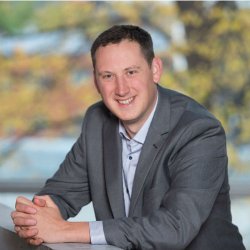
Professor John Collomosse
Academic and research departments
Centre for Vision, Speech and Signal Processing (CVSSP), Surrey Centre for Cyber Security, School of Computer Science and Electronic Engineering.About
Biography
John Collomosse is a Professor of Computer Vision at the Centre for Vision Speech and Signal Processing (CVSSP), one the UK’s largest academic research groups for Artificial Intelligence with over 150 researchers John is a Visiting Professor at Adobe Research, Creative Intelligence Lab (San Jose, CA). John joined CVSSP in 2009. Previously he was an Assistant Professor at the Department of Computer Science, University of Bath where he completed his PhD in 2004 on the topic of AI for Image Stylization. John has also spent periods of time in commercial R&D, working for IBM UK Labs (Hursley), Vodafone R&D (Munich), Hewlett Packard Labs (Bristol); the latter under a Royal Academy of Engineering fellowship. John is a Chartered Engineer (C.Eng, 2013) and since 2018 a member of the EPSRC ICT Strategic Advisory Team (SAT) and UKRI Digital Economy Programme Advisory Board (PAB).
John leads the digital creativity lab at CVSSP focusing on the interaction of Computer Vision, Graphics and AI for the creative industries, specifically for human performance capture, video post-production and vfx, and intuitive visual search (particularly sketch search). He also heads up the Surrey Blockchain activity exploring the fusion of AI and Distributed Ledger Technologies.
John is the Course Director for MSc in Computer Vision, Robotics and Machine Learning and the Deputy Director of Teaching for undergraduate programmes with the Department of Electrical and Electronic Engineering. John teaches the Image Processing and Deep Learning (EEEM063), and Computer Vision and Pattern Recognition (EEE3032) modules.
Full details of John's publications including PDFs are on his personal website - the publications tab on this page is automatically generated and may not be complete or accurate.
News
Publications
Image attribution - matching an image back to a trusted source - is an emerging tool in the fight against online misinformation. Deep visual fingerprinting models have recently been explored for this purpose. However, they are not robust to tiny input perturbations known as adversarial examples. First we illustrate how to generate valid adversarial images that can easily cause incorrect image attribution. Then we describe an approach to prevent imperceptible adversarial attacks on deep visual fingerprinting models, via robust contrastive learning. The proposed training procedure leverages training on ℓ ∞ -bounded adversarial examples, it is conceptually simple and incurs only a small computational overhead. The resulting models are substantially more robust, are accurate even on unperturbed images, and perform well even over a database with millions of images. In particular, we achieve 91.6% standard and 85.1% adversarial recall under ℓ ∞ -bounded perturbations on manipulated images compared to 80.1% and 0.0% from prior work. We also show that robustness generalizes to other types of imperceptible perturbations unseen during training. Finally, we show how to train an adversarially robust image comparator model for detecting editorial changes in matched images. Project page: https://max-andr.github.io/robust_image_attribution.
We propose a self-supervised learning approach for videos that learns representations of both the RGB frames and the accompanying audio without human supervision. In contrast to images that capture the static scene appearance, videos also contain sound and temporal scene dynamics. To leverage the temporal and aural dimension inherent to videos, our method extends temporal self-supervision to the audio-visual setting and integrates it with multi-modal contrastive objectives. As temporal self-supervision, we pose playback speed and direction recognition in both modalities and propose intra- and inter-modal temporal ordering tasks. Furthermore, we design a novel contrastive objective in which the usual pairs are supplemented with additional sample-dependent positives and negatives sampled from the evolving feature space. In our model, we apply such losses among video clips and between videos and their temporally corresponding audio clips. We verify our model design in extensive ablation experiments and evaluate the video and audio representations in transfer experiments to action recognition and retrieval on UCF101 and HMBD51, audio classification on ESC50, and robust video fingerprinting on VGG-Sound, with state-of-the-art results.
We present DECORAIT; a decentralized registry through which content creators may assert their right to opt in or out of AI training as well as receive reward for their contributions. Generative AI (GenAI) enables images to be synthesized using AI models trained on vast amounts of data scraped from public sources. Model and content creators who may wish to share their work openly without sanctioning its use for training are thus presented with a data governance challenge. Further, establishing the provenance of GenAI training data is important to creatives to ensure fair recognition and reward for their such use. We report a prototype of DECORAIT, which explores hierarchical clustering and a combination of on/off-chain storage to create a scalable decentralized registry to trace the provenance of GenAI training data in order to determine training consent and reward creatives who contribute that data. DECORAIT combines distributed ledger technology (DLT) with visual fingerprinting, leveraging the emerging C2PA (Coalition for Content Provenance and Authenticity) standard to create a secure, open registry through which creatives may express consent and data ownership for GenAI.
We present VPN - a content attribution method for recovering provenance information from videos shared online. Platforms, and users, often transform video into different quality, codecs, sizes, shapes, etc. or slightly edit its content such as adding text or emoji, as they are redistributed online. We learn a robust search embedding for matching such video, invariant to these transformations, using full-length or truncated video queries. Once matched against a trusted database of video clips, associated information on the provenance of the clip is presented to the user. We use an inverted index to match temporal chunks of video using late-fusion to combine both visual and audio features. In both cases, features are extracted via a deep neural network trained using contrastive learning on a dataset of original and augmented video clips. We demonstrate high accuracy recall over a corpus of 100,000 videos.
We propose VADER, a spatio-temporal matching, alignment, and change summarization method to help fight misinformation spread via manipulated videos. VADER matches and coarsely aligns partial video fragments to candidate videos using a robust visual descriptor and scalable search over adaptively chunked video content. A transformer-based alignment module then refines the temporal localization of the query fragment within the matched video. A space-time comparator module identifies regions of manipulation between aligned content, invariant to any changes due to any residual temporal misalignments or artifacts arising from non-editorial changes of the content. Robustly matching video to a trusted source enables conclusions to be drawn on video provenance, enabling informed trust decisions on content encountered.
We present an algorithm for searching image collections using free-hand sketches that describe the appearance and relative positions of multiple objects 1 Sketch based image retrieval (SBIR) methods predominantly match queries containing a single, dominant object invariant to its position within an image. Our work exploits drawings as a concise and intuitive representation for specifying entire scene compositions. We train a convolutional neural network (CNN) to encode masked visual features from sketched objects, pooling these into a spatial descriptor encoding the spatial relationships and appearances of objects in the composition. Training the CNN backbone as a Siamese network under triplet loss yields a metric search embedding for measuring compositional similarity which may be efficiently leveraged for visual search by applying product quantization.
Accurate camera synchronisation is indispensable for many video processing tasks, such as surveillance and 3D modelling. Video-based synchronisation facilitates the design and setup of networks with moving cameras or devices without an external synchronisation capability, such as low-cost web cameras, or Kinects. In this paper, we present an algorithm which can work with such heterogeneous networks. The algorithm first finds the corresponding frame indices between each camera pair, by the help of image feature correspondences and epipolar geometry. Then, for each pair, a relative frame rate and offset are computed by fitting a 2D line to the index correspondences. These pairwise relations define a graph, in which each spanning cycle comprises an absolute synchronisation hypothesis. The optimal solution is found by an exhaustive search over the spanning cycles. The algorithm is experimentally demonstrated to yield highly accurate estimates in a number of scenarios involving static and moving cameras, and Kinect.
Sketchformer is a novel transformer-based representation for encoding free-hand sketches input in a vector form, i.e. as a sequence of strokes. Sketchformer effectively addresses multiple tasks: sketch classification, sketch based image retrieval (SBIR), and the reconstruction and interpolation of sketches. We report several variants exploring continuous and tokenized input representations, and contrast their performance. Our learned embedding, driven by a dictionary learning tokenization scheme, yields state of the art performance in classification and image retrieval tasks, when compared against baseline representations driven by LSTM sequence to sequence architectures: SketchRNN and derivatives. We show that sketch reconstruction and interpolation are improved significantly by the Sketchformer embedding for complex sketches with longer stroke sequences.
We present ARCHANGEL; a novel distributed ledger based system for assuring the long-term integrity of digital video archives. First, we introduce a novel deep network architecture using a hierarchical attention autoencoder (HAAE) to compute temporal content hashes (TCHs) from minutes or hourlong audio-visual streams. Our TCHs are sensitive to accidental or malicious content modification (tampering). The focus of our self-supervised HAAE is to guard against content modification such as frame truncation or corruption but ensure invariance against format shift (i.e. codec change). This is necessary due to the curatorial requirement for archives to format shift video over time to ensure future accessibility. Second, we describe how the TCHs (and the models used to derive them) are secured via a proof-of-authority blockchain distributed across multiple independent archives.We report on the efficacy of ARCHANGEL within the context of a trial deployment in which the national government archives of the United Kingdom, United States of America, Estonia, Australia and Norway participated.
Style transfer is the task of reproducing the semantic contents of a source image in the artistic style of a second target image. In this paper, we present NeAT, a new state-of-the art feed-forward style transfer method. We re-formulate feed-forward style transfer as image editing, rather than image generation, resulting in a model which improves over the state-of-the-art in both preserving the source content and matching the target style. An important component of our model's success is identifying and fixing "style halos", a commonly occurring artefact across many style transfer techniques. In addition to training and testing on standard datasets, we introduce the BBST-4M dataset, a new, large scale, high resolution dataset of 4M images. As a component of curating this data, we present a novel model able to classify if an image is stylistic. We use BBST-4M to improve and measure the generalization of NeAT across a huge variety of styles. Not only does NeAT offer state-of-the-art quality and generalization, it is designed and trained for fast inference at high resolution.
Neural Style Transfer (NST) is the field of study applying neural techniques to modify the artistic appearance of a content image to match the style of a reference style image. Traditionally, NST methods have focused on texture-based image edits, affecting mostly low level information and keeping most image structures the same. However, style-based deformation of the content is desirable for some styles, especially in cases where the style is abstract or the primary concept of the style is in its deformed rendition of some content. With the recent introduction of diffusion models, such as Stable Diffusion, we can access far more powerful image generation techniques, enabling new possibilities. In our work, we propose using this new class of models to perform style transfer while enabling deformable style transfer, an elusive capability in previous models. We show how leveraging the priors of these models can expose new artistic controls at inference time, and we document our findings in exploring this new direction for the field of style transfer.
Representation learning aims to discover individual salient features of a domain in a compact and descriptive form that strongly identifies the unique characteristics of a given sample respective to its domain. Existing works in visual style representation literature have tried to disentangle style from content during training explicitly. A complete separation between these has yet to be fully achieved. Our paper aims to learn a representation of visual artistic style more strongly disentangled from the semantic content depicted in an image. We use Neural Style Transfer (NST) to measure and drive the learning signal and achieve state-of-the-art representation learning on explicitly disentangled metrics. We show that strongly addressing the disentanglement of style and content leads to large gains in style-specific metrics, encoding far less semantic information and achieving state-of-the-art accuracy in downstream multimodal applications.
We present a novel method for generating robust adversarial image examples building upon the recent `deep image prior' (DIP) that exploits convolutional network architectures to enforce plausible texture in image synthesis. Adversarial images are commonly generated by perturbing images to introduce high frequency noise that induces image misclassification, but that is fragile to subsequent digital manipulation of the image. We show that using DIP to reconstruct an image under adversarial constraint induces perturbations that are more robust to affine deformation, whilst remaining visually imperceptible. Furthermore we show that our DIP approach can also be adapted to produce local adversarial patches (`adversarial stickers'). We demonstrate robust adversarial examples over a broad gamut of images and object classes drawn from the ImageNet dataset.
We present Vax-a-Net; a technique for immunizing convolutional neural networks (CNNs) against adversarial patch attacks (APAs). APAs insert visually overt, local regions (patches) into an image to induce misclassification. We introduce a conditional Generative Adversarial Network (GAN) architecture that simultaneously learns to synthesise patches for use in APAs, whilst exploiting those attacks to adapt a pre-trained target CNN to reduce its susceptibility to them. This approach enables resilience against APAs to be conferred to pre-trained models, which would be impractical with conventional adversarial training due to the slow convergence of APA methods. We demonstrate transferability of this protection to defend against existing APAs, and show its efficacy across several contemporary CNN architectures.
Discussions of decentralization with respect to blockchain have tended to focus on the architecture of decentralization, its influence, and potential technical hurdles. Furthermore, decentralization is often conflated with distribution in these discussions. The authors argue, instead, for a relational definition of decentralization that considers blockchain within a data-social-technical framework. By considering conceptual ambiguities and the influence of decentralization on each of the data, technical, and social layers of blockchain, the authors analyze the disruptive power of decentralization. Shifting to a decentralized organization of activity necessarily requires a transition that threatens existing power structures and dynamics, requiring actors to consider how such changes in power might interplay with, and be mitigated by changes in, trust dynamics. These institutional barriers to adoption must be understood as part of the design of the technology to help realize its ultimate societal success. As the process of decentralization evolves, further research will be needed to expose and mitigate associated challenges to data preservation and security, and to consider how societal resistance and related pitfalls might impact and restrict adoption of the democratization process.
We propose a novel representation learning technique for measuring the similarity of user interface designs. A triplet network is used to learn a search embedding for layout similarity, with a hybrid encoder-decoder backbone comprising a graph convolutional network (GCN) and convolutional decoder (CNN). The properties of interface components and their spatial relationships are encoded via a graph which also models the containment (nesting) relationships of interface components. We supervise the training of a dual reconstruction and pair-wise loss using an auxiliary measure of layout similarity based on intersection over union (IoU) distance. The resulting embedding is shown to exceed state of the art performance for visual search of user interface layouts over the public Rico dataset, and an auto-annotated dataset of interface layouts collected from the web. We release the codes and dataset (https://github.com/dips4717/gcn-cnn.)
We present a novel hierarchical modeling method for layout representation learning, the core of design documents (e.g., user interface, poster, template). Existing works on layout representation often ignore element hierarchies, which is an important facet of layouts, and mainly rely on the spatial bounding boxes for feature extraction. This paper proposes a Spatial-Structural Hierarchical Auto-Encoder (SSH-AE) that learns hierarchical representation by treating a hierarchically annotated layout as a tree format. On the one side, we model SSH-AE from both spatial (semantic views) and structural (organization and relationships) perspectives, which are two complementary aspects to represent a layout. On the other side, the semantic/geometric properties are associated at multiple resolutions/granularities, naturally handling complex layouts. Our learned representations are used for effective layout search from both spatial and structural similarity perspectives. We also newly involve the tree-edit distance (TED) as an evaluation metric to construct a comprehensive evaluation protocol for layout similarity assessment, which benefits a systematic and customized layout search. We further present a new dataset of POSTER layouts which we believe will be useful for future layout research. We show that our proposed SSH-AE outperforms the existing methods achieving state-of-the-art performance on two benchmark datasets. Code is available at github.com/yueb17/SSH-AE.
We present a novel view synthesis method based upon latent voxel embeddings of an object, which encode both shape and appearance information and are learned without explicit 3D occupancy supervision. Our method uses an encoder-decoder architecture to learn such deep volumetric representations from a set of images taken at multiple viewpoints. Compared with DeepVoxels, our DeepVoxels++ applies a series of enhancements: a) a patch-based image feature extraction and neural rendering scheme that learns local shape and texture patterns, and enables neural rendering at high resolution; b) learned view-dependent feature transformation kernels to explicitly model perspective transformations induced by viewpoint changes; c) a recurrent-concurrent aggregation technique to alleviate single-view update bias of the voxel embeddings recurrent learning process. Combined with d) a simple yet effective implementation trick of frustum representation sufficient sampling, we achieve improved visual quality over the prior deep voxel-based methods (33%\documentclass[12pt]{minimal} \usepackage{amsmath} \usepackage{wasysym} \usepackage{amsfonts} \usepackage{amssymb} \usepackage{amsbsy} \usepackage{mathrsfs} \usepackage{upgreek} \setlength{\oddsidemargin}{-69pt} \begin{document}$$\%$$\end{document} SSIM error reduction and 22%\documentclass[12pt]{minimal} \usepackage{amsmath} \usepackage{wasysym} \usepackage{amsfonts} \usepackage{amssymb} \usepackage{amsbsy} \usepackage{mathrsfs} \usepackage{upgreek} \setlength{\oddsidemargin}{-69pt} \begin{document}$$\%$$\end{document} PSNR improvement) on 360∘\documentclass[12pt]{minimal} \usepackage{amsmath} \usepackage{wasysym} \usepackage{amsfonts} \usepackage{amssymb} \usepackage{amsbsy} \usepackage{mathrsfs} \usepackage{upgreek} \setlength{\oddsidemargin}{-69pt} \begin{document}$$360^\circ $$\end{document} novel-view synthesis benchmarks.
We present Magic Layouts; a method for parsing screen-shots or hand-drawn sketches of user interface (UI) layouts. Our core contribution is to extend existing detectors to exploit a learned structural prior for UI designs, enabling robust detection of UI components; buttons, text boxes and similar. Specifically we learn a prior over mobile UI layouts, encoding common spatial co-occurrence relationships between different UI components. Conditioning region proposals using this prior leads to performance gains on UI layout parsing for both hand-drawn UIs and app screen-shots, which we demonstrate within the context an interactive application for rapidly acquiring digital prototypes of user experience (UX) designs.
Rapid advances in Generative Adversarial Networks (GANs) raise new challenges for image attribution; detecting whether an image is synthetic and, if so, determining which GAN architecture created it. Uniquely, we present a solution to this task capable of 1) matching images invariant to their semantic content; 2) robust to benign transformations (changes in quality, resolution, shape, etc.) commonly encountered as images are re-shared online. In order to formalize our research, a challenging benchmark, Attribution88, is collected for robust and practical image attribution. We then propose RepMix, our GAN fingerprinting technique based on representation mixing and a novel loss. We validate its capability of tracing the provenance of GAN-generated images invariant to the semantic content of the image and also robust to perturbations. We show our approach improves significantly from existing GAN finger-printing works on both semantic generalization and robustness. Data and code are available at https://github.com/TuBui/image attribution.
The ubiquity and commoditisation of wearable biosensors (fitness bands) has led to a deluge of personal healthcare data, but with limited analytics typically fed back to the user. The feasibility of feeding back more complex, seemingly unrelated measures to users was investigated, by assessing whether increased levels of stress, anxiety and depression (factors known to affect cardiac function) and general health measures could be accurately predicted using heart rate variability (HRV) data from wrist wearables alone. Levels of stress, anxiety, depression and general health were evaluated from subjective questionnaires completed on a weekly or twice-weekly basis by 652 participants. These scores were then converted into binary levels (either above or below a set threshold) for each health measure and used as tags to train Deep Neural Networks (LSTMs) to classify each health measure using HRV data alone. Three data input types were investigated: time domain, frequency domain and typical HRV measures. For mental health measures, classification accuracies of up to 83% and 73% were achieved, with five and two minute HRV data streams respectively, showing improved predictive capability and potential future wearable use for tracking stress and well-being. [Display omitted] •A novel study further exploiting data collected from wearable biosensors.•Heart rate variability (HRV) was recorded using wearables in 652 participants.•Long Short Term Memory networks link HRV to mental & general health.
We propose Geo-PIFu, a method to recover a 3D mesh from a monocular color image of a clothed person. Our method is based on a deep implicit function-based representation to learn latent voxel features using a structure-aware 3D U-Net, to constrain the model in two ways: first, to resolve feature ambiguities in query point encoding, second, to serve as a coarse human shape proxy to regularize the high-resolution mesh and encourage global shape regularity. We show that, by both encoding query points and constraining global shape using latent voxel features, the reconstruction we obtain for clothed human meshes exhibits less shape distortion and improved surface details compared to competing methods. We evaluate Geo-PIFu on a recent human mesh public dataset that is \(10 \times\) larger than the private commercial dataset used in PIFu and previous derivative work. On average, we exceed the state of the art by \(42.7\%\) reduction in Chamfer and Point-to-Surface Distances, and \(19.4\%\) reduction in normal estimation errors.
As tools for content editing mature, and artificial intelligence (AI) based algorithms for synthesizing media grow, the presence of manipulated content across online media is increasing. This phenomenon causes the spread of misinformation, creating a greater need to distinguish between "real" and "manipulated" content. To this end, we present VIDEOSHAM, a dataset consisting of 826 videos (413 real and 413 manipulated). Many of the existing deepfake datasets focus exclusively on two types of facial manipulations-swapping with a different subject's face or altering the existing face. VIDEOSHAM, on the other hand, contains more diverse, context-rich, and human-centric, high-resolution videos manipulated using a combination of 6 different spatial and temporal attacks. Our analysis shows that state-of-the-art manipulation detection algorithms only work for a few specific attacks and do not scale well on VIDEOSHAM. We performed a user study on Amazon Mechanical Turk with 1200 participants to understand if they can differentiate between the real and manipulated videos in VIDEOSHAM. Finally, we dig deeper into the strengths and weaknesses of performances by humans and SOTA-algorithms to identify gaps that need to be filled with better AI algorithms. We present the dataset here(1).
We present CoGS, a novel method for the style-conditioned, sketch-driven synthesis of images. CoGS enables exploration of diverse appearance possibilities for a given sketched object, enabling decoupled control over the structure and the appearance of the output. Coarse-grained control over object structure and appearance are enabled via an input sketch and an exemplar "style" conditioning image to a transformer-based sketch and style encoder to generate a discrete codebook representation. We map the codebook representation into a metric space, enabling fine-grained control over selection and interpolation between multiple synthesis options before generating the image via a vector quantized GAN (VQGAN) decoder. Our framework thereby unifies search and synthesis tasks, in that a sketch and style pair may be used to run an initial synthesis which may be refined via combination with similar results in a search corpus to produce an image more closely matching the user's intent. We show that our model, trained on the 125 object classes of our newly created Pseudosketches dataset, is capable of producing a diverse gamut of semantic content and appearance styles.
Scene Designer is a novel method for searching and generating images using free-hand sketches of scene compositions; i.e. drawings that describe both the appearance and relative positions of objects. Our core contribution is a single unified model to learn both a cross-modal search embedding for matching sketched compositions to images, and an object embedding for layout synthesis. We show that a graph neural network (GNN) followed by Transformer under our novel contrastive learning setting is required to allow learning correlations between object type, appearance and arrangement, driving a mask generation module that synthesizes coherent scene layouts, whilst also delivering state of the art sketch based visual search of scenes.
Scene Designer is a novel method for Compositional Sketch-based Image Retrieval (CSBIR) that combines semantic layout synthesis with its main task both to boost performance and enable new creative workflows. While most studies on sketch focus on single-object retrieval, we look to multi-object scenes instead for increased query specificity and flexibility. Our training protocol improves contrastive learning by synthesising harder negative samples and introduces a layout synthesis task that further improves the semantic scene representations. We show that our object-oriented graph neural network (GNN) more than doubles the current SoTA recall@1 on the SketchyCOCO CSBIR benchmark under our novel contrastive learning setting and combined search and synthesis tasks. Furthermore, we introduce the first large-scale sketched scene dataset and benchmark in QuickDrawCOCO.
Figure 1: DECORAIT enables creatives to register consent (or not) for Generative AI training using their content, as well as to receive recognition and reward for that use. Provenance is traced via visual matching, and consent and ownership registered using a distributed ledger (blockchain). Here, a synthetic image is generated via the Dreambooth[32] method using prompt "a photo of [Subject]" and concept images (left). The red cross indicates images whose creatives have opted out of AI training via DECORAIT, which when taken into account leads to a significant visual change (right). DECORAIT also determines credit apportionment across the opted-in images and pays a proportionate reward to creators via crypto-currency micropyament. ABSTRACT We present DECORAIT; a decentralized registry through which content creators may assert their right to opt in or out of AI training as well as receive reward for their contributions. Generative AI (GenAI) enables images to be synthesized using AI models trained on vast amounts of data scraped from public sources. Model and content creators who may wish to share their work openly without sanctioning its use for training are thus presented with a data gov-ernance challenge. Further, establishing the provenance of GenAI training data is important to creatives to ensure fair recognition and reward for their such use. We report a prototype of DECO-RAIT, which explores hierarchical clustering and a combination of on/off-chain storage to create a scalable decentralized registry to trace the provenance of GenAI training data in order to determine training consent and reward creatives who contribute that data. DECORAIT combines distributed ledger technology (DLT) with visual fingerprinting, leveraging the emerging C2PA (Coalition for Content Provenance and Authenticity) standard to create a secure, open registry through which creatives may express consent and data ownership for GenAI.
We present a novel hybrid representation for character animation from 4D Performance Capture (4DPC) data which combines skeletal control with surface motion graphs. 4DPC data are temporally aligned 3D mesh sequence reconstructions of the dynamic surface shape and associated appearance from multiple view video. The hybrid representation supports the production of novel surface sequences which satisfy constraints from user specified key-frames or a target skeletal motion. Motion graph path optimisation concatenates fragments of 4DPC data to satisfy the constraints whilst maintaining plausible surface motion at transitions between sequences. Spacetime editing of the mesh sequence using a learnt part-based Laplacian surface deformation model is performed to match the target skeletal motion and transition between sequences. The approach is quantitatively evaluated for three 4DPC datasets with a variety of clothing styles. Results for key-frame animation demonstrate production of novel sequences which satisfy constraints on timing and position of less than 1% of the sequence duration and path length. Evaluation of motion capture driven animation over a corpus of 130 sequences shows that the synthesised motion accurately matches the target skeletal motion. The combination of skeletal control with the surface motion graph extends the range and style of motion which can be produced whilst maintaining the natural dynamics of shape and appearance from the captured performance.
This article reports a user-experience study in which a group of 18 older adults used a location-based mobile multimedia service in the setting of a rural nature reserve. The prototype system offered a variety of means of obtaining rich multimedia content from oak waymarker posts using a mobile phone. Free text questionnaires and focus groups were employed to investigate participants' experiences with the system and their attitudes to the use of mobile and pervasive systems in general. The users' experiences with the system were positive with respect to the design of the system in the context of the surrounding natural environment. However, the authors found some significant barriers to their adoption of mobile and pervasive systems as replacements for traditional information sources.
We present a robust algorithm for temporally coherent video segmentation. Our approach is driven by multi-label graph cut applied to successive frames, fusing information from the current frame with an appearance model and labeling priors propagated forwarded from past frames. We propagate using a novel motion diffusion model, producing a per-pixel motion distribution that mitigates against cumulative estimation errors inherent in systems adopting “hard” decisions on pixel motion at each frame. Further, we encourage spatial coherence by imposing label consistency constraints within image regions (super-pixels) obtained via a bank of unsupervised frame segmentations, such as mean-shift. We demonstrate quantitative improvements in accuracy over state-of-the-art methods on a variety of sequences exhibiting clutter and agile motion, adopting the Berkeley methodology for our comparative evaluation.
This paper surveys the field of non-photorealistic rendering (NPR), focusing on techniques for transforming 2D input (images and video) into artistically stylized renderings. We first present a taxonomy of the 2D NPR algorithms developed over the past two decades, structured according to the design characteristics and behavior of each technique. We then describe a chronology of development from the semi-automatic paint systems of the early nineties, through to the automated painterly rendering systems of the late nineties driven by image gradient analysis. Two complementary trends in the NPR literature are then addressed, with reference to our taxonomy. First, the fusion of higher level computer vision and NPR, illustrating the trends toward scene analysis to drive artistic abstraction and diversity of style. Second, the evolution of local processing approaches toward edge-aware filtering for real-time stylization of images and video. The survey then concludes with a discussion of open challenges for 2D NPR identified in recent NPR symposia, including topics such as user and aesthetic evaluation.
We introduce a simple but versatile camera model that we call the Rational Tensor Camera (RTcam). RTcams are well principled mathematically and provably subsume several important contemporary camera models in both computer graphics and vision; their generality Is one contribution. They can be used alone or compounded to produce more complicated visual effects. In this paper, we apply RTcams to generate synthetic artwork with novel perspective effects from real photographs. Existing Nonphotorealistic Rendering from Photographs (NPRP) is constrained to the projection inherent in the source photograph, which is most often linear. RTcams lift this restriction and so contribute to NPRP via multiperspective projection. This paper describes RTcams, compares them to contemporary alternatives, and discusses how to control them in practice. Illustrative examples are provided throughout.
Three dimensional (3D) displays typically rely on stereo disparity, requiring specialized hardware to be worn or embedded in the display. We present a novel 3D graphics display system for volumetric scene visualization using only standard 2D display hardware and a pair of calibrated web cameras. Our computer vision-based system requires no worn or other special hardware. Rather than producing the depth illusion through disparity, we deliver a full volumetric 3D visualization - enabling users to interactively explore 3D scenes by varying their viewing position and angle according to the tracked 3D position of their face and eyes. We incorporate a novel wand-based calibration that allows the cameras to be placed at arbitrary positions and orientations relative to the display. The resulting system operates at real-time speeds (~25 fps) with low latency (120-225 ms) delivering a compelling natural user interface and immersive experience for 3D viewing. In addition to objective evaluation of display stability and responsiveness, we report on user trials comparing users' timings on a spatial orientation task.
A real-time motion capture system is presented which uses input from multiple standard video cameras and inertial measurement units (IMUs). The system is able to track multiple people simultaneously and requires no optical markers, specialized infra-red cameras or foreground/background segmentation, making it applicable to general indoor and outdoor scenarios with dynamic backgrounds and lighting. To overcome limitations of prior video or IMU-only approaches, we propose to use flexible combinations of multiple-view, calibrated video and IMU input along with a pose prior in an online optimization-based framework, which allows the full 6-DoF motion to be recovered including axial rotation of limbs and drift-free global position. A method for sorting and assigning raw input 2D keypoint detections into corresponding subjects is presented which facilitates multi-person tracking and rejection of any bystanders in the scene. The approach is evaluated on data from several indoor and outdoor capture environments with one or more subjects and the trade-off between input sparsity and tracking performance is discussed. State-of-the-art pose estimation performance is obtained on the Total Capture (mutli-view video and IMU) and Human 3.6M (multi-view video) datasets. Finally, a live demonstrator for the approach is presented showing real-time capture, solving and character animation using a light-weight, commodity hardware setup.
We describe a system for matching human posture (pose) across a large cross-media archive of dance footage spanning nearly 100 years, comprising digitized photographs and videos of rehearsals and performances. This footage presents unique challenges due to its age, quality and diversity. We propose a forest-like pose representation combining visual structure (self-similarity) descriptors over multiple scales, without explicitly detecting limb positions which would be infeasible for our data. We explore two complementary multi-scale representations, applying passage retrieval and latent Dirichlet allocation (LDA) techniques inspired by the the text retrieval domain, to the problem of pose matching. The result is a robust system capable of quickly searching large cross-media collections for similarity to a visually specified query pose. We evaluate over a crosssection of the UK National Research Centre for Dance’s (UK-NRCD), and the Siobhan Davies Replay’s (SDR) digital dance archives, using visual queries supplied by dance professionals. We demonstrate significant performance improvements over two base-lines; classical single and multi-scale Bag of Visual Words (BoVW) and spatial pyramid kernel (SPK) matching.
We present an efficient representation for sketch based image retrieval (SBIR) derived from a triplet loss convolutional neural network (CNN). We treat SBIR as a cross-domain modelling problem, in which a depiction invariant embedding of sketch and photo data is learned by regression over a siamese CNN architecture with half-shared weights and modified triplet loss function. Uniquely, we demonstrate the ability of our learned image descriptor to generalise beyond the categories of object present in our training data, forming a basis for general cross-category SBIR. We explore appropriate strategies for training, and for deriving a compact image descriptor from the learned representation suitable for indexing data on resource constrained e. g. mobile devices. We show the learned descriptors to outperform state of the art SBIR on the defacto standard Flickr15k dataset using a significantly more compact (56 bits per image, i. e. ≈ 105KB total) search index than previous methods.
We describe a non-parametric algorithm for multiple-viewpoint video inpainting. Uniquely, our algorithm addresses the domain of wide baseline multiple-viewpoint video (MVV) with no temporal look-ahead in near real time speed. A Dictionary of Patches (DoP) is built using multi-resolution texture patches reprojected from geometric proxies available in the alternate views. We dynamically update the DoP over time, and a Markov Random Field optimisation over depth and appearance is used to resolve and align a selection of multiple candidates for a given patch, this ensures the inpainting of large regions in a plausible manner conserving both spatial and temporal coherence. We demonstrate the removal of large objects (e.g. people) on challenging indoor and outdoor MVV exhibiting cluttered, dynamic backgrounds and moving cameras.
We propose an approach to accurately esti- mate 3D human pose by fusing multi-viewpoint video (MVV) with inertial measurement unit (IMU) sensor data, without optical markers, a complex hardware setup or a full body model. Uniquely we use a multi-channel 3D convolutional neural network to learn a pose em- bedding from visual occupancy and semantic 2D pose estimates from the MVV in a discretised volumetric probabilistic visual hull (PVH). The learnt pose stream is concurrently processed with a forward kinematic solve of the IMU data and a temporal model (LSTM) exploits the rich spatial and temporal long range dependencies among the solved joints, the two streams are then fused in a final fully connected layer. The two complemen- tary data sources allow for ambiguities to be resolved within each sensor modality, yielding improved accu- racy over prior methods. Extensive evaluation is per- formed with state of the art performance reported on the popular Human 3.6M dataset [26], the newly re- leased TotalCapture dataset and a challenging set of outdoor videos TotalCaptureOutdoor. We release the new hybrid MVV dataset (TotalCapture) comprising of multi- viewpoint video, IMU and accurate 3D skele- tal joint ground truth derived from a commercial mo- tion capture system. The dataset is available online at http://cvssp.org/data/totalcapture/.
We propose and evaluate several deep network architectures for measuring the similarity between sketches and photographs, within the context of the sketch based image retrieval (SBIR) task. We study the ability of our networks to generalize across diverse object categories from limited training data, and explore in detail strategies for weight sharing, pre-processing, data augmentation and dimensionality reduction. In addition to a detailed comparative study of network configurations, we contribute by describing a hybrid multi-stage training network that exploits both contrastive and triplet networks to exceed state of the art performance on several SBIR benchmarks by a significant margin.
We present a novel de-centralised service for proving the provenance of online digital identity, exposed as an assistive tool to help non-expert users make better decisions about whom to trust online. Our service harnesses the digital personhood (DP); the longitudinal and multi-modal signals created through users' lifelong digital interactions, as a basis for evidencing the provenance of identity. We describe how users may exchange trust evidence derived from their DP, in a granular and privacy-preserving manner, with other users in order to demonstrate coherence and longevity in their behaviour online. This is enabled through a novel secure infrastructure combining hybrid on- and off-chain storage combined with deep learning for DP analytics and visualization. We show how our tools enable users to make more effective decisions on whether to trust unknown third parties online, and also to spot behavioural deviations in their own social media footprints indicative of account hijacking.
The falling cost of digital cameras and camcorders has encouraged the creation of massive collections of personal digital media. However, once captured, this media is infrequently accessed and often lies dormant on users' PCs. We present a system to breathe life into home digital media collections, drawing upon artistic stylization to create a “Digital Ambient Display” that automatically selects, stylizes and transitions between digital contents in a semantically meaningful sequence. We present a novel algorithm based on multi-label graph cut for segmenting video into temporally coherent region maps. These maps are used to both stylize video into cartoons and paintings, and measure visual similarity between frames for smooth sequence transitions. The system automatically structures the media collection into a hierarchical representation based on visual content and semantics. Graph optimization is applied to adaptively sequence content for display in a coarse-to-fine manner, driven by user attention level (detected in real-time by a webcam). Our system is deployed on embedded hardware in the form of a compact digital photo frame. We demonstrate coherent segmentation and stylization over a variety of home videos and photos. We evaluate our media sequencing algorithm via a small-scale user study, indicating that our adaptive display conveys a more compelling media consumption experience than simple linear “slide-shows”.
We present TouchCut; a robust and efficient algorithm for segmenting image and video sequences with minimal user interaction. Our algorithm requires only a single finger touch to identify the object of interest in the image or first frame of video. Our approach is based on a level set framework, with an appearance model fusing edge, region texture and geometric information sampled local to the touched point. We first present our image segmentation solution, then extend this framework to progressive (per-frame) video segmentation, encouraging temporal coherence by incorporating motion estimation and a shape prior learned from previous frames. This new approach to visual object cut-out provides a practical solution for image and video segmentation on compact touch screen devices, facilitating spatially localized media manipulation. We describe such a case study, enabling users to selectively stylize video objects to create a hand-painted effect. We demonstrate the advantages of TouchCut by quantitatively comparing against the state of the art both in terms of accuracy, and run-time performance.
We present an image retrieval system for the interactive search of photo collections using free-hand sketches depicting shape. We describe Gradient Field HOG (GF-HOG); an adapted form of the HOG descriptor suitable for Sketch Based Image Retrieval (SBIR). We incorporate GF-HOG into a Bag of Visual Words (BoVW) retrieval framework, and demonstrate how this combination may be harnessed both for robust SBIR, and for localizing sketched objects within an image. We evaluate over a large Flickr sourced dataset comprising 33 shape categories, using queries from 10 non-expert sketchers. We compare GF-HOG against state-of-the-art descriptors with common distance measures and language models for image retrieval, and explore how affine deformation of the sketch impacts search performance. GF-HOG is shown to consistently outperform retrieval versus SIFT, multi-resolution HOG, Self Similarity, Shape Context and Structure Tensor. Further, we incorporate semantic keywords into our GF-HOG system to enable the use of annotated sketches for image search. A novel graph-based measure of semantic similarity is proposed and two applications explored: semantic sketch based image retrieval and a semantic photo montage.
We present EKILA; a decentralized framework that enables creatives to receive recognition and reward for their contributions to generative AI (GenAI). EKILA proposes a robust visual attribution technique and combines this with an emerging content provenance standard (C2PA) to address the problem of synthetic image provenance – determining the generative model and training data responsible for an AI-generated image. Furthermore, EKILA extends the non-fungible token (NFT) ecosystem to introduce a tokenized representation for rights, enabling a triangular relationship between the asset’s Ownership, Rights, and Attribution (ORA). Leveraging the ORA relationship enables creators to express agency over training consent and, through our attribution model, to receive apportioned credit, including royalty payments for the use of their assets in GenAI.
A real-time full-body motion capture system is presented which uses input from a sparse set of inertial measurement units (IMUs) along with images from two or more standard video cameras and requires no optical markers or specialized infra-red cameras. A real-time optimization-based framework is proposed which incorporates constraints from the IMUs, cameras and a prior pose model. The combination of video and IMU data allows the full 6-DOF motion to be recovered including axial rotation of limbs and drift-free global position. The approach was tested using both indoor and outdoor captured data. The results demonstrate the effectiveness of the approach for tracking a wide range of human motion in real time in unconstrained indoor/outdoor scenes.
We introduce the concept of 4D model flow for the precomputed alignment of dynamic surface appearance across 4D video sequences of different motions reconstructed from multi-view video. Precomputed 4D model flow allows the efficient parametrization of surface appearance from the captured videos, which enables efficient real-time rendering of interpolated 4D video sequences whilst accurately reproducing visual dynamics, even when using a coarse underlying geometry. We estimate the 4D model flow using an image-based approach that is guided by available geometry proxies. We propose a novel representation in surface texture space for efficient storage and online parametric interpolation of dynamic appearance. Our 4D model flow overcomes previous requirements for computationally expensive online optical flow computation for data-driven alignment of dynamic surface appearance by precomputing the appearance alignment. This leads to an efficient rendering technique that enables the online interpolation between 4D videos in real time, from arbitrary viewpoints and with visual quality comparable to the state of the art.
4D Video Textures (4DVT) introduce a novel representation for rendering video-realistic interactive character animation from a database of 4D actor performance captured in a multiple camera studio. 4D performance capture reconstructs dynamic shape and appearance over time but is limited to free-viewpoint video replay of the same motion. Interactive animation from 4D performance capture has so far been limited to surface shape only. 4DVT is the final piece in the puzzle enabling video-realistic interactive animation through two contributions: a layered view-dependent texture map representation which supports efficient storage, transmission and rendering from multiple view video capture; and a rendering approach that combines multiple 4DVT sequences in a parametric motion space, maintaining video quality rendering of dynamic surface appearance whilst allowing high-level interactive control of character motion and viewpoint. 4DVT is demonstrated for multiple characters and evaluated both quantitatively and through a user-study which confirms that the visual quality of captured video is maintained. The 4DVT representation achieves >90% reduction in size and halves the rendering cost.
We aim to simultaneously estimate the 3D articulated pose and high fidelity volumetric occupancy of human performance, from multiple viewpoint video (MVV) with as few as two views. We use a multi-channel symmetric 3D convolutional encoder-decoder with a dual loss to enforce the learning of a latent embedding that enables inference of skeletal joint positions and a volumetric reconstruction of the performance. The inference is regularised via a prior learned over a dataset of view-ablated multi-view video footage of a wide range of subjects and actions, and show this to generalise well across unseen subjects and actions. We demonstrate improved reconstruction accuracy and lower pose estimation error relative to prior work on two MVV performance capture datasets: Human 3.6M and TotalCapture.
We present StyleBabel, a unique open access dataset of natural language captions and free-form tags describing the artistic style of over 135K digital artworks, collected via a novel participatory method from experts studying at specialist art and design schools. StyleBabel was collected via an iterative method, inspired by `Grounded Theory': a qualitative approach that enables annotation while co-evolving a shared language for fine-grained artistic style attribute description. We demonstrate several downstream tasks for StyleBabel, adapting the recent ALADIN architecture for fine-grained style similarity, to train cross-modal embeddings for: 1) free-form tag generation; 2) natural language description of artistic style; 3) fine-grained text search of style. To do so, we extend ALADIN with recent advances in Visual Transformer (ViT) and cross-modal representation learning, achieving a state of the art accuracy in fine-grained style retrieval.
We present HyperNST; a neural style transfer (NST) technique for the artistic stylization of images, based on Hyper-networks and the StyleGAN2 architecture. Our contribution is a novel method for inducing style transfer parameterized by a metric space, pre-trained for style-based visual search (SBVS). We show for the first time that such space may be used to drive NST, enabling the application and interpolation of styles from an SBVS system. The technical contribution is a hyper-network that predicts weight updates to a StyleGAN2 pre-trained over a diverse gamut of artistic content (portraits), tailoring the style parameterization on a per-region basis using a semantic map of the facial regions. We show HyperNST to exceed state of the art in content preservation for our stylized content while retaining good style transfer performance
•Representation and method for evolutionary neural architecture search of encoder-decoder architectures for Deep Image prior,•Leveraging a state-of-the-art perceptual metric to guide the optimization.•State of the art DIP results for inpainting, denoising, up-scaling, beating the hand-optimized DIP architectures proposed.•Demonstrated the content- style dependency of DIP architectures. We present a neural architecture search (NAS) technique to enhance image denoising, inpainting, and super-resolution tasks under the recently proposed Deep Image Prior (DIP). We show that evolutionary search can automatically optimize the encoder-decoder (E-D) structure and meta-parameters of the DIP network, which serves as a content-specific prior to regularize these single image restoration tasks. Our binary representation encodes the design space for an asymmetric E-D network that typically converges to yield a content-specific DIP within 10-20 generations using a population size of 500. The optimized architectures consistently improve upon the visual quality of classical DIP for a diverse range of photographic and artistic content.
This response to the Law Commission Call for Evidence on The Changing Law on Ownership in Digital Assetsis written on behalf of DeCaDe, the UKRI funded Centre for the decentralised digital economy. DECaDE is a multi-disciplinary collaboration between the Universities of Surrey, Edinburgh and the Digital Catapult. https://decade.ac.uk/ The response was coordinated by Professor Burkhard Schafer, University of Edinburgh
This submission is made on behalf of DECaDE, the UKRI Centre for the Decentralised Digital Economy, and Creative Informatics, the Research and Development accelerator for the creative industries.
We describe a novel fully automatic algorithm for identifying salient objects in video based on their motion. Spatially coherent clusters of optical flow vectors are sampled to generate estimates of affine motion parameters local to super-pixels identified within each frame. These estimates, combined with spatial data, form coherent point distributions in a 5D solution space corresponding to objects or parts there-of. These distributions are temporally denoised using a particle filtering approach, and clustered to estimate the position and motion parameters of salient moving objects in the clip. We demonstrate localization of salient object/s in a variety of clips exhibiting moving and cluttered backgrounds.
We present an image retrieval system driven by free-hand sketched queries depicting shape. We introduce Gradient Field HoG (GF-HOG) as a depiction invariant image descriptor, encapsulating local spatial structure in the sketch and facilitating efficient codebook based retrieval. We show improved retrieval accuracy over 3 leading descriptors (Self Similarity, SIFT, HoG) across two datasets (Flickr160, ETHZ extended objects), and explain how GF-HOG can be combined with RANSAC to localize sketched objects within relevant images. We also demonstrate a prototype sketch driven photo montage application based on our system.
We present a fast technique for retrieving video clips using free-hand sketched queries. Visual keypoints within each video are detected and tracked to form short trajectories, which are clustered to form a set of spacetime tokens summarising video content. A Viterbi process matches a space-time graph of tokens to a description of colour and motion extracted from the query sketch. Inaccuracies in the sketched query are ameliorated by computing path cost using a Levenshtein (edit) distance. We evaluate over datasets of sports footage.
This paper presents a sketch-based image retrieval system using a bag-of-region representation of images. Regions from the nodes of a hierarchical region tree range in various scales of details. They have appealing properties for object level inference such as the naturally encoded shape and scale information of objects and the specified domains on which to compute features without being affected by clutter from outside the region. The proposed approach builds shape descriptor on the salient shape among the clutters and thus yields significant performance improvements over the previous results on three leading descriptors in Bag-of-Words framework for sketch based image retrieval. Matched region also facilitates the localization of sketched object within the retrieved image.
Content-aware image completion or in-painting is a fundamental tool for the correction of defects or removal of objects in images. We propose a non-parametric in-painting algorithm that enforces both structural and aesthetic (style) consistency within the resulting image. Our contributions are two-fold: 1) we explicitly disentangle image structure and style during patch search and selection to ensure a visually consistent look and feel within the target image. 2) we perform adaptive stylization of patches to conform the aesthetics of selected patches to the target image, so harmonizing the integration of selected patches into the final composition. We show that explicit consideration of visual style during in-painting delivers excellent qualitative and quantitative results across the varied image styles and content, over the Places2 scene photographic dataset and a challenging new in-painting dataset of artwork derived from BAM!
We present ALADIN (All Layer AdaIN); a novel architecture for searching images based on the similarity of their artistic style. Representation learning is critical to visual search, where distance in the learned search embedding reflects image similarity. Learning an embedding that discriminates fine-grained variations in style is hard, due to the difficulty of defining and labelling style. ALADIN takes a weakly supervised approach to learning a representation for fine-grained style similarity of digital artworks, leveraging BAM-FG, a novel large-scale dataset of user generated content groupings gathered from the web. ALADIN sets a new state of the art accuracy for style-based visual search over both coarse labelled style data (BAM) and BAM-FG; a new 2.62 million image dataset of 310,000 fine-grained style groupings also contributed by this work.
We present a novel algorithm for the semantic labeling of photographs shared via social media. Such imagery is diverse, exhibiting high intra-class variation that demands large training data volumes to learn representative classifiers. Unfortunately image annotation at scale is noisy resulting in errors in the training corpus that confound classifier accuracy. We show how evolutionary algorithms may be applied to select a ’purified’ subset of the training corpus to optimize classifier performance. We demonstrate our approach over a variety of image descriptors (including deeply learned features) and support vector machines.
This Pictorial documents the process of designing a device as an intervention within a field study of new parents. The device was deployed in participating parents’ homes to invite reflection on their everyday experiences of portraying self and others through social media in their transition to parenthood. The design creates a dynamic representation of each participant’s Facebook photo collection, extracting and amalgamating ‘faces’ from it to create an alternative portrait of an online self. We document the rationale behind our design, explaining how its features were inspired and developed, and how they function to address research questions about human experience.
We describe a semi-automatic video logging system, ca- pable of annotating frames with semantic metadata describ- ing the objects present. The system learns by visual exam- ples provided interactively by the logging operator, which are learned incrementally to provide increased automation over time. Transfer learning is initially used to bootstrap the sys- tem using relevant visual examples from ImageNet. We adapt the hard-assignment Bag of Word strategy for object recogni- tion to our interactive use context, showing transfer learning to significantly reduce the degree of interaction required.
We present a new incremental learning framework for realtime object recognition in video streams. ImageNet is used to bootstrap a set of one-vs-all incrementally trainable SVMs which are updated by user annotation events during streaming. We adopt an inductive transfer learning (ITL) approach to warp the video feature space to the ImageNet feature space, so enabling the incremental updates. Uniquely, the transformation used for the ITL warp is also learned incrementally using the same update events. We demonstrate a semiautomated video logging (SAVL) system using our incrementally learned ITL approach and show this to outperform existing SAVL which uses non-incremental transfer learning.
We describe a novel system for synthesising video choreography using sketched visual storyboards comprising human poses (stick men) and action labels. First, we describe an algorithm for searching archival dance footage using sketched pose. We match using an implicit representation of pose parsed from a mix of challenging low and high delity footage. In a training pre-process we learn a mapping between a set of exemplar sketches and corresponding pose representations parsed from the video, which are generalized at query-time to enable retrieval over previously unseen frames, and over additional unseen videos. Second, we describe how a storyboard of sketched poses, interspersed with labels indicating connecting actions, may be used to drive the synthesis of novel video choreography from the archival footage. We demonstrate both our retrieval and synthesis algorithms over both low delity PAL footage from the UK Digital Dance Archives (DDA) repository of contemporary dance, circa 1970, and over higher-defi nition studio captured footage.
We present 'Screen codes' - a space- and time-efficient, aesthetically compelling method for transferring data from a display to a camera-equipped mobile device. Screen codes encode data as a grid of luminosity fluctuations within an arbitrary image, displayed on the video screen and decoded on a mobile device. These 'twinkling' images are a form of 'visual hyperlink', by which users can move dynamically generated content to and from their mobile devices. They help bridge the 'content divide' between mobile and fixed computing.
We present a novel video retrieval system that accepts annotated free-hand sketches as queries. Existing sketch based video retrieval (SBVR) systems enable the appearance and movements of objects to be searched naturally through pictorial representations. Whilst visually expressive, such systems present an imprecise vehicle for conveying the semantics (e.g. object types) within a scene. Our contribution is to fuse the semantic richness of text with the expressivity of sketch, to create a hybrid `semantic sketch' based video retrieval system. Trajectory extraction and clustering are applied to pre-process each clip into a video object representation that we augment with object classification and colour information. The result is a system capable of searching videos based on the desired colour, motion path, and semantic labels of the objects present. We evaluate the performance of our system over the TSF dataset of broadcast sports footage.
We present the "empathie painting" - an interactive painterly rendering whose appearance adapts in real time to reflect the perceived emotional state of the viewer. The empathie painting is an experiment into the feasibility of using high level control parameters (namely, emotional state) to replace the plethora of low-level constraints users must typically set to affect the output of artistic rendering algorithms. We describe a suite of Computer Vision algorithms capable of recognising users' facial expressions through the detection of facial action units derived from the FACS scheme. Action units are mapped to vectors within a continuous 2D space representing emotional state, from which we in turn derive a continuous mapping to the style parameters of a simple but fast segmentation-based painterly rendering algorithm. The result is a digital canvas capable of smoothly varying its painterly style at approximately 4 frames per second, providing a novel user interactive experience using only commodity hardware.
Falls in the home are a major source of injury for the elderly. The affordability of commodity video cameras is prompting the development of ambient intelligent environments to monitor the occurence of falls in the home. This paper describes an automated fall detection system, capable of tracking movement and detecting falls in real-time. In particular we explore the application of the Bag of Features paradigm, frequently applied to general activity recognition in Computer Vision, to the domestic fall detection problem. We show that fall detection is feasible using such a framework, evaluted our approach in both controlled test scenarios and domestic scenarios exhibiting uncontrolled fall direction and visually cluttered environments.
In this paper, we propose an object segmentation algorithm driven by minimal user interactions. Compared to previous user-guided systems, our system can cut out the desired object in a given image with only a single finger touch minimizing user effort. The proposed model harnesses both edge and region based local information in an adaptive manner as well as geometric cues implied by the user-input to achieve fast and robust segmentation in a level set framework. We demonstrate the advantages of our method in terms of computational efficiency and accuracy comparing qualitatively and quantitatively with graph cut based techniques.
We present an algorithm for visually searching image collections using free-hand sketched queries. Prior sketch based image retrieval (SBIR) algorithms adopt either a category-level or fine-grain (instance-level) definition of cross-domain similarity—returning images that match the sketched object class (category-level SBIR), or a specific instance of that object (fine-grain SBIR). In this paper we take the middle-ground; proposing an SBIR algorithm that returns images sharing both the object category and key visual characteristics of the sketched query without assuming photo-approximate sketches from the user. We describe a deeply learned cross-domain embedding in which ‘mid-grain’ sketch-image similarity may be measured, reporting on the efficacy of unsupervised and semi-supervised manifold alignment techniques to encourage better intra-category (mid-grain) discrimination within that embedding. We propose a new mid-grain sketch-image dataset (MidGrain65c) and demonstrate not only mid-grain discrimination, but also improved category-level discrimination using our approach.
We present ARCHANGEL; a novel distributed ledger based system for assuring the long-term integrity of digital video archives. First, we describe a novel deep network architecture for computing compact temporal content hashes (TCHs) from audio-visual streams with durations of minutes or hours. Our TCHs are sensitive to accidental or malicious content modification (tampering) but invariant to the codec used to encode the video. This is necessary due to the curatorial requirement for archives to format shift video over time to ensure future accessibility. Second, we describe how the TCHs (and the models used to derive them) are secured via a proof-of-authority blockchain distributed across multiple independent archives. We report on the efficacy of ARCHANGEL within the context of a trial deployment in which the national government archives of the United Kingdom, Estonia and Norway participated.
We present a new algorithm for segmenting video frames into temporally stable colored regions, applying our technique to create artistic stylizations (e.g. cartoons and paintings) from real video sequences. Our approach is based on a multilabel graph cut applied to successive frames, in which the color data term and label priors are incrementally updated and propagated over time. We demonstrate coherent segmentation and stylization over a variety of home videos.
4D Video Textures (4DVT) introduce a novel representation for rendering video-realistic interactive character animation from a database of 4D actor performance captured in a multiple camera studio. 4D performance capture reconstructs dynamic shape and appearance over time but is limited to free-viewpoint video replay of the same motion. Interactive animation from 4D performance capture has so far been limited to surface shape only. 4DVT is the final piece in the puzzle enabling video-realistic interactive animation through two contributions: a layered view-dependent texture map representation which supports efficient storage, transmission and rendering from multiple view video capture; and a rendering approach that combines multiple 4DVT sequences in a parametric motion space, maintaining video quality rendering of dynamic surface appearance whilst allowing high-level interactive control of character motion and viewpoint. 4DVT is demonstrated for multiple characters and evaluated both quantitatively and through a user-study which confirms that the visual quality of captured video is maintained. The 4DVT representation achieves >90% reduction in size and halves the rendering cost.
We propose a human performance capture system employing convolutional neural networks (CNN) to estimate human pose from a volumetric representation of a performer derived from multiple view-point video (MVV).We compare direct CNN pose regression to the performance of an affine invariant pose descriptor learned by a CNN through a classification task. A non-linear manifold embedding is learned between the descriptor and articulated pose spaces, enabling regression of pose from the source MVV. The results are evaluated against ground truth pose data captured using a Vicon marker-based system and demonstrate good generalisation over a range of human poses, providing a system that requires no special suit to be worn by the performer.
We present a method for simultaneously estimating 3D hu- man pose and body shape from a sparse set of wide-baseline camera views. We train a symmetric convolutional autoencoder with a dual loss that enforces learning of a latent representation that encodes skeletal joint positions, and at the same time learns a deep representation of volumetric body shape. We harness the latter to up-scale input volumetric data by a factor of 4X, whilst recovering a 3D estimate of joint positions with equal or greater accuracy than the state of the art. Inference runs in real-time (25 fps) and has the potential for passive human behaviour monitoring where there is a requirement for high fidelity estimation of human body shape and pose.
We present a novel human performance capture technique capable of robustly estimating the pose (articulated joint positions) of a performer observed passively via multiple view-point video (MVV). An affine invariant pose descriptor is learned using a convolutional neural network (CNN) trained over volumetric data extracted from a MVV dataset of diverse human pose and appearance. A manifold embedding is learned via Gaussian Processes for the CNN descriptor and articulated pose spaces enabling regression and so estimation of human pose from MVV input. The learned descriptor and manifold are shown to generalise over a wide range of human poses, providing an efficient performance capture solution that requires no fiducials or other markers to be worn. The system is evaluated against ground truth joint configuration data from a commercial marker-based pose estimation system
We present ‘Screen codes’ - a space- and time-efficient, aesthetically compelling method for transferring data from a display to a camera-equipped mobile device. Screen codes encode data as a grid of luminosity fluctuations within an arbitrary image, displayed on the video screen and decoded on a mobile device. These ‘twinkling’ images are a form of ‘visual hyperlink’, by which users can move dynamically generated content to and from their mobile devices. They help bridge the ‘content divide’ between mobile and fixed computing.
LiveSketch is a novel algorithm for searching large image collections using hand-sketched queries. LiveSketch tackles the inherent ambiguity of sketch search by creating visual suggestions that augment the query as it is drawn, making query specification an iterative rather than one-shot process that helps disambiguate users' search intent. Our technical contributions are: a triplet convnet architecture that incorporates an RNN based variational autoencoder to search for images using vector (stroke-based) queries; real-time clustering to identify likely search intents (and so, targets within the search embedding); and the use of backpropagation from those targets to perturb the input stroke sequence, so suggesting alterations to the query in order to guide the search. We show improvements in accuracy and time-to-task over contemporary baselines using a 67M image corpus.
Falling hardware costs have prompted an explosion in casual video capture by domestic users. Yet, this video is infrequently accessed post-capture and often lies dormant on users’ PCs. We present a system to breathe life into home video repositories, drawing upon artistic stylization to create a “Digital Ambient Display” that automatically selects, stylizes and transitions between videos in a semantically meaningful sequence. We present a novel algorithm based on multi-label graph cut for segmenting video into temporally coherent region maps. These maps are used to both stylize video into cartoons and paintings, and measure visual similarity between frames for smooth sequence transitions. We demonstrate coherent segmentation and stylization over a variety of home videos.
We present a novel algorithm for stylizing photographs into portrait paintings comprised of curved brush strokes. Rather than drawing upon a prescribed set of heuristics to place strokes, our system learns a flexible model of artistic style by analyzing training data from a human artist. Given a training pair — a source image and painting of that image—a non-parametric model of style is learned by observing the geometry and tone of brush strokes local to image features. A Markov Random Field (MRF) enforces spatial coherence of style parameters. Style models local to facial features are learned using a semantic segmentation of the input face image, driven by a combination of an Active Shape Model and Graph-cut. We evaluate style transfer between a variety of training and test images, demonstrating a wide gamut of learned brush and shading styles.
We describe a novel algorithm for visually identifying the font used in a scanned printed document. Our algorithm requires no pre-recognition of characters in the string (i. e. optical character recognition). Gradient orientation features are collected local the character boundaries, and quantized into a hierarchical Bag of Visual Words representation. Following stop-word analysis, classification via logistic regression (LR) of the codebooked features yields per-character probabilities which are combined across the string to decide the posterior for each font. We achieve 93.4% accuracy over a 1000 font database of scanned printed text comprising Latin characters.
We describe a novel framework for segmenting a time- and view-coherent foreground matte sequence from synchronised multiple view video. We construct a Markov Random Field (MRF) comprising links between superpixels corresponded across views, and links between superpixels and their constituent pixels. Texture, colour and disparity cues are incorporated to model foreground appearance. We solve using a multi-resolution iterative approach enabling an eight view high definition (HD) frame to be processed in less than a minute. Furthermore we incorporate a temporal diffusion process introducing a prior on the MRF using information propagated from previous frames, and a facility for optional user correction. The result is a set of temporally coherent mattes that are solved for simultaneously across views for each frame, exploiting similarities across views and time.
We present a novel steganographic technique for concealing information within an image. Uniquely we explore the practicality of hiding, and recovering, data within the pattern of brush strokes generated by a non-photorealistic rendering (NPR) algorithm. We incorporate a linear binary coding (LBC) error correction scheme over a raw data channel established through the local statistics of NPR stroke orientations. This enables us to deliver a robust channel for conveying short (e.g. 30 character) strings covertly within a painterly rendering. We evaluate over a variety of painterly renderings, parameter settings and message lengths.
A real-time full-body motion capture system is presented which uses input from a sparse set of inertial measurement units (IMUs) along with images from two or more standard video cameras and requires no optical markers or specialized infra-red cameras. A real-time optimization-based framework is proposed which incorporates constraints from the IMUs, cameras and a prior pose model. The combination of video and IMU data allows the full 6-DOF motion to be recovered including axial rotation of limbs and drift-free global position. The approach was tested using both indoor and outdoor captured data. The results demonstrate the effectiveness of the approach for tracking a wide range of human motion in real time in unconstrained indoor/outdoor scenes.
We describe a mobile augmented reality application that is based on 3D snapshotting using multiple photographs. Optical square markers provide the anchor for reconstructed virtual objects in the scene. A novel approach based on pixel flow highly improves tracking performance. This dual tracking approach also allows for a new single-button user interface metaphor for moving virtual objects in the scene. The development of the AR viewer was accompanied by user studies confirming the chosen approach.
Deep Learning methods are currently the state-of-the-art in many Computer Vision and Image Processing problems, in particular image classification. After years of intensive investigation, a few models matured and became important tools, including Convolutional Neural Networks (CNNs), Siamese and Triplet Networks, Auto-Encoders (AEs) and Generative Adversarial Networks (GANs). The field is fast-paced and there is a lot of terminologies to catch up for those who want to adventure in Deep Learning waters. This paper has the objective to introduce the most fundamental concepts of Deep Learning for Computer Vision in particular CNNs, AEs and GANs, including architectures, inner workings and optimization. We offer an updated description of the theoretical and practical knowledge of working with those models. After that, we describe Siamese and Triplet Networks, not often covered in tutorial papers, as well as review the literature on recent and exciting topics such as visual stylization, pixel-wise prediction and video processing. Finally, we discuss the limitations of Deep Learning for Computer Vision.
We present a scalable system for sketch-based image retrieval (SBIR), extending the state of the art Gradient Field HoG (GF-HoG) retrieval framework through two technical contributions. First, we extend GF-HoG to enable color-shape retrieval and comprehensively evaluate several early-and late-fusion approaches for integrating the modality of color, considering both the accuracy and speed of sketch retrieval. Second, we propose an efficient inverse-index representation for GF-HoG that delivers scalable search with interactive query times over millions of images. A mobile app demo accompanies this paper (Android).
This guide s cutting-edge coverage explains the full spectrum of NPR techniques used in photography, TV and film.
We propose a novel measure of visual similarity for image retrieval that incorporates both structural and aesthetic (style) constraints. Our algorithm accepts a query as sketched shape, and a set of one or more contextual images specifying the desired visual aesthetic. A triplet network is used to learn a feature embedding capable of measuring style similarity independent of structure, delivering significant gains over previous networks for style discrimination. We incorporate this model within a hierarchical triplet network to unify and learn a joint space from two discriminatively trained streams for style and structure. We demonstrate that this space enables, for the first time, style-constrained sketch search over a diverse domain of digital artwork comprising graphics, paintings and drawings. We also briefly explore alternative query modalities.
We present an algorithm for fusing multi-viewpoint video (MVV) with inertial measurement unit (IMU) sensor data to accurately estimate 3D human pose. A 3-D convolutional neural network is used to learn a pose embedding from volumetric probabilistic visual hull data (PVH) derived from the MVV frames. We incorporate this model within a dual stream network integrating pose embeddings derived from MVV and a forward kinematic solve of the IMU data. A temporal model (LSTM) is incorporated within both streams prior to their fusion. Hybrid pose inference using these two complementary data sources is shown to resolve ambiguities within each sensor modality, yielding improved accuracy over prior methods. A further contribution of this work is a new hybrid MVV dataset (TotalCapture) comprising video, IMU and a skeletal joint ground truth derived from a commercial motion capture system. The dataset is available online at http://cvssp.org/data/totalcapture/.
We propose a novel video inpainting algorithm that simultaneously hallucinates missing appearance and motion (optical flow) information, building upon the recent 'Deep Image Prior' (DIP) that exploits convolutional network architectures to enforce plausible texture in static images. In extending DIP to video we make two important contributions. First, we show that coherent video inpainting is possible without a priori training. We take a generative approach to inpainting based on internal (within-video) learning without reliance upon an external corpus of visual data to train a one-size-fits-all model for the large space of general videos. Second, we show that such a framework can jointly generate both appearance and flow, whilst exploiting these complementary modalities to ensure mutual consistency. We show that leveraging appearance statistics specific to each video achieves visually plausible results whilst handling the challenging problem of long-term consistency.
We present a novel Content Based Video Retrieval (CBVR) system, driven by free-hand sketch queries depicting both objects and their movement (via dynamic cues; streak-lines and arrows). Our main contribution is a probabilistic model of video clips (based on Linear Dynamical Systems), leading to an algorithm for matching descriptions of sketched objects to video. We demonstrate our model fitting to clips under static and moving camera conditions, exhibiting linear and oscillatory motion. We evaluate retrieval on two real video data sets, and on a video data set exhibiting controlled variation in shape, color, motion and clutter.
We present an algorithm for searching image collections using free-hand sketches that describe the appearance and relative positions of multiple objects. Sketch based image retrieval (SBIR) methods predominantly match queries containing a single, dominant object invariant to its position within an image. Our work exploits drawings as a concise and intuitive representation for specifying entire scene compositions. We train a convolutional neural network (CNN) to encode masked visual features from sketched objects, pooling these into a spatial descriptor encoding the spatial relationships and appearances of objects in the composition. Training the CNN backbone as a Siamese network under triplet loss yields a metric search embedding for measuring compositional similarity which may be efficiently leveraged for visual search by applying product quantization.
We present a novel architecture for comparing a pair of images to identify image regions that have been subjected to editorial manipulation. We first describe a robust near-duplicate search, for matching a potentially manipulated image circulating online to an image within a trusted database of originals. We then describe a novel architecture for comparing that image pair, to localize regions that have been manipulated to differ from the retrieved original. The localization ignores discrepancies due to benign image transformations that commonly occur during online redistribution. These include artifacts due to noise and recom-pression degradation, as well as out-of-place transformations due to image padding, warping, and changes in size and shape. Robustness towards out-of-place transformations is achieved via the end-to-end training of a differen-tiable warping module within the comparator architecture. We demonstrate effective retrieval and comparison of benign transformed and manipulated images, over a dataset of millions of photographs.
Multi-view video acquisition is widely used for reconstruction and free-viewpoint rendering of dynamic scenes by directly resampling from the captured images. This paper addresses the problem of optimally resampling and representing multi-view video to obtain a compact representation without loss of the view-dependent dynamic surface appearance. Spatio-temporal optimisation of the multi-view resampling is introduced to extract a coherent multi-layer texture map video. This resampling is combined with a surface-based optical flow alignment between views to correct for errors in geometric reconstruction and camera calibration which result in blurring and ghosting artefacts. The multi-view alignment and optimised resampling results in a compact representation with minimal loss of information allowing high-quality free-viewpoint rendering. Evaluation is performed on multi-view datasets for dynamic sequences of cloth, faces and people. The representation achieves >90% compression without significant loss of visual quality.
We present ARCHANGEL; a decentralised platform for ensuring the long-term integrity of digital documents stored within public archives. Document integrity is fundamental to public trust in archives. Yet currently that trust is built upon institutional reputation --- trust at face value in a centralised authority, like a national government archive or University. ARCHANGEL proposes a shift to a technological underscoring of that trust, using distributed ledger technology (DLT) to cryptographically guarantee the provenance, immutability and so the integrity of archived documents. We describe the ARCHANGEL architecture, and report on a prototype of that architecture build over the Ethereum infrastructure. We report early evaluation and feedback of ARCHANGEL from stakeholders in the research data archives space.
The contribution of this paper is a novel non-photorealistic rendering (NPR) technique, capable of producing an artificial 'hand-painted' effect on 2D images, such as photographs. Our method requires no user interaction, and makes use of image salience and gradient information to determine the implicit ordering and attributes of individual brush strokes. The benefits of our technique are complete automation, and mitigation against the loss of image detail during painting. Strokes from lower salience regions of the image do not encroach upon higher salience regions; this can occur with some existing painting methods. We describe our algorithm in detail, and illustrate its application with a gallery of images.
Bag-of-visual words (BOVW) is a local feature based framework for content-based image and video retrieval. Its performance relies on the discriminative power of visual vocabulary, i.e. the cluster set on local features. However, the optimisation of visual vocabulary is of a high complexity in a large collection. This paper aims to relax such a dependence by adapting the query generative model to BOVW based retrieval. Local features are directly projected onto latent content topics to create effective visual queries; visual word distributions are learnt around local features to estimate the contribution of a visual word to a query topic; the relevance is justified by considering concept distributions on visual words as well as on local features. Massive experiments are carried out the TRECVid 2009 collection. The notable improvement on retrieval performance shows that this probabilistic framework alleviates the problem of visual ambiguity and is able to afford visual vocabulary with relatively low discriminative power.
We present a novel method for generating robust adversarial image examples building upon the recent ‘deep image prior’ (DIP) that exploits convolutional network architectures to enforce plausible texture in image synthesis. Adversarial images are commonly generated by perturbing images to introduce high frequency noise that induces image misclassification, but that is fragile to subsequent digital manipulation of the image. We show that using DIP to reconstruct an image under adversarial constraint induces perturbations that are more robust to affine deformation, whilst remaining visually imperceptible. Furthermore we show that our DIP approach can also be adapted to produce local adversarial patches (‘adversarial stickers’). We demonstrate robust adversarial examples over a broad gamut of images and object classes drawn from the ImageNet dataset.
We present a convolutional autoencoder that enables high fidelity volumetric reconstructions of human performance to be captured from multi-view video comprising only a small set of camera views. Our method yields similar end-to-end reconstruction error to that of a prob- abilistic visual hull computed using significantly more (double or more) viewpoints. We use a deep prior implicitly learned by the autoencoder trained over a dataset of view-ablated multi-view video footage of a wide range of subjects and actions. This opens up the possibility of high-end volumetric performance capture in on-set and prosumer scenarios where time or cost prohibit a high witness camera count.
We present an image retrieval system driven by free-hand sketched queries depicting shape. We introduce Gradient Field HoG (GF-HOG) as a depiction invariant image descriptor, encapsulating local spatial structure in the sketch and facilitating efficient codebook based retrieval. We show improved retrieval accuracy over 3 leading descriptors (Self Similarity, SIFT, HoG) across two datasets (Flickr160, ETHZ extended objects), and explain how GF-HOG can be combined with RANSAC to localize sketched objects within relevant images. We also demonstrate a prototype sketch driven photo montage application based on our system.
We introduce a trainable system that simultaneously filters and classifies low-level features into types specified by the user. The system operates over full colour images, and outputs a vector at each pixel indicating the probability that the pixel belongs to each feature type. We explain how common features such as edge, corner, and ridge can all be detected within a single framework, and how we combine these detectors using simple probability theory. We show its efficacy, using stereo-matching as an example.
Scene Designer is a novel method for searching and generating images using free-hand sketches of scene compositions; i.e. drawings that describe both the appearance and relative positions of objects. Our core contribution is a single unified model to learn both a cross-modal search embedding for matching sketched compositions to images, and an object embedding for layout synthesis. We show that a graph neural network (GNN) followed by Transformer under our novel contrastive learning setting is required to allow learning correlations between object type, appearance and arrangement, driving a mask generation module that synthesizes coherent scene layouts, whilst also delivering state of the art sketch based visual search of scenes.
Images tell powerful stories but cannot always be trusted. Matching images back to trusted sources (attribution) enables users to make a more informed judgment of the images they encounter online. We propose a robust image hashing algorithm to perform such matching. Our hash is sensitive to manipulation of subtle, salient visual details that can substantially change the story told by an image. Yet the hash is invariant to benign transformations (changes in quality, codecs, sizes, shapes, etc.) experienced by images during online redistribution. Our key contribution is OSCAR-Net1 (Object-centric Scene Graph Attention for Image Attribution Network); a robust image hashing model inspired by recent successes of Transformers in the visual domain. OSCAR-Net constructs a scene graph representation that attends to fine-grained changes of every object’s visual appearance and their spatial relationships. The network is trained via contrastive learning on a dataset of original and manipulated images yielding a state of the art image hash for content fingerprinting that scales to millions of images