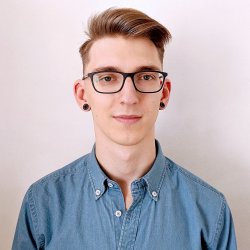
Dr Alexander Black
About
My research project
Robust video fingerprinting and comparison for content authenticityThe aim of this project is to make it possible to compare a video encountered 'in the wild' on the internet to its original source. We identify two main parts of this problem: retrieval and comparison.
Retrieval requires not only the ability to produce video fingerprints that are robust to existing and emergent types of transformations, but also a scalable retrieval system capable of searching through millions of videos in a matter of seconds or less.
Comparison refers to identification of salient differences between two videos. Salient differences are the ones that change the semantic meaning or the story of the video, as opposed to benign changes, such as format, resolution, size, brightness etc.
Supervisors
The aim of this project is to make it possible to compare a video encountered 'in the wild' on the internet to its original source. We identify two main parts of this problem: retrieval and comparison.
Retrieval requires not only the ability to produce video fingerprints that are robust to existing and emergent types of transformations, but also a scalable retrieval system capable of searching through millions of videos in a matter of seconds or less.
Comparison refers to identification of salient differences between two videos. Salient differences are the ones that change the semantic meaning or the story of the video, as opposed to benign changes, such as format, resolution, size, brightness etc.
Publications
We present a new method for learning a fine-grained representation of visual style. Representation learning aims to discover individual salient features of a domain in a compact and descriptive form that strongly identifies the unique characteristics of that domain. Prior visual style representation works attempt to disentangle style (i.e. appearance) from content (i.e. semantics) yet a complete separation has yet to be achieved. We present a technique to learn a representation of visual style more strongly disentangled from the semantic content depicted in an image. We use Neural Style Transfer (NST) to measure and drive the learning signal and achieve state-of-the-art representation learning on explicitly disentangled metrics. We show that strongly addressing the disentanglement of style and content leads to large gains in style-specific metrics, encoding far less semantic information and achieving state-of-the-art accuracy in downstream style matching (retrieval) and zero-shot style tagging tasks.
Figure 1: DECORAIT enables creatives to register consent (or not) for Generative AI training using their content, as well as to receive recognition and reward for that use. Provenance is traced via visual matching, and consent and ownership registered using a distributed ledger (blockchain). Here, a synthetic image is generated via the Dreambooth[32] method using prompt "a photo of [Subject]" and concept images (left). The red cross indicates images whose creatives have opted out of AI training via DECORAIT, which when taken into account leads to a significant visual change (right). DECORAIT also determines credit apportionment across the opted-in images and pays a proportionate reward to creators via crypto-currency micropyament. ABSTRACT We present DECORAIT; a decentralized registry through which content creators may assert their right to opt in or out of AI training as well as receive reward for their contributions. Generative AI (GenAI) enables images to be synthesized using AI models trained on vast amounts of data scraped from public sources. Model and content creators who may wish to share their work openly without sanctioning its use for training are thus presented with a data gov-ernance challenge. Further, establishing the provenance of GenAI training data is important to creatives to ensure fair recognition and reward for their such use. We report a prototype of DECO-RAIT, which explores hierarchical clustering and a combination of on/off-chain storage to create a scalable decentralized registry to trace the provenance of GenAI training data in order to determine training consent and reward creatives who contribute that data. DECORAIT combines distributed ledger technology (DLT) with visual fingerprinting, leveraging the emerging C2PA (Coalition for Content Provenance and Authenticity) standard to create a secure, open registry through which creatives may express consent and data ownership for GenAI.
Representation learning aims to discover individual salient features of a domain in a compact and descriptive form that strongly identifies the unique characteristics of a given sample respective to its domain. Existing works in visual style representation literature have tried to disentangle style from content during training explicitly. A complete separation between these has yet to be fully achieved. Our paper aims to learn a representation of visual artistic style more strongly disentangled from the semantic content depicted in an image. We use Neural Style Transfer (NST) to measure and drive the learning signal and achieve state-of-the-art representation learning on explicitly disentangled metrics. We show that strongly addressing the disentanglement of style and content leads to large gains in style-specific metrics, encoding far less semantic information and achieving state-of-the-art accuracy in downstream multimodal applications.
We present VPN - a content attribution method for recovering provenance information from videos shared online. Platforms, and users, often transform video into different quality, codecs, sizes, shapes, etc. or slightly edit its content such as adding text or emoji, as they are redistributed online. We learn a robust search embedding for matching such video, invariant to these transformations, using full-length or truncated video queries. Once matched against a trusted database of video clips, associated information on the provenance of the clip is presented to the user. We use an inverted index to match temporal chunks of video using late-fusion to combine both visual and audio features. In both cases, features are extracted via a deep neural network trained using contrastive learning on a dataset of original and augmented video clips. We demonstrate high accuracy recall over a corpus of 100,000 videos.
We propose a self-supervised learning approach for videos that learns representations of both the RGB frames and the accompanying audio without human supervision. In contrast to images that capture the static scene appearance, videos also contain sound and temporal scene dynamics. To leverage the temporal and aural dimension inherent to videos, our method extends temporal self-supervision to the audio-visual setting and integrates it with multi-modal contrastive objectives. As temporal self-supervision, we pose playback speed and direction recognition in both modalities and propose intra- and inter-modal temporal ordering tasks. Furthermore, we design a novel contrastive objective in which the usual pairs are supplemented with additional sample-dependent positives and negatives sampled from the evolving feature space. In our model, we apply such losses among video clips and between videos and their temporally corresponding audio clips. We verify our model design in extensive ablation experiments and evaluate the video and audio representations in transfer experiments to action recognition and retrieval on UCF101 and HMBD51, audio classification on ESC50, and robust video fingerprinting on VGG-Sound, with state-of-the-art results.
We present DECORAIT; a decentralized registry through which content creators may assert their right to opt in or out of AI training as well as receive reward for their contributions. Generative AI (GenAI) enables images to be synthesized using AI models trained on vast amounts of data scraped from public sources. Model and content creators who may wish to share their work openly without sanctioning its use for training are thus presented with a data governance challenge. Further, establishing the provenance of GenAI training data is important to creatives to ensure fair recognition and reward for their such use. We report a prototype of DECORAIT, which explores hierarchical clustering and a combination of on/off-chain storage to create a scalable decentralized registry to trace the provenance of GenAI training data in order to determine training consent and reward creatives who contribute that data. DECORAIT combines distributed ledger technology (DLT) with visual fingerprinting, leveraging the emerging C2PA (Coalition for Content Provenance and Authenticity) standard to create a secure, open registry through which creatives may express consent and data ownership for GenAI.
We propose VADER, a spatio-temporal matching, alignment, and change summarization method to help fight misinformation spread via manipulated videos. VADER matches and coarsely aligns partial video fragments to candidate videos using a robust visual descriptor and scalable search over adaptively chunked video content. A transformer-based alignment module then refines the temporal localization of the query fragment within the matched video. A space-time comparator module identifies regions of manipulation between aligned content, invariant to any changes due to any residual temporal misalignments or artifacts arising from non-editorial changes of the content. Robustly matching video to a trusted source enables conclusions to be drawn on video provenance, enabling informed trust decisions on content encountered.
We present an algorithm for searching image collections using free-hand sketches that describe the appearance and relative positions of multiple objects 1 Sketch based image retrieval (SBIR) methods predominantly match queries containing a single, dominant object invariant to its position within an image. Our work exploits drawings as a concise and intuitive representation for specifying entire scene compositions. We train a convolutional neural network (CNN) to encode masked visual features from sketched objects, pooling these into a spatial descriptor encoding the spatial relationships and appearances of objects in the composition. Training the CNN backbone as a Siamese network under triplet loss yields a metric search embedding for measuring compositional similarity which may be efficiently leveraged for visual search by applying product quantization.