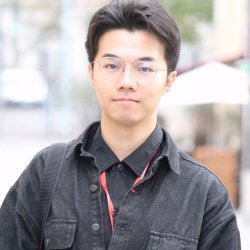
Haohe Liu
About
My research project
Automatic sound labelling for broadcast audioI’m Haohe Liu, a first-year Ph.D. student at the Centre for Vision Speech and Signal Processing (CVSSP), University of Surrey. My research includes topics related to speech, music, and general audio. I like 🎧 🎼 🎹 🏃🏼 🏂🏼 in my spare time. Most of my studies are fully open-sourced. I’m also interested in/researching on some other novel topics on audio. If you would like to discuss or cooperate, please drop me an email.
Highlighted research as the first author:
- AudioLDM: State-of-the-art text-to-audio generation model.
- NaturalSpeech: First text-to-speech model that achieves on par CMOS with human recording.
- VoiceFixer: Restore the quality of human speech signal regardless how the signal is degraded.
- CWS-PResUNet: The music source separation system that achieves leading performance on Music Demixing Challenge 2021.
- NVSR: Speech super-resolution.
- DiffRes: A module that make temporal-resolution of the spectrogram differentiable for efficient audio classificaiton.
- Few-shot bioacoustic detection: The 2rd ranking system in the DCASE 2022 Challenge Task 5.
- …
At the University of Surrey, I am fortunate to be co-advised by Prof. Mark D. Plumbley and Prof. Wenwu Wang. And I’m lucky to be jointly funded by BBC Research & Development (R&D) and the Doctoral College. In the CVSSP, I’m working as part of the AI for Sound project with the goal of developing new methods for automatic labeling of sound environments and events in broadcast audio, assisting production staff to find and search through content, and helping the general public access archive content. I’m also working closely with BBC R&D Audio Team on putting our audio recognition algorithms into production, such as generating machine tags in BBC sound effect library.
Internship
2021.10-2022.04 Microsoft Research Asia, Beijing - Research Intern - with Xu Tan
2020.07-2021.10 ByteDance AI lab, Shanghai - Research Intern - with Qiuqiang Kong
Challenges
- (main contributor) 1st in DCASE 2022 Challenge Task 5:Few-shot Bioacoustic Event Detection. [code][details][leaderboard]
- (main contributor) 2nd on the vocal score and 5th place on the overall score in ISMIR 2021 Music Demixing Challenge. [code][details] [leaderboard]
- 3rd in DCASE 2022 Challenge Task 6A: Automated Audio Captioning.
- 2nd in DCASE 2022 Challenge Task 6B: Language-Based Audio Retrieval.
Supervisors
I’m Haohe Liu, a first-year Ph.D. student at the Centre for Vision Speech and Signal Processing (CVSSP), University of Surrey. My research includes topics related to speech, music, and general audio. I like 🎧 🎼 🎹 🏃🏼 🏂🏼 in my spare time. Most of my studies are fully open-sourced. I’m also interested in/researching on some other novel topics on audio. If you would like to discuss or cooperate, please drop me an email.
Highlighted research as the first author:
- AudioLDM: State-of-the-art text-to-audio generation model.
- NaturalSpeech: First text-to-speech model that achieves on par CMOS with human recording.
- VoiceFixer: Restore the quality of human speech signal regardless how the signal is degraded.
- CWS-PResUNet: The music source separation system that achieves leading performance on Music Demixing Challenge 2021.
- NVSR: Speech super-resolution.
- DiffRes: A module that make temporal-resolution of the spectrogram differentiable for efficient audio classificaiton.
- Few-shot bioacoustic detection: The 2rd ranking system in the DCASE 2022 Challenge Task 5.
- …
At the University of Surrey, I am fortunate to be co-advised by Prof. Mark D. Plumbley and Prof. Wenwu Wang. And I’m lucky to be jointly funded by BBC Research & Development (R&D) and the Doctoral College. In the CVSSP, I’m working as part of the AI for Sound project with the goal of developing new methods for automatic labeling of sound environments and events in broadcast audio, assisting production staff to find and search through content, and helping the general public access archive content. I’m also working closely with BBC R&D Audio Team on putting our audio recognition algorithms into production, such as generating machine tags in BBC sound effect library.
Internship
2021.10-2022.04 Microsoft Research Asia, Beijing - Research Intern - with Xu Tan
2020.07-2021.10 ByteDance AI lab, Shanghai - Research Intern - with Qiuqiang Kong
Challenges
- (main contributor) 1st in DCASE 2022 Challenge Task 5:Few-shot Bioacoustic Event Detection. [code][details][leaderboard]
- (main contributor) 2nd on the vocal score and 5th place on the overall score in ISMIR 2021 Music Demixing Challenge. [code][details] [leaderboard]
- 3rd in DCASE 2022 Challenge Task 6A: Automated Audio Captioning.
- 2nd in DCASE 2022 Challenge Task 6B: Language-Based Audio Retrieval.
Teaching
2023/02-2023/05 Demonstrator for EEEM068 Applied Machine Learning
2022/10-2023/01 Demonstrator for EEE1033 Computer and Digital Logic
2022/10-2023/01 Demonstrator for EEE3008 Fundamentals of Digital Signal Processing
Publications
Audio captioning aims at using language to describe the content of an audio clip. Existing audio captioning systems are generally based on an encoder-decoder architecture, in which acoustic information is extracted by an audio encoder and then a language decoder is used to generate the captions. Training an audio captioning system often encounters the problem of data scarcity. Transferring knowledge from pre-trained audio models such as Pre-trained Audio Neural Networks (PANNs) have recently emerged as a useful method to mitigate this issue. However, there is less attention on exploiting pre-trained language models for the decoder, compared with the encoder. BERT is a pre-trained language model that has been extensively used in natural language processing tasks. Nevertheless, the potential of using BERT as the language decoder for audio captioning has not been investigated. In this study, we demonstrate the efficacy of the pre-trained BERT model for audio captioning. Specifically, we apply PANNs as the encoder and initialize the decoder from the publicly available pre-trained BERT models. We conduct an empirical study on the use of these BERT models for the decoder in the audio captioning model. Our models achieve competitive results with the existing audio captioning methods on the AudioCaps dataset.
Language-queried audio source separation (LASS) is a new paradigm for computational auditory scene analysis (CASA). LASS aims to separate a target sound from an audio mixture given a natural language query, which provides a natural and scalable interface for digital audio applications. Recent works on LASS, despite attaining promising separation performance on specific sources (e.g., musical instruments, limited classes of audio events), are unable to separate audio concepts in the open domain. In this work, we introduce AudioSep, a foundation model for open-domain audio source separation with natural language queries. We train AudioSep on large-scale multimodal datasets and extensively evaluate its capabilities on numerous tasks including audio event separation, musical instrument separation, and speech enhancement. AudioSep demonstrates strong separation performance and impressive zero-shot generalization ability using audio captions or text labels as queries, substantially outperforming previous audio-queried and language-queried sound separation models. Specifically, AudioSep achieved strong results including a Signal-to-Distortion Ratio Improvement (SDRi) of 7.74 dB across 527 sound classes of the AudioSet; 9.14 dB on the VGGSound dataset; 8.22 dB on the AudioCaps dataset; 6.85 dB on the Clotho dataset; 10.51 dB on the MUSIC dataset; 10.04 dB on the ESC-50 dataset; 8.16 dB on the DCASE 2024 Task 9 dataset; and an SSNR of 9.21 dB on the VoicebankDemand dataset.
Contrastive language-audio pretraining (CLAP) has become a new paradigm to learn audio concepts with audio-text pairs. CLAP models have shown unprecedented performance as zero-shot classifiers on downstream tasks. To further adapt CLAP with domain-specific knowledge, a popular method is to fine-tune its audio encoder with available labelled examples. However, this is challenging in low-shot scenarios, as the amount of annotations is limited compared to the model size. In this work, we introduce a Training-efficient (Treff) adapter to rapidly learn with a small set of examples while maintaining the capacity for zero-shot classification. First, we propose a crossattention linear model (CALM) to map a set of labelled examples and test audio to test labels. Second, we find initialising CALM as a cosine measurement improves our Treff adapter even without training. The Treff adapter outperforms metricbased methods in few-shot settings and yields competitive results to fully-supervised methods.
This study defines a new evaluation metric for audio tagging tasks to alleviate the limitation of the mean average precision (mAP) metric. The mAP metric treats different kinds of sound as independent classes without considering their relations. The proposed metric, ontology-aware mean average precision (OmAP), addresses the weaknesses of mAP by utilizing additional on-tology during evaluation. Specifically, we reweight the false positive events in the model prediction based on the AudioSet ontology graph distance to the target classes. The OmAP also provides insights into model performance by evaluating different coarse-grained levels in the ontology graph. We conduct a human assessment and show that OmAP is more consistent with human perception than mAP. We also propose an ontology-based loss function (OBCE) that reweights binary cross entropy (BCE) loss based on the ontology distance. Our experiment shows that OBCE can improve both mAP and OmAP metrics on the AudioSet tagging task.
Sounds carry an abundance of information about activities and events in our everyday environment, such as traffic noise, road works, music, or people talking. Recent machine learning methods, such as convolutional neural networks (CNNs), have been shown to be able to automatically recognize sound activities, a task known as audio tagging. One such method, pre-trained audio neural networks (PANNs), provides a neural network which has been pre-trained on over 500 sound classes from the publicly available AudioSet dataset, and can be used as a baseline or starting point for other tasks. However, the existing PANNs model has a high computational complexity and large storage requirement. This could limit the potential for deploying PANNs on resource-constrained devices, such as on-the-edge sound sensors, and could lead to high energy consumption if many such devices were deployed. In this paper, we reduce the computational complexity and memory requirement of the PANNs model by taking a pruning approach to eliminate redundant parameters from the PANNs model. The resulting Efficient PANNs (E-PANNs) model, which requires 36% less computations and 70% less memory, also slightly improves the sound recognition (audio tagging) performance. The code for the E-PANNs model has been released under an open source license.
Language-queried audio source separation (LASS) focuses on separating sounds using textual descriptions of the desired sources. Current methods mainly use discriminative approaches, such as time-frequency masking, to separate target sounds and minimize interference from other sources. However, these models face challenges when separating overlapping sound-tracks, which may lead to artifacts such as spectral holes or incomplete separation. Rectified flow matching (RFM), a generative model that establishes linear relations between the distribution of data and noise, offers superior theoretical properties and simplicity, but has not yet been explored in sound separation. In this work, we introduce FlowSep, a new generative model based on RFM for LASS tasks. FlowSep learns linear flow trajectories from noise to target source features within the variational autoencoder (VAE) latent space. During inference, the RFM-generated latent features are reconstructed into a mel-spectrogram via the pre-trained VAE decoder, followed by a pre-trained vocoder to synthesize the waveform. Trained on 1, 680 hours of audio data, FlowSep outperforms the state-of-the-art models across multiple benchmarks, as evaluated with subjective and objective metrics. Additionally, our results show that FlowSep surpasses a diffusion-based LASS model in both separation quality and inference efficiency, highlighting its strong potential for audio source separation tasks. Code, pre-trained models and demos can be found at: https://audio-agi.github.io/FlowSep_demo/.
Large language models (LLMs) have significantly advanced audio processing through audio codecs that convert audio into discrete tokens, enabling the application of language modelling techniques to audio data. However, traditional codecs often operate at high bitrates or within narrow domains such as speech and lack the semantic clues required for efficient language modelling. Addressing these challenges, we introduce SemantiCodec, a novel codec designed to compress audio into fewer than a hundred tokens per second across diverse audio types, including speech, general sound, and music, without compromising quality. SemantiCodec features a dual-encoder architecture: a semantic encoder using a self-supervised pre-trained Audio Masked Autoencoder (AudioMAE), discretized using k-means clustering on extensive audio data, and an acoustic encoder to capture the remaining details. The semantic and acoustic encoder outputs are used to reconstruct audio via a diffusion-model-based decoder. SemantiCodec is presented in three variants with token rates of 25, 50, and 100 per second, supporting a range of ultra-low bit rates between 0.31 kbps and 1.40 kbps. Experimental results demonstrate that SemantiCodec significantly outperforms the state-of-the-art Descript codec on reconstruction quality. Our results also suggest that SemantiCodec contains significantly richer semantic information than all evaluated state-of-the-art audio codecs, even at significantly lower bitrates. Our code and demos are available at https://haoheliu.github.io/SemantiCodec/ .
Although audio generation shares commonalities across different types of audio, such as speech, music, and sound effects, designing models for each type requires careful consideration of specific objectives and biases that can significantly differ from those of other types. To bring us closer to a unified perspective of audio generation, this paper proposes a holistic framework that utilizes the same learning method for speech, music, and sound effect generation. Our framework utilizes a general representation of audio, called “language of audio” (LOA). Any audio can be translated into LOA based on AudioMAE, a self-supervised pre-trained representation learning model. In the generation process, we translate other modalities into LOA by using a GPT-2 model, and we perform self-supervised audio generation learning with a latent diffusion model conditioned on the LOA of audio in our training set. The proposed framework naturally brings advantages such as reusable self-supervised pretrained latent diffusion models. Experiments on the major benchmarks of text-to-audio, text-to-music, and text-to-speech with three AudioLDM 2 variants demonstrate competitive performance of the AudioLDM 2 framework against previous approaches.
Fish feeding intensity assessment (FFIA) aims to evaluate fish appetite changes during feeding, which is crucial in industrial aquaculture applications. Existing FFIA methods are limited by their robustness to noise, computational complexity, and the lack of public datasets for developing the models. To address these issues, we first introduce AV-FFIA, a new dataset containing 27,000 labeled audio and video clips that capture different levels of fish feeding intensity. Then, we introduce multi-modal approaches for FFIA by leveraging the models pre-trained on individual modalities and fused with data fusion methods. We perform benchmark studies of these methods on AV-FFIA, and demonstrate the advantages of the multi-modal approach over the single-modality based approach, especially in noisy environments. However, compared to the methods developed for individual modalities, the multimodal approaches may involve higher computational costs due to the need for independent encoders for each modality. To overcome this issue, we further present a novel unified mixed-modality based method for FFIA, termed as U-FFIA. U-FFIA is a single model capable of processing audio, visual, or audio-visual modalities, by leveraging modality dropout during training and knowledge distillation using the models pre-trained with data from single modality. We demonstrate that U-FFIA can achieve performance better than or on par with the state-of-the-art modality-specific FFIA models, with significantly lower computational overhead, enabling robust and efficient FFIA for improved aquaculture management. To encourage further research, we have released the AV-FFIA dataset, the pre-trained model and codes at https://github.com/FishMaster93/U-FFIA. Note to Practitioners-Feeding is one of the most important costs in aquaculture. However, current feeding machines usually operate with fixed thresholds or human experiences, lacking the ability to automatically adjust to fish feeding intensity. FFIA can evaluate the intensity changes in fish appetite during the feeding process and optimize the control strategies of the feeding machine to avoid inadequate feeding or overfeeding, thereby reducing the feeding cost and improving the well-being of fish in industrial aquaculture. The existing methods have mainly exploited single-modality data, and have a high sensitivity to input noise. Using video and audio offers improved chances to address the challenges brought by various environments. However, compared with processing data from single modalities, using multiple modalities simultaneously often involves increased computational resources, including memory, processing power, and storage. This can impact system performance and scalability. To address these issues, we focus on the efficient unified model, which is capable of processing both multimodal and single-modal input. Our proposed model achieved state-of-the-art (SOTA) performance in FFIA with high computational efficiency.
Digital aquaculture leverages advanced technologies and data‐driven methods, providing substantial benefits over traditional aquaculture practices. This article presents a comprehensive review of three interconnected digital aquaculture tasks, namely, fish tracking, counting, and behaviour analysis, using a novel and unified approach. Unlike previous reviews which focused on single modalities or individual tasks, we analyse vision‐based (i.e., image‐ and video‐based), acoustic‐based, and biosensor‐based methods across all three tasks. We examine their advantages, limitations, and applications, highlighting recent advancements and identifying critical cross‐cutting research gaps. The review also includes emerging ideas such as applying multitask learning and large language models to address various aspects of fish monitoring, an approach not previously explored in aquaculture literature. We identify the major obstacles hindering research progress in this field, including the scarcity of comprehensive fish datasets and the lack of unified evaluation standards. To overcome the current limitations, we explore the potential of using emerging technologies such as multimodal data fusion and deep learning to improve the accuracy, robustness, and efficiency of integrated fish monitoring systems. In addition, we provide a summary of existing datasets available for fish tracking, counting, and behaviour analysis. This holistic perspective offers a roadmap for future research, emphasizing the need for comprehensive datasets and evaluation standards to facilitate meaningful comparisons between technologies and to promote their practical implementations in real‐world settings.
Language-queried audio source separation (LASS) is a new paradigm for computational auditory scene analysis (CASA). LASS aims to separate a target sound from an audio mixture given a natural language query, which provides a natural and scalable interface for digital audio applications. Recent works on LASS, despite attaining promising separation performance on specific sources (e.g., musical instruments, limited classes of audio events), are unable to separate audio concepts in the open domain. In this work, we introduce AudioSep, a foundation model for open-domain audio source separation with natural language queries. We train AudioSep on large-scale multimodal datasets and extensively evaluate its capabilities on numerous tasks including audio event separation, musical instrument separation, and speech enhancement. AudioSep demonstrates strong separation performance and impressive zero-shot generalization ability using audio captions or text labels as queries, substantially outperforming previous audio-queried and language-queried sound separation models. For reproducibility of this work, we will release the source code, evaluation benchmark and pre-trained model at: https://github.com/Audio-AGI/AudioSep.
The advancement of audio-language (AL) multi-modal learning tasks has been significant in recent years, yet the limited size of existing audio-language datasets poses challenges for researchers due to the costly and time-consuming collection process. To address this data scarcity issue, we introduce WavCaps, the first large-scale weakly-labelled audio captioning dataset, comprising approximately 400k audio clips with paired captions. We sourced audio clips and their raw descriptions from web sources and a sound event detection dataset. However, the online-harvested raw descriptions are highly noisy and unsuitable for direct use in tasks such as automated audio captioning. To overcome this issue, we propose a three-stage processing pipeline for filtering noisy data and generating high-quality captions, where ChatGPT, a large language model, is leveraged to filter and transform raw descriptions automatically. We conduct a comprehensive analysis of the characteristics of WavCaps dataset and evaluate it on multiple downstream audio-language multimodal learning tasks. The systems trained on WavCaps outperform previous state-of-the-art (SOTA) models by a significant margin. Our aspiration is for the WavCaps dataset we have proposed to facilitate research in audio-language multimodal learning and demonstrate the potential of utilizing large language models (LLMs) to enhance academic research. Our dataset and codes are available at https://github.com/XinhaoMei/WavCaps.
Audio and visual signals can be used jointly to provide complementary information for multi-speaker tracking. Face detectors and color histogram can provide visual measurements while Direction of Arrival (DOA) lines and global coherence field (GCF) maps can provide audio measurements. GCF, as a traditional sound source localization method, has been widely used to provide audio measurements in audio-visual speaker tracking by estimating the positions of speakers. However, GCF cannot directly deal with the scenarios of multiple speakers due to the emergence of spurious peaks on the GCF map, making it difficult to find the non-dominant speakers. To overcome this limitation, we propose a phase-aware VoiceFilter and a separation-before-localization method, which enables the audio mixture to be separated into individual speech sources while retaining their phases. This allows us to calculate the GCF map for multiple speakers, thereby their positions accurately and concurrently. Based on this method, we design an adaptive audio measurement likelihood for audio-visual multiple speaker tracking using Poisson multi-Bernoulli mixture (PMBM) filter. The experiments demonstrate that our proposed tracker achieves state-of-the-art results on the AV16.3 dataset.
Data-driven approaches hold promise for audio captioning. However, the development of audio captioning methods can be biased due to the limited availability and quality of text-audio data. This paper proposes a SynthAC framework, which leverages recent advances in audio generative models and commonly available text corpus to create synthetic text-audio pairs, thereby enhancing text-audio representation. Specifically, the text-to-audio generation model, i.e., AudioLDM, is used to generate synthetic audio signals with captions from an image captioning dataset. Our SynthAC expands the availability of well-annotated captions from the text-vision domain to audio captioning, thus enhancing text-audio representation by learning relations within synthetic text-audio pairs. Experiments demonstrate that our SynthAC framework can benefit audio captioning models by incorporating well-annotated text corpus from the text-vision domain, offering a promising solution to the challenge caused by data scarcity. Furthermore, SynthAC can be easily adapted to various state-of-the-art methods, leading to substantial performance improvements.
Foley sound generation aims to synthesise the background sound for multimedia content. Previous models usually employ a large development set with labels as input (e.g., single numbers or one-hot vector). In this work, we propose a diffusion model based system for Foley sound generation with text conditions. To alleviate the data scarcity issue, our model is initially pre-trained with large-scale datasets and fine-tuned to this task via transfer learning using the contrastive language-audio pertaining (CLAP) technique. We have observed that the feature embedding extracted by the text encoder can significantly affect the performance of the generation model. Hence, we introduce a trainable layer after the encoder to improve the text embedding produced by the encoder. In addition, we further refine the generated waveform by generating multiple candidate audio clips simultaneously and selecting the best one, which is determined in terms of the similarity score between the embedding of the candidate clips and the embedding of the target text label. Using the proposed method, our system ranks \({1}^{st}\) among the systems submitted to DCASE Challenge 2023 Task 7. The results of the ablation studies illustrate that the proposed techniques significantly improve sound generation performance. The codes for implementing the proposed system are available online.
Foley sound presents the background sound for multimedia content and the generation of Foley sound involves computationally modelling sound effects with specialized techniques. In this work, we proposed a system for DCASE 2023 challenge task 7: Foley Sound Synthesis. The proposed system is based on AudioLDM, which is a diffusion-based text-to-audio generation model. To alleviate the data-hungry problem, the system first trained with large-scale datasets and then downstreamed into this DCASE task via transfer learning. Through experiments, we found out that the feature extracted by the encoder can significantly affect the performance of the generation model. Hence, we improve the results by leveraging the input label with related text embedding features obtained by a significant language model, i.e., contrastive language-audio pertaining (CLAP). In addition, we utilize a filtering strategy to further refine the output, i.e. by selecting the best results from the candidate clips generated in terms of the similarity score between the sound and target labels. The overall system achieves a Frechet audio distance (FAD) score of 4.765 on average among all seven different classes, substantially outperforming the baseline system which performs a FAD score of 9.7.
Sounds carry an abundance of information about activities and events in our everyday environment, such as traffic noise, road works, music, or people talking. Recent machine learning methods, such as convolutional neural networks (CNNs), have been shown to be able to automatically recognize sound activities, a task known as audio tagging. One such method, pre-trained audio neural networks (PANNs), provides a neural network which has been pre-trained on over 500 sound classes from the publicly available AudioSet dataset, and can be used as a baseline or starting point for other tasks. However, the existing PANNs model has a high computational complexity and large storage requirement. This could limit the potential for deploying PANNs on resource-constrained devices, such as on-the-edge sound sensors, and could lead to high energy consumption if many such devices were deployed. In this paper, we reduce the computational complexity and memory requirement of the PANNs model by taking a pruning approach to eliminate redundant parameters from the PANNs model. The resulting Efficient PANNs (E-PANNs) model, which requires 36% less computations and 70% less memory, also slightly improves the sound recognition (audio tagging) performance. The code for the E-PANNs model has been released under an open source license.
Despite recent progress in text-to-audio (TTA) generation, we show that the state-of-the-art models, such as AudioLDM, trained on datasets with an imbalanced class distribution, such as AudioCaps, are biased in their generation performance. Specifically, they excel in generating common audio classes while underperforming in the rare ones, thus degrading the overall generation performance. We refer to this problem as long-tailed text-to-audio generation. To address this issue, we propose a simple retrieval-augmented approach for TTA models. Specifically, given an input text prompt, we first leverage a Contrastive Language Audio Pretraining (CLAP) model to retrieve relevant text-audio pairs. The features of the retrieved audio-text data are then used as additional conditions to guide the learning of TTA models. We enhance AudioLDM with our proposed approach and denote the resulting augmented system as Re-AudioLDM. On the AudioCaps dataset, Re-AudioLDM achieves a state-of-the-art Frechet Audio Distance (FAD) of 1.37, outperforming the existing approaches by a large margin. Furthermore, we show that Re-AudioLDM can generate realistic audio for complex scenes, rare audio classes, and even unseen audio types, indicating its potential in TTA tasks.
Text-to-audio (TTA) system has recently gained attention for its ability to synthesize general audio based on text descriptions. However, previous studies in TTA have limited generation quality with high computational costs. In this study, we propose AudioLDM, a TTA system that is built on a latent space to learn the continuous audio representations from contrastive language-audio pretraining (CLAP) latents. The pretrained CLAP models enable us to train LDMs with audio embedding while providing text embedding as a condition during sampling. By learning the latent representations of audio signals and their compositions without modeling the cross-modal relationship, AudioLDM is advantageous in both generation quality and computational efficiency. Trained on AudioCaps with a single GPU, AudioLDM achieves state-of-the-art TTA performance measured by both objective and subjective metrics (e.g., frechet distance). Moreover, AudioLDM is the first TTA system that enables various text-guided audio manipulations (e.g., style transfer) in a zero-shot fashion. Our implementation and demos are available at https://audioldm.github.io.
In this paper, we introduce the task of language-queried audio source separation (LASS), which aims to separate a target source from an audio mixture based on a natural language query of the target source (e.g., “a man tells a joke followed by people laughing”). A unique challenge in LASS is associated with the complexity of natural language description and its relation with the audio sources. To address this issue, we proposed LASSNet, an end-to-end neural network that is learned to jointly process acoustic and linguistic information, and separate the target source that is consistent with the language query from an audio mixture. We evaluate the performance of our proposed system with a dataset created from the AudioCaps dataset. Experimental results show that LASS-Net achieves considerable improvements over baseline methods. Furthermore, we observe that LASS-Net achieves promising generalization results when using diverse human-annotated descriptions as queries, indicating its potential use in real-world scenarios. The separated audio samples and source code are available at https://liuxubo717.github.io/LASS-demopage.
The audio spectrogram is a time-frequency representation that has been widely used for audio classification. One of the key attributes of the audio spectrogram is the temporal resolution, which depends on the hop size used in the Short-Time Fourier Transform (STFT). Previous works generally assume the hop size should be a constant value (e.g., 10 ms). However, a fixed temporal resolution is not always optimal for different types of sound. The temporal resolution affects not only classification accuracy but also computational cost. This paper proposes a novel method, DiffRes, that enables differentiable temporal resolution modeling for audio classification. Given a spectrogram calculated with a fixed hop size, DiffRes merges non-essential time frames while preserving important frames. DiffRes acts as a "drop-in" module between an audio spectrogram and a classifier and can be jointly optimized with the classification task. We evaluate DiffRes on five audio classification tasks, using mel-spectrograms as the acoustic features, followed by off-the-shelf classifier backbones. Compared with previous methods using the fixed temporal resolution, the DiffRes-based method can achieve the equivalent or better classification accuracy with at least 25% computational cost reduction. We further show that DiffRes can improve classification accuracy by increasing the temporal resolution of input acoustic features, without adding to the computational cost.
Large Language Models (LLMs) have shown great promise in integrating diverse expert models to tackle intricate language and vision tasks. Despite their significance in advancing the field of Artificial Intelligence Generated Content (AIGC), their potential in intelligent audio content creation remains unexplored. In this work, we tackle the problem of creating audio content with storylines encompassing speech, music, and sound effects, guided by text instructions. We present WavJourney, a system that leverages LLMs to connect various audio models for audio content generation. Given a text description of an auditory scene, WavJourney first prompts LLMs to generate a structured script dedicated to audio storytelling. The audio script incorporates diverse audio elements, organized based on their spatio-temporal relationships. As a conceptual representation of audio, the audio script provides an interactive and interpretable rationale for human engagement. Afterward, the audio script is fed into a script compiler, converting it into a computer program. Each line of the program calls a task-specific audio generation model or computational operation function (e.g., concatenate, mix). The computer program is then executed to obtain an explainable solution for audio generation. We demonstrate the practicality of WavJourney across diverse real-world scenarios, including science fiction, education, and radio play. The explainable and interactive design of WavJourney fosters human-machine co-creation in multi-round dialogues, enhancing creative control and adaptability in audio production. WavJourney audiolizes the human imagination, opening up new avenues for creativity in multimedia content creation.
Contrastive language-audio pretraining (CLAP) has been developed to align the representations of audio and language, achieving remarkable performance in retrieval and classification tasks. However, current CLAP struggles to capture temporal information within audio and text features, presenting substantial limitations for tasks such as audio retrieval and generation. To address this gap, we introduce T-CLAP, a temporal-enhanced CLAP model. We use Large Language Models (LLMs) and mixed-up strategies to generate temporal-contrastive captions for audio clips from extensive audio-text datasets. Subsequently, a new temporal-focused contrastive loss is designed to fine-tune the CLAP model by incorporating these synthetic data. We conduct comprehensive experiments and analysis in multiple downstream tasks. T-CLAP shows improved capability in capturing the temporal relationship of sound events and outperforms state-of-the-art models by a significant margin. Our code and models will be released soon.
Audio captioning aims to generate text descriptions of audio clips. In the real world, many objects produce similar sounds. How to accurately recognize ambiguous sounds is a major challenge for audio captioning. In this work, inspired by inherent human multimodal perception, we propose visually-aware audio captioning, which makes use of visual information to help the description of ambiguous sounding objects. Specifically , we introduce an off-the-shelf visual encoder to extract video features and incorporate the visual features into an audio captioning system. Furthermore, to better exploit complementary audiovisual contexts, we propose an audiovisual attention mechanism that adaptively integrates audio and visual context and removes the redundant information in the latent space. Experimental results on AudioCaps, the largest audio captioning dataset, show that our proposed method achieves state-of-the-art results on machine translation metrics.
Deep neural networks have recently achieved break-throughs in sound generation. Despite the outstanding sample quality, current sound generation models face issues on small-scale datasets (e.g., overfitting), significantly limiting performance. In this paper, we make the first attempt to investigate the benefits of pre-training on sound generation with AudioLDM, the cutting-edge model for audio generation, as the backbone. Our study demonstrates the advantages of the pre-trained AudioLDM, especially in data-scarcity scenarios. In addition, the baselines and evaluation protocol for sound generation systems are not consistent enough to compare different studies directly. Aiming to facilitate further study on sound generation tasks, we benchmark the sound generation task on various frequently-used datasets. We hope our results on transfer learning and benchmarks can provide references for further research on conditional sound generation.
Audio super-resolution is a fundamental task that predicts high-frequency components for low-resolution audio, enhancing audio quality in digital applications. Previous methods have limitations such as the limited scope of audio types (e.g., music, speech) and specific bandwidth settings they can handle (e.g., 4kHz to 8kHz). In this paper, we introduce a diffusion-based generative model, AudioSR, that is capable of performing robust audio super-resolution on versatile audio types, including sound effects, music, and speech. Specifically, AudioSR can upsample any input audio signal within the bandwidth range of 2kHz to 16kHz to a high-resolution audio signal at 24kHz bandwidth with a sampling rate of 48kHz. Extensive objective evaluation on various audio super-resolution benchmarks demonstrates the strong result achieved by the proposed model. In addition, our subjective evaluation shows that AudioSR can acts as a plug-and-play module to enhance the generation quality of a wide range of audio generative models, including AudioLDM, Fastspeech2, and MusicGen. Our code and demo are available at https://audioldm.github.io/audiosr.
Although audio generation shares commonalities across different types of audio, such as speech, music, and sound effects, designing models for each type requires careful consideration of specific objectives and biases that can significantly differ from those of other types. To bring us closer to a unified perspective of audio generation, this paper proposes a framework that utilizes the same learning method for speech, music, and sound effect generation. Our framework introduces a general representation of audio, called "language of audio" (LOA). Any audio can be translated into LOA based on AudioMAE, a self-supervised pre-trained representation learning model. In the generation process, we translate any modalities into LOA by using a GPT-2 model, and we perform self-supervised audio generation learning with a latent diffusion model conditioned on LOA. The proposed framework naturally brings advantages such as in-context learning abilities and reusable self-supervised pretrained AudioMAE and latent diffusion models. Experiments on the major benchmarks of text-to-audio, text-to-music, and text-to-speech demonstrate state-of-the-art or competitive performance against previous approaches. Our code, pretrained model, and demo are available at https://audioldm.github.io/audioldm2.
The choice of data augmentation is pivotal in contrastive self-supervised learning. Current augmentation techniques for audio data, such as the widely used Random Resize Crop (RRC), underperform in pitch-sensitive music tasks and lack generalisation across various types of audio. This study aims to address these limitations by introducing Neural Compression Augmentation (NCA), an approach based on lossy neural compression. We use the Audio Barlow Twins (ABT), a contrastive self-supervised framework for audio, as our backbone. We experiment with both NCA and several baseline augmentation methods in the augmentation block of ABT and train the models on AudioSet. Experimental results show that models integrated with NCA considerably surpass the original performance of ABT, especially in the music tasks of the HEAR benchmark, demonstrating the effectiveness of compression-based augmentation for audio contrastive self-supervised learning.
First-shot (FS) unsupervised anomalous sound detection (ASD) is a brand-new task introduced in DCASE 2023 Challenge Task 2, where the anomalous sounds for the target machine types are unseen in training. Existing methods often rely on the availability of normal and abnormal sound data from the target machines. However, due to the lack of anomalous sound data for the target machine types, it becomes challenging when adapting the existing ASD methods to the first-shot task. In this paper, we propose a new framework for the first-shot unsupervised ASD, where metadata-assisted audio generation is used to estimate unknown anomalies, by utilising the available machine information (i.e., metadata and sound data) to fine-tune a text-to-audio generation model for generating the anomalous sounds that contain unique acoustic characteristics accounting for each different machine types. We then use the method of Time-Weighted Frequency domain audio Representation with Gaussian Mixture Model (TWFR-GMM) as the backbone to achieve the first-shot unsupervised ASD. Our proposed FS-TWFR-GMM method achieves competitive performance amongst top systems in DCASE 2023 Challenge Task 2, while requiring only 1% model parameters for detection, as validated in our experiments.
Significant improvement has been achieved in automated audio captioning (AAC) with recent models. However, these models have become increasingly large as their performance is enhanced. In this work, we propose a knowledge distillation (KD) framework for AAC. Our analysis shows that in the encoder-decoder based AAC models, it is more effective to dis-till knowledge into the encoder as compared with the decoder. To this end, we incorporate encoder-level KD loss into training, in addition to the standard supervised loss and sequence-level KD loss. We investigate two encoder-level KD methods, based on mean squared error (MSE) loss and contrastive loss, respectively. Experimental results demonstrate that contrastive KD is more robust than MSE KD, exhibiting superior performance in data-scarce situations. By leveraging audio-only data into training in the KD framework, our student model achieves competitive performance, with an inference speed that is 19 times faster.
Universal source separation (USS) is a fundamental research task for computational auditory scene analysis, which aims to separate mono recordings into individual source tracks. There are three potential challenges awaiting the solution to the audio source separation task. First, previous audio source separation systems mainly focus on separating one or a limited number of specific sources. There is a lack of research on building a unified system that can separate arbitrary sources via a single model. Second, most previous systems require clean source data to train a separator, while clean source data are scarce. Third, there is a lack of USS system that can automatically detect and separate active sound classes in a hierarchical level. To use large-scale weakly labeled/unlabeled audio data for audio source separation, we propose a universal audio source separation framework containing: 1) an audio tagging model trained on weakly labeled data as a query net; and 2) a conditional source separation model that takes query net outputs as conditions to separate arbitrary sound sources. We investigate various query nets, source separation models, and training strategies and propose a hierarchical USS strategy to automatically detect and separate sound classes from the AudioSet ontology. By solely leveraging the weakly labelled AudioSet, our USS system is successful in separating a wide variety of sound classes, including sound event separation, music source separation, and speech enhancement. The USS system achieves an average signal-to-distortion ratio improvement (SDRi) of 5.57 dB over 527 sound classes of AudioSet; 10.57 dB on the DCASE 2018 Task 2 dataset; 8.12 dB on the MUSDB18 dataset; an SDRi of 7.28 dB on the Slakh2100 dataset; and an SSNR of 9.00 dB on the voicebank-demand dataset. We release the source code at https://github.com/bytedance/uss
Recently, there has been increasing interest in building efficient audio neural networks for on-device scenarios. Most existing approaches are designed to reduce the size of audio neural networks using methods such as model pruning. In this work, we show that instead of reducing model size using complex methods, eliminating the temporal redundancy in the input audio features (e.g., mel-spectrogram) could be an effective approach for efficient audio classification. To do so, we proposed a family of simple pooling front-ends (SimPFs) which use simple non-parametric pooling operations to reduce the redundant information within the mel-spectrogram. We perform extensive experiments on four audio classification tasks to evaluate the performance of SimPFs. Experimental results show that SimPFs can achieve a reduction in more than half of the number of floating point operations (FLOPs) for off-the-shelf audio neural networks, with negligible degradation or even some improvements in audio classification performance.
Fish feeding intensity assessment (FFIA) aims to evaluate the intensity change of fish appetite during the feeding process, which is vital in industrial aquaculture applications. The main challenges surrounding FFIA are two-fold. 1) robustness: existing work has mainly leveraged single-modality (e.g., vision, audio) methods, which have a high sensitivity to input noise. 2) efficiency: FFIA models are generally expected to be employed on devices. This presents a challenge in terms of computational efficiency. In this work, we first introduce an audio-visual dataset, called AV-FFIA. AV-FFIA consists of 27,000 labeled audio and video clips that capture different levels of fish feeding intensity. To our knowledge, AV-FFIA is the first large-scale multimodal dataset for FFIA research. Then, we introduce a multi-modal approach for FFIA by leveraging single-modality pre-trained models and modality-fusion methods, with benchmark studies on AV-FFIA. Our experimental results indicate that the multi-modal approach substantially outperforms the single-modality based approach, especially in noisy environments. While multimodal approaches provide a performance gain for FFIA, it inherently increase the computational cost. To overcome this issue, we further present a novel unified model, termed as U-FFIA. U-FFIA is a single model capable of processing audio, visual, or audio-visual modalities, by leveraging modality dropout during training and knowledge distillation from single-modality pre-trained models. We demonstrate that U-FFIA can achieve performance better than or on par with the state-of-the-art modality-specific FFIA models, with significantly lower computational overhead. Our proposed U-FFIA approach enables a more robust and efficient method for FFIA, with the potential to contribute to improved management practices and sustainability in aquaculture.
Particle filters (PFs) have been widely used in speaker tracking due to their capability in modeling a non-linear process or a non-Gaussian environment. However, particle filters are limited by several issues. For example, pre-defined handcrafted measurements are often used which can limit the model performance. In addition, the transition and update models are often preset which make PF less flexible to be adapted to different scenarios. To address these issues, we propose an end-to-end differentiable particle filter framework by employing the multi-head attention to model the long-range dependencies. The proposed model employs the self-attention as the learned transition model and the cross-attention as the learned update model. To our knowledge, this is the first proposal of combining particle filter and transformer for speaker tracking, where the measurement extraction, transition and update steps are integrated into an end-to-end architecture. Experimental results show that the proposed model achieves superior performance over the recurrent baseline models.
Automatic detection and classification of animal sounds has many applications in biodiversity monitoring and animal behavior. In the past twenty years, the volume of digitised wildlife sound available has massively increased, and automatic classification through deep learning now shows strong results. However, bioacoustics is not a single task but a vast range of small-scale tasks (such as individual ID, call type, emotional indication) with wide variety in data characteristics, and most bioacoustic tasks do not come with strongly-labelled training data. The standard paradigm of supervised learning, focussed on a single large-scale dataset and/or a generic pretrained algorithm, is insufficient. In this work we recast bioacoustic sound event detection within the AI framework of few-shot learning. We adapt this framework to sound event detection, such that a system can be given the annotated start/end times of as few as 5 events, and can then detect events in long-duration audio-even when the sound category was not known at the time of algorithm training. We introduce a collection of open datasets designed to strongly test a system's ability to perform few-shot sound event detections, and we present the results of a public contest to address the task. Our analysis shows that prototypical networks are a very common used strategy and they perform well when enhanced with adaptations for general characteristics of animal sounds. However, systems with high time resolution capabilities perform the best in this challenge. We demonstrate that widely-varying sound event durations are an important factor in performance, as well as nonstationarity, i.e. gradual changes in conditions throughout the duration of a recording. For fine-grained bioacoustic recognition tasks without massive annotated training data, our analysis demonstrate that few-shot sound event detection is a powerful new method, strongly outperforming traditional signal-processing detection methods in the fully automated scenario.
Automatic detection and classification of animal sounds has many applications in biodiversity monitoring and animal behavior. In the past twenty years, the volume of digitised wildlife sound available has massively increased, and automatic classification through deep learning now shows strong results. However, bioacoustics is not a single task but a vast range of small-scale tasks (such as individual ID, call type, emotional indication) with wide variety in data characteristics, and most bioacoustic tasks do not come with strongly-labelled training data. The standard paradigm of supervised learning, focussed on a single large-scale dataset and/or a generic pretrained algorithm, is insufficient. In this work we recast bioacoustic sound event detection within the AI framework of few-shot learning. We adapt this framework to sound event detection, such that a system can be given the annotated start/end times of as few as 5 events, and can then detect events in long-duration audio-even when the sound category was not known at the time of algorithm training. We introduce a collection of open datasets designed to strongly test a system's ability to perform few-shot sound event detections, and we present the results of a public contest to address the task. Our analysis shows that prototypical networks are a very common used strategy and they perform well when enhanced with adaptations for general characteristics of animal sounds. However, systems with high time resolution capabilities perform the best in this challenge. We demonstrate that widely-varying sound event durations are an important factor in performance, as well as nonstationarity, i.e. gradual changes in conditions throughout the duration of a recording. For fine-grained bioacoustic recognition tasks without massive annotated training data, our analysis demonstrate that few-shot sound event detection is a powerful new method, strongly outperforming traditional signal-processing detection methods in the fully automated scenario.
Additional publications
Preprint
- Haohe Liu, Zehua Chen, Yi Yuan, Xinhao Mei, Xubo Liu, Danilo Mandic, Wenwu Wang, and Mark D. Plumbley. AudioLDM: Text-to-Audio Generation with Latent Diffusion Models, arXiv preprint 2023.
- Haohe Liu, Xubo Liu, Qiuqiang Kong, Wenwu Wang, and Mark D. Plumbley. Learning the Spectrogram Temporal Resolution for Audio Classification, arXiv preprint 2022.
- Zehua Chen, Yihan Wu, Yichong Leng, Jiawei Chen, Haohe Liu, Xu Tan, Yang Cui, Ke Wang, Lei He, Sheng zhao, Jiang Bian, Danilo Mandic, ResGrad: Residual Denoising Diffusion Probabilistic Models for Text to Speech, arXiv preprint 2022.
- Xu Tan*, Jiawei Chen*, Haohe Liu*, Jian Cong, Chen Zhang, Yanqing Liu, Xi Wang, Yichong Leng, Yuanhao Yi, Lei He, Frank Soong, Tao Qin, Sheng Zhao, Tie-Yan Liu, NaturalSpeech: End-to-End Text to Speech Synthesis with Human-Level Quality, arxiv preprint 2022. [pdf][demo]
- Haohe Liu, Qiuqiang Kong, Qiao Tian, Yan Zhao, DeLiang Wang, Chuanzeng Huang, Yuxuan Wang, VoiceFixer: Toward General Speech Restoration with Neural Vocoder, arXiv preprint 2021. [pdf][code][demo]
Conference paper
- Haohe Liu, Qiuqiang Kong, Xubo Liu, Xinhao Mei, Wenwu Wang, Mark D. Plumbley, Ontology-aware learning and evaluation for audio tagging, IEEE International Conference on Acoustics, Speech and Signal Processing (ICASSP), 2023. [pdf] (Under Review)
- Xubo Liu, Haohe Liu, Qiuqiang Kong, Xinhao Mei, Mark D. Plumbley, Wenwu Wang, Simple Pooling Front-ends For Efficient Audio Classification, IEEE International Conference on Acoustics, Speech and Signal Processing (ICASSP), 2023. [pdf] (Under Review)
- Xubo Liu*, Qiushi Huang*, Xinhao Mei*, Haohe Liu, Qiuqiang Kong, Jianyuan Sun, Ko Tom, Yu Zhang, Lilian H. Tang, Mark D. Plumbley, Volkan Kılıc4, Wenwu Wang Visually-awared Audio Captioning with Adaptive Audio-Visual Attention, IEEE International Conference on Acoustics, Speech and Signal Processing (ICASSP), 2023 (Under Review)
- Haohe Liu, Xubo Liu, Xinhao Mei, Qiuqiang Kong, Wenwu Wang, Mark D. Plumbley, Segment-level Metric Learning for Few-shot Bioacoustic Event Detection, DCASE Workshop 2022
- Yichong Leng, Zehua Chen, Junliang Guo, Haohe Liu, Jiawei Chen, Xu Tan, Danilo Mandic, Lei He, Xiang-Yang Li, Tao Qin, Sheng Zhao, Tie-Yan Liu, BinauralGrad: A Two-Stage Conditional Diffusion Probabilistic Model for Binaural Audio Synthesis, Conference on Neural Information Processing Systems (NeurIPS) 2022. [pdf][demo]
- Haohe Liu, Xubo Liu, Qiuqiang Kong, Qiao Tian, Yan Zhao, Deliang Wang, Chuanzeng Huang, Yuxuan Wang, VoiceFixer: A Unified Framework for High-Fidelity Speech Restoration, in Proceedings of INTERSPEECH 2022. [pdf][code][demo]
- Haohe Liu, Woosung Choi, Xubo Liu, Qiuqiang Kong, Qiao Tian, DeLiang Wang, Neural Vocoder is All You Need for Speech Super-resolution, in Proceedings of INTERSPEECH 2022. [pdf][code][demo]
- Jinzheng Zhao, Peipei Wu, Xubo Liu, Shidrokh Goudarzi, Haohe Liu, Yong Xu, Wenwu Wang, Multiple Speakers Tracking with Audio and Visual Signals, in Proceedings of INTERSPEECH 2022.
- Xubo Liu, Haohe Liu, Qiuqiang Kong, Xinhao Mei, Jinzheng Zhao, Qiushi Huang, Mark D. Plumbley, Wenwu Wang, Separate What You Describe: Language-Queried Audio Source Separation, in Proceedings of INTERSPEECH 2022. [pdf][code][demo]
- Xubo Liu, Xinhao Mei, Qiushi Huang, Jianyuan Sun, Jinzheng Zhao, Haohe Liu, Mark D. Plumbley, Volkan Kılıc, Wenwu Wang, Leveraging Pre-trained BERT for Audio Captioning, in Proceedings of EUSIPCO 2022. [pdf]
- Haohe Liu, Qiuqiang Kong, Jiafeng Liu, CWS-PResUNet: Music Source Separation with Channel-wise Subband Phase-aware ResUNet, ISMIR Music Demixing Workshop 2021. [pdf][code]
- Qiuqiang Kong, Yin Cao, Haohe Liu, Keunwoo Choi, Yuxuan Wang, Decoupling Magnitude and Phase Estimation with Deep ResUNet for Music Source Separation, ISMIR 2021. [pdf]
- Qiuqiang Kong, Haohe Liu, Xingjian Du, Li Chen, Rui Xia, YuxuanWang, Speech Enhancement with weakly labeled data from audioset, INTERSPEECH 2021. [pdf]
- Haohe Liu, Lei Xie, Jian Wu, Geng Yang, Channel-wise Subband Input for Better Voice and Accompaniment Separation on High Resolution Music, INTERSPEECH 2020. [pdf][code][demo]
- Haohe Liu, Siqi Yao, Yulin Wang, Design and Visualization of Guided GAN on MNIST dataset, Proceedings of the 3rd international conference on Graphics and Signal Processing 2019.
Technical report
- Haohe Liu, Xubo Liu, Xinhao Mei, Qiuqiang Kong, Wenwu Wang, Mark D. Plumbley, Surrey System for DCASE 2022 Task 5: Few-shot Bio-acoustic Event Detection with Segment-level Metric Learning, DCASE2022 Challenge Technical Report 2022. [pdf]
- Xinhao Mei, Xubo Liu, Haohe Liu, Jianyuan Sun, Mark D. Plumbley, Wenwu Wang, Automated Audio Captioning with Keywords Guidance, DCASE2022 Challenge Technical Report 2022. [pdf]
- Xinhao Mei, Xubo Liu, Haohe Liu, Jianyuan Sun, Mark D. Plumbley, Wenwu Wang, Language-Based Audio Retrieval with Pre-trained Models, DCASE2022 Challenge Technical Report 2022. [pdf]
Updated on Feb. 2023