and Genomic Sciences where I was funded by the EU Horizon 2020 Virogenesis project, working on next-generation DNA sequencing using signal and image processing techniques coupled with unsupervised machine learning.
I obtained my PhD in Computer Science at Surrey in 2015. My PhD focused on developing novel multi-way techniques for source separation with application to biomedical signals.
Areas of specialism
Biomedical signal processing; deep supervised/semi-supervised learning for healthcare data; graph learning and embedding for omics data; time-series data processing and pattern analysis.
ResearchResearch interests
Dr Kouchaki’s research is aimed at improving patient care by providing decision support. Her objective is to develop intelligent tools, based on hybrid architectures of advanced probabilistic and deep learning techniques, that facilitate improved patient outcomes.
Research collaborations
Collaboration with the UK Dementia Research Institute (UK DRI) Care Research & Technology Centre on machine learning for health and dementia care.
PhD research positions
Please contact me if you are interested in PhD in Machine learning for Healthcare applications.
Research interests
Dr Kouchaki’s research is aimed at improving patient care by providing decision support. Her objective is to develop intelligent tools, based on hybrid architectures of advanced probabilistic and deep learning techniques, that facilitate improved patient outcomes.
Research collaborations
Collaboration with the UK Dementia Research Institute (UK DRI) Care Research & Technology Centre on machine learning for health and dementia care.
PhD research positions
Please contact me if you are interested in PhD in Machine learning for Healthcare applications.
Supervision
Postgraduate research supervision
Narges Pourshahrokhi
Elaheh Kalantari
Nivedita Bijlani
Bahar Khorram
Jamal Hashem
Co-supervision:
Adam Dowse
Hesam Alaei
Tingyu Guo
Charlie Baskerville
Completed postgraduate research projects I have supervised
Roonak Rezvani
Teaching
LABORATORIES DESIGN & PROFESSIONAL STUDIES III and IV (EEE2036 and EEE2037)
COMPUTER AND DIGITAL LOGIC (EEE1033)
Publications
Large Language Models (LLMs), particularly those similar to ChatGPT, have significantly influenced the field of Natural Language Processing (NLP). While these models excel in general language tasks, their performance in domain-specific downstream tasks such as biomedical and clinical Named Entity Recognition (NER), Relation Extraction (RE), and Medical Natural Language Inference (NLI) is still evolving. In this context, our study investigates the potential of instruction tuning for biomedical language processing, applying this technique to two general LLMs of substantial scale. We present a comprehensive, instruction-based model trained on a dataset that consists of approximately 200,000 instruction-focused samples. This dataset represents a carefully curated compilation of existing data, meticulously adapted and reformatted to align with the specific requirements of our instruction-based tasks. This initiative represents an important step in utilising such models to achieve results on par with specialised encoder-only models like BioBERT and BioClinicalBERT for various classical biomedical NLP tasks. Our work includes an analysis of the dataset’s composition and its impact on model performance, providing insights into the intricacies of instruction tuning. By sharing our codes, models, and the distinctively assembled instruction-based dataset, we seek to encourage ongoing research and development in this area.22Our code repository is available at https://github.com/nlpie-research/BioInstTune-LLM. •Developed Llama2-MedTuned models, specifically for biomedical NER, RE, and NLI tasks among others.•Created a unique, instruction-focused dataset with around 200,000 samples for biomedical tuning.•Demonstrated instruction tuning’s effectiveness in aligning general LLMs with biomedical tasks.•Tested on classical biomedical NLP tasks, showing potential for broader clinical application.
Background: Sensor-based remote health monitoring is increasingly used to detect adverse health in people living with dementia (PLwD) at home, aiming to prevent hospitalizations and reduce caregiver burden. However, home sensor data is often noisy, overly granular, and suffers from unreliable labeling, data drift and high variability between households. Current anomaly detection methods lack generalizability and personalization, often requiring anomaly-free training data and frequent model updates.Objective: To develop a lightweight, explainable, self-supervised approach with personalized alert thresholds to detect adverse health events in PLwD, using changes in home activity.Methods: We hypothesized that health downturns manifest as detectable shifts in household movement patterns. Our approach leverages a Graph Barlow Twins contrastive model, which uses granular activity data and a macroscopic view to extract noise-robust, high-level and low-level discriminative features that represent daily activity patterns. Household-personalized alert thresholds are calculated based on clinician-set target alert rates, and daily anomaly scores are compared against these thresholds, triggering alerts for the clinical monitoring team. Model attention weights support explainability. Data were collected from a real-world dataset by the UK Dementia Research Institute (August 2019-April 2022).Results: Our model outperformed state-of-the-art temporal graph algorithms in detecting agitation and fall events across three patient cohorts, achieving 81% average recall and 88% generalizability at a target alert rate of 7%.Conclusion: We developed a novel, lightweight, explainable, and personalized Graph Barlow Twins model for real-world remote health monitoring in dementia care, with potential for broader applications in healthcare and sensor-based environments.
Machine learning, including deep learning, reinforcement learning, and generative artificial intelligence are revolutionising every area of our lives when data are made available. With the help of these methods, we can decipher information from larger datasets while addressing the complex nature of biological systems in a more efficient way. Although machine learning methods have been introduced to human genetic epidemiological research as early as 2004, those were never used to their full capacity. In this review, we outline some of the main applications of machine learning to assigning human genetic loci to health outcomes. We summarise widely used methods and discuss their advantages and challenges. We also identify several tools, such as Combi, GenNet, and GMSTool, specifically designed to integrate these methods for hypothesis-free analysis of genetic variation data. We elaborate on the additional value and limitations of these tools from a geneticist’s perspective. Finally, we discuss the fast-moving field of foundation models and large multi-modal omics biobank initiatives.
Abstract Specialised pre-trained language models are becoming more frequent in Natural language Processing (NLP) since they can potentially outperform models trained on generic texts. BioBERT (Sanh et al., Distilbert, a distilled version of bert: smaller, faster, cheaper and lighter. arXiv preprint arXiv: 1910.01108 , 2019) and BioClinicalBERT (Alsentzer et al., Publicly available clinical bert embeddings. In Proceedings of the 2nd Clinical Natural Language Processing Workshop , pp. 72–78, 2019) are two examples of such models that have shown promise in medical NLP tasks. Many of these models are overparametrised and resource-intensive, but thanks to techniques like knowledge distillation, it is possible to create smaller versions that perform almost as well as their larger counterparts. In this work, we specifically focus on development of compact language models for processing clinical texts (i.e. progress notes, discharge summaries, etc). We developed a number of efficient lightweight clinical transformers using knowledge distillation and continual learning, with the number of parameters ranging from $15$ million to $65$ million. These models performed comparably to larger models such as BioBERT and ClinicalBioBERT and significantly outperformed other compact models trained on general or biomedical data. Our extensive evaluation was done across several standard datasets and covered a wide range of clinical text-mining tasks, including natural language inference, relation extraction, named entity recognition and sequence classification. To our knowledge, this is the first comprehensive study specifically focused on creating efficient and compact transformers for clinical NLP tasks. The models and code used in this study can be found on our Huggingface profile at https://huggingface.co/nlpie and Github page at https://github.com/nlpie-research/Lightweight-Clinical-Transformers , respectively, promoting reproducibility of our results.
Urinary Tract Infections (UTIs) are one of the most prevalent bacterial infections in older adults and a significant contributor to unplanned hospital admissions in People Living with Dementia (PLWD), with early detection being crucial due to the predicament of reporting symptoms and limited help-seeking behaviour. The most common diagnostic tool is urine sample analysis, which can be time-consuming and is only employed where UTI clinical suspicion exists. In this method development and proof-of-concept study, participants living with dementia were monitored via low-cost devices in the home that passively measure activity, sleep, and nocturnal physiology. Using 27828 person-days of remote monitoring data (from 117 participants), we engineered features representing symptoms used for diagnosing a UTI. We then evaluate explainable machine learning techniques in passively calculating UTI risk and perform stratification on scores to support clinical translation and allow control over the balance between alert rate and sensitivity and specificity. The proposed UTI algorithm achieves a sensitivity of 65.3% (95% Confidence Interval (CI) = 64.3-66.2) and specificity of 70.9% (68.6-73.1) when predicting UTIs on unseen participants and after risk stratification, a sensitivity of 74.7% (67.9-81.5) and specificity of 87.9% (85.0-90.9). In addition, feature importance methods reveal that the largest contributions to the predictions were bathroom visit statistics, night-time respiratory rate, and the number of previous UTI events, aligning with the literature. Our machine learning method alerts clinicians of UTI risk in subjects, enabling earlier detection and enhanced screening when considering treatment.
Due to the chronic nature of diabetes, patient self-care factors play an important role in any treatment plan. In order to understand the behaviour of patients in response to medical advice on self-care, clinicians often conduct cross-sectional surveys. When analysing the survey data, statistical machine learning methods can potentially provide additional insight into the data either through deeper understanding of the patterns present or making information available to clinicians in an intuitive manner. In this study, we use self-organising maps (SOMs) to visualise the responses of patients who share similar responses to survey questions, with the goal of helping clinicians understand how patients are managing their treatment and where action should be taken. The principle behavioural patterns revealed through this are that: patients who take the correct dose of insulin also tend to take their injections at the correct time, patients who eat on time also tend to correctly manage their food portions and patients who check their blood glucose with a monitor also tend to adjust their insulin dosage and carry snacks to counter low blood glucose. The identification of these positive behavioural patterns can also help to inform treatment by exploiting their negative corollaries.
Early prediction of pathogen infestation is a key factor to reduce the disease spread in plants. Macrophomina phaseolina (Tassi) Goid, as one of the main causes of charcoal rot disease, suppresses the plant productivity significantly. Charcoal rot disease is one of the most severe threats to soybean productivity. Prediction of this disease in soybeans is very tedious and non-practical using traditional approaches. Machine learning (ML) techniques have recently gained substantial traction across numerous domains. ML methods can be applied to detect plant diseases, prior to the full appearance of symptoms. In this paper, several ML techniques were developed and examined for prediction of charcoal rot disease in soybean for a cohort of 2,000 healthy and infected plants. A hybrid set of physiological and morphological features were suggested as inputs to the ML models. All developed ML models were performed better than 90% in terms of accuracy. Gradient Tree Boosting (GBT) was the best performing classifier which obtained 96.25% and 97.33% in terms of sensitivity and specificity. Our findings supported the applicability of ML especially GBT for charcoal rot disease prediction in a real environment. Moreover, our analysis demonstrated the importance of including physiological featured in the learning. The collected dataset and source code can be found in https://github.com/Elham-khalili/Soybean-Charcoal-Rot-Disease-Prediction-Dataset-code.
Algorithms in bioinformatics use textual representations of genetic information, sequences of the characters A, T, G and C represented computationally as strings or sub-strings. Signal and related image processing methods offer a rich source of alternative descriptors as they are designed to work in the presence of noisy data without the need for exact matching. Here we introduce a method, multi-resolution local binary patterns (MLBP) adapted from image processing to extract local 'texture' changes from nucleotide sequence data. We apply this feature space to the alignment-free binning of metagenomic data. The effectiveness of MLBP is demonstrated using both simulated and real human gut microbial communities. Sequence reads or contigs can be represented as vectors and their 'texture' compared efficiently using machine learning algorithms to perform dimensionality reduction to capture eigengenome information and perform clustering (here using randomized singular value decomposition and BH-tSNE). The intuition behind our method is the MLBP feature vectors permit sequence comparisons without the need for explicit pairwise matching. We demonstrate this approach outperforms existing methods based on k-mer frequencies. The signal processing method, MLBP, thus offers a viable alternative feature space to textual representations of sequence data. The source code for our Multi-resolution Genomic Binary Patterns method can be found at https://github.com/skouchaki/MrGBP .
Pre-trained Language Models (LMs) have become an integral part of Natural Language Processing (NLP) in recent years, due to their superior performance in downstream applications. In spite of this resounding success, the usability of LMs is constrained by computational and time complexity, along with their increasing size; an issue that has been referred to as `overparameterisation'. Different strategies have been proposed in the literature to alleviate these problems, with the aim to create effective compact models that nearly match the performance of their bloated counterparts with negligible performance losses. One of the most popular techniques in this area of research is model distillation. Another potent but underutilised technique is cross-layer parameter sharing. In this work, we combine these two strategies and present MiniALBERT, a technique for converting the knowledge of fully parameterised LMs (such as BERT) into a compact recursive student. In addition, we investigate the application of bottleneck adapters for layer-wise adaptation of our recursive student, and also explore the efficacy of adapter tuning for fine-tuning of compact models. We test our proposed models on a number of general and biomedical NLP tasks to demonstrate their viability and compare them with the state-of-the-art and other existing compact models. All the codes used in the experiments are available at https://github.com/nlpie-research/MiniALBERT. Our pre-trained compact models can be accessed from https://huggingface.co/nlpie.
Resistance prediction and mutation ranking are important tasks in the analysis of Tuberculosis sequence data. Due to standard regimens for the use of first-line antibiotics, resistance co-occurrence, in which samples are resistant to multiple drugs, is common. Analysing all drugs simultaneously should therefore enable patterns reflecting resistance co-occurrence to be exploited for resistance prediction. Here, multi-label random forest (MLRF) models are compared with single-label random forest (SLRF) for both predicting phenotypic resistance from whole genome sequences and identifying important mutations for better prediction of four first-line drugs in a dataset of 13402 Mycobacterium tuberculosis isolates. Results confirmed that MLRFs can improve performance compared to conventional clinical methods (by 18.10%) and SLRFs (by 0.91%). In addition, we identified a list of candidate mutations that are important for resistance prediction or that are related to resistance co-occurrence. Moreover, we found that retraining our analysis to a subset of top-ranked mutations was sufficient to achieve satisfactory performance. The source code can be found at .http://www.robots.ox.ac.uk/davidc/code.php.
Resistance co-occurrence within first-line anti-tuberculosis (TB) drugs is a common phenomenon. Existing methods based on genetic data analysis of Mycobacterium tuberculosis (MTB) have been able to predict resistance of MTB to individual drugs, but have not considered the resistance co-occurrence and cannot capture latent structure of genomic data that corresponds to lineages. Results: We used a large cohort of TB patients from 16 countries across six continents where whole-genome sequences for each isolate and associated phenotype to anti-TB drugs were obtained using drug susceptibility testing recommended by the World Health Organization. We then proposed an end-to-end multi-task model with deep denoising auto-encoder (DeepAMR) for multiple drug classification and developed DeepAMR-cluster, a clustering variant based on DeepAMR, for learning clusters in latent space of the data. The results showed that DeepAMR outperformed baseline model and four machine learning models with mean AUROC from 94.4% to 98.7% for predicting resistance to four first-line drugs [i.e. isoniazid (INH), ethambutol (EMB), rifampicin (RIF), pyrazinamide (PZA)], multi-drug resistant TB (MDR-TB) and pan-susceptible TB (PANS-TB: MTB that is susceptible to all four first-line anti-TB drugs). In the case of INH, EMB, PZA and MDR-TB, DeepAMR achieved its best mean sensitivity of 94.3%, 91.5%, 87.3% and 96.3%, respectively. While in the case of RIF and PANS-TB, it generated 94.2% and 92.2% sensitivity, which were lower than baseline model by 0.7% and 1.9%, respectively. t-SNE visualization shows that DeepAMR-cluster captures lineage-related clusters in the latent space. Availability and implementation: The details of source code are provided at http://www.robots.ox.ac.uk/∼davidc/code.php. Supplementary information: Supplementary data are available at Bioinformatics online.
Dementia is a progressive condition that affects cognitive and functional abilities. There is a need for reliable and continuous health monitoring of People Living with Dementia (PLWD) to improve their quality of life and support their independent living. Healthcare services often focus on addressing and treating already established health conditions that affect PLWD. Managing these conditions continuously can inform better decision-making earlier for higher-quality care management for PLWD. The Technology Integrated Health Management (TIHM) project developed a new digital platform to routinely collect longitudinal, observational, and measurement data, within the home and apply machine learning and analytical models for the detection and prediction of adverse health events affecting the well-being of PLWD. This work describes the TIHM dataset collected during the second phase (i.e., feasibility study) of the TIHM project. The data was collected from homes of 56 PLWD and associated with events and clinical observations (daily activity, physiological monitoring, and labels for health-related conditions). The study recorded an average of 50 days of data per participant, totalling 2803 days.
Language models pre-trained on biomedical corpora, such as BioBERT, have recently shown promising results on downstream biomedical tasks. Many existing pre-trained models, on the other hand, are resource-intensive and computationally heavy owing to factors such as embedding size, hidden dimension and number of layers. The natural language processing community has developed numerous strategies to compress these models utilizing techniques such as pruning, quantization and knowledge distillation, resulting in models that are considerably faster, smaller and subsequently easier to use in practice. By the same token, in this article, we introduce six lightweight models, namely, BioDistilBERT, BioTinyBERT, BioMobileBERT, DistilBioBERT, TinyBioBERT and CompactBioBERT which are obtained either by knowledge distillation from a biomedical teacher or continual learning on the Pubmed dataset. We evaluate all of our models on three biomedical tasks and compare them with BioBERT-v1.1 to create the best efficient lightweight models that perform on par with their larger counterparts. We trained six different models in total, with the largest model having 65 million in parameters and the smallest having 15 million; a far lower range of parameters compared with BioBERT's 110M. Based on our experiments on three different biomedical tasks, we found that models distilled from a biomedical teacher and models that have been additionally pre-trained on the PubMed dataset can retain up to 98.8% and 98.6% of the performance of the BioBERT-v1.1, respectively. Overall, our best model below 30 M parameters is BioMobileBERT, while our best models over 30 M parameters are DistilBioBERT and CompactBioBERT, which can keep up to 98.2% and 98.8% of the performance of the BioBERT-v1.1, respectively. Codes are available at: https://github.com/nlpie-research/Compact-Biomedical-Transformers. Trained models can be accessed at: https://huggingface.co/nlpie.
The emergence of drug resistant tuberculosis is a major global public health concern that threatens the ability to control the disease. Whole genome sequencing as a tool to rapidly diagnose resistant infections can transform patient treatment and clinical practice. While resistance mechanisms are well understood for some drugs, there are likely many mechanisms yet to be uncovered, particularly for new and repurposed drugs. We sequenced 10,228 Mycobacterium tuberculosis (MTB) isolates worldwide and determined the minimum inhibitory concentration (MIC) on a grid of twofold concentration dilutions for 13 antimicrobials using quantitative microtiter plate assays. We performed oligopeptide- and oligonucleotide-based genome-wide association studies using linear mixed models to discover resistance-conferring mechanisms not currently catalogued. Use of MIC over binary resistance phenotypes increased heritability for the new and repurposed drugs by 26-37%, increasing our ability to detect novel associations. For all drugs, we discovered uncatalogued variants associated with MIC, including in the Rv1218c promoter binding site of the transcriptional repressor Rv1219c (isoniazid), upstream of the vapBC20 operon that cleaves 23S rRNA (linezolid) and in the region encoding an α-helix lining the active site of Cyp142 (clofazimine, all p
Brain signals arise as a mixture of various neural processes that occur in different spatial, frequency and temporal locations. In detection paradigms, algorithms are developed that target specific processes. In this work, we apply tensor factorisation to a set of intracranial electroencephalography data from a group of epileptic patients and factorise the data into three modes; space, time and frequency with each mode containing a number of components or signatures that are common between the subjects. We train separate classifiers on various feature sets corresponding to complementary combinations of those modes and components. These classifiers are then combined in a leave-subject-out fashion and subsequently used to estimate the classification accuracy of each combination on left-out subjects' data. The relative influence on the classification accuracy of the respective spatial, temporal or frequency signatures can then be analysed and useful interpretations can be made.
10.1093/molbev/msaa069 Molecular Biology and Evolution 37 8 2450-2460
Being able to determine the rank of a symmetric tensor and estimate the number of sources within single channel mixtures are the motives for developing a new approach for decomposition of single channel mixtures. The single channel data is converted to a symmetric tensor and decomposed. As another contribution, the inherent frequency diversity of the time series has been effectively exploited to highlight the subspace of interest. As a useful application, the method has been applied to detect the beta rebound for use in brain computer interfacing.
Tuberculosis is a respiratory disease that is treatable with antibiotics. An increasing prevalence of resistance means that to ensure a good treatment outcome it is desirable to test the susceptibility of each infection to different antibiotics. Conventionally this is done by culturing a clinical sample and then exposing aliquots to a panel of antibiotics, thereby determining the minimum inhibitory concentration (MIC) of each drug. Using 96-well broth micro dilution plates with each well containing a lyophilised pre-determined amount of an antibiotic is a convenient and cost-effective way to measure the MICs of several drugs at once for a clinical sample. Although accurate, this is an expensive and slow process that requires highly-skilled and experienced laboratory scientists. Here we show that, through the BashTheBug project hosted on the Zooniverse citizen science platform, a crowd of volunteers can reproducibly and accurately determine the MICs for 13 drugs and that simply taking the median or mode of 11-17 independent classifications is sufficient. There is therefore a potential role for crowds to support (but not supplant) the role of experts in antibiotic susceptibility testing. Competing Interest Statement The authors have declared no competing interest.
Background: Manual sleep scoring is deemed to be tedious and time consuming. Even among automatic methods such as time-frequency (T-F) representations, there is still room for more improvement. New method: To optimise the efficiency of T-F domain analysis of sleep electroencephalography (EEG) a novel approach for automatically identifying the brain waves, sleep spindles, and IC-complexes from the sleep EEG signals is proposed. The proposed method is based on singular spectrum analysis (SSA). The single-channel EEG signal (C3-A2) is initially decomposed and then the desired components are automatically separated. In addition, the noise is removed to enhance the discrimination ability of features. The obtained T-F features after preprocessing stage are classified using a multi-class support vector machines (SVMs) and used for the identification of four sleep stages over three sleep types. Furthermore, to emphasise on the usefulness of the proposed method the automatically-determined spindles are parameterised to discriminate three sleep types. Result: The four sleep stages are classified through SVM twice: with and without preprocessing stage. The mean accuracy, sensitivity, and specificity for before the preprocessing stage are: 71.5 +/- 0.11%, 56.1 +/- 0.09% and 86.8 +/- 0.04% respectively. However, these values increase significantly to 83.6 +/- 0.07%, 70.6 +/- 0.14% and 90.8 +/- 0.03% after applying SSA. Comparison with existing method: The new T-F representation has been compared with the existing benchmarks. Our results prove that, the proposed method well outperforms the previous methods in terms of identification and representation of sleep stages. Conclusion: Experimental results confirm the performance improvement in terms of classification rate and also representative T-F domain. (C) 2016 Elsevier B.V. All rights reserved.
Tuberculosis is a respiratory disease that is treatable with antibiotics. An increasing prevalence of resistance means that to ensure a good treatment outcome it is desirable to test the susceptibility of each infection to different antibiotics. Conventionally this is done by culturing a clinical sample and then exposing aliquots to a panel of antibiotics, Using 96-well broth micro dilution plates with each well containing a lyophilised predetermined amount of an antibiotic is a convenient and cost-effective way to measure the MICs of several drugs at once for a clinical sample. Although accurate, this is still an expensive and slow process that requires highly skilled and experienced laboratory scientists. Here we show that, through the BashTheBug project hosted on the Zooniverse citizen science platform, a crowd of volunteers can reproducibly and accurately determine the MICs for 13 drugs and that simply taking the median or mode of 11-17 independent classifications is sufficient. There is therefore a potential role for crowds to support (but not supplant) the role of experts in antibiotic susceptibility testing.
Shotgun sequencing has facilitated the analysis of complex microbial communities. Recently we have shown how local binary patterns (LBP) from image processing can be used to analyse the sequenced samples. LBP codes represent the data in a sparse high dimensional space. To improve the performance of our pipeline, marginalised stacked autoencoders are used here to learn frequent LBP codes and map the high dimensional space to a lower dimension dense space. We demonstrate its performance using both low and high complexity simulated metagenomic data and compare the performance of our method with several existing techniques including principal component analysis (PCA) in the dimension reduction step and fc-mer frequency in feature extraction step.
Motivation Timely identification of Mycobacterium tuberculosis (MTB) resistance to existing drugs is vital to decrease mortality and prevent the amplification of existing antibiotic resistance. Machine learning methods have been widely applied for timely predicting resistance of MTB given a specific drug and identifying resistance markers. However, they have been not validated on a large cohort of MTB samples from multi-centers across the world in terms of resistance prediction and resistance marker identification. Several machine learning classifiers and linear dimension reduction techniques were developed and compared for a cohort of 13 402 isolates collected from 16 countries across 6 continents and tested 11 drugs. Results Compared to conventional molecular diagnostic test, area under curve of the best machine learning classifier increased for all drugs especially by 23.11%, 15.22% and 10.14% for pyrazinamide, ciprofloxacin and ofloxacin, respectively (P
In this study, a novel hybrid tensor factorisation and deep learning approach has been proposed and implemented for sleep pose identification and classification of twelve different sleep postures. We have applied tensor factorisation to infrared (IR) images of 10 subjects to extract group-level data patterns, undertake dimensionality reduction and reduce occlusion for IR images. Pre-trained VGG-19 neural network has been used to predict the sleep poses under the blanket. Finally, we compared our results with those without the factorisation stage and with CNN network. Our new pose detection method outperformed the methods solely based on VGG-19 and 4-layer CNN network. The average accuracy for 10 volunteers increased from 78.1% and 75.4% to 86.0%.
Shotgun sequencing has facilitated the analysis of complex microbial communities. However, clustering and visualising these communities without prior taxonomic information is a major challenge. Feature descriptor methods can be utilised to extract these taxonomic relations from the data. Here, we present a novel approach consisting of local binary patterns (LBP) coupled with randomised singular value decomposition (RSVD) and Barnes-Hut t-stochastic neighbor embedding (BH-tSNE) to highlight the underlying taxonomic structure of the metagenomic data. The effectiveness of our approach is demonstrated using several simulated and a real metagenomic datasets.
Security concerns give biometrics an important role in security solutions. There is strong evidence that we can use electrocardiogram (ECG) signals to identify individuals. In other words, they contain sufficient discriminative information to allow the identification of individuals from a large population. Therefore, this paper presents an individual identification system using 20 healthy subjects from Physikalisch-Technische Bundesanstalt (PTB) database. In this way, Empirical mode decomposition (EMD) is used to decompose our signals to their base component. Then, the instantaneous frequency of the last component is computed by using Hilbert transform. Finally, 1 nearest neighbor (1NN) classifier is utilized to identify the accuracy of our method. With this procedure, we obtained a high identification rate (93.22%).
Measuring mental fatigue is essential in assessing the performance of those subjects whose careers involve severe mental activity. Recently, many analytical methods have been applied to electroencephalograms (EEGs) in order to quantitatively detect the fatigue state, but their accuracy is still not satisfactory. Factorization methods have been employed in our study to extract fatigue-related features from information captured from the ongoing raw EEG signals. The EEG signals were recorded from 32 channels from 17 healthy subjects before and after 3 h of severe mental activity. After preprocessing the raw EEGs, it was arranged in matrices to be decomposed by non-negative methods named NMF, LNMF, SNMF, DNMF, NTF, and DNTF. A comparative study of the methods was carried out by using support vector machine (SVM) (Sameni et al. in IEEE Trans Signal Process 58:2363-2374, 2010; Kadirgama et al. in Arab J Sci Eng 37:2269-2275, 2012) with extracted discriminative subspaces in order to classify raw EEGs into two "mental states" (fatigued/not fatigued). Experimental results demonstrated that discriminant DNTF outperformed (p < 0.05) the other compared non-negative methods in terms of accuracy, feature storage, and robustness.
The Comprehensive Resistance Prediction for Tuberculosis: an International Consortium (CRyPTIC) presents here a compendium of 15,211 Mycobacterium tuberculosis global clinical isolates, all of which have undergone whole genome sequencing (WGS) and have had their minimum inhibitory concentrations to 13 antitubercular drugs measured in a single assay. It is the largest matched phenotypic and genotypic dataset for M. tuberculosis to date. Here, we provide a summary detailing the breadth of data collected, along with a description of how the isolates were collected and uniformly processed in CRyPTIC partner laboratories across 23 countries. The compendium contains 6,814 isolates resistant to at least one drug, including 2,129 samples that fully satisfy the clinical definitions of rifampicin resistant (RR), multi-drug resistant (MDR), pre-extensively drug resistant (pre-XDR) or extensively drug resistant (XDR). Accurate prediction of resistance status (sensitive/resistant) to eight antitubercular drugs by using a genetic mutation catalogue is presented along with the presence of suspected resistance-conferring mutations for isolates resistant to the newly introduced drugs bedaquiline, clofazimine, delamanid and linezolid. Finally, a case study of rifampicin mono-resistance demonstrates how this compendium could be used to advance our genetic understanding of rare resistance phenotypes. The compendium is fully open-source and it is hoped that the dataset will facilitate and inspire future research for years to come. Competing Interest Statement E.R. is employed by Public Health England and holds an honorary contract with Imperial College London. I.F.L. is Director of the Scottish Mycobacteria Reference Laboratory. S.N. receives funding from German Center for Infection Research, Excellenz Cluster Precision Medicine in Chronic Inflammation, Leibniz Science Campus Evolutionary Medicine of the LUNG (EvoLUNG)tion EXC 2167. P.S. is a consultant at Genoscreen. T.R. is funded by NIH and DoD and receives salary support from the non-profit organization FIND. T.R. is a co-founder, board member and shareholder of Verus Diagnostics Inc, a company that was founded with the intent of developing diagnostic assays. Verus Diagnostics was not involved in any way with data collection, analysis or publication of the results. T.R. has not received any financial support from Verus Diagnostics. UCSD Conflict of Interest office has reviewed and approved T.R.s role in Verus Diagnostics Inc. T.R. is a co-inventor of a provisional patent for a TB diagnostic assay (provisional patent #: 63/048.989). T.R. is a co-inventor on a patent associated with the processing of TB sequencing data (European Patent Application No. 14840432.0 & USSN 14/912,918). T.R. has agreed to donate all present and future interest in and rights to royalties from this patent to UCSD to ensure that he does not receive any financial benefits from this patent. S.S. is working and holding ESOPs at HaystackAnalytics Pvt. Ltd. (Product: Using whole genome sequencing for drug susceptibility testing for Mycobacterium tuberculosis). G.F.G. is listed as an inventor on patent applications for RBD-dimer-based CoV vaccines. The patents for RBD-dimers as protein subunit vaccines for SARS-CoV-2 have been licensed to Anhui Zhifei Longcom Biopharmaceutical Co. Ltd, China. Footnotes * Updates to the manuscript include re-arrangement of figures and main text editing.
In this paper common spatial patterns filter has been combined with conventional PARAFAC2 tensor decomposition in the design of a new spatially constrained source separation system. This approach is particularly useful in separation of weak intermittent signal components such as interictal discharges originated from deep brain sources. The results of applying the method to synthetic data show that it outperforms conventional blind source separation methods which are often unable to separate weak intermittent nonstationary sources.
Traditional authentication mechanisms use passwords, Personal Identification Numbers (PINs) and biometrics, but these only authenticate at the point of entry. Continuous authentication schemes instead allow systems to verify identity and mitigate unauthorised access continuously. However, recent developments in generative modelling can significantly threaten continuous authentication systems, allowing attackers to craft adversarial examples to gain unauthorised access and may even limit a legitimate user from accessing protected data in the network. The research available on the use of generative models for attacking continuous authentication is relatively scarce. This paper explores the feasibility of bypassing continuous authentication using generative models, measuring the impact of the damage, and advising the usage of metrics to compare the various advertised attacks in such a system. Our empirical results demonstrate that generative models cause a higher Equal Error Rate and misclassification error in attack scenarios. At the same time, training and detection time during attack scenarios is increased compared to perturbation models. The results prove that data samples crafted by generative models can be a severe threat to continuous authentication schemes using motion sensor data.
Background Sleep disturbances are both risk factors for and symptoms of dementia. Current methods for assessing sleep disturbances are largely based on either polysomnography (PSG) which is costly and inconvenient, or self‐ or care‐giver reports which are prone to measurement error. Low‐cost methods to monitor sleep disturbances longitudinally and at scale can be useful for assessing symptom development. Here, we develop deep learning models that use multimodal variables (accelerometers and temperature) recorded by the AX3 to accurately identify sleep and wake epochs and derive sleep parameters. Method Eighteen men and women (65‐80y) participated in a sleep laboratory‐based study in which multiple devices for sleep monitoring were evaluated. PSGs were recorded over a 10‐h period and scored according to established criteria per 30 sec epochs. Tri‐axial accelerometers and temperature signals were captured with an Axivity AX3, at 100Hz and 1Hz, respectively, throughout a 19‐h period, including 10‐h concurrent PSG recording and 9‐h of wakefulness. We developed and evaluated a supervised deep learning algorithm to detect sleep and wake epochs and determine sleep parameters from the multimodal AX3 raw data. We validated our results with gold standard PSG measurements and compared our algorithm to the Biobank accelerometer analysis toolbox. Single modality (accelerometer or temperature) and multimodality (both signals) approaches were evaluated using the 3‐fold cross‐validation. Result The proposed deep learning model outperformed baseline models such as the Biobank accelerometer analysis toolbox and conventional machine learning classifiers (Random Forest and Support Vector Machine) by up to 25%. Using multimodal data improved sleep and wake classification performance (up to 18% higher) compared with the single modality. In terms of the sleep parameters, our approach boosted the accuracy of estimations by 11% on average compared to the Biobank accelerometer analysis toolbox. Conclusion In older adults without dementia, combining multimodal data from AX3 with deep learning methods allows satisfactory quantification of sleep and wakefulness. This approach holds promise for monitoring sleep behaviour and deriving accurate sleep parameters objectively and longitudinally from a low‐cost wearable sensor. A limitation of our current study is that the participants were healthy older adults: future work will focus on people living with dementia.
The d(N)/d(S) ratio provides evidence of adaptation or functional constraint in protein-coding genes by quantifying the relative excess or deficit of amino acid-replacing versus silent nucleotide variation. Inexpensive sequencing promises a better understanding of parameters, such as d(N)/d(S), but analyzing very large data sets poses a major statistical challenge. Here, I introduce genomegaMap for estimating within-species genome-wide variation in d(N)/d(S), and I apply it to 3,979 genes across 10,209 tuberculosis genomes to characterize the selection pressures shaping this global pathogen. GenomegaMap is a phylogeny-free method that addresses two major problems with existing approaches: 1) It is fast no matter how large the sample size and 2) it is robust to recombination, which causes phylogenetic methods to report artefactual signals of adaptation. GenomegaMap uses population genetics theory to approximate the distribution of allele frequencies under general, parent-dependent mutation models. Coalescent simulations show that substitution parameters are well estimated even when genomegaMap's simplifying assumption of independence among sites is violated. I demonstrate the ability of genomegaMap to detect genuine signatures of selection at antimicrobial resistance-conferring substitutions in Mycobacterium tuberculosis and describe a novel signature of selection in the cold-shock DEAD-box protein A gene deaD/csdA. The genomegaMap approach helps accelerate the exploitation of big data for gaining new insights into evolution within species.
It is evident that the electroencephalogram (EEG) rhythms are slightly changed when the efficacy of mental activity declines (brain fatigue). Nonetheless, this slight change is not easily detectable by the so far suggested scalp EEG features. The goal of this paper is to propose an EEG-based biomarker, which has a congruity to the mental fatigue variation to detect the transition from non-fatigue to the fatigue mental state. The strength of the dominant EEG source, extracted by minimum variance beamformer (MVB), is proposed here as a discriminative feature to remarkably classify the two mental states. To assess the proposed scheme, EEG signals of 17 volunteers were recorded via 32 electrodes before and after taking an exhausting mental exam (3 h) and the extracted EEG features were labeled as non-fatigue and fatigue, respectively. After removing the eye-blink effect, the proposed feature along with the conventional EEG features were extracted from the recorded EEGs and then applied to support vector machine (SVM) and 1-nearest neighbor (1NN) classifiers in order to differentiate these two mental states. The best result is achieved by applying the proposed feature to the SVM classifier providing 97.06% classification accuracy which is significantly (p < 0.05) superior to its counter parts.
Purpose: Sleep is a complex physiological state and an indicator of the changes in the brain function similar to those occurring in many psychiatric and neurological conditions. Since visual sleep scoring consuming process, automatic sleep staging methods, also called scoring, hold promise in diagnosing alterations in the sleep process and the sleep EEG more effectively. Method: In this paper, a supervised approach for sleep scoring from single channel EEG signals is proposed. First, a supervised singular spectrum analysis (SSA) which is a subspace based method is used to extract the desired signal for each stage of sleep. Then, two recursive least squares (RLS) adaptive filters are trained and used to identify first and deep sleep stages. Result: The proposed system which can be considered as a filter bank for separating multiple signal subbands is tested using real EEG where the results verify the accuracy of the proposed method. Conclusion: The overall result show the effectiveness of algorithm for detection of sleep stages from EEG signals often characterised by a sharp increase in delta and a rapid decrease in alpha as sleep deepens.
Bedaquiline is a core drug for the treatment of multidrug-resistant tuberculosis; however, the understanding of resistance mechanisms is poor, which is hampering rapid molecular diagnostics. Some bedaquiline-resistant mutants are also cross-resistant to clofazimine. To decipher bedaquiline and clofazimine resistance determinants, we combined experimental evolution, protein modelling, genome sequencing, and phenotypic data. For this in-vitro and in-silico data analysis, we used a novel in-vitro evolutionary model using subinhibitory drug concentrations to select bedaquiline-resistant and clofazimine-resistant mutants. We determined bedaquiline and clofazimine minimum inhibitory concentrations and did Illumina and PacBio sequencing to characterise selected mutants and establish a mutation catalogue. This catalogue also includes phenotypic and genotypic data of a global collection of more than 14 000 clinical Mycobacterium tuberculosis complex isolates, and publicly available data. We investigated variants implicated in bedaquiline resistance by protein modelling and dynamic simulations. We discerned 265 genomic variants implicated in bedaquiline resistance, with 250 (94%) variants affecting the transcriptional repressor (Rv0678) of the MmpS5-MmpL5 efflux system. We identified 40 new variants in vitro, and a new bedaquiline resistance mechanism caused by a large-scale genomic rearrangement. Additionally, we identified in vitro 15 (7%) of 208 mutations found in clinical bedaquiline-resistant isolates. From our in-vitro work, we detected 14 (16%) of 88 mutations so far identified as being associated with clofazimine resistance and also seen in clinically resistant strains, and catalogued 35 new mutations. Structural modelling of Rv0678 showed four major mechanisms of bedaquiline resistance: impaired DNA binding, reduction in protein stability, disruption of protein dimerisation, and alteration in affinity for its fatty acid ligand. Our findings advance the understanding of drug resistance mechanisms in M tuberculosis complex strains. We have established an extended mutation catalogue, comprising variants implicated in resistance and susceptibility to bedaquiline and clofazimine. Our data emphasise that genotypic testing can delineate clinical isolates with borderline phenotypes, which is essential for the design of effective treatments. Leibniz ScienceCampus Evolutionary Medicine of the Lung, Deutsche Forschungsgemeinschaft, Research Training Group 2501 TransEvo, Rhodes Trust, Stanford University Medical Scientist Training Program, National Institute for Health and Care Research Oxford Biomedical Research Centre, Oxford University Hospitals NHS Foundation Trust, Bill & Melinda Gates Foundation, Wellcome Trust, and Marie Skłodowska-Curie Actions.
The World Health Organization goal of universal drug susceptibility testing for patients with tuberculosis is most likely to be achieved through molecular diagnostics; however, to date these have focused largely on first-line drugs, and always on predicting binary susceptibilities. Here, we used whole genome sequencing and a quantitative microtiter plate assay to relate genomic mutations to minimum inhibitory concentration in 15,211 Mycobacterium tuberculosis patient isolates from 27 countries across five continents. This work identifies 449 unique MIC-elevating genetic determinants across thirteen drugs, as well as 91 mutations resulting in hypersensitivity for eleven drugs. Our results provide a guide for further implementation of personalized medicine for the treatment of tuberculosis using genetics-based diagnostics and can serve as a training set for novel approaches to predict drug resistance. Competing Interest Statement E.R. is employed by Public Health England and holds an honorary contract with Imperial College London. I.F.L. is Director of the Scottish Mycobacteria Reference Laboratory. S.N. receives funding from German Center for Infection Research, Excellenz Cluster Precision Medicine in Chronic Inflammation, Leibniz Science Campus Evolutionary Medicine of the LUNG (EvoLUNG)tion EXC 2167. P.S. is a consultant at Genoscreen. T.R. is funded by NIH and DoD and receives salary support from the non-profit organization FIND. T.R. is a co-founder, board member and shareholder of Verus Diagnostics Inc, a company that was founded with the intent of developing diagnostic assays. Verus Diagnostics was not involved in any way with data collection, analysis or publication of the results. T.R. has not received any financial support from Verus Diagnostics. UCSD Conflict of Interest office has reviewed and approved T.R.s role in Verus Diagnostics Inc. T.R. is a co-inventor of a provisional patent for a TB diagnostic assay (provisional patent #: 63/048.989). T.R. is a co-inventor on a patent associated with the processing of TB sequencing data (European Patent Application No. 14840432.0 & USSN 14/912,918). T.R. has agreed to donate all present and future interest in and rights to royalties from this patent to UCSD to ensure that he does not receive any financial benefits from this patent. S.S. is working and holding ESOPs at HaystackAnalytics Pvt. Ltd. (Product: Using whole genome sequencing for drug susceptibility testing for Mycobacterium tuberculosis). Footnotes * Article shortened to meet journal submission requirements.
The early clinical course of COVID-19 can be difficult to distinguish from other illnesses driving presentation to hospital. However, viral-specific PCR testing has limited sensitivity and results can take up to 72 h for operational reasons. We aimed to develop and validate two early-detection models for COVID-19, screening for the disease among patients attending the emergency department and the subset being admitted to hospital, using routinely collected health-care data (laboratory tests, blood gas measurements, and vital signs). These data are typically available within the first hour of presentation to hospitals in high-income and middle-income countries, within the existing laboratory infrastructure. We trained linear and non-linear machine learning classifiers to distinguish patients with COVID-19 from pre-pandemic controls, using electronic health record data for patients presenting to the emergency department and admitted across a group of four teaching hospitals in Oxfordshire, UK (Oxford University Hospitals). Data extracted included presentation blood tests, blood gas testing, vital signs, and results of PCR testing for respiratory viruses. Adult patients (>18 years) presenting to hospital before Dec 1, 2019 (before the first COVID-19 outbreak), were included in the COVID-19-negative cohort; those presenting to hospital between Dec 1, 2019, and April 19, 2020, with PCR-confirmed severe acute respiratory syndrome coronavirus 2 infection were included in the COVID-19-positive cohort. Patients who were subsequently admitted to hospital were included in their respective COVID-19-negative or COVID-19-positive admissions cohorts. Models were calibrated to sensitivities of 70%, 80%, and 90% during training, and performance was initially assessed on a held-out test set generated by an 80:20 split stratified by patients with COVID-19 and balanced equally with pre-pandemic controls. To simulate real-world performance at different stages of an epidemic, we generated test sets with varying prevalences of COVID-19 and assessed predictive values for our models. We prospectively validated our 80% sensitivity models for all patients presenting or admitted to the Oxford University Hospitals between April 20 and May 6, 2020, comparing model predictions with PCR test results. We assessed 155 689 adult patients presenting to hospital between Dec 1, 2017, and April 19, 2020. 114 957 patients were included in the COVID-negative cohort and 437 in the COVID-positive cohort, for a full study population of 115 394 patients, with 72 310 admitted to hospital. With a sensitive configuration of 80%, our emergency department (ED) model achieved 77·4% sensitivity and 95·7% specificity (area under the receiver operating characteristic curve [AUROC] 0·939) for COVID-19 among all patients attending hospital, and the admissions model achieved 77·4% sensitivity and 94·8% specificity (AUROC 0·940) for the subset of patients admitted to hospital. Both models achieved high negative predictive values (NPV; >98·5%) across a range of prevalences (≤5%). We prospectively validated our models for all patients presenting and admitted to Oxford University Hospitals in a 2-week test period. The ED model (3326 patients) achieved 92·3% accuracy (NPV 97·6%, AUROC 0·881), and the admissions model (1715 patients) achieved 92·5% accuracy (97·7%, 0·871) in comparison with PCR results. Sensitivity analyses to account for uncertainty in negative PCR results improved apparent accuracy (ED model 95·1%, admissions model 94·1%) and NPV (ED model 99·0%, admissions model 98·5%). Our models performed effectively as a screening test for COVID-19, excluding the illness with high-confidence by use of clinical data routinely available within 1 h of presentation to hospital. Our approach is rapidly scalable, fitting within the existing laboratory testing infrastructure and standard of care of hospitals in high-income and middle-income countries. Wellcome Trust, University of Oxford, Engineering and Physical Sciences Research Council, National Institute for Health Research Oxford Biomedical Research Centre.
Sensor-based remote health monitoring is used in industrial, urban and healthcare settings to monitor ongoing operation of equipment and human health. An important aim is to intervene early if anomalous events or adverse health is detected. In the wild, these anomaly detection approaches are challenged by noise, label scarcity, high dimensionality, explainability and wide variability in operating environments. The Contextual Matrix Profile (CMP) is a configurable 2-dimensional version of the Matrix Profile (MP) that uses the distance matrix of all subsequences of a time series to discover patterns and anomalies. The CMP is shown to enhance the effectiveness of the MP and other SOTA methods at detecting, visualising and interpreting true anomalies in noisy real world data from different domains. It excels at zooming out and identifying temporal patterns at configurable time scales. However, the CMP does not address cross-sensor information, and cannot scale to high dimensional data. We propose a novel, self-supervised graph- based approach for temporal anomaly detection that works on context graphs generated from the CMP distance matrix. The learned graph embeddings encode the anomalous nature of a time context. In addition, we evaluate other graph outlier algorithms for the same task. Given our pipeline is modular, graph construction, generation of graph embeddings, and pattern recognition logic can all be chosen based on the specific pattern detection application.We verified the effectiveness of graph-based anomaly detection and compared it with the CMP and 3 state-of-the art methods on two real-world healthcare datasets with different anomalies. Our proposed method demonstrated better recall, alert rate and generalisability.
The emergence of drug-resistant tuberculosis is a major global public health concern that threatens the ability to control the disease. Whole-genome sequencing as a tool to rapidly diagnose resistant infections can transform patient treatment and clinical practice. While resistance mechanisms are well understood for some drugs, there are likely many mechanisms yet to be uncovered, particularly for new and repurposed drugs. We sequenced 10,228 Mycobacterium tuberculosis (MTB) isolates worldwide and determined the minimum inhibitory concentration (MIC) on a grid of 2-fold concentration dilutions for 13 antimicrobials using quantitative microtiter plate assays. We performed oligopeptide- and oligonucleotidebased genome-wide association studies using linear mixed models to discover resistance-conferring mechanisms not currently catalogued. Use of MIC over binary resistance phenotypes increased sample heritability for the new and repurposed drugs by 26% to 37%, increasing our ability to detect novel associations. For all drugs, we discovered uncatalogued variants associated with MIC, including in the Rv1218c promoter binding site of the transcriptional repressor Rv1219c (isoniazid), upstream of the vapBC20 operon that cleaves 23S rRNA (linezolid) and in the region encoding an a- helix lining the active site of Cyp142 (clofazimine, all p < 10- 7.7). We observed that artefactual signals of cross-resistance could be unravelled based on the relative effect size on MIC. Our study demonstrates the ability of very large-scale studies to substantially improve our knowledge of genetic variants associated with antimicrobial resistance in M. tuberculosis.
The Comprehensive Resistance Prediction for Tuberculosis: an International Consortium (CRyPTIC) presents here a data compendium of 12,289 Mycobacterium tuberculosis global clinical isolates, all of which have undergone whole-genome sequencing and have had their minimum inhibitory concentrations to 13 antitubercular drugs measured in a single assay. It is the largest matched phenotypic and genotypic dataset for M . tuberculosis to date. Here, we provide a summary detailing the breadth of data collected, along with a description of how the isolates were selected, collected, and uniformly processed in CRyPTIC partner laboratories across 23 countries. The compendium contains 6,814 isolates resistant to at least 1 drug, including 2,129 samples that fully satisfy the clinical definitions of rifampicin resistant (RR), multidrug resistant (MDR), pre-extensively drug resistant (pre-XDR), or extensively drug resistant (XDR). The data are enriched for rare resistance-associated variants, and the current limits of genotypic prediction of resistance status (sensitive/resistant) are presented by using a genetic mutation catalogue, along with the presence of suspected resistance-conferring mutations for isolates resistant to the newly introduced drugs bedaquiline, clofazimine, delamanid, and linezolid. Finally, a case study of rifampicin monoresistance demonstrates how this compendium could be used to advance our genetic understanding of rare resistance phenotypes. The data compendium is fully open source and it is hoped that it will facilitate and inspire future research for years to come.
Recently, several attempts have been made to find the depth of anesthesia (DOA) by analyzing the ongoing electroencephalogram (EEG) signals during surgical operations. Nevertheless, specialists still do not rely on these indexes because they cannot accurately track the transitions of anesthetic depth. This paper presents an effective EEG-based index that is fast to compute and acts very accurate in practice. To determine the proposed index, first EEG signals are denoised with an adaptive thresholding method. The wavelet transform is then applied to the clean EEG signals in order to decompose the signal into brain-match subspaces and the proposed feature extracted from each subspace to monitor the DOA. EEG signals of 8 subjects were recorded during the surgical operation. Experimental results exhibit the proposed features highly correlated with the BIS index (the most popular EEG-based index)through different anesthetic levels. Moreover, in some cases the introduced index outperformed the BIS and the clinical observation confirmed this superiority.
A novel quaternion-valued singular spectrum analysis (SSA) is introduced for multichannel analysis of electroencephalogram (EEG). The analysis of EEG typically requires the decomposition of data channels into meaningful components despite the notoriously noisy nature of EEG-which is the aim of SSA. However, the singular value decomposition involved in SSA implies the strict orthogonality of the decomposed components, which may not reflect accurately the sources which exhibit similar neural activities. To allow for the modelling of such co-channel coupling, the quaternion domain is considered for the first time to formulate the SSA using the augmented statistics. As an application, we demonstrate how the augmented quaternion-valued SSA (AQSSA) can be used to extract the sources, even at a signal-to-noise ratio as low as -10 dB. To illustrate the usefulness of our quaternion-valued SSA in a rehabilitation setting, we employ the proposed SSA for sleep analysis to extract statistical descriptors for five-stage classification (Awake, N1, N2, N3 and REM). The level of agreement using these descriptors was 74% as quantified by the Cohen's kappa.
Background Sensor‐based remote health monitoring of persons living with dementia (PLwD) can be used to gain insights into their health and monitor the progression of their condition, with minimal intrusion. This helps minimize preventable hospital admissions, while allowing researchers to improve their understanding of dementia. Existing approaches for detecting activity and behavioural anomalies in PLwD are challenged by noise in data, lack of annotated datasets, multivariate data, scalability, data drift and explainability. Method We propose and evaluate a solution based on the Matrix Profile, an exact, ultra‐fast distance‐based anomaly detection algorithm, specifically the Contextual Matrix Profile (CMP), to detect anomalies that may indicate unusual activity and onset of UTI. Daily household movement data collected via passive infrared (PIR) sensors are used to generate CMPs from location‐wise sensor counts, duration and change in hourly movement patterns. We create CMP‐based multivariate anomaly detection models to generate a single daily normalized anomaly score for each patient. We discover digital biomarkers of anomalies and evaluate our method vs. three state‐of‐the‐art algorithms. Result CMP‐based models yield up to 85% recall with only a 5% alert rate, when evaluated on a subset of 9363 days from 15 participant households with 41 clinically validated incidences of urinary tract infections (UTI) and hospitalization, collected by the UK Dementia Research Institute between August 2019 and July 2021. Our multidimensional CMP model offers the best balance of recall vs. anomalies raised, with excellent generalisation. We discover that bathroom early AM activity (midnight to 6 am) is the prime cross‐patient digital biomarker of anomalies. This validates findings in literature that unusual bathroom activity is a clinically significant feature in UTI for dementia. We also demonstrate a cross‐patient view of anomaly patterns. Conclusion We address the need for anomaly detection and scoring using multivariate time series sensor data in remote health monitoring. The CMP allows configurability, ability to denoise and detect patterns, and explainability to clinical practitioners. With higher sensitivity, fewer alerts and better overall performance than state‐of‐the‐art methods, and the ability to discover digital biomarkers of anomalies, the CMP is a clinically meaningful unsupervised anomaly detection technique for dementia and beyond.
Advances in DNA sequencing technology are facilitating genomic analyses of unprecedented scope and scale, widening the gap between our abilities to generate and fully exploit biological sequence data. Comparable analytical challenges are encountered in other data-intensive fields involving sequential data, such as signal processing, in which dimensionality reduction (i.e., compression) methods are routinely used to lessen the computational burden of analyses. In this work we explore the application of dimensionality reduction methods to numerically represent high-throughput sequence data for three important biological applications of virus sequence data: reference-based mapping, short sequence classification and de novo assembly. Despite using highly compressed sequence transformations to accelerate the processes, our sequence processing approach yielded comparable accuracy to existing approaches, and are ideally suited for sequences originating from highly diverse virus populations. We demonstrate the application of our methodology to both synthetic and real viral pathogen sequence data. Our results show that the use of highly compressed sequence approximations can provide accurate results and that useful analytical performance can be retained and even enhanced through appropriate dimensionality reduction of sequence data.
Electroencephalography (EEG) signals arise as mixtures of various neural processes which occur in particular spatial, frequency, and temporal brain locations. In classification paradigms, algorithms are developed that can distinguish between these processes. In this work, we apply tensor factorisation to a set of EEG data from a group of epileptic patients and factorise the data into three modes; space, time, and frequency with each mode containing a number of components or signatures. We train separate classifiers on various feature sets corresponding to complementary combinations of those modes and components and test the classification accuracy for each set. The relative influence on the classification accuracy of the respective spatial, temporal, or frequency signatures can then be analysed and useful interpretations can be made. Additionaly, we show that through tensor factorisation we can perform dimensionality reduction by evaluating the classification performance with regards to the number of components in each mode and also by rejecting components with insignificant contribution to the classification accuracy.
It is evident that biological signals of each subject (e.g., electrocardiogram signal) carry his/her unique signature; consequently, several attempts have been made to extract subject-dependent features from these signals with application to human verification. Despite numerous efforts to characterize electrocardiogram (ECG) signals and provide promising results for low population of subjects, the performance of state-of-the-art methods mostly fail in the presence of noise or arrhythmia. This paper presented an efficient and fast-to-compute ECG feature by applying empirical mode decomposition (EMD) to ECG signals, and then, instantaneous frequency, instantaneous phase, amplitude, and entropy features were extracted from the analytical form of the last EMD component. Finally, the k-nearest neighbor (kNN) classifier was utilized to classify the individuals' features. The proposed method was compared to the conventional features such as fiducial points, correlation, wavelet coefficients, and principal component analysis (PCA). These methods were all applied to ECG signals of 34 healthy subjects derived from the Physikalisch-Technische Bundesanstalt (PTB) database. The results implied the effectiveness of the proposed method, providing 95% verification accuracy, which was not the best among the competitors but provided much lower dimensional feature space compared to the top-rank counterparts.
Complex tensor factorisation of correlated brain sources is addressed in this paper. The electrical brain responses due to motory, sensory, or cognitive stimuli, i.e. event related potentials (ERPs), particularly P300, have been used for cognitive information processing. P300 has two subcomponents, P3a and P3b which are correlated and therefore, the traditional blind source separation approaches cannot solve the problem. In this work, a complex-valued tensor factorisation of electroencephalography (EEG) signals is introduced with the aim of separating P300 subcomponents. The proposed method uses complex-valued statistics to exploit the data correlation. In this way, the variations of P3a and p3b can be tracked for the assessment of the brain state. The results of this work will be compared with those of spatial principal component analysis (SPCA) method.
Discrimination of neuromuscular diseases based on electromyogram (EMG) is still a hot topic among the rehabilitation society. Although many attempts have been made to elicit informative features from the discretized EMG signals, traditional visual inspection is still their gold-standard method. Therefore, this paper is aimed at introducing an effective combinational feature to enhance the classification rate among the control group and subjects with neuropathy and myopathy diseases. All EMG signals were artificially simulated, by incorporating statistical and morphological properties of each group into their signal models, in the EMG laboratory of Waterloo University. To classify the subjects by the proposed method, first, EMG signals are decomposed by empirical mode decomposition (EMD) to its natural subspaces, then number of subspaces is aligned through all windowed signals, and Kolmogorov Complexity (KC) and other informative feature are determined to reveal the amount of irregularity within each subspace. Finally, these features are applied to support vector machine (SVM). Experimental results show our method can differentiate these three groups efficiently.
In many subspace signal decomposition methods such as principal component analysis (PCA) or its extension, singular spectrum analysis (SSA), particularly meant for processing of single channel signals, there is need for a robust determination and validation of the number of sources. Here, we attempt to find a relation between the number of sources within single channel mixtures and the rank of a symmetric tensor constructed from such mixtures by adjusting the embedding dimension. This leads to a new approach for decomposition of single channel mixtures using tensor factorisation. Consequently, the effect of model order is analysed for simulated narrowband data. The inherent frequency diversity of the time series has also been effectively exploited in selection of the desired subspace. The proposed method has been applied to both simulated and real data. The results have been discussed and compared with those of a number of benchmark algorithms.
In this study, a novel sleep pose identification method has been proposed for classifying 12 different sleep postures using a two-step deep learning process. For this purpose, transfer learning as an initial stage retrains a well-known CNN network (VGG-19) to categorise the data into four main pose classes, namely: supine, left, right, and prone. According to the decision made by VGG-19, subsets of the image data are next passed to one of four dedicated sub-class CNNs. As a result, the pose estimation label is further refined from one of four sleep pose labels to one of 12 sleep pose labels. 10 participants contributed for recording infrared (IR) images of 12 pre-defined sleep positions. Participants were covered by a blanket to occlude the original pose and present a more realistic sleep situation. Finally, we have compared our results with (1) the traditional CNN learning from scratch and (2) retrained VGG-19 network in one stage. The average accuracy increased from 74.5% & 78.1% to 85.6% compared with (1) & (2) respectively.
Early detection of COVID-19 is an ongoing area of research that can help with triage, monitoring and general health assessment of potential patients and may reduce operational strain on hospitals that cope with the coronavirus pandemic. Different machine learning techniques have been used in the literature to detect potential cases of coronavirus using routine clinical data (blood tests, and vital signs measurements). Data breaches and information leakage when using these models can bring reputational damage and cause legal issues for hospitals. In spite of this, protecting healthcare models against leakage of potentially sensitive information is an understudied research area. In this study, two machine learning techniques that aim to predict a patient's COVID-19 status are examined. Using adversarial training, robust deep learning architectures are explored with the aim to protect attributes related to demographic information about the patients. The two models examined in this work are intended to preserve sensitive information against adversarial attacks and information leakage. In a series of experiments using datasets from the Oxford University Hospitals (OUH), Bedfordshire Hospitals NHS Foundation Trust (BH), University Hospitals Birmingham NHS Foundation Trust (UHB), and Portsmouth Hospitals University NHS Trust (PUH), two neural networks are trained and evaluated. These networks predict PCR test results using information from basic laboratory blood tests, and vital signs collected from a patient upon arrival to the hospital. The level of privacy each one of the models can provide is assessed and the efficacy and robustness of the proposed architectures are compared with a relevant baseline. One of the main contributions in this work is the particular focus on the development of effective COVID-19 detection models with built-in mechanisms in order to selectively protect sensitive attributes against adversarial attacks. The results on hold-out test set and external validation confirmed that there was no impact on the generalisibility of the model using adversarial learning.
Drug susceptibility testing of M. tuberculosis is rooted in a binary susceptible/resistant paradigm. While there are considerable advantages in measuring the minimum inhibitory concentrations (MICs) of a panel of drugs for an isolate, it is necessary to measure the epidemiological cut-off values (ECOFF/ECVs) to permit comparison with qualitative data. Here we present ECOFF/ECVs for 13 anti-tuberculosis compounds, including bedaquiline and delamanid, derived from 20 637 clinical isolates collected by 14 laboratories based in 11 countries on five continents. Each isolate was incubated for 14 days on a dry 96-well broth microdilution plate and then read. Resistance to most of the drugs due to prior exposure is expected and the MIC distributions for many of the compounds are complex, and therefore a phenotypically wild-type population could not be defined. Since a majority of samples also underwent genetic sequencing, we defined a genotypically wild-type population and measured the MIC of the 99th percentile by direct measurement and via fitting a Gaussian using interval regression. The proposed ECOFF/ECVs were then validated by comparing with the MIC distributions of high-confidence genetic variants that confer resistance and with qualitative drug susceptibility tests obtained via the Mycobacterial Growth Indicator Tube (MGIT) system or Microscopic-Observation Drug Susceptibility (MODS) assay. These ECOFF/ECVs will inform and encourage the more widespread adoption of broth microdilution: this is a cheap culture-based method that tests the susceptibility of 12-14 antibiotics on a single 96-well plate and so could help personalise the treatment of tuberculosis.
BACKGROUNDSensor-based remote health monitoring can be used for the timely detection of health deterioration in people living with dementia with minimal impact on their day-to-day living. Anomaly detection approaches have been widely applied in various domains, including remote health monitoring. However, current approaches are challenged by noisy, multivariate data and low generalizability. OBJECTIVEThis study aims to develop an online, lightweight unsupervised learning-based approach to detect anomalies representing adverse health conditions using activity changes in people living with dementia. We demonstrated its effectiveness over state-of-the-art methods on a real-world data set of 9363 days collected from 15 participant households by the UK Dementia Research Institute between August 2019 and July 2021. Our approach was applied to household movement data to detect urinary tract infections (UTIs) and hospitalizations. METHODSWe propose and evaluate a solution based on Contextual Matrix Profile (CMP), an exact, ultrafast distance-based anomaly detection algorithm. Using daily aggregated household movement data collected via passive infrared sensors, we generated CMPs for location-wise sensor counts, duration, and change in hourly movement patterns for each patient. We computed a normalized anomaly score in 2 ways: by combining univariate CMPs and by developing a multidimensional CMP. The performance of our method was evaluated relative to Angle-Based Outlier Detection, Copula-Based Outlier Detection, and Lightweight Online Detector of Anomalies. We used the multidimensional CMP to discover and present the important features associated with adverse health conditions in people living with dementia. RESULTSThe multidimensional CMP yielded, on average, 84.3% recall with 32.1 alerts, or a 5.1% alert rate, offering the best balance of recall and relative precision compared with Copula-Based and Angle-Based Outlier Detection and Lightweight Online Detector of Anomalies when evaluated for UTI and hospitalization. Midnight to 6 AM bathroom activity was shown to be the most important cross-patient digital biomarker of anomalies indicative of UTI, contributing approximately 30% to the anomaly score. We also demonstrated how CMP-based anomaly scoring can be used for a cross-patient view of anomaly patterns. CONCLUSIONSTo the best of our knowledge, this is the first real-world study to adapt the CMP to continuous anomaly detection in a health care scenario. The CMP inherits the speed, accuracy, and simplicity of the Matrix Profile, providing configurability, the ability to denoise and detect patterns, and explainability to clinical practitioners. We addressed the need for anomaly scoring in multivariate time series health care data by developing the multidimensional CMP. With high sensitivity, a low alert rate, better overall performance than state-of-the-art methods, and the ability to discover digital biomarkers of anomalies, the CMP is a clinically meaningful unsupervised anomaly detection technique extensible to multimodal data for dementia and other health care scenarios.
There are many short-read variant-calling tools, with different strengths and weaknesses. We present a tool, Minos, which combines outputs from arbitrary variant callers, increasing recall without loss of precision. We benchmark on 62 samples from three bacterial species and an outbreak of 385 Mycobacterium tuberculosis samples. Minos also enables joint genotyping; we demonstrate on a large (N=13k) M. tuberculosis cohort, building a map of non-synonymous SNPs and indels in a region where all such variants are assumed to cause rifampicin resistance. We quantify the correlation with phenotypic resistance and then replicate in a second cohort (N=10k).
Oncology patients experience numerous co-occurring symptoms during their treatment. The identification of sentinel/core symptoms is a vital prerequisite for therapeutic interventions. In this study, using Network Analysis, we investigated the inter-relationships among 38 common symptoms over time (i.e., a total of six time points over two cycles of chemotherapy) in 987 oncology patients with four different types of cancer (i.e., breast, gastrointestinal, gynaecological, and lung). In addition, we evaluated the associations between and among symptoms and symptoms clusters and examined the strength of these interactions over time. Eight unique symptom clusters were identified within the networks. Findings from this research suggest that changes occur in the relationships and interconnections between and among co-occurring symptoms and symptoms clusters that depend on the time point in the chemotherapy cycle and the type of cancer. The evaluation of the centrality measures provides new insights into the relative importance of individual symptoms within various networks that can be considered as potential targets for symptom management interventions.
Due to the inevitable role of emotions in human learning and decision-making, different types of emotions in the form of emotional weights/neurons have also been considered in shallow neural networks. Emotional neural networks suffer from a low convergence rate as well as batch learning instability mainly because of the improper tuning of learning coefficients. To overcome these drawbacks, we introduced two solutions: (i) a heuristic upgrading method, inspiring by the behavior of dopamine secretion in the human brain, to adaptively regulate the learning rate based on positive and negative emotional states at each epoch and (ii) a stochastic learning technique to stabilize the learning process. The proposed dopamine based adaptive emotional neural network statistically outperforms state-of-the-art methods like emotional neural network, prototype-incorporated emotional neural network, multi-layer perceptron, and deep convolutional neural networks such as LeNet, AlexNet, DenseNet, MobileNet and EfficientNet in terms of different measures such as accuracy and convergence rate on several high dimensional and big datasets.
COVID‐19 is a major, urgent, and ongoing threat to global health. Globally more than 24 million have been infected and the disease has claimed more than a million lives as of November 2020. Predicting which patients will need respiratory support is important to guiding individual patient treatment and also to ensuring sufficient resources are available. The ability of six common Early Warning Scores (EWS) to identify respiratory deterioration defined as the need for advanced respiratory support (high‐flow nasal oxygen, continuous positive airways pressure, non‐invasive ventilation, intubation) within a prediction window of 24 h is evaluated. It is shown that these scores perform sub‐optimally at this specific task. Therefore, an alternative EWS based on the Gradient Boosting Trees (GBT) algorithm is developed that is able to predict deterioration within the next 24 h with high AUROC 94% and an accuracy, sensitivity, and specificity of 70%, 96%, 70%, respectively. The GBT model outperformed the best EWS (LDTEWS:NEWS), increasing the AUROC by 14%. Our GBT model makes the prediction based on the current and baseline measures of routinely available vital signs and blood tests.
Abstract Antimicrobial resistance (AMR) poses a threat to global public health. To mitigate the impacts of AMR, it is important to identify the molecular mechanisms of AMR and thereby determine optimal therapy as early as possible. Conventional machine learning-based drug-resistance analyses assume genetic variations to be homogeneous, thus not distinguishing between coding and intergenic sequences. In this study, we represent genetic data from Mycobacterium tuberculosis as a graph, and then adopt a deep graph learning method—heterogeneous graph attention network (‘HGAT–AMR’)—to predict anti-tuberculosis (TB) drug resistance. The HGAT–AMR model is able to accommodate incomplete phenotypic profiles, as well as provide ‘attention scores’ of genes and single nucleotide polymorphisms (SNPs) both at a population level and for individual samples. These scores encode the inputs, which the model is ‘paying attention to’ in making its drug resistance predictions. The results show that the proposed model generated the best area under the receiver operating characteristic (AUROC) for isoniazid and rifampicin (98.53 and 99.10%), the best sensitivity for three first-line drugs (94.91% for isoniazid, 96.60% for ethambutol and 90.63% for pyrazinamide), and maintained performance when the data were associated with incomplete phenotypes (i.e. for those isolates for which phenotypic data for some drugs were missing). We also demonstrate that the model successfully identifies genes and SNPs associated with drug resistance, mitigating the impact of resistance profile while considering particular drug resistance, which is consistent with domain knowledge.
Phosphorylation of proteins is one of the most significant post-translational modifications (PTMs) and plays a crucial role in plant functionality due to its impact on signaling, gene expression, enzyme kinetics, protein stability and interactions. Accurate prediction of plant phosphorylation sites (p-sites) is vital as abnormal regulation of phosphorylation usually leads to plant diseases. However, current experimental methods for PTM prediction suffers from high-computational cost and are error-prone. The present study develops machine learning-based prediction techniques, including a high-performance interpretable deep tabular learning network (TabNet) to improve the prediction of protein p-sites in soybean. Moreover, we use a hybrid feature set of sequential-based features, physicochemical properties and position-specific scoring matrices to predict serine (Ser/S), threonine (Thr/T) and tyrosine (Tyr/Y) p-sites in soybean for the first time. The experimentally verified p-sites data of soybean proteins are collected from the eukaryotic phosphorylation sites database and database post-translational modification. We then remove the redundant set of positive and negative samples by dropping protein sequences with >40% similarity. It is found that the developed techniques perform >70% in terms of accuracy. The results demonstrate that the TabNet model is the best performing classifier using hybrid features and with window size of 13, resulted in 78.96 and 77.24% sensitivity and specificity, respectively. The results indicate that the TabNet method has advantages in terms of high-performance and interpretability. The proposed technique can automatically analyze the data without any measurement errors and any human intervention. Furthermore, it can be used to predict putative protein p-sites in plants effectively.
Agitation is one of the neuropsychiatric symptoms with high prevalence in de-mentia which can negatively impact the Activities of Daily Living (ADL) and the independence of individuals. Detecting agitation episodes can assist in providing People Living with Dementia (PLWD) with early and timely interventions. Analysing agitation episodes will also help identify modifiable factors such as ambient temperature and sleep as possible components causing agitation in an individual. This preliminary study presents a supervised learning model to anal-* We apply a recurrent deep learning model to identify agitation episodes validated and recorded by a clinical monitoring team. We present the experiments to assess the efficacy of the proposed model. The proposed model achieves an average of 79.78% recall, 27.66% precision and 37.64% F1 scores when employing the optimal parameters, suggesting a good ability to recognise agitation events. We also discuss using machine learning models for analysing the behavioural patterns using continuous monitoring data and explore clinical applicability and the choices between specificity and specificity in home monitoring applications.
Molecular diagnostics are considered the most promising route to achieving rapid, universal drug susceptibility testing for complex (MTBC). We aimed to generate a WHO endorsed catalogue of mutations to serve as a global standard for interpreting molecular information for drug resistance prediction. A candidate gene approach was used to identify mutations as associated with resistance, or consistent with susceptibility, for 13 WHO endorsed anti-tuberculosis drugs. 38,215 MTBC isolates with paired whole-genome sequencing and phenotypic drug susceptibility testing data were amassed from 45 countries. For each mutation, a contingency table of binary phenotypes and presence or absence of the mutation computed positive predictive value, and Fisher's exact tests generated odds ratios and Benjamini-Hochberg corrected p-values. Mutations were graded as Associated with Resistance if present in at least 5 isolates, if the odds ratio was >1 with a statistically significant corrected p-value, and if the lower bound of the 95% confidence interval on the positive predictive value for phenotypic resistance was >25%. A series of expert rules were applied for final confidence grading of each mutation. 15,667 associations were computed for 13,211 unique mutations linked to one or more drugs. 1,149/15,667 (7·3%) mutations were classified as associated with phenotypic resistance and 107/15,667 (0·7%) were deemed consistent with susceptibility. For rifampicin, isoniazid, ethambutol, fluoroquinolones, and streptomycin, the mutations' pooled sensitivity was >80%. Specificity was over 95% for all drugs except ethionamide (91·4%), moxifloxacin (91·6%) and ethambutol (93·3%). Only two resistance mutations were classified for bedaquiline, delamanid, clofazimine, and linezolid as prevalence of phenotypic resistance was low for these drugs. This first WHO endorsed catalogue of molecular targets for MTBC drug susceptibility testing provides a global standard for resistance interpretation. Its existence should encourage the implementation of molecular diagnostics by National Tuberculosis Programmes. UNITAID, Wellcome, MRC, BMGF.
Missing values exist in nearly all clinical studies because data for a variable or question are not collected or not available. Imputing missing values and augmenting data can significantly improve generalisation and avoid bias in machine learning models. We propose a Hybrid Bayesian inference using Hamiltonian Monte Carlo (F-HMC) as a more practical approach to process cross-dimensional relations by applying a random walk and Hamiltonian dynamics to adapt posterior distribution and generate large-scale samples. The proposed method is applied to cancer symptom assessment, and MNIST datasets confirmed to enrich data quality in precision, accuracy, recall, F1-score, and propensity metric.
Background Behavioural changes and neuropsychiatric symptoms such as agitation are common in people with dementia. These symptoms impact the quality of life of people with dementia and can increase the stress on caregivers. This study aims to identify the likelihood of having agitation in people affected by dementia (i.e., patients and carers) using routinely collected data from in‐home monitoring technologies. We have used a digital platform and analytical methods, developed in our previous study, to generate alerts when changes occur in the digital markers collected using in‐home sensing technologies (i.e., vital signs, environmental and activity data). A care monitoring team use the platform and interact with participants and caregivers when an alert is generated. Method We have used connected sensory devices to collect environmental markers, including Passive Infra‐Red (PIR), smart power plugs for monitoring home appliance use, motion and door sensors. The environmental marker data have been aggregated within each hour and used to train an agitation risk analysis model. We have trained a model using data collected from 88 homes (∼6 months of data from each home). The proposed model has two components: a self‐supervised transformation learning and an ensemble classification model for agitation likelihood. Ten different neural network encoders are learned to create pseudo‐labels using the samples from the unlabelled data. We use these pseudo‐labels to train a classification model with a convolutional block and a decision layer. The trained convolutional block is then used to learn a latent representation of the data for an ensemble classification block. Results Comparing with baseline models such as LSTM network, Bidirectional LSTM (BiLSTM) network, VGG, ResNet, Inception, Random Forest (RF), Support Vector Machine (SVM) and Gaussian Process (GP) classifiers, the proposed model performs better in sensitivity (recall) and area under the precision‐recall curve with at most 40% improvement. The recall measure using the 10‐fold cross‐validation technique is 61%. Conclusion This method can support early interventions and help develop new pathways to support people affected by dementia. A limitation in our current study is that the environmental and movement data is at the home level and not personalised.