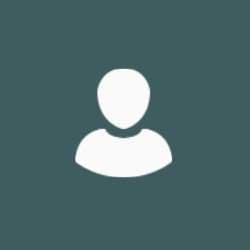
Dr Brian Gardner
About
Biography
I completed my Masters in Physics at the University of Exeter in 2011. I then obtained my PhD in Computer Science from the University of Surrey in 2016: concerning the theoretical aspects of spiking neural networks (supervised by Prof. André Grüning). Currently I am employed as a Research Fellow in the School of Veterinary Medicine, where I'm involved with computational biology research on the topics of antibiotic resistance prediction and mathematical modelling of microbial communities.
ResearchResearch interests
- Mathematical biology
- Computational neuroscience
- Machine learning
- Neural networks
- Synaptic plasticity
Research interests
- Mathematical biology
- Computational neuroscience
- Machine learning
- Neural networks
- Synaptic plasticity
Publications
Background Human, animal, and environmental health are increasingly threatened by the emergence and spread of antibiotic resistance. Inappropriate use of antibiotic treatments commonly contributes to this threat, but it is also becoming apparent that multiple, interconnected environmental factors can play a significant role. Thus, a One Health approach is required for a comprehensive understanding of the environmental dimensions of antibiotic resistance and inform science-based decisions and actions. The broad and multidisciplinary nature of the problem poses several open questions drawing upon a wide heterogeneous range of studies. Objective This study seeks to collect and catalogue the evidence of the potential effects of environmental factors on the abundance or detection of antibiotic resistance determinants in the outdoor environment, i.e., antibiotic resistant bacteria and mobile genetic elements carrying antibiotic resistance genes, and the effect on those caused by local environmental conditions of either natural or anthropogenic origin. Methods Here, we describe the protocol for a systematic evidence map to address this, which will be performed in adherence to best practice guidelines. We will search the literature from 1990 to present, using the following electronic databases: MEDLINE, Embase, and the Web of Science Core Collection as well as the grey literature. We shall include full-text, scientific articles published in English. Reviewers will work in pairs to screen title, abstract and keywords first and then full-text documents. Data extraction will adhere to a code book purposely designed. Risk of bias assessment will not be conducted as part of this SEM. We will combine tables, graphs, and other suitable visualisation techniques to compile a database i) of studies investigating the factors associated with the prevalence of antibiotic resistance in the environment and ii) map the distribution, network, cross-disciplinarity, impact and trends in the literature.
Predicting Antimicrobial Resistance (AMR) from genomic sequence data has become a significant component of overcoming the AMR challenge, especially given its potential for facilitating more rapid diagnostics and personalised antibiotic treatments. With the recent advances in sequencing technologies and computing power, deep learning models for genomic sequence data have been widely adopted to predict AMR more reliably and error-free. There are more than 30 different types of AMR; therefore, any practical AMR prediction system must be able to identify multiple AMRs present in a genomic sequence. Unfortunately, most genomic sequence datasets do not have all the labels marked, thereby making a deep learning modelling approach challenging owing to its reliance on labels for reliability and accuracy. This paper addresses this issue by presenting an effective deep learning solution, Mask-Loss 1D convolution neural network (ML-ConvNet), for AMR prediction on datasets with many missing labels. The core component of ML-ConvNet utilises a masked loss function that overcomes the effect of missing labels in predicting AMR. The proposed ML-ConvNet is demonstrated to outperform state-of-the-art methods in the literature by 10.5%, according to the F1 score. The proposed model's performance is evaluated using different degrees of the missing label and is found to outperform the conventional approach by 76% in the F1 score when 86.68% of labels are missing. Furthermore, the proposed ML-ConvNet is established with an explainable artificial intelligence (XAI) pipeline, thereby making it ideally suited for hospitals and healthcare settings where model interpretability is an essential requirement.
Predicting Antimicrobial Resistance (AMR) from genomic data has important implications for human and animal healthcare, and especially given its potential for more rapid diagnostics and informed treatment choices. With the recent advances in sequencing technologies, applying machine learning techniques for AMR prediction have indicated promising results. Despite this, there are shortcomings in the literature concerning methodologies suitable for multi-drug AMR prediction and especially where samples with missing labels exist. To address this shortcoming, we introduce a Rectified Classifier Chain (RCC) method for predicting multi-drug resistance. This RCC method was tested using annotated features of genomics sequences and compared with similar multi-label classification methodologies. We found that applying the eXtreme Gradient Boosting (XGBoost) base model to our RCC model outperformed the second-best model, XGBoost based binary relevance model, by 3.3% in Hamming accuracy and 7.8% in F1-score. Additionally, we note that in the literature machine learning models applied to AMR prediction typically are unsuitable for identifying biomarkers informative of their decisions; in this study, we show that biomarkers contributing to AMR prediction can also be identified using the proposed RCC method. We expect this can facilitate genome annotation and pave the path towards identifying new biomarkers indicative of AMR.
CTX-M ESBL-producing E. coli is an increasing AMR public health issue with the transmission between animals and humans via zoonotic pathogens now a major area of interest. Accurate and timely identification of ESBL-expressing E. coli CTX-M variants is essential for disease monitoring, targeted antibiotic treatment and infection control. Cefotaximase-Munich (CTX-M) extended-spectrum beta-lactamase (ESBL) enzymes produced by Enterobacteriaceae confer resistance to clinically relevant third-generation cephalosporins. CTX-M group 1 variants, CTX-M-1 and CTX-M-15, are the leading ESBL-producing Enterobacteriaceae associated with animal and human infection, respectively, and are an increasing antimicrobial resistance (AMR) global health concern. The bla(CTX-M-1) and bla(CTX-M-15) genes encoding these variants have an approximate nucleotide sequence similarity of 98.7%, making effective differential diagnostic monitoring difficult. Loop-primer endonuclease cleavage loop-mediated isothermal amplification (LEC-LAMP) enables rapid real-time multiplex pathogen detection with single-base specificity and portable on-site testing. We have developed an internally controlled multiplex CTX-M-1/15 LEC-LAMP assay for the differential detection of bla(CTX-M-1) and bla(CTX-M-15). Assay analytical specificity was established using a panel of human, animal, and environmental Escherichia coli isolates positive for bla(CTX-M-1) (n = 18), bla(CTX-M-15) (n = 35), and other closely related bla(CTX-Ms) (n = 38) from Ireland, Germany, and Portugal, with analytical sensitivity determined using probit regression analysis. Animal fecal sample testing using the CTX-M-1/15 LEC-LAMP assay in combination with a rapid DNA extraction protocol was carried out on porcine fecal samples previously confirmed to be PCR-positive for E. coli bla(CTX-M). Portable instrumentation was used to further analyze each fecal sample and demonstrate the on-site testing capabilities of the LEC-LAMP assay with the rapid DNA extraction protocol. The CTX-M-1/15 LEC-LAMP assay demonstrated complete analytical specificity for the differential detection of both variants with sensitive low-level detection of 8.5 and 9.8 copies per reaction for bla(CTX-M-1) and bla(CTX-M-15), respectively, and E. coli bla(CTX-M-1) was identified in all bla(CTX-M) positive porcine fecal samples tested.IMPORTANCE CTX-M ESBL-producing E. coli is an increasing AMR public health issue with the transmission between animals and humans via zoonotic pathogens now a major area of interest. Accurate and timely identification of ESBL-expressing E. coli CTX-M variants is essential for disease monitoring, targeted antibiotic treatment and infection control. This study details the first report of portable diagnostics technology for the rapid differential detection of CTX-M AMR markers bla(CTX-M-1) and bla(CTX-M-15), facilitating improved identification and surveillance of these closely related variants. Further application of this portable internally controlled multiplex CTX-M-1/15 LEC-LAMP assay will provide new information on the transmission and prevalence of these CTX-M ESBL alleles. Furthermore, this transferable diagnostic technology can be applied to other new and emerging relevant AMR markers of interest providing more efficient and specific portable pathogen detection for improved epidemiological surveillance.
Precise spike timing as a means to encode information in neural networks is biologically supported, and is advantageous over frequency-based codes by processing input features on a much shorter time-scale. For these reasons, much recent attention has been focused on the development of supervised learning rules for spiking neural networks that utilise a temporal coding scheme. However, despite significant progress in this area, there still lack rules that are theoretically justified, and yet can be considered biologically relevant. Here we examine the general conditions under which optimal synaptic plasticity takes place to support the supervised learning of a precise temporal code. As part of our analysis we introduce two analytically derived learning rules, one of which relies on an instantaneous error signal to optimise synaptic weights in a network (INST rule), and the other one relying on a filtered error signal to minimise the variance of synaptic weight modifications (FILT rule). We test the optimality of the solutions provided by each rule with respect to their temporal encoding precision, and then measure the maximum number of input patterns they can learn to memorise using the precise timings of individual spikes. Our results demonstrate the optimality of the FILT rule in most cases, underpinned by the rule's error-filtering mechanism which provides smooth convergence during learning. We also find the FILT rule to be most efficient at performing input pattern memorisations, and most noticeably when patterns are identified using spikes with sub-millisecond temporal precision. In comparison with existing work, we determine the performance of the FILT rule to be consistent with that of the highly efficient E-learning Chronotron rule, but with the distinct advantage that our FILT rule is also implementable as an online method for increased biological realism.
Reward-modulated learning rules for spiking neural networks have emerged, that have been demonstrated to solve a wide range of reinforcement learning tasks. Despite this, little work has aimed to classify spike patterns by the timing of output spikes. Here, we apply a rewardmaximising learning rule to teach a spiking neural network to classify input patterns by the latency of output spikes. Furthermore, we compare the performance of two escape rate functions that drive output spiking activity: the Arrhenius & Current (A&C) model and Exponential (EXP) model. We find A&C consistently outperforms EXP, and especially in terms of the time taken to converge in learning. We also show that jittering input patterns with a low noise amplitude leads to an improvement in learning, by reducing the variation in the performance. © 2014 Springer International Publishing Switzerland.
Experimental studies support the notion of spike-based neuronal information processing in the brain, with neural circuits exhibiting a wide range of temporally-based coding strategies to rapidly and efficiently represent sensory stimuli. Accordingly, it would be desirable to apply spike-based computation to tackling real-world challenges, and in particular transferring such theory to neuromorphic systems for low-power embedded applications. Motivated by this, we propose a new supervised learning method that can train multilayer spiking neural networks to solve classification problems based on a rapid, first-to-spike decoding strategy. The proposed learning rule supports multiple spikes fired by stochastic hidden neurons, and yet is stable by relying on first-spike responses generated by a deterministic output layer. In addition to this, we also explore several distinct, spike-based encoding strategies in order to form compact representations of presented input data. We demonstrate the classification performance of the learning rule as applied to several benchmark datasets, including MNIST. The learning rule is capable of generalizing from the data, and is successful even when used with constrained network architectures containing few input and hidden layer neurons. Furthermore, we highlight a novel encoding strategy, termed “scanline encoding,” that can transform image data into compact spatiotemporal patterns for subsequent network processing. Designing constrained, but optimized, network structures and performing input dimensionality reduction has strong implications for neuromorphic applications.
Information encoding in the nervous system is supported through the precise spike timings of neurons; however, an understanding of the underlying processes by which such representations are formed in the first place remains an open question. Here we examine how multilayered networks of spiking neurons can learn to encode for input patterns using a fully temporal coding scheme. To this end, we introduce a new supervised learning rule, MultilayerSpiker, that can train spiking networks containing hidden layer neurons to perform transformations between spatiotemporal input and output spike patterns. The performance of the proposed learning rule is demonstrated in terms of the number of pattern mappings it can learn, the complexity of network structures it can be used on, and its classification accuracy when using multispike-based encodings. In particular, the learning rule displays robustness against input noise and can generalize well on an example data set. Our approach contributes to both a systematic understanding of how computations might take place in the nervous system and a learning rule that displays strong technical capability.
Precise spike timing as a means to encode information in neural networks is biologically supported, and is advantageous over frequency-based codes by processing input features on a much shorter time-scale. For these reasons, much recent attention has been focused on the development of supervised learning rules for spiking neural networks that utilise a temporal coding scheme. However, despite significant progress in this area, there still lack rules that have a theoretical basis, and yet can be considered biologically relevant. Here we examine the general conditions under which synaptic plasticity most effectively takes place to support the supervised learning of a precise temporal code. As part of our analysis we examine two spike-based learning methods: one of which relies on an instantaneous error signal to modify synaptic weights in a network (INST rule), and the other one relying on a filtered error signal for smoother synaptic weight modifications (FILT rule). We test the accuracy of the solutions provided by each rule with respect to their temporal encoding precision, and then measure the maximum number of input patterns they can learn to memorise using the precise timings of individual spikes as an indication of their storage capacity. Our results demonstrate the high performance of the FILT rule in most cases, underpinned by the rule’s error-filtering mechanism, which is predicted to provide smooth convergence towards a desired solution during learning. We also find the FILT rule to be most efficient at performing input pattern memorisations, and most noticeably when patterns are identified using spikes with sub-millisecond temporal precision. In comparison with existing work, we determine the performance of the FILT rule to be consistent with that of the highly efficient E-learning Chronotron rule, but with the distinct advantage that our FILT rule is also implementable as an online method for increased biological realism.