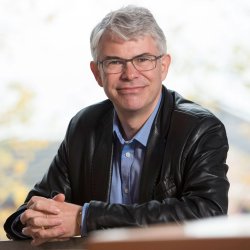
Professor Mark Plumbley FIET, FIEEE
Academic and research departments
Centre for Vision, Speech and Signal Processing (CVSSP), School of Computer Science and Electronic Engineering.About
Biography
Mark Plumbley is Professor of Signal Processing at the Centre for Vision, Speech and Signal Processing (CVSSP), School of Computer Science and Electronic Engineering, at the University of Surrey, in Guildford, UK.
After receiving his PhD degree in neural networks in 1991, he became a Lecturer at King’s College London, before moving to Queen Mary University of London in 2002. He subsequently became Professor and Director of the Centre for Digital Music, before joining the University of Surrey in 2015.
His current research concerns AI for Sound: using machine learning, AI and signal processing for analysis and recognition of sounds, particularly real-world everyday sounds. He led the first data challenge on Detection and Classification of Acoustic Scenes and Events (DCASE 2013).
He holds an EPSRC Fellowship in "AI for Sound", recently led EPSRC-funded projects on making sense of everyday sounds and on audio source separation, and two EU-funded research training networks in sparse representations and compressed sensing. He is a co-editor of the Springer book on "Computational Analysis of Sound Scenes and Events". He is a Fellow of the IET and IEEE.
Previous roles
Affiliations and memberships
News and events
2025
- 13 May 2025: Recruiting! Deadline for Research Fellow in Generative Audio AI
- 2 May 2025: Extended deadline for Call for Expressions of Interest in Noise Network Plus Working Groups [LinkedIn]
- 28-30 Apr 2025: See you at the Urban Sound Symposium 2025, Zurich, Switzerland.
- 8-11 Apr 2025: Papers presented at the IEEE International Conference on Acoustics, Speech, and Signal Processing (ICASSP 2025), Hyderabad, India:
- Apr 11: A decade of DCASE: Achievements, practices, evaluations and future challenges (Annamaria Mesaros, Romain Serizel, Toni Heittola, Tuomas Virtanen, Mark D. Plumbley) Special Sesssion: 50 years of Audio and Acoustic Signal Processing [Paper] [AAM] [Preprint]
- Apr 11: Selective-Memory Meta-Learning with Environment Representations for Sound Event Localization and Detection (Jinbo Hu, Yin Cao, Ming Wu, Qiuqiang Kong, Feiran Yang, Mark D. Plumbley, Jun Yang). SPS Journal Paper, published as: IEEE/ACM Transactions on Audio, Speech, and Language Processing 32, pp 4313-4327, 2024. [Paper] [AAM] [Preprint] [Code]
- Apr 11: Personalized live sound recognition using efficient PANNs (Arshdeep Singh, Haohe Liu, Gabriel Bibbó, Thomas Deacon, Mark D. Plumbley) Show and Tell [LinkedIn]
- Apr 10: Sound-VECaps: Improving Audio Generation With Visual Enhanced Captions (Yi Yuan, Dongya Jia, Xiaobin Zhuang, Yuanzhe Chen, Zhuo Chen, Wang Yuping, Yuxuan Wang, Xubo Liu, Xiyuan Kang, Mark D. Plumbley, Wenwu Wang) [Paper] [AAM] [Preprint] [Code] [Dataset] [Demos]
- Apr 8: FlowSep: Language-Queried Sound Separation with Rectified Flow Matching (Yi Yuan, Xubo Liu, Haohe Liu, Mark D. Plumbley, Wenwu Wang) [Paper] [AAM] [Preprint] [Code] [Demos]
- 19 Mar 2025: See you at the UKAN+ Celebration event, Institute of Physics, London
- 18 Mar 2025: Thanks for everyone who attended the Launch Meeting of Noise Network Plus, Royal Academy of Engineering, London / Hybrid [Slides & Photos] [X/Twitter, 2, 3]
- 4 Mar 2025: Launch Event for the AI Hub in Generative Models (Gen AI Hub), Francis Crick Institute, London.
- 11 Feb 2025: EPSRC TERC Network Plus Launch Event, Royal Academy of Engineering, London
- Bringing together the six funded projects, including our Noise Network Plus
2024
- 10-15 Dec 2024: See you at the Thirty-Eighth Annual Conference on Neural Information Processing Systems (NeurIPS 2024), Vancouver, Canada.
- 6 Dec 2024: See you at the NeurIPS @ Cambridge meetup.
- 23-25 Oct 2024: See you at DCASE 2024 Workshop on Detection and Classification of Acoustic Scenes and Events, Tokyo, Japan
- 23-24 Sep 2024: See you at the 34th IEEE International Workshop on Machine Learning for Signal Processing (IEEE MLSP 2024), London, UK
- 12-13 Sep 2024: See you at the IOA ACOUSTICS 2024 50th Anniversary Conference, including the UKAN+ Annual Meeting 2024
- 3-7 Sept 2024: See you at the 27th International Conference on Digital Audio Effects (DAFx24), University of Surrey, UK.
Paper submission deadline: 20 Mar 2024 - 14-19 Apr 2024: Papers from our group at the International Conference on Acoustics, Speech, and Signal Processing (ICASSP 2024), Seoul, Korea. Accepted papers from the group:
- 20-27 Feb 2024: Xubo Liu will be at 38th AAAI Conference on Artificial Intelligence (AAAI-24), Vancouver, Canada, presenting our paper: Haohe Liu, Xubo Liu, Qiuqiang Kong, Wenwu Wang and Mark D. Plumbley. Learning Temporal Resolution in Spectrogram for Audio Classification. [arXiv]
- 31 Jan 2024: Attending the University of Surrey Annual Open Research Culture Event, University of Surrey, UK.
Haohe Liu presenting, shortlisted for an Open Research Award for his case study: Haohe Liu, Wenwu Wang, Mark D. Plumbley: Building the research community with open-source practice. - 16-17 Jan 2024: Attending Workshop on Interdisciplinary Perspectives on Soundscapes and Wellbeing, University of Surrey, UK.
(Day 1: Workshop & Webinar; Day 2: World Café & Sandpit Sessions
2023
- 26 Oct 2023: Attending Speech and Audio in the Northeast (SANE 2023), NYU, Brooklyn, New York.
Presentations from the group: - 22-25 Oct 2023: Attending IEEE Workshop on Applications of Signal Processing to Audio and Acoustics (WASPAA 2023), New Paltz, New York, USA. Presentations from the group:
- 20-22 Sep 2023: Attending DCASE 2023 Workshop Workshop on Detection and Classification of Acoustic Scenes and Events, 20-22 September 2023, Tampere, Finland. [Twitter/X]
Papers from the group:- Thu 21 Sep, P1-6: Jinbo Hu, Yin Cao, Ming Wu, Feiran Yang, Ziying Yu, Wenwu Wang, Mark D. Plumbley, Jun Yang: META-SELD: Meta-Learning for Fast Adaptation to the New Environment in Sound Event Localization and Detection
- Thu 21 Sep, P2-20: Yi Yuan, Haohe Liu, Xubo Liu, Xiyuan Kang, Peipei Wu, Mark D. Plumbley, Wenwu Wang: Text-Driven Foley Sound Generation with Latent Diffusion Model
- Fri 22 Sep, P3-25: Peipei Wu, Jinzheng Zhao, Yaru Chen, Davide Berghi, Yi Yuan, Chenfei Zhu, Yin Cao, Yang Liu, Philip JB Jackson, Mark D. Plumbley, Wenwu Wang: PLDISET: Probabilistic Localization and Detection of Independent Sound Events with Transformers
- Also participating in Discussion Panel (16:00 Thu 21 Sep)
- 19 Jul 2023: Great to see everyone on the CVSSP Summer Walk! [Twitter/X]
- 9-13 Jul 2023: Presenting Distinguished Plenary Lecture at 29th International Congress on Sound and Vibration (ICSV29), Prague.
- 22 Jun 2023: Presenting at the C4DM 20th Anniversary Celebration, Centre for Digital Music, Queen Mary University of London. [Twitter/X]
- 5-10 Jun 2023: Attending ICASSP 2023, Rhodes Island, Greece.
Presentations from the group:- Arshdeep Singh and Mark D. Plumbley: Efficient similarity-based passive filter pruning for compressing CNNs. [arXiv] [Open Access] [Twitter/X]
- Xubo Liu, Haohe Liu, Qiuqiang Kong, Xinhao Mei, Mark D. Plumbley and Wenwu Wang. Simple pooling front-ends for efficient audio classification. [arXiv] [Code]
- Haohe Liu, Zehua Chen, Yi Yuan, Xinhao Mei, Xubo Liu, Danilo Mandic, Wenwu Wang and Mark D Plumbley. Generating sound effects, music, speech, and beyond, with text [Show and Tell Demo, presenting AudioLDM]. [Twitter/X]
- 31 May 2023: Team placed first in DCASE Challenge Task 7 Track A "Foley Sound Synthesis" for submission: Yi Yuan, Haohe Liu, Xubo Liu, Xiyuan Kang, Mark D. Plumbley, Wenwu Wang: Latent Diffusion Model Based Foley Sound Generation System for DCASE Challenge 2023 Task 7 [Paper] [Code]
- 24 Apr 2023: Paper accepted for ICML 2023: Haohe Liu, Zehua Chen, Yi Yuan, Xinhao Mei, Xubo Liu, Danilo Mandic, Wenwu Wang, Mark D Plumbley. AudioLDM: Text-to-Audio Generation with Latent Diffusion Models [OpenReview]
- 19-21 Apr 2023: Presenting invited plenary talk at Urban Sound Symposum 2022, Barcelona, Spain. [Twitter/X]
- 20 Mar 2023: Open Research Case Study with Arshdeep Singh: Efficient Audio-based CNNs via Filter Pruning
- 14-21 Mar 2023: Presenting series of talks during visits to Redmond, WA & San Francisco Bay Area, CA, USA. Visits include:
- 14 Mar: Microsoft Research Lab, Redmond - with thanks to host: Ivan Tashev
- 15 Mar: Meta Reality Labs, Redmond - with thanks to host: Buye Xu
- 16 Mar: Adobe Research, San Francisco - with thanks to hosts: Gautham Mysore, Justin Salamon & Nick Bryan
- 16 Mar: Dolby Laboratories, San Francisco - with thanks to host: Mark Thomas
- 17 Mar: Stanford University, Center for Computer Research in Music and Acoustics (CCRMA) - with thanks to host: Malcolm Slaney
- 20 Mar: ByteDance, San Francisco - with thanks to host: Yuxuan Wang
- 20 Mar: Apple, Cupertino - with thanks to hosts: Miquel Espi Marques & Ahmed Tewfik
- 21 Mar: Amazon Lab126, Sunnyvale - with thanks to host: Trausti Kristjansson
- 29 Jan 2023: New paper on arXiv: Haohe Liu, Zehua Chen, Yi Yuan, Xinhao Mei, Xubo Liu, Danilo Mandic, Wenwu Wang, Mark D. Plumbley. AudioLDM: Text-to-Audio Generation with Latent Diffusion Models. arXiv:2301.12503 [cs.SD]. [Project Page] [Code on GitHub] [Hugging Face Space] [Twitter]
- 18 Jan 2023: Invited talk at LISTEN Lab Workshop, Télécom Paris.
- 12 Jan 2023: Invited talk at RISE Learning Machines Seminar series, RISE Research Institutes of Sweden. [YouTube] [Twitter]
- 9 Jan 2023: Welcome to Thomas Deacon, joining the AI for Sound project today!
2022
- 26 Dec 2022: Annamaria Mesaros awarded First Prize in the IEEE Finland Joint Chapter on Signal Processing and Circuits and Systems (SP/CAS) Paper Award 2022 for our paper: A. Mesaros, T. Heittola, T. Virtanen and M. D. Plumbley, Sound Event Detection: A tutorial, IEEE Signal Processing Magazine, vol. 38, no. 5, pp. 67-83, Sept. 2021 [arXiv] [Open Access] [Twitter/X]
- 12 Dec 2022: Congratulations to former PhD students Qiuqiang Kong and Turab Iqbal on being selected for the IEEE Signal Processing Young Author Best Paper Award, for the paper: Qiuqiang Kong*; Yin Cao; Turab Iqbal*; Yuxuan Wang; Wenwu Wang; Mark D. Plumbley: "PANNs: Large-Scale Pretrained Audio Neural Networks for Audio Pattern Recognition" IEEE/ACM Transactions on Audio, Speech, and Language Processing, 28, 2880-2894, 19 October 2020. DOI: 10.1109/TASLP.2020.3030497 [IEEE SPS Newsletter] [IEEE award page] [Paper] [arXiv] [Open Access] [Code]
- 1 Dec 2022: Virtual Keynote talk at the Amazon Audio Tech Summit, on "AI for Sound"
- 29 Nov 2022: Talk at the Centre for Digital Music at Queen Mary University of London, plus external PhD examiner.
- 21 Nov 2022: Welcome to Gabriel Bibbó, joining the AI for Sound project today!
- 3-4 Nov 2022: Attending (virtually) the DCASE 2022 Workshop on Detection and Classification of Acoustic Scenes and Events. Papers from the group:
- Arshdeep Singh, Mark D. Plumbley. Low-complexity CNNs for Acoustic Scene Classification. [arXiv]
- Yang Xiao, Xubo Liu, James King, Arshdeep Singh, Eng Siong Chng, Mark D. Plumbley, Wenwu Wang. Continual Learning For On-Device Environmental Sound Classification. [arXiv]
- Haohe Liu, Xubo Liu, Xinhao Mei, Qiuqiang Kong, Wenwu Wang, Mark D. Plumbley. Segment-level Metric Learning for Few-shot Bioacoustic Event Detection. [arXiv]
- Jinbo Hu, Yin Cao, Ming Wu, Qiuqiang Kong, Feiran Yang, Mark D. Plumbley, Jun Yang. Sound event localization and detection for real spatial sound scenes: event-independent network and data augmentation chains. [arXiv]
- 21-24 Aug 2022: Attending 51st International Congress and Exposition on Noise Control Engineering (Inter-noise 2022), Glasgow, Scotland [Full Programme]
- 22 Aug: Presenting our paper: Creating a new research community on detection and classification of acoustic scenes and events: Lessons from the first ten years of DCASE challenges and workshops (Mark D. Plumbley and Tuomas Virtanen)
Session: MON9.1/d - Advances in Environmental Noise (14:40-17:00)
- 22 Aug: Presenting our paper: Creating a new research community on detection and classification of acoustic scenes and events: Lessons from the first ten years of DCASE challenges and workshops (Mark D. Plumbley and Tuomas Virtanen)
- 17-23 Jul 2022: Attending (virtually) 39th International Conference on Machine Learning (ICML 2022).
- 13 Jul 2022: On CVSSP Summer Walk to Albury [Twitter]
- 11-12 Jul 2022: At two-day face-to-face workshop on "Designing AI for Home Wellbeing"
- 12 Jul: "Designing AI for Home Wellbeing" Seminar Day, supported by the Surrey Institute for People-Centred AI [LinkedIn]
- 11 Jul: "Designing AI for Home Wellbeing" World Cafe, supported by the University of Surrey Institute of Advanced Studies [LinkedIn]
- 30 Jun 2022: Dr Emily Corrigan-Kavanagh co-organizing in-person "Designing AI for Home Wellbeing" World Cafe at the Design Research Society conference DRS 2022 Bilbao (25 Jun - 3 Jul)
- 27 Jun - 1 Jul 2022: Interviewed by Tech Monitor (27 Jun) and BBC Tech Tent [0:17:20-0:22:00] (1 Jul) on virtual reality sound.
- 16 Jun 2022: Paper accepted for 32nd IEEE International Workshop on Machine Learning for Signal Processing (MLSP 2022), Xi'an, China, 22-25 Aug 2022:
- Meng Cui, Xubo Liu, Jinzheng Zhao, Jianyuan Sun, Guoping Lian, Tao Chen, Mark D. Plumbley, Daoliang Li, Wenwu Wang. Fish Feeding Intensity Assessment in Aquaculture: A New Audio Dataset AFFIA3K and A Deep Learning Algorithm
- 15 Jun 2022: Three papers accepted for INTERSPEECH 2022, Incheon, Korea, 18-22 September 2022:
- Xubo Liu, Haohe Liu, Qiuqiang Kong, Xinhao Mei, Jinzheng Zhao, Qiushi Huang, Mark D. Plumbley, Wenwu Wang: Separate What You Describe: Language-Queried Audio Source Separation [arXiv] [Code] [Demo]
- Xinhao Mei, Xubo Liu, Jianyuan Sun, Mark Plumbley and Wenwu Wang. On Metric Learning for Audio-Text Cross-Modal Retrieval [arXiv] [Code]
- Arshdeep Singh and Mark D. Plumbley. A Passive Similarity based CNN Filter Pruning for Efficient Acoustic Scene Classification [arXiv]
- 16 May 2022: Papers accepted for 30th European Signal Processing Conference (EUSIPCO), Belgrade, Serbia, 30 Aug - 2 Sep 2022:
- Xubo Liu, Xinhao Mei, Qiushi Huang, Jianyuan Sun, Jinzheng Zhao, Haohe Liu, Mark D. Plumbley, Volkan Kılıç, Wenwu Wang: Leveraging Pre-trained BERT for Audio Captioning [arXiv]
- Jianyuan Sun, Xubo Liu, Xinhao Mei, Jinzheng Zhao, Mark D. Plumbley, Volkan Kılıç, Wenwu Wang. Deep Neural Decision Forest for Acoustic Scene Classification [arXiv]
- 7-13 May 2022: At IEEE International Conference on Acoustics, Speech and Signal Processing (ICASSP 2022) virtual sessions
- 13 May: Bin Li presents our Journal of Selected Topics in Signal Processing article: Sparse Analysis Model Based Dictionary Learning for Signal Declipping (Bin Li, Lucas Rencker, Jing Dong, Yuhui Luo, Mark D. Plumbley and Wenwu Wang) [Presentation] [Paper] [Open Access] [Code]
- 11 May: Xinhao Mei presents our paper: Diverse Audio Captioning via Adversarial Training (Xinhao Mei, Xubo Liu, Jianyuan Sun, Mark Plumbley and Wenwu Wang) [Presentation] [Paper] [Open Access] [arXiv]
- 10 May: Zhao Ren presents our IEEE Transactions on Multimedia article: CAA-Net: Conditional Atrous CNNs With Attention for Explainable Device-Robust Acoustic Scene Classification (Zhao Ren, Qiuqiang Kong, Jing Han, Mark D. Plumbley and Bjorn W Schuller) [Presentation] [Paper] [Open Access] [arXiv] [Code]
- 8 May: Annamaria Mesaros presents our Signal Processing Magazine article: Sound Event Detection: A Tutorial (Annamaria Mesaros, Toni Heittola, Tuomas Virtanen and Mark D Plumbley) [Presentation] [Paper] [Open Access] [arXiv]
- 7 May: Jinbo Hu presents our challenge paper: A Track-Wise Ensemble Event Independent Network for Polyphonic Sound Event Localization and Detection (Jinbo Hu, Ming Wu, Feiran Yang, Jun Yang, Yin Cao, Mark Plumbley) [Presentation] [Paper] [Open Access] [arXiv] [Code]
- 5 Apr 2022: At UKAN Workshop on Soundscapes, including leading the afternoon in-person World Cafe, at Imperial College London. [Flyer (PDF)] [Twitter] [2] [3]
- 7 Mar 2022: At Virtual World Cafe on Exploring Sounds Sensing to Improve Workplace Wellbeing, organised by Dr Emily Corrigan-Kavanagh
2021
- 3 Dec 2021: Dr Emily Corrigan-Kavanagh presents our paper: Envisioning Sound Sensing Technologies for Enhancing Urban Living (Emily Corrigan-Kavanagh, Andres Fernandez, Mark D. Plumbley) at Environments By Design: Health, Wellbeing And Place, Virtual, 1-3 Dec 2021
- 15-19 Nov 2021: At the DCASE 2021 Workshop on Detection and Classification of Acoustic Scenes and Events, Online
- 19 Nov: Moderating the Town Hall discussion
[Twitter] [Webpage] - 18 Nov: Turab Iqbal presents our poster: ARCA23K: An Audio Dataset for Investigating Open-Set Label Noise (Turab Iqbal, Yin Cao, Andrew Bailey, Mark D. Plumbley, Wenwu Wang)
[Twitter] [Paper] [Poster] [Video] [Code] [Data] - 18 Nov: Xinhao Mei presents our poster: Audio Captioning Transformer (Xinhao Mei, Xubo Liu, Qiushi Huang, Mark D. Plumbley, Wenwu Wang)
[Twitter] [Paper] [Poster] [Video] - 17 Nov: Andres Fernandez presents our poster: Using UMAP to Inspect Audio Data for Unsupervised Anomaly Detection Under Domain-Shift Conditions (Andres Fernandez, Mark D. Plumbley)
[Twitter] [Paper] [Poster] [arXiv] [Video] [Code] [Data] [Webpage] - 17 Nov: Xubo Liu and Qiushi Huang present our poster: CL4AC: A Contrastive Loss for Audio Captioning (Xubo Liu, Qiushi Huang, Xinhao Mei, Tom Ko, H. Tang, Mark D. Plumbley, Wenwu Wang)
[Paper] [Poster] [Video] [Code] - 17 Nov: Xinhao Mei presents our poster: An Encoder-Decoder Based Audio Captioning System with Transfer and Reinforcement Learning (Xinhao Mei, Qiushi Huang, Xubo Liu, Gengyun Chen, Jingqian Wu, Yusong Wu, Jinzheng Zhao, Shengchen Li, Tom Ko, H. Tang, Xi Shao, Mark D. Plumbley, Wenwu Wang)
[Paper] [Poster] [Video] [Code]
- 19 Nov: Moderating the Town Hall discussion
- 12 Nov 2021: At "Envisioning Future Homes for Wellbeing" online event, as a "virtual world cafe". [News] [Graphic]
- 2 Nov 2021: At "Technologies for Home Wellbeing" in-person event, including "Research and Innovation in Technologies for Home Wellbeing" and "Future Technologies for Home Wellbeing" [Programme (PDF)] [News]
- 18-20 Oct 2021: At IEEE Workshop on Applications of Signal Processing to Audio and Acoustics (WASPAA 2021) (virtual)
- 15 Sep 2021: Francesco Renna presents our paper: Francesco Renna, Mark Plumbley and Miguel Coimbra: Source separation of the second heart sound via alternating optimization, Proc Computing in Cardiology - CinC 2021, Brno, Czech Republic [Abstract] [PDF Abstract] [Preprint] [Preprint]
- 23-27 Aug 2021: At 29th European Signal Processing Conference (EUSIPCO 2021) (virtual)
- 27 Aug: Andrew Bailey presents our paper: Andrew Bailey and Mark D. Plumbley. Gender bias in depression detection using audio features. [Paper]
- 25 Aug: Lam Pham presents our paper: Lam Pham, Chris Baume, Qiuqiang Kong, Tassadaq Hussain, Wenwu Wang, Mark Plumbley. An Audio-Based Deep Learning Framework For BBC Television Programme Classification. [Paper]
- 30 July 2021: Presenting invited talk on "Machine Learning for Sound Sensing" in the Workshop on Applications of Machine Learning at the Twenty Seventh National Conference on Communications (NCC-2021) Virtual Conference, organized by IIT Kanpur and IIT Roorkee, India, 27-30 July 2021 [Twitter]
- 6-11 June 2021: At the 2021 IEEE International Conference on Acoustics, Speech and Signal Processing (ICASSP 2021), Toronto (Virtual), Canada
- 10 June: Yin Cao, Qiuqiang Kong and Turab Iqbal present our poster: An Improved Event-Independent Network for Polyphonic Sound Event Localization and Detection (Yin Cao, Turab Iqbal, Qiuqiang Kong, Fengyan An, Wenwu Wang, Mark D. Plumbley) [Twitter] [Paper] [arXiv] [Code]
- 8 June: Jingzhu Zhang presents our poster: Weighted magnitude-phase loss for speech dereverberation (Jingshu Zhang, Mark D. Plumbley, Wenwu Wang) [Twitter] [Paper] [Open Access]
- 19-21 April 2021: See you at the Urban Sound Symposium
- Accepted poster with Dr Emily Corrigan-Kavanagh: "Exploring Sound Sensing to Improve Quality of Life in Urban Living"
- 24 Mar 2021: Presenting Connected Places Catapult Breakfast Briefing: Enabling Thriving Urban Communities through Sound Sensing AI Technology, with Dr Emily Corrigan-Kavanagh [Twitter] [LinkedIn] [Video]
- 23-24 Mar 2021: See you at The Turing Presents: AI UK 2021
- Tue 23 Mar (Day 1) at 16:00 Presenting a Spotlight Talk on "AI for Sound"
- 19-22 Jan 2021: At EUSIPCO 2020: 28th European Signal Processing Conference, Virtal Amsterdam.
- Wed 20 Jan: Emad Grais presents our paper in session ASMSP-7: Speech and Audio Separation: Multi-Band Multi-Resolution Fully Convolutional Neural Networks for Singing Voice Separation (Emad M. Grais, Fei Zhao, Mark D. Plumbley) [Paper]
- Fri 22 Jan: Attending meeting of EURASIP Technical Area Committee on Acoustic, Speech and Music Signal Processing (ASMSP TAC)
2020
- 2 Dec 2020: Plenary lecture at iTWIST 2020: international Traveling Workshop on Interactions between low-complexity data models and Sensing Techniques, Virtual Nantes, France, December 2-4, 2020
- 12 Nov 2020: Invited talk with Dr Emily Corrigan-Kavanagh at #LboroAppliedAI seminar series on Applied AI, Univ of Loughborough (virtual)
- 2-4 Nov 2020: At Workshop on Detection and Classification of Acoustic Scenes and Events (DCASE 2020) Virtual Tokyo, Japan
- Yin Cao presents our paper: Event-independent network for polyphonic sound event localization and detection (Yin Cao, Turab Iqbal, Qiuqiang Kong, Yue Zhong, Wenwu Wang, Mark D. Plumbley) [Paper] [Video] [Code]
- Saeid Safavi presents our poster: Open-window: A sound event dataset for window state detection and recognition (Saeid Safavi, Turab Iqbal, Wenwu Wang, Philip Coleman, Mark D. Plumbley) [Paper] [Video] [Dataset] [Code]
- Our team wins Judges’ Award for DCASE 2020 Challenge Task 5 “Urban Sound Tagging with Spatiotemporal Context”!
Congratulations to Turab Iqbal, Yin Cao, and Wenwu Wang [News Item] [Twitter] - Our team wins Reproducible System Award for DCASE 2020 Challenge Task 3 “Sound Event Localization and Detection”!
Congratulations to Yin Cao, Turab Iqbal, Qiuqiang Kong, Zhong Yue, and Wenwu Wang [News Item] [Twitter] [Paper] [Code] - Joint 1st place team in DCASE 2020 Challenge Task 5 “Urban Sound Tagging with Spatiotemporal Context” with entry: Turab Iqbal, Yin Cao, Mark D. Plumbley and Wenwu Wang: Incorporating Auxiliary Data for Urban Sound Tagging [Paper] [Code]
- 14-16 Oct 2020: Attending BBC Sounds Amazing 2020
- 1 Oct 2020: New award of £2.2 million Multimodal Video Search by Examples (MVSE) [2][3], collaborative project including Hui Wang (Ulster), Mark Gales (Cambridge) and Josef Kittler, Miroslav Bober & Wenwu Wang (Surrey)
- 1 Oct 2020: Welcome to Marc Green, joining the AI for Sound project
- 24 Sep 2020: New award of £1.4 million UK Acoustics Network Plus (UKAN+) [News Item]
- 21 Sep 2020: Welcome to Andres Fernandez, joining the AI for Sound project
- 16 Sep 2020: Presentation at Huawei Future Device Technology Summit 2020
- 2 Sep 2020: Welcome to Dr Emily Corrigan-Kavanagh, joining the AI for Sound project
- 27 Aug 2020: Presentation at online meeting of the Association of Noise Consultants (ANC) [LinkedIn]
- 1 Jul 2020: Presentation to Samsung AI Centre Cambridge, partners of the EPSRC Fellowship in "AI for Sound".
- 17 Jun 2020: Recruiting for three researchers on AI for Sound project (deadline 17 July 2020):
- 5 Jun 2020: Starting new EPSRC Fellowship in "AI for Sound". Press Release: Fellowship to advance sound to new frontiers using AI
- 27 May 2020: Recruiting for Research Fellow on Advanced Machine Learning for Audio Tagging (deadline 27 June 2020)
- 4-8 May 2020: (Virtually) at 45th International Conference on Acoustics, Speech, and Signal Processing (ICASSP 2020)
- [Postponed to 2021: 24 Mar 2020: Talk on "AI for Sound" at The Turing Presents: AI UK, London, UK.]
- 24 Jan 2020: Papers accepted for ICASSP 2020:
- Turab Iqbal, Yin Cao, Qiuqiang Kong, Mark D. Plumbley and Wenwu Wang. Learning with out-of-distribution data for audio classification.
- Qiuqiang Kong, Yuxuan Wang, Xuchen Song, Yin Cao, Wenwu Wang and Mark D. Plumbley. Source separation with weakly labelled data: An approach to computational auditory scene analysis. [arXiv:2002.02065]
- 15 Jan 2020: At PHEE 2020 Conference, London, UK
2019
- 22 Nov 2019: Zhao Ren presented our paper at 9th International Conference on Digital Public Health (DPH 2019), Marseille, France: Zhao Ren, Jing Han, Nicholas Cummins, Qiuqiang Kong, Mark D. Plumbley and Björn W. Schuller. Multi-instance learning for bipolar disorder diagnosis using weakly labelled speech data. [Paper] [Open Access]
- 1 Nov 2019: At UK Acoustics Network (UKAN) 2nd Anniversary Event, London, UK [Twitter]
- 25-26 Oct 2019: At DCASE2019 Workshop on Detection and Classification of Acoustic Scenes and Events, 25-26 October 2019, New York, USA [Twitter]
- Our team wins the Reproducible System Award for DCASE2019 Task 3!
Well done to Yin Cao, Turab Iqbal, Qiuqiang Kong, Miguel Blanco Galindo, Wenwu Wang.
[Paper and Code (reproducible!)] - Yin Cao presenting our poster: Polyphonic Sound Event Detection and Localization using a Two-Stage Strategy.
[Paper] [Code] [Twitter] - Francois Grondin presenting our paper with Iwona Sobieraj and James Glass: Sound event localization and detection using CRNN on pairs of microphones [Paper] [Twitter]
- Our team wins the Reproducible System Award for DCASE2019 Task 3!
- 24 Oct 2019: At Speech and Audio in the Northeast (SANE 2019), Columbia University, New York City, USA [Twitter: #SANE2019]
- Yin Cao and Saeid Safavi presented our demo on sound recognition, generalisation and visualisations [Twitter] [Slides] [YouTube]
- Turab Iqbal presented our poster: Learning with Out-of-Distribution Data for Audio Classification [Twitter]
- Yin Cao presented our poster: Cross-task learning for audio tagging, sound event detection and spatial localization: DCASE 2019 baseline systems [Twitter]
- 20-23 Oct 2019: At IEEE Workshop on Applications of Signal Processing to Audio and Acoustics (WASPAA 2019), New Paltz, New York, USA [Twitter: #WASPAA2019]
- 16 Oct 2019: Talk at Sound Sensing in Smart Cities 2 at Connected Places Catapult, London, on "AI for Sound: A Future Technology for Smart Cities"
- 30 Sep 2019: Opening Keynote at the 50th Annual Conference of the International Association of Sound and Audiovisual Archives (IASA 2019), Hilversum, The Netherlands [Photos]
- 5 Sep 2019: Emad Grais presented our paper at EUSIPCO 2019: Grais, Wierstorf, Ward, Mason & Plumbley. Referenceless performance evaluation of audio source separation using deep neural networks [Session: ThAP1: ASMSP PIII, Thursday, September 5 13:40 - 15:40, Poster Area 1] [PDF] [Open Access]
- 15 Aug 2019: Wenwu Wang presented our paper at IJCAI-19: Kong, Xu, Jackson, Wang, Plumbley. Single-Channel Signal Separation and Deconvolution with Generative Adversarial Networks [Session: ML|DL - Deep Learning 7 (L), Thu 15 Aug, 17:15] [Abstract] [Proceedings] [PDF] [arXiv:1906.07552] [Source Code]
- 23-25 Jul 2019: Co-organized AI Summer School at University of Surrey [Twitter: #AISurreySummerSchool] [Videos]
- 28 Jun 2019: Great result in DCASE2019 Task 3 "Sound Event Localization and Detection" for CVSSP Team (Yin Cao, Turab Iqbal, Qiuqiang Kong, Miguel Blanco Galindo, Wenwu Wang, Mark Plumbley). Top academic team and 2nd overall [Abstract] [Report] [Source Code]
- 26 Jun 2019: At Human Motion Analysis for Healthcare Applications Conference, IET London. [Twitter: #Human_Motion_Analysis]
- 25 Jun 2019: At Soundscape Workshop 2019, London [Twitter: #SoundscapeWorkshop2019]
- 20 Jun 2019: Talk at Connected Places Catapult, London, on "AI for Sound: A Future Technology for Smarter Living and Travelling" [Twitter (2) (3) (4)]
- See report by UKAuthority: Sound sensors ‘could support smart cities and assistive tech’
- 13 Jun 2019: Talk at the International Hearing Instruments Developer Forum (HADF 2019), Oldenburg, Germany on "Computational analysis of sound scenes and events"
- 3 Jun 2019: Paper accepted for EUSIPCO 2019: Emad M. Grais, Hagen Wierstorf, Dominic Ward, Russell Mason and Mark D. Plumbley. Referenceless performance evaluation of audio source separation using deep neural networks. [arXiv:1811.00454]
- 12-17 May 2019: At IEEE International Conference on Acoustics, Speech, and Signal Processing Signal Processing (ICASSP 2019), Brighton, UK
- Fri 17 May (pm): Qiuqiang Kong presented our poster: Kong, Xu, Iqbal, Cao, Wang & Plumbley: Acoustic Scene Generation with Conditional SampleRNN [Twitter] [Open Access] [Source Code] [Sound Samples]
- Fri 17 May (am): Christian Kroos presented our talk: Kroos, Bones, Cao, Harris, Jackson, Davies, Wang, Cox & Plumbley: Generalisation in Environmental Sound Classification: The 'Making Sense of Sounds' Data Set and Challenge [Open Access] [MSoS Challenge] [Dataset]
- Tue 14 May: Co-authoring three papers in session "Detection and Classification of Acoustic Scenes and Events II"
- Zhao Ren presented our talk: Ren, Kong, Han, Plumbley, Schuller: Attention-based Atrous Convolutional Neural Networks: Visualisation and Understanding Perspectives of Acoustic Scenes[Twitter] [Open Access]
- Yuanbo Hou presented our talk: Hou, Kong, Li, Plumbley: Sound Event Detection with Sequentially Labelled Data Based on Connectionist Temporal Classification and Unsupervised Clustering [Twitter] [Open Access]
- Zuzanna Podwinska presented our talk: Podwinska, Sobieraj, Fazenda, Davies, Plumbley: Acoustic Event Detection from Weakly Labeled Data Using Auditory Salience [Twitter] [Open Access]
- 13 May: Many congratulations to Zhijin Qin, presented with IEEE SPS Young Author Best Paper Award for: Qin, Gao, Plumbley, Parini. Wideband spectrum sensing on real-time signals at sub-Nyquist sampling rates in single and cooperative multiple nodes. IEEE Transactions on Signal Processing. 64: 3106-3117, 2016 [Twitter]
- 12-13 May: Tutorials Co-chair
- 10 May 2019: Paper accepted for IJCAI-19: Qiuqiang Kong, Yong Xu, Wenwu Wang, Philip J.B. Jackson and Mark D. Plumbley. Single-Channel Signal Separation and Deconvolution with Generative Adversarial Networks. [Abstract] [arXiv:1906.07552] [Source Code] (Acceptance rate: 18%)
- 18 Apr 2019: Now availble Open Access: Chungeun Kim, Emad M. Grais, Russell Mason and Mark D. Plumbley. Perception of phase changes in the context of musical audio source separation. AES 145th Convention, New York, 17-20 October 2018. [Open Access]
- 16 Apr 2019: Cross-task baseline code for all DCASE 2019 tasks released. Report: Qiuqiang Kong, Yin Cao, Turab Iqbal, Yong Xu, Wenwu Wang and Mark D. Plumbley. Cross-task learning for audio tagging, sound event detection and spatial localization: DCASE 2019 baseline systems [arXiv:1904.03476]
Source Code: - 4 Apr 2019: Presenting work from our sound recognition projects at the CVSSP 30th Anniversary event [Twitter (2)]
- 13 Mar 2019: Co-organizing kick-off meeting of AI@Surrey, bringing together AI related research across the University of Surrey [Twitter (2)]
- 20 Feb 2019: Paper published: Qiuqiang Kong, Yong Xu, Iwona Sobieraj, Wenwu Wang and Mark D. Plumbley. Sound event detection and time-frequency segmentation from weakly labelled data. IEEE/ACM Transactions on Audio, Speech and Language Processing 27(4): 777-787, April 2019. DOI:10.1109/TASLP.2019.2895254 [Open Access] [Source Code]
- 31 Jan 2019: AudioCommons project completed. See also: Deliverables & Papers; Tools & Resources
- 2 Jan 2019: Paper published: Estefanía Cano, Derry FitzGerald, Antoine Liutkus, Mark D. Plumbley and Fabian-Robert Stöter. Musical Source Separation: An Introduction. IEEE Signal Processing Magazine 36(1):31-40, January 2019. DOI:10.1109/MSP.2018.2874719 [Open Access]
2018
- 18 Dec 2018: Tropical-themed CVSSP Christmas Party! [Twitter]
- 21-22 Nov 2018: Hosting the 5th General Meeting of the AudioCommons project at the University of Surrey [Twitter]
- 19-20 Nov 2018: Co-chair of DCASE 2018 Workshop on Detection and Classification of Acoustic Scenes and Events, Surrey, UK [Twitter: #DCASE2018] [Proceedings]
- 20 Nov:
- Chairing the final Panel Session [Twitter]
- Qiuqiang Kong presenting our poster: Kong, Iqbal, Xu, Wang, Plumbley: DCASE 2018 Challenge Surrey cross-task convolutional neural network baseline [Twitter]
Code: - 19 Nov:
- Turab Iqbal and Qiuqiang Kong presenting our poster: Iqbal, Kong, Plumbley, Wang: General-purpose audio tagging from noisy labels using convolutional neural networks
- Christian Kroos presenting our poster: Kroos, Bones, Cao, Harris, Jackson, Davies, Wang, Cox, Plumbley: The Making Sense of Sounds Challenge
- Zhao Ren presenting our talk: Ren, Kong, Qian, Plumbley, Schuller: Attention-based convolutional neural networks for acoustic scene classification [Twitter]
- Opening the workshop [Twitter]
- 18 Sep 2018: CVSSP team (with Turab Iqbal, Qiuqiang Kong, Wenwu Wang) ranked 3rd out of 558 systems on Kaggle for DCASE 2018 Challenge Task 2: Freesound General-Purpose Audio Tagging[News item]
- Report: Turab Iqbal, Qiuqiang Kong, Mark D. Plumbley and Wenwu Wang. Stacked convolutional neural networks for general-purpose audio tagging. Technical Report, Detection and Classification of Acoustic Scenes and Events 2018 (DCASE 2018) Challenge, September 2018 [Software Code]
- 8 Aug 2018: Making Sense of Sounds Challenge announced
- 26 Jul 2018: Shengchen Li presents our paper at the 37th Chinese Control Conference: Li, Dixon & Plumbley. A demonstration of hierarchical structure usage in expressive timing analysis by model selection tests. In: Proceedings of the 37th Chinese Control Conference, CCC2018, Wuhan, China, 25-27 July 2018, pp 3190-3195. DOI:10.23919/ChiCC.2018.8483169 [Open Access]
- 6 Jul 2018: Co-Chair of Audio Day 2018, University of Surrey, Guildford, UK [Twitter: #SurreyAudioDay]
- Hosting the closing Panel Discussion [Twitter]
- From the AudioCommons project:
- I present an overview of our work on the Making Sense of Sounds project [Twitter (2)]
- From the Musical Audio Repurposing using Source Separation project:
- 2-5 Jul 2018: Co-Chair of LVA/ICA 2018: 14th International Conference on Latent Variable Analysis and Signal Separation, University of Surrey, Guildford, UK [Twitter: #LVAICA2018]
- See also: Dan Stowell: Notes from LVA-ICA conference 2018
- 5 Jul
- Congratulations to Lucas Rencker for a "Best Student Paper Award"!
- Lucas Rencker presents our talk: Rencker, Bach, Wang & Plumbley. Consistent dictionary learning for signal declipping [Twitter] [Open Access]
- Alfredo Zermini presents our poster: Zermini, Kong, Xu, Plumbley & Wang. Improving Reverberant Speech Separation with Binaural Cues Using Temporal Context and Convolutional Neural Networks [Twitter] [Open Access]
- 4 Jul
- Cian O’Brien presenting our late-breaking poster: O’Brien & Plumbley: Latent Mixture Models for Automatic Music Transcription
- Qiuqiang Kong presenting our SiSEC poster: Ward, Kong & Plumbley. Source Separation with Long Time Dependency Gated Recurrent Units Neural Networks [Description] [Twitter]
- 3 Jul
- Emad M. Grais presenting our poster: Grais, Wierstorf, Ward & Plumbley. Multi-Resolution Fully Convolutional Neural Networks for Monaural Audio Source Separation [Twitter] [Open Access]
- Iwona Sobieraj presenting our late-breaking poster: Sobieraj, Rencker & Plumbley. Orthogonality-Regularized Masked NMF with KL-Divergence for Learning on Weakly Labeled Audio Data[Twitter]
- Opening the conference [Twitter]
- 5 Jun 2018: At introductory meeting of Communication and Room Acoustics SIG of UK Acoustics Network
- 23-26 May 2018: Group members at AES Milan 2018: 144th International Pro Audio Convention.
- Fri 25 May: Emad Grais presented our poster Grais & Plumbley: Combining Fully Convolutional and Recurrent Neural Networks for Single Channel Audio Source Separation [Twitter] [Details] [Poster (pdf) ]
- Thu 24 May: PhD student Qiuqiang Kong (Panellist) at Workshop W11: Deep Learning for Audio Applications [Twitter]
- Wed 23 May: Workshop (panel discussion) on Audio Repurposing Using Source Separation organized by our EPSRC project Musical Audio Repurposing using Source Separation, Chaired by Phil Coleman with postdoc Chungeun (Ryan) Kim (Panellist) [Twitter] [Blog from Phil Coleman]
- 18 May 2018: Visit to Audio Analytic at end of successful stay by PhD student Iwona Sobieraj.
- 18 May 2018: Papers accepted for EUSIPCO 2018:
- Emad M. Grais, Dominic Ward and Mark D. Plumbley. Raw Multi-Channel Audio Source Separation using Multi-Resolution Convolutional Auto-Encoders.
- Cian O’Brien and Mark D. Plumbley. A Hierarchical Latent Mixture Model for Polyphonic Music Analysis.
- 3 May 2018: Congratulations to Prof Trevor Cox, collaborator on the EPSRC-funded Making Sense of Sounds project, on new book Now You're Talking: Human Conversation from the Neanderthals to Artificial Intelligence.
- 30 Apr 2018: Congratulations to Dr Chris Baume on official award of PhD, for his thesis Semantic Audio Tools for Radio Production,
funded by BBC R&D as part of the BBC Audio Research Partnership. - 29 Apr 2018: Now Published (Open Access): Chris Baume, Mark D. Plumbley, David Frohlich, Janko Ćalić. PaperClip: A digital pen interface for semantic speech editing in radio production. Journal of the Audio Engineering Society 66(4):241-252, April 2018. DOI:10.17743/jaes.2018.0006 [Full text also at: http://epubs.surrey.ac.uk/845786/]
- 16-20 Apr 2018: At IEEE International Conference on Acoustics, Speech and Signal Processing (ICASSP 2018), Calgary, Alberta, Canada. [Twitter: #ICASSP2018]
- Iwona Sobieraj presented our poster Sobieraj, Rencker & Plumbley: Orthogonality-regularized masked NMF for learning on weakly labeled audio data [Tue 17 April, 13:30 - 15:30, Poster Area E] [Twitter]
- Qiuqiang Kong & Yong Xu presented two posters: (a) Kong, Xu, Wang & Plumbley: Audio Set classification with attention model: A probabilistic perspective and (b) Kong, Xu, Wang & Plumbley: A joint separation-classification model for sound event detection of weakly labelled data [Wed 18 Apr, 08:30 - 10:30, Poster Area C] [Twitter]
- Wenwu Wang & Philip Jackson presented our poster Huang, Jackson, Plumbley & Wang: Synthesis of images by two-stage generative adversarial networks [Wed 18 Apr, 13:30 - 15:30, Poster Area G] [Twitter]
- Yong Xu presented talk Xu, Kong, Wang & Plumbley: Large-scale weakly supervised audio classification using gated convolutional neural network [Thu 19 Apr, 13:30 - 13:50] [Twitter]
- Emad Grais presented our poster Ward, Wierstorf, Mason, Grais & Plumbley. BSS Eval or PEASS? Predicting the perception of singing-voice separation [Thu 19 April, 16:00 - 18:00, Poster Area D] [Twitter] [Details] [Study info] [Code]
- Cian O'Brien presented our poster O'Brien & Plumbley: Inexact proximal operators for lp-quasinorm minimization [Fri 20 Apr, 16:00 - 18:00, Poster Area G] [Twitter]
- Also: Springer stand with our new book: Tuomas Virtanen, Mark D. Plumbley &Dan Ellis Computational Analysis of Sound Scenes and Events [Twitter]
- 1 Apr 2018: Now Published (Open Access): Chris Baume, Mark D. Plumbley, Janko Ćalić and David Frohlich. A contextual study of semantic speech editing in radio production. International Journal of Human-Computer Studies 115:67-80, July 2018. DOI:10.1016/j.ijhcs.2018.03.006 [Full text also at: http://epubs.surrey.ac.uk/846079/]
- 1 Apr 2018: Paper accepted for 37th Chinese Control Conference, CCC2018: Shengchen Li, Simon Dixon and Mark D. Plumbley. A demonstration of hierarchical structure usage in expressive timing analysis by model selection tests.
- 23 Mar 2018: At SpaRTaN-MacSeNet Workshop on Sparse Representations and Compressed Sensing in Paris, organized by our SpaRTaN and MacSeNet Initial/Innovative Training Networks. [Twitter: #SpartanMacsenet]
- 22 Mar 2018: At SpaRTaN-MacSeNet Training Workshop, Paris [Twitter]
- 19 Mar 2018: Papers accepted for LVA/ICA 2018:
- Emad M. Grais, Hagen Wierstorf, Dominic Ward and Mark D. Plumbley. Multi-resolution fully convolutional neural networks for monaural audio source separation.
- Lucas Rencker, Francis Bach, Wenwu Wang and Mark D. Plumbley. Consistent dictionary learning for signal declipping.
- Alfredo Zermini, Qiuqiang Kong, Yong Xu, Mark D. Plumbley and Wenwu Wang. Improving reverberant speech separation with binaural cues using temporal context and convolutional neural networks.
- 14 Mar 2018: At launch of the Digital Catapult's Machine Intelligence Garage, London [Twitter: #MachineIntelligenceGarage]
- 6 Mar 2018: Welcome to Saeid Safavi, joining the EU H2020 project Audio Commons.
- 5 Mar 2018: Welcome to Ryan Kim, joining the EPSRC-funded project Musical Audio Repurposing using Source Separation
- 23 Feb 2018: Paper accepted for HCI International 2018: Tijs Duel, David M. Frohlich, Christian Kroos, Yong Xu, Philip J. B. Jackson and Mark D. Plumbley. Supporting audiography: Design of a system for sentimental sound recording, classification and playback.
- 22 Feb 2018: At 4th General Meeting of the AudioCommons project in Barcelona [Twitter (2)]
- 14 Feb 2018: Paper accepted for AES Milan 2018: Emad M. Grais and Mark D. Plumbley. Combining fully convolutional and recurrent neural networks for single channel audio source separation.
- 12 Feb 2018: Welcome to Manal Helal, joining the EPSRC-funded project Musical Audio Repurposing using Source Separation
- 29 Jan 2018: Papers accepted for ICASSP 2018:
- Qiang Huang, Philip Jackson, Mark D. Plumbley and Wenwu Wang. Synthesis of images by two-stage generative adversarial networks.
- Qiuqiang Kong, Yong Xu, Wenwu Wang and Mark D. Plumbley. A joint separation-classification model for sound event detection of weakly labelled data.
- Qiuqiang Kong, Yong Xu, Wenwu Wang and Mark D. Plumbley. Audio Set classification with attention model: A probabilistic perspective.
- Cian O'Brien and Mark D. Plumbley. Inexact proximal operators for lp-quasinorm minimization.
- Iwona Sobieraj, Lucas Rencker and Mark D. Plumbley. Orthogonality-regularized masked NMF for learning on weakly labeled audio data.
- Dominic Ward, Hagen Wierstorf, Russell D. Mason, Emad M. Grais and Mark D. Plumbley. BSS Eval or PEASS? Predicting the perception of singing-voice separation.
- Yong Xu, Qiuqiang Kong, Wenwu Wang and Mark D. Plumbley. Large-scale weakly supervised audio classification using gated convolutional neural network.
2017
- 4 Dec 2017: Iwona Sobieraj presents poster at Women in Machine Learning Workshop (WiML 2017): Masked Non-negative Matrix Factorization for Bird Detection Using Weakly Labelled Data
- 27 Nov 2017: Introducing the Communication Acoustics SIG at the Launch of UK Acoustics Network [Event] [Photos] [Twitter: #UKAcousticsLaunch]
- 16-17 Nov 2017: At Workshop on Detection and Classification of Acoustic Scenes and Events (DCASE 2017), Munch, Germany. [Twitter: #DCASE2017]
- Christian Kroos presented our paper: Kroos & Plumbley: Neuroevolution for Sound Event Detection in Real Life Audio: A Pilot Study [Twitter (2) (3) (4)] [Slides] [Video]
- Yong Xu and Qiuqiang Kong presented our technical report poster: Surrey-CVSSP System for DCASE2017 Challenge Task4 [Twitter]
- 15 Nov 2017: At Meeting of RCUK Digital Economy Programme Advisory Board (DE PAB), Swindon.
- 14 Nov 2017: Emad Grais at GlobalSIP 2017 presenting our poster: Grais & Plumbley: Single Channel Audio Source Separation using Convolutional Denoising Autoencoders.
- 1 Nov 2017: Chairing Advisory Board of the Software Sustainability Institute, Southampton.
- 25 Oct 2017: At Dutch Design Week 2017, Eindhoven, The Netherlands.
- 23-27 Oct 2017: Presentations from group at ISMIR 2017, Suzhou, China
- 27 Oct: Qiuqiang Kong presents our Late-Breaking poster "Music source separation using weakly labelled data" at [Extended abstract]
- 25 Oct: Shengchen Li presents our poster "Clustering expressive timing with regressed polynomial coefficients demonstrated by a model selection test" [Paper]
- 20 Oct 2017: Hagen Wierstorf presents our talk "Perceptual Evaluation of Source Separation for Remixing Music" at AES New York 2017 [Abstract] [Twitter] [Paper] [Open Access]
- 19 Oct 2017: At Speech and Audio in the North East (SANE 2017), New York, NY, USA [Twitter: #SANE2017]
- Presented our poster: Signal Processing, Psychoacoustic Engineering and Digital Worlds: Interdisciplinary Audio Research at the University of Surrey [Twitter]
- 16-18 Oct 2017: At WASPAA 2017, New Paltz, NY, USA
- 18 Oct: Keynote talk: Making Sense of Sounds: Machine Listening in the Real World [Twitter]
- 6 Oct 2017: Delighted that Department of Computer Science receives Athena SWAN Bronze award, glad to have helped the April 2017 submission.
- 4-7 Sep 2017: Handing back to Helen Treharne as returning Head of Department of Computer Science.
- 28 Aug - 2 Sep 2017: Presentations from group at EUSIPCO 2017:
- Qiuqiang Kong, Yong Xu and Mark D. Plumbley. Joint detection and classification convolutional neural network on weakly labelled bird audio detection.
- Cian O’Brien and Mark D. Plumbley. Automatic music transcription using low rank non-negative matrix decomposition.
- Lucas Rencker, Wenwu Wang and Mark D. Plumbley. Multivariate Iterative Hard Thresholding for sparse decomposition with flexible sparsity patterns.
- Iwona Sobieraj, Qiuqiang Kong and Mark D. Plumbley. Masked non-negative matrix factorization for bird detection using weakly labeled data.
- 22 Jun 2017: At 2017 AES International Conference on Semantic Audio, Erlangen, Germany [Twitter: #aessa17]
- See also: Report from Brecht De Man
- Presenting Opening Keynote: "Audio Event Detection and Scene Recognition" [Twitter]
- 8 Jun 2017: At Cheltenham Science Festival for Panel Discussion on Is Your Tech Listening To You? with Jason Nurse and Rory Cellan-Jones. Featured with interview in BBC Tech Tent 9 Jun 2017
News
In the media
ResearchResearch interests
My research concerns AI for Sound: using machine learning and signal processing for analysis and recognition of sounds. My focus is on detection, classification and separation of acoustic scenes and events, particularly real-world sounds, using methods such as deep learning, sparse representations and probabilistic models.
I have published over 400 papers in journals, conferences and books, including over 70 journal papers and the recent Springer co-edited book on Computational Analysis of Sound Scenes and Events.
Much of my research is funded by grants from EPSRC and EU, Innovate UK and other sources. I currently hold an EPSRC Fellowship on "AI for Sound", and recently led EPSRC projects Making Sense of Sounds and Musical Audio Repurposing using Source Separation, and two EU research training networks, SpaRTaN and MacSeNet. My total grant funding is around £54M, including £20M as Principal Investigator, Coordinator or Lead Applicant.
I was co-Chair of the DCASE 2018 Workshop on Detection and Classification of Acoustic Scenes and Events, co-Chair of the 14th International Conference on Latent Variable Analysis and Signal Separation (LVA/ICA 2018) and co-Chair of the Signal Processing with Adaptive Sparse Structured Representations (SPARS 2017) workshop.
Research projects
EPSRC grant EP/V007866/1, £1.4M total, £40k to Surrey, Apr 2021 – Mar 2025. Joint with Horoshenkov (Sheffield), Bristow (Surrey), plus 10 others at Imperial, Loughborough, Salford, LSBU, Manchester, Bath, Reading, Southampton, Nottingham Trent.
EPSRC grant EP/V002856/1, £2.2M total, £863k to Surrey, Apr 2021 – Sep 2024. Joint with with Kittler, Wang & Bober (Surrey); Wang (QUB), Bond & Mulvenna (Ulster); Gales (Cambridge), BBC R&D.
EPSRC Fellowship EP/T019751/1, £2.1M, May 2020 – Apr 2025.
EPSRC grant EP/N014111/1, £1.28M total, £875k to Surrey, Mar 2016 – Mar 2019. Joint with University of Salford.
EPSRC grant EP/L027119/1 (Queen Mary) & EP/L027119/2 (Surrey), £887,607, Nov 2014 – Oct 2018.
EPSRC grant EP/P022529/1, £1.58M, Aug 2017 – Jul 2022.
EU H2020 Research and Innovation Grant 688382 total €2.98M (~£2.2M), £470k to Surrey, Feb 2016 - Jan 2019.
EU Marie Skłodowska-Curie Action (MSCA) Innovative Training Network H2020-MSCA-ITN-2014 project 642685, Total €3.8M (£3.0M), £560k to Surrey, 2015-2018.
EU Marie Curie Actions Initial Training Networks, FP7-PEOPLE-2013-ITN project 607290. Total €2.8M (£2.1M), €840k (£630k) to Surrey, 2014-2018.
Research interests
My research concerns AI for Sound: using machine learning and signal processing for analysis and recognition of sounds. My focus is on detection, classification and separation of acoustic scenes and events, particularly real-world sounds, using methods such as deep learning, sparse representations and probabilistic models.
I have published over 400 papers in journals, conferences and books, including over 70 journal papers and the recent Springer co-edited book on Computational Analysis of Sound Scenes and Events.
Much of my research is funded by grants from EPSRC and EU, Innovate UK and other sources. I currently hold an EPSRC Fellowship on "AI for Sound", and recently led EPSRC projects Making Sense of Sounds and Musical Audio Repurposing using Source Separation, and two EU research training networks, SpaRTaN and MacSeNet. My total grant funding is around £54M, including £20M as Principal Investigator, Coordinator or Lead Applicant.
I was co-Chair of the DCASE 2018 Workshop on Detection and Classification of Acoustic Scenes and Events, co-Chair of the 14th International Conference on Latent Variable Analysis and Signal Separation (LVA/ICA 2018) and co-Chair of the Signal Processing with Adaptive Sparse Structured Representations (SPARS 2017) workshop.
Research projects
EPSRC grant EP/V007866/1, £1.4M total, £40k to Surrey, Apr 2021 – Mar 2025. Joint with Horoshenkov (Sheffield), Bristow (Surrey), plus 10 others at Imperial, Loughborough, Salford, LSBU, Manchester, Bath, Reading, Southampton, Nottingham Trent.
EPSRC grant EP/V002856/1, £2.2M total, £863k to Surrey, Apr 2021 – Sep 2024. Joint with with Kittler, Wang & Bober (Surrey); Wang (QUB), Bond & Mulvenna (Ulster); Gales (Cambridge), BBC R&D.
EPSRC Fellowship EP/T019751/1, £2.1M, May 2020 – Apr 2025.
EPSRC grant EP/N014111/1, £1.28M total, £875k to Surrey, Mar 2016 – Mar 2019. Joint with University of Salford.
EPSRC grant EP/L027119/1 (Queen Mary) & EP/L027119/2 (Surrey), £887,607, Nov 2014 – Oct 2018.
EPSRC grant EP/P022529/1, £1.58M, Aug 2017 – Jul 2022.
EU H2020 Research and Innovation Grant 688382 total €2.98M (~£2.2M), £470k to Surrey, Feb 2016 - Jan 2019.
EU Marie Skłodowska-Curie Action (MSCA) Innovative Training Network H2020-MSCA-ITN-2014 project 642685, Total €3.8M (£3.0M), £560k to Surrey, 2015-2018.
EU Marie Curie Actions Initial Training Networks, FP7-PEOPLE-2013-ITN project 607290. Total €2.8M (£2.1M), €840k (£630k) to Surrey, 2014-2018.
Supervision
Postgraduate research supervision
I welcome applications from excellent students who wish to study for a PhD in my areas of research interest. Some example potential PhD projects are given below. I am also happy to discuss your own ideas for a project that you think may be of interest.
For more information on undertaking PhD study see: Centre for Vision, Speech and Signal Processing: PhD Study. For information on how to apply, see: Vision, Speech and Signal Processing PhD.
Example potential PhD projects
Deep learning for audio models and representations
The aim of this project is to investigate new machine learning methods for discovering good representations from audio sensor data. Deep learning has demonstrated very good performance on many machine learning problems, including audio event detection and acoustic scene classification. However, the theory of the deep learning process is not well understood, and these models typically require large amounts of training data. Recent advances based on information theory, such as the Information Bottleneck method, and model compression offer a promising direction for learning deep learning models and representations. The project will pay particular attention to methods for learning without labels, such as unsupervised and self-supervised learning. Project outcomes are expected to include new methods for constructing and learning efficient models and representations of audio data, making best use of available training data and labels, where available.
Privacy-preserving machine learning for sound sensing
The aim of this project is to build methods for machine learning for sound sensors, while preserving privacy of people or activities that are part of the sensed sound environment. For sound monitoring, we may wish to deploy sound sensors in public spaces, homes, or on personal devices. If the traditional approach of a large central data store were used for machine learning on deployed sensors, if may be difficult to achieve privacy of individuals whose sounds were picked up by sensors. Recently methods have been proposed that attempt to preserve privacy. For example, federated learning allows training to take place on distributed client devices, without the data itself being transferred to a central server, while differential privacy allows aggregate information about a dataset to be disclosed without disclosing information on individual records. This project will use these and related methods to investigate and develop new methods to allow sound sensors to be updated based on sounds captured by deployed sensors, while avoiding distribution of private or sensitive information.
Fair machine learning of sound data
The aim of this project is to investigate potential biases in sound-related datasets and challenges, and develop ways to overcome such biases to ensure fair machine learning approaches for sound data. When machine learning is used to tackle real-world problems, we should ensure that these models do not incorporate bias. Biases in datasets may not only result in skewed classification performance, such as over-reporting of performance, but can also result in subjects with protected characteristics, such as race or gender, being unfairly penalised. Biases can occur due to many factors, such as imbalanced data collection, correlations of factors with protected characteristics, or the machine learning models themselves. Fair Machine Learning (FML) is an area of research that explores the idea of fair, unbiased classification, in an attempt to work towards a fairer society through the use of machine learning. This project will investigate potential biases in databases such as DCASE challenge databases, and investigate methodologies and tools to document, measure and counteract biases. We will also consider how to make such methods understandable, including investigating metrics to evaluate the transparency of different approaches, and explore interpretability frameworks for deep learning models used for audio classification, and how to improve these.
Active Learning for sounds
We will investigate methods for active learning for sound classification, where users are queried interactively for labels which are used to update labels and class boundaries. As well as reducing the burden of labelling compared to "brute force" labelling from scratch, active learning offer the potential for individual users to create personalized sets of classes which are more useful to their intended application. Active learning can also help preserve user privacy, using models created locally based on the user data, without transmitting this data outside of the device. Initial investigation may start from simulated semi-supervised active learning, where datasets are used which are fully labelled but where the labels are queried one at a time. Developed methods will be tested on real subjects, which may include users recruited via crowdsourcing approaches. We will also investigate combinations of active learning with methods designed to work with small numbers of labelled data, such as few-shot learning and self-supervised learning, to develop efficient methods that can learn sound classes from a small number of user queries.
Measurement of soundscapes and noise
The aim of this PhD project is to use sound recognition to develop new estimates of sound impacts on people, to enable improved measurement and design of soundscapes. Exposure to noise can have significant physical and mental health implications: The annual UK cost of noise impacts is 7 billion–10 billion GBP. Most currently noise measurements and noise policies rely on simple loudness measures (Sound Pressure Level, measured in e.g. dBA), which do not fully reflect the real impact on individual people. Automatically recognizing different sound sources, and creating new measures that reflect the impact of these sounds on different people, could help us to improve people’s experience of our sonic environment. In this project we will investigate and propose predictive soundscape models based on sound source recognition. The project may start from baseline sound recognition models, mapping these onto a valence-arousal affect (emotion) space, and would include soundwalks and laboratory studies to inform the development of new models, and to validate models on unseen data and surveys on indoor and/or outdoor settings. Methods produced by this project may contribute to future work on soundscape standards, and how these are used in future noise and soundscape policies to improve wellbeing of citizens.
Teaching
My main teaching is for EEE3008 Fundamentals of Digital Signal Processing.
I also supervise Year 3 Projects for EEE programmes (EEE3017) and MSc Dissertation Projects for EEE and CS (EEEM004 and COMM002).
Publications
Indoor soundscapes significantly impact wellbeing, yet methodologies for understanding their perception among older adults remain underdeveloped. This paper presents Soundscape Experience Mapping (SEM), combining ecological momentary assessment with participatory design methods to capture and analyse indoor acoustic environments. Through structured listening activities and audio data collection, participants document their acoustic experiences in context. Our pilot study engaged eight older adults (57+) in a town in Belgium, collecting continuous audio recordings and qualitative data over one week. Using momentary judgements , retrospective evaluations, and sound journalling, we gained insights into how older adults perceive their indoor soundscapes. The method produced findings on the personal control of sound environments, soundscape preferences, and how situational factors influence acoustic perception. Participants demonstrated agency in curating their sonic environments, while expressing frustration with uncontrollable sounds. Daily routines and domestic rhythms emerged as key contextual factors shaping sound-scape experiences. This work advances AI-assisted indoor soundscape design by providing evidence-based methods to understand occupant needs, particularly for older adults who could benefit from tailored acoustic environments.
As wireless technology continues to expand, there is a growing concern about the efficient use of spectrum resources. Even though a significant portion of the spectrum is allocated to licensed primary users (PUs), studies indicate that their actual utilization is often limited to between 5% to 10% [1]. The underutilization of spectrum has given rise to cognitive radio (CR) technology, which allows secondary users (SUs) to opportunistically access these underused resources [2]. However, wideband spectrum sensing, the key of CR, is limited by the need for high-speed analog-to-digital converters (ADCs), which are costly and power-hungry. Compressed spectrum sensing (CSS) addresses this challenge by employing sub-Nyquist rate sampling. The efficiency of active transmission detection heavily depends on the quality of spectrum reconstruction. There are various reconstruction methods in CSS, each with its merits and drawbacks. Still, existing algorithms have not tapped into the full potential of sub-sampling sequences, and their performance notably drops in noisy environments [3,4]. The GHz Bandwidth Sensing (GBSense) project1) introduces an innovative approach for GHz bandwidth sensing. GBSense incorporates advanced sub-Nyquist sampling methods and is compatible with low-power devices. This project also prompted the GBSense Challenge 2021, which centered on sub-Nyquist reconstruction algorithms, with four leading algorithms to be presented and evaluated in this paper.
The goal of Unsupervised Anomaly Detection (UAD) is to detect anomalous signals under the condition that only non-anomalous (normal) data is available beforehand. In UAD under DomainShift Conditions (UAD-S), data is further exposed to contextual changes that are usually unknown beforehand. Motivated by the difficulties encountered in the UAD-S task presented at the 2021 edition of the Detection and Classification of Acoustic Scenes and Events (DCASE) challenge, we visually inspect Uniform Manifold Approximations and Projections (UMAPs) for log-STFT, logmel and pretrained Look, Listen and Learn (L3) representations of the DCASE UAD-S dataset. In our exploratory investigation, we look for two qualities, Separability (SEP) and Discriminative Support (DSUP), and formulate several hypotheses that could facilitate diagnosis and developement of further representation and detection approaches. Particularly, we hypothesize that input length and pretraining may regulate a relevant tradeoff between SEP and DSUP. Our code as well as the resulting UMAPs and plots are publicly available.
Automated Audio captioning (AAC) is a cross-modal translation task that aims to use natural language to describe the content of an audio clip. As shown in the submissions received for Task 6 of the DCASE 2021 Challenges, this problem has received increasing interest in the community. The existing AAC systems are usually based on an encoder-decoder architecture, where the audio signal is encoded into a latent representation, and aligned with its corresponding text descriptions, then a decoder is used to generate the captions. However, training of an AAC system often encounters the problem of data scarcity, which may lead to inaccurate representation and audio-text alignment. To address this problem, we propose a novel encoder-decoder framework called Contrastive Loss for Audio Captioning (CL4AC). In CL4AC, the self-supervision signals derived from the original audio-text paired data are used to exploit the correspondences between audio and texts by contrasting samples, which can improve the quality of latent representation and the alignment between audio and texts, while trained with limited data. Experiments are performed on the Clotho dataset to show the effectiveness of our proposed approach.
The availability of audio data on sound sharing platforms such as Freesound gives users access to large amounts of annotated audio. Utilising such data for training is becoming increasingly popular, but the problem of label noise that is often prevalent in such datasets requires further investigation. This paper introduces ARCA23K, an Automatically Retrieved and Curated Audio dataset comprised of over 23 000 labelled Freesound clips. Unlike past datasets such as FSDKaggle2018 and FSDnoisy18K, ARCA23K facilitates the study of label noise in a more controlled manner. We describe the entire process of creating the dataset such that it is fully reproducible, meaning researchers can extend our work with little effort. We show that the majority of labelling errors in ARCA23K are due to out-of-vocabulary audio clips, and we refer to this type of label noise as open-set label noise. Experiments are carried out in which we study the impact of label noise in terms of classification performance and representation learning.
This paper presents a residential audio dataset to support sound event detection research for smart home applications aimed at promoting wellbeing for older adults. The dataset is constructed by deploying audio recording systems in the homes of 8 participants aged 55-80 years for a 7-day period. Acoustic characteristics are documented through detailed floor plans and construction material information to enable replication of the recording environments for AI model deployment. A novel automated speech removal pipeline is developed, using pre-trained audio neural networks to detect and remove segments containing spoken voice, while preserving segments containing other sound events. The resulting dataset consists of privacy-compliant audio recordings that accurately capture the sound-scapes and activities of daily living within residential spaces. The paper details the dataset creation methodology, the speech removal pipeline utilizing cascaded model architectures, and an analysis of the vocal label distribution to validate the speech removal process. This dataset enables the development and benchmarking of sound event detection models tailored specifically for in-home applications.
Convolution neural networks (CNNs) have shown great success in various applications. However, the computational complexity and memory storage of CNNs is a bottleneck for their deployment on resource-constrained devices. Recent efforts towards reducing the computation cost and the memory overhead of CNNs involve similarity-based passive filter pruning methods. Similarity-based passive filter pruning methods compute a pairwise similarity matrix for the filters and eliminate a few similar filters to obtain a small pruned CNN. However, the computational complexity of computing the pairwise similarity matrix is high, particularly when a convolutional layer has many filters. To reduce the computational complexity in obtaining the pairwise similarity matrix, we propose to use an efficient method where the complete pairwise similarity matrix is approximated from only a few of its columns by using a Nyström approximation method. The proposed efficient similarity-based passive filter pruning method is 3 times faster and gives same accuracy at the same reduction in computations for CNNs compared to that of the similarity-based pruning method that computes a complete pairwise similarity matrix. Apart from this, the proposed efficient similarity-based pruning method performs similarly or better than the existing norm-based pruning methods. The efficacy of the proposed pruning method is evaluated on CNNs such as DCASE 2021 Task 1A baseline network and a VGGish network designed for acoustic scene classification.
Continuously learning new classes without catastrophic forgetting is a challenging problem for on-device environmental sound classification given the restrictions on computation resources (e.g., model size, running memory). To address this issue, we propose a simple and efficient continual learning method. Our method selects the historical data for the training by measuring the per-sample classification uncertainty. Specifically, we measure the uncertainty by observing how the classification probability of data fluctuates against the parallel perturbations added to the classifier embedding. In this way, the computation cost can be significantly reduced compared with adding perturbation to the raw data. Experimental results on the DCASE 2019 Task 1 and ESC-50 dataset show that our proposed method outperforms baseline continual learning methods on classification accuracy and computational efficiency, indicating our method can efficiently and incrementally learn new classes without the catastrophic forgetting problem for on-device environmental sound classification.
Convolutional neural networks (CNNs) have exhibited state-of-the-art performance in various audio classification tasks. However, their real-time deployment remains a challenge on resource-constrained devices like embedded systems. In this paper, we present a demonstration of our standalone hardware device designed for real-time recognition of sound events commonly known as audio tagging. Our system incorporates a real-time implementation of a CNN-based pre-trained audio neural networks (PANNs) on an embedded hardware device, Raspberry Pi. We refer to our standalone device as "PiSoundSensing" system, which makes sense of surrounding sounds using a Raspberry Pi based hardware. Users can interact with the system through a physical button or using an online web interface. The web interface allows users to remotely control the standalone device, and visualize sound events detected over time. We provide a detailed description of the hardware and software used to build PiSoundSensing device. Also, we highlight useful observations including hardware-based standalone device performance compared to that of the software-based performance.
Convolutional neural networks (CNNs) have exhibited state-of-the-art performance in various audio classification tasks. However, their real-time deployment remains a challenge on resource constrained devices such as embedded systems. In this paper, we analyze how the performance of large-scale pre-trained audio neural networks designed for audio pattern recognition changes when deployed on a hardware such as a Raspberry Pi. We empirically study the role of CPU temperature, microphone quality and audio signal volume on performance. Our experiments reveal that the continuous CPU usage results in an increased temperature that can trigger an automated slowdown mechanism in the Raspberry Pi, impacting inference latency. The quality of a microphone, specifically with affordable devices such as the Google AIY Voice Kit, and audio signal volume, all affect the system performance. In the course of our investigation, we encounter substantial complications linked to library compatibility and the unique processor architecture requirements of the Raspberry Pi, making the process less straightforward compared to conventional computers (PCs). Our observations, while presenting challenges, pave the way for future researchers to develop more compact machine learning models, design heat-dissipative hardware, and select appropriate microphones when AI models are deployed for real-time applications on edge devices.
Fish feeding intensity assessment (FFIA) aims to evaluate the change of fish appetite during the feeding process, which is potentially useful in industrial aquaculture. Previous methods are mainly based on computer vision techniques. However, these methods are limited by water refraction and uneven illumination. In this paper, we introduce a new approach for FFIA using audio. We create a new audio dataset for FFIA, namely AFFIA3K, which contains 3000 labelled audio clips of different fish feeding intensity (None, Weak, Medium, Strong). We present a deep learning framework for FFIA, where the audio signal is first transformed into acoustic features, i.e. mel spectrogram, then a convolutional neural network (CNN)-based model is used to classify the fish feeding intensity. Experimental results show that our approach achieves an mAP of 0.74 on the test set of AFFIA3K, and considerably outperforms baseline systems. This indicates the potential of our proposed approach in aquaculture applications.
Audio-text retrieval aims at retrieving a target audio clip or caption from a pool of candidates given a query in another modality. Solving such cross-modal retrieval task is challenging because it not only requires learning robust feature representations for both modalities, but also requires capturing the fine-grained alignment between these two modalities. Existing cross-modal retrieval models are mostly optimized by metric learning objectives as both of them attempt to map data to an embedding space, where similar data are close together and dissimilar data are far apart. Unlike other cross-modal retrieval tasks such as image-text and video-text retrievals, audio-text retrieval is still an unexplored task. In this work, we aim to study the impact of different metric learning objectives on the audio-text retrieval task. We present an extensive evaluation of popular metric learning objectives on the AudioCaps and Clotho datasets. We demonstrate that NT-Xent loss adapted from self-supervised learning shows stable performance across different datasets and training settings, and outperforms the popular triplet-based losses. Our code is available at https://github.com/XinhaoMei/audio-text_retrieval.
Environmental sound classification (ESC) aims to automatically recognize audio recordings from the underlying environment, such as " urban park " or " city centre ". Most of the existing methods for ESC use hand-crafted time-frequency features such as log-mel spectrogram to represent audio recordings. However, the hand-crafted features rely on transformations that are defined beforehand and do not consider the variability in the environment due to differences in recording conditions or recording devices. To overcome this, we present an alternative representation framework by leveraging a pre-trained convolutional neural network, SoundNet, trained on a large-scale audio dataset to represent raw audio recordings. We observe that the representations obtained from the intermediate layers of SoundNet lie in low-dimensional subspace. However, the dimensionality of the low-dimensional subspace is not known. To address this, an automatic compact dictionary learning framework is utilized that gives the dimensionality of the underlying subspace. The low-dimensional embeddings are then aggregated in a late-fusion manner in the ensemble framework to incorporate hierarchical information learned at various intermediate layers of SoundNet. We perform experimental evaluation on publicly available DCASE 2017 and 2018 ASC datasets. The proposed ensemble framework improves performance between 1 and 4 percentage points compared to that of existing time-frequency representations.
The auditory system plays a substantial role in shaping the overall human perceptual experience. While prevailing large language models (LLMs) and visual language models (VLMs) have shown their promise in solving a wide variety of language and vision understanding tasks, only a few of them can be generalised to the audio domain without compromising their domain-specific capability. In this work, we introduce Acoustic Prompt Tuning (APT), a new adapter extending LLMs and VLMs to the audio domain by injecting audio embeddings to the input of LLMs, namely soft prompting. Specifically, APT applies an instruction-aware audio aligner to generate soft prompts, conditioned on both input text and sounds, as the inputs to the language model. To mitigate data scarcity in the audio domain, a curriculum learning strategy is proposed by formulating diverse audio tasks in a sequential manner. Moreover, we improve the audio language model by using interleaved audio-text embeddings as the input sequence. In this improved model, zero constraints are imposed on the input format, thus it is capable of tackling diverse modelling tasks, such as few-shot audio classification and audio comparison. To further evaluate the advanced ability of the audio networks, we introduce natural language audio reasoning (NLAR), a new task that analyses two audio clips by comparison and summarisation. Experiments show that APT-enhanced LLMs (namely APT-LLMs) achieve competitive results compared to the expert models (i.e., the networks trained on the target datasets) across various tasks. We finally demonstrate APT's ability in extending frozen VLMs to the audio domain without fine-tuning, achieving promising results in audiovisual question and answering. Our code and model weights will be released at https://github.com/JinhuaLiang/APT. Index Terms—Audio understanding, large language model, audio-language learning, audio recognition, automated audio captioning, natural language audio reasoning.
Audio captioning aims at using language to describe the content of an audio clip. Existing audio captioning systems are generally based on an encoder-decoder architecture, in which acoustic information is extracted by an audio encoder and then a language decoder is used to generate the captions. Training an audio captioning system often encounters the problem of data scarcity. Transferring knowledge from pre-trained audio models such as Pre-trained Audio Neural Networks (PANNs) have recently emerged as a useful method to mitigate this issue. However, there is less attention on exploiting pre-trained language models for the decoder, compared with the encoder. BERT is a pre-trained language model that has been extensively used in natural language processing tasks. Nevertheless, the potential of using BERT as the language decoder for audio captioning has not been investigated. In this study, we demonstrate the efficacy of the pre-trained BERT model for audio captioning. Specifically, we apply PANNs as the encoder and initialize the decoder from the publicly available pre-trained BERT models. We conduct an empirical study on the use of these BERT models for the decoder in the audio captioning model. Our models achieve competitive results with the existing audio captioning methods on the AudioCaps dataset.
Acoustic scene classification (ASC) aims to classify an audio clip based on the characteristic of the recording environment. In this regard, deep learning based approaches have emerged as a useful tool for ASC problems. Conventional approaches to improving the classification accuracy include integrating auxiliary methods such as attention mechanism, pre-trained models and ensemble multiple sub-networks. However, due to the complexity of audio clips captured from different environments, it is difficult to distinguish their categories without using any auxiliary methods for existing deep learning models using only a single classifier. In this paper, we propose a novel approach for ASC using deep neural decision forest (DNDF). DNDF combines a fixed number of convolutional layers and a decision forest as the final classifier. The decision forest consists of a fixed number of decision tree classifiers, which have been shown to offer better classification performance than a single classifier in some datasets. In particular, the decision forest differs substantially from traditional random forests as it is stochastic, differentiable, and capable of using the back-propagation to update and learn feature representations in neural network. Experimental results on the DCASE2019 and ESC-50 datasets demonstrate that our proposed DNDF method improves the ASC performance in terms of classification accuracy and shows competitive performance as compared with state-of-the-art baselines.
Audio captioning aims at generating natural language descriptions for audio clips automatically. Existing audio captioning models have shown promising improvement in recent years. However, these models are mostly trained via maximum likelihood estimation (MLE), which tends to make captions generic, simple and deterministic. As different people may describe an audio clip from different aspects using distinct words and grammars, we argue that an audio captioning system should have the ability to generate diverse captions for a fixed audio clip and across similar audio clips. To address this problem, we propose an adversarial training framework for audio captioning based on a conditional generative adversarial network (C-GAN), which aims at improving the naturalness and diversity of generated captions. Unlike processing data of continuous values in a classical GAN, a sentence is composed of discrete tokens and the discrete sampling process is non-differentiable. To address this issue, policy gradient, a reinforcement learning technique, is used to back-propagate the reward to the generator. The results show that our proposed model can generate more diverse captions, as compared to state-of-the-art methods.
Audio captioning aims to automatically generate a natural language description of an audio clip. Most captioning models follow an encoder-decoder architecture, where the decoder predicts words based on the audio features extracted by the encoder. Convolutional neural networks (CNNs) and recurrent neural networks (RNNs) are often used as the audio encoder. However, CNNs can be limited in modelling temporal relationships among the time frames in an audio signal, while RNNs can be limited in modelling the long-range dependencies among the time frames. In this paper, we propose an Audio Captioning Transformer (ACT), which is a full Transformer network based on an encoder-decoder architecture and is totally convolution-free. The proposed method has a better ability to model the global information within an audio signal as well as capture temporal relationships between audio events. We evaluate our model on AudioCaps, which is the largest audio captioning dataset publicly available. Our model shows competitive performance compared to other state-of-the-art approaches.
Automated audio captioning aims to use natural language to describe the content of audio data. This paper presents an audio captioning system with an encoder-decoder architecture, where the decoder predicts words based on audio features extracted by the encoder. To improve the proposed system, transfer learning from either an upstream audio-related task or a large in-domain dataset is introduced to mitigate the problem induced by data scarcity. Moreover, evaluation metrics are incorporated into the optimization of the model with reinforcement learning, which helps address the problem of " exposure bias " induced by " teacher forcing " training strategy and the mismatch between the evaluation metrics and the loss function. The resulting system was ranked 3rd in DCASE 2021 Task 6. Abla-tion studies are carried out to investigate how much each component in the proposed system can contribute to final performance. The results show that the proposed techniques significantly improve the scores of the evaluation metrics, however, reinforcement learning may impact adversely on the quality of the generated captions.
Noise Network Plus is a new interdisciplinary network designed to address the grand challenges for noise pollution for the next 10-15 years, supported by the UK Engineering and Physical Science Research Council (EPSRC).Noise has a major impact on public health, society and wildlife. It has a profound effect on human health and wellbeing, causing heart disease, high annoyance and sleep disturbance. Road noise in England alone is estimated to cause £7-10bn of health costs, with 130,000 healthy life years lost each year. It affects wildlife, including birds and marine mammals, damaging wildlife health and reproduction. Noise also affects AI systems and sensors, including sonar echo sounders and underwater acoustic modems.As well as existing sources of human-made noise, such as road, rail, and air transport, new technologies such as drones, air taxis and air source heat pumps could introduce new sources of noise. However, noise is often neglected until late in the engineering design process, resulting in products, systems and buildings that may create or transmit unnecessary noise.To address these challenges, we will create a new research and innovation network, bringing together diverse, dynamic teams from across disciplines to promote dialogue, co-design missions, form lasting and inclusive collaborations, and build unprecedented noise research capabilities. The Network will support pilot projects to test new research ideas, gather missing evidence and carry out feasibility studies.We will also reimagine the education and training of engineers, to include systems thinking in general, and sound and noise in particular, across engineering education.By understanding the complex systems that build noise into the world, we will begin a long-lasting programme of research and engagement to reduce noise and its impact on people, the environment, and the economy.
Language-queried audio source separation (LASS) is a new paradigm for computational auditory scene analysis (CASA). LASS aims to separate a target sound from an audio mixture given a natural language query, which provides a natural and scalable interface for digital audio applications. Recent works on LASS, despite attaining promising separation performance on specific sources (e.g., musical instruments, limited classes of audio events), are unable to separate audio concepts in the open domain. In this work, we introduce AudioSep, a foundation model for open-domain audio source separation with natural language queries. We train AudioSep on large-scale multimodal datasets and extensively evaluate its capabilities on numerous tasks including audio event separation, musical instrument separation, and speech enhancement. AudioSep demonstrates strong separation performance and impressive zero-shot generalization ability using audio captions or text labels as queries, substantially outperforming previous audio-queried and language-queried sound separation models. Specifically, AudioSep achieved strong results including a Signal-to-Distortion Ratio Improvement (SDRi) of 7.74 dB across 527 sound classes of the AudioSet; 9.14 dB on the VGGSound dataset; 8.22 dB on the AudioCaps dataset; 6.85 dB on the Clotho dataset; 10.51 dB on the MUSIC dataset; 10.04 dB on the ESC-50 dataset; 8.16 dB on the DCASE 2024 Task 9 dataset; and an SSNR of 9.21 dB on the VoicebankDemand dataset.
Understanding how individuals experience their acoustic environments is essential for sound planning and design. While standardised methods exist for measuring and analysing soundscapes, AI audio systems require more design-oriented, inclusive approaches to capture personal listening experiences. This poster introduces accessible Sound Awareness Activities that help participants explore and reflect on sounds in their homes and neighbourhoods to support indoor soundscape improvements.Our approach, originally part of a participatory design study, combines deep listening, sound journaling, memory-sound associations, and structured soundscape listening activities. Each activity is adaptable for different abilities and contexts to support inclusive participation. These activities go beyond measuring sound to capture personal meanings, emotional responses, and contextual factors that shape acoustic experiences. Though designed for a particular study, activities should work equally well in workshops and other engagement formats.The University of Surrey team conducted a week-long experience sampling study at Belgium's Living and Care Lab (LiCaLab) to explore the activities' effectiveness. Drawing on a thorough understanding of the local participant panel, the LiCaLab team helped refine Surrey's original protocol by providing insights for translation, localization, and adaptation. This collaboration demonstrated how activities can be carefully tailored while preserving core methodological objectives.The poster presentation provides an opportunity to share progress and gather feedback on a novel soundscape research methodology. We will provide practical implementation guidance, including strategies for making activities accessible, supporting diverse participation styles, and creating comfortable environments for sound exploration.This methodological contribution advances soundscape research by offering structured yet adaptable ways to gather rich qualitative data about residential sound experiences. For future work, we aim to consolidate the approach into a toolkit that supports the development of inclusive acoustic design approaches that consider diverse ways of listening and experiencing sound environments.
Depression is a large-scale mental health problem and a challenging area for machine learning researchers in detection of depression. Datasets such as Distress Analysis Interview Corpus - Wizard of Oz (DAIC-WOZ) have been created to aid research in this area. However, on top of the challenges inherent in accurately detecting depression, biases in datasets may result in skewed classification performance. In this paper we examine gender bias in the DAIC-WOZ dataset. We show that gender biases in DAIC-WOZ can lead to an overreporting of performance. By different concepts from Fair Machine Learning, such as data re-distribution, and using raw audio features, we can mitigate against the harmful effects of bias.
This paper proposes a deep learning framework for classification of BBC television programmes using audio. The audio is firstly transformed into spectrograms, which are fed into a pre-trained Convolutional Neural Network (CNN), obtaining predicted probabilities of sound events occurring in the audio recording. Statistics for the predicted probabilities and detected sound events are then calculated to extract discriminative features representing the television programmes. Finally, the embedded features extracted are fed into a classifier for classifying the programmes into different genres. Our experiments are conducted over a dataset of 6,160 programmes belonging to nine genres labelled by the BBC. We achieve an average classification accuracy of 93.7% over 14-fold cross validation. This demonstrates the efficacy of the proposed framework for the task of audio-based classification of television programmes.
Contrastive language-audio pretraining (CLAP) has become a new paradigm to learn audio concepts with audio-text pairs. CLAP models have shown unprecedented performance as zero-shot classifiers on downstream tasks. To further adapt CLAP with domain-specific knowledge, a popular method is to fine-tune its audio encoder with available labelled examples. However, this is challenging in low-shot scenarios, as the amount of annotations is limited compared to the model size. In this work, we introduce a Training-efficient (Treff) adapter to rapidly learn with a small set of examples while maintaining the capacity for zero-shot classification. First, we propose a crossattention linear model (CALM) to map a set of labelled examples and test audio to test labels. Second, we find initialising CALM as a cosine measurement improves our Treff adapter even without training. The Treff adapter outperforms metricbased methods in few-shot settings and yields competitive results to fully-supervised methods.
This study defines a new evaluation metric for audio tagging tasks to alleviate the limitation of the mean average precision (mAP) metric. The mAP metric treats different kinds of sound as independent classes without considering their relations. The proposed metric, ontology-aware mean average precision (OmAP), addresses the weaknesses of mAP by utilizing additional on-tology during evaluation. Specifically, we reweight the false positive events in the model prediction based on the AudioSet ontology graph distance to the target classes. The OmAP also provides insights into model performance by evaluating different coarse-grained levels in the ontology graph. We conduct a human assessment and show that OmAP is more consistent with human perception than mAP. We also propose an ontology-based loss function (OBCE) that reweights binary cross entropy (BCE) loss based on the ontology distance. Our experiment shows that OBCE can improve both mAP and OmAP metrics on the AudioSet tagging task.
—Convolutional neural networks (CNNs) have shown state-of-the-art performance in various applications. However, CNNs are resource-hungry due to their requirement of high computational complexity and memory storage. Recent efforts toward achieving computational and memory efficiency in CNNs involve filter pruning methods that eliminate some of the filters in CNNs based on the " importance " of the filters. The majority of existing filter pruning methods are either " active " , which use a dataset and generate feature maps to quantify filter importance, or " passive " , which compute filter importance using entry-wise norm of the filters or by measuring similarity among filters without involving data. However, the existing passive filter pruning methods eliminate relatively smaller norm filters or similar filters without considering the significance of the filters in producing the node output, resulting in degradation in the performance. To address this, we present a passive filter pruning method where the least significant filters with relatively smaller contribution in producing output are pruned away by incorporating the operator norm of the filters. The proposed pruning method results in better performance across various CNNs compared to that of the existing passive filter pruning methods. In comparison to the existing active filter pruning methods, the proposed pruning method is more efficient and achieves similar performance as well. The efficacy of the proposed pruning method is evaluated on audio scene classification and audio tagging tasks using various CNNs architecture such as VGGish, DCASE21 Net and PANNs. The proposed pruning method reduces number of computations and parameters of the unrpuned CNNs by at least 40% and 50% respectively, enhancing inference latency while maintaining similar performance as obtained using the unpruned CNNs.
Sounds carry an abundance of information about activities and events in our everyday environment, such as traffic noise, road works, music, or people talking. Recent machine learning methods, such as convolutional neural networks (CNNs), have been shown to be able to automatically recognize sound activities, a task known as audio tagging. One such method, pre-trained audio neural networks (PANNs), provides a neural network which has been pre-trained on over 500 sound classes from the publicly available AudioSet dataset, and can be used as a baseline or starting point for other tasks. However, the existing PANNs model has a high computational complexity and large storage requirement. This could limit the potential for deploying PANNs on resource-constrained devices, such as on-the-edge sound sensors, and could lead to high energy consumption if many such devices were deployed. In this paper, we reduce the computational complexity and memory requirement of the PANNs model by taking a pruning approach to eliminate redundant parameters from the PANNs model. The resulting Efficient PANNs (E-PANNs) model, which requires 36% less computations and 70% less memory, also slightly improves the sound recognition (audio tagging) performance. The code for the E-PANNs model has been released under an open source license.
Designing artificial intelligence (AI) using participatory design (PD) methods is becoming fundamental as AI increasingly augments everyday life. Well documented cases of machine bias, where AI systems informing hiring, loan approvals and prison sentencing have discriminated against people with certain demographics (i.e. gender, race), have highlighted the need to engage end-users in AI design. PD methods show promise in designing AI systems for maximum societal benefits as they allow users to collaborate with researchers and make decisions about how AI systems should be designed. This paper presents a modified virtual world café method, based on the World Café method, as a PD method to identify relevant design requirements for designing AI systems from the beginning of development. Specifically, we describe how this method was developed and used to create design requirements for sound sensing AI for the home with UK-based residents. Findings suggest that the method can be used as a PD method at the beginning of AI system development to define design requirements. The paper concludes with reflections on how the virtual world café method performs as a PD method for designing AI and how findings might be taken forward in future PD research for designing AI systems more generally.
This paper introduces briefly the history and growth of the Detection and Classification of Acoustic Scenes and Events (DCASE) challenge, workshop, research area and research community. Created in 2013 as a data evaluation challenge, DCASE has become a major research topic in the Audio and Acoustic Signal Processing area. Its success comes from a combination of factors: the challenge offers a large variety of tasks that are renewed each year; and the workshop offers a channel for dissemination of related work, engaging a young and dynamic community. At the same time, DCASE faces its own challenges, growing and expanding to different areas. One of the core principles of DCASE is open science and reproducibility: publicly available datasets, baseline systems, technical reports and workshop publications. While the DCASE challenge and workshop are independent of IEEE SPS, the challenge receives annual endorsement from the AASP TC, and the DCASE community contributes significantly to the ICASSP flagship conference and the success of SPS in many of its activities.
Generative models have shown significant achievements in audio generation tasks. However, existing models struggle with complex and detailed prompts, leading to potential performance degradation. We hypothesize that this problem stems from the simplicity and scarcity of the training data. This work aims to create a large-scale audio dataset with rich captions for improving audio generation models. We first develop an automated pipeline to generate detailed captions by transforming predicted visual captions, audio captions, and tagging labels into comprehensive descriptions using a Large Language Model (LLM). The resulting dataset, Sound-VECaps, comprises 1.66M high-quality audio-caption pairs with enriched details including audio event orders, occurred places and environment information. We then demonstrate that training the text-to-audio generation models with Sound-VECaps significantly improves the performance on complex prompts. Furthermore, we conduct ablation studies of the models on several downstream audio-language tasks, showing the potential of Sound-VECaps in advancing audio-text representation learning.Dataset and demos are available at https://yyua8222.github.io/Sound-VECaps-demo/.
Language-queried audio source separation (LASS) focuses on separating sounds using textual descriptions of the desired sources. Current methods mainly use discriminative approaches, such as time-frequency masking, to separate target sounds and minimize interference from other sources. However, these models face challenges when separating overlapping sound-tracks, which may lead to artifacts such as spectral holes or incomplete separation. Rectified flow matching (RFM), a generative model that establishes linear relations between the distribution of data and noise, offers superior theoretical properties and simplicity, but has not yet been explored in sound separation. In this work, we introduce FlowSep, a new generative model based on RFM for LASS tasks. FlowSep learns linear flow trajectories from noise to target source features within the variational autoencoder (VAE) latent space. During inference, the RFM-generated latent features are reconstructed into a mel-spectrogram via the pre-trained VAE decoder, followed by a pre-trained vocoder to synthesize the waveform. Trained on 1, 680 hours of audio data, FlowSep outperforms the state-of-the-art models across multiple benchmarks, as evaluated with subjective and objective metrics. Additionally, our results show that FlowSep surpasses a diffusion-based LASS model in both separation quality and inference efficiency, highlighting its strong potential for audio source separation tasks. Code, pre-trained models and demos can be found at: https://audio-agi.github.io/FlowSep_demo/.
Energy disaggregation, a.k.a. Non-Intrusive Load Monitoring, aims to separate the energy consumption of individual appliances from the readings of a mains power meter measuring the total energy consumption of, e.g., a whole house. Energy consumption of individual appliances can be useful in many applications, e.g., providing appliance-level feedback to the end users to help them understand their energy consumption and ultimately save energy. Recently, with the availability of large-scale energy consumption datasets, various neural network models such as convolutional neural networks and recurrent neural networks have been investigated to solve the energy disaggregation problem. Neural network models can learn complex patterns from large amounts of data and have been shown to outperform the traditional machine learning methods such as variants of hiddenMarkov models. However, current neural network methods for energy disaggregation are either computational expensive or are not capable of handling long-term dependencies. In this article, we investigate the application of the recently developed WaveNet models for the task of energy disaggregation. Based on a real-world energy dataset collected from 20 households over 2 years, we show that WaveNet models outperforms the state-of-the-art deep learning methods proposed in the literature for energy disaggregation in terms of both error measures and computational cost. On the basis of energy disaggregation, we then investigate the performance of two deep-learning based frameworks for the task of on/off detection which aims at estimating whether an appliance is in operation or not. The first framework obtains the on/off states of an appliance by binarising the predictions of a regression model trained for energy disaggregation, while the second framework obtains the on/off states of an appliance by directly training a binary classifier with binarised energy readings of the appliance serving as the target values. Based on the same dataset, we show that for the task of on/off detection the second framework, i.e., directly training a binary classifier, achieves better performance in terms of F1 score.
Large language models (LLMs) have significantly advanced audio processing through audio codecs that convert audio into discrete tokens, enabling the application of language modelling techniques to audio data. However, traditional codecs often operate at high bitrates or within narrow domains such as speech and lack the semantic clues required for efficient language modelling. Addressing these challenges, we introduce SemantiCodec, a novel codec designed to compress audio into fewer than a hundred tokens per second across diverse audio types, including speech, general sound, and music, without compromising quality. SemantiCodec features a dual-encoder architecture: a semantic encoder using a self-supervised pre-trained Audio Masked Autoencoder (AudioMAE), discretized using k-means clustering on extensive audio data, and an acoustic encoder to capture the remaining details. The semantic and acoustic encoder outputs are used to reconstruct audio via a diffusion-model-based decoder. SemantiCodec is presented in three variants with token rates of 25, 50, and 100 per second, supporting a range of ultra-low bit rates between 0.31 kbps and 1.40 kbps. Experimental results demonstrate that SemantiCodec significantly outperforms the state-of-the-art Descript codec on reconstruction quality. Our results also suggest that SemantiCodec contains significantly richer semantic information than all evaluated state-of-the-art audio codecs, even at significantly lower bitrates. Our code and demos are available at https://haoheliu.github.io/SemantiCodec/ .
This paper proposes a new design research approach for “Designing Artificial Intelligence (AI) for Home Wellbeing” as a new research field to collaboratively develop AI technologies for home wellbeing with stakeholders, such as end-users, and disciplinary experts, rather than leaving design solely to AI experts. Designing AI for home wellbeing is significant as AI applications progressively aBect home life. AI can identify trends and/or themes from live data, such as of images, video clips, sounds, or text, (Samoili et al. 2021) and is increasingly deployed in the home, such as recognising abnormal activity for security or monitoring occupants’ behavioural patterns for managing physical health (Guo et al. 2019). The home can play a significant part in supporting both psychological and physiological wellbeing through its central role in everyday living. For example, the home can satisfy fundamental physiological needs for wellbeing, such as shelter and security through its physicality, and psychological needs for wellbeing, such as social connection and intimacy, by facilitating communal eating and social interaction between occupants (Corrigan-Kavanagh and Escobar-Tello 2018). Given the strong influence of home on wellbeing, AI for the home should enhance, introduce, or support home wellbeing. However, there currently exists limited research on design methods and approaches for engaging stakeholders, including end-users, and diBerent disciplines in AI development and deployment (Hossain and Ahmed 2021; Delgado, Barocas, and Levy 2022), creating barriers for designing AI for home wellbeing. This paper calls for further scholarship in “Designing AI for Home Wellbeing” while presenting initial findings from research developing this new field.
Although audio generation shares commonalities across different types of audio, such as speech, music, and sound effects, designing models for each type requires careful consideration of specific objectives and biases that can significantly differ from those of other types. To bring us closer to a unified perspective of audio generation, this paper proposes a holistic framework that utilizes the same learning method for speech, music, and sound effect generation. Our framework utilizes a general representation of audio, called “language of audio” (LOA). Any audio can be translated into LOA based on AudioMAE, a self-supervised pre-trained representation learning model. In the generation process, we translate other modalities into LOA by using a GPT-2 model, and we perform self-supervised audio generation learning with a latent diffusion model conditioned on the LOA of audio in our training set. The proposed framework naturally brings advantages such as reusable self-supervised pretrained latent diffusion models. Experiments on the major benchmarks of text-to-audio, text-to-music, and text-to-speech with three AudioLDM 2 variants demonstrate competitive performance of the AudioLDM 2 framework against previous approaches.
Language-queried audio source separation (LASS) is a new paradigm for computational auditory scene analysis (CASA). LASS aims to separate a target sound from an audio mixture given a natural language query, which provides a natural and scalable interface for digital audio applications. Recent works on LASS, despite attaining promising separation performance on specific sources (e.g., musical instruments, limited classes of audio events), are unable to separate audio concepts in the open domain. In this work, we introduce AudioSep, a foundation model for open-domain audio source separation with natural language queries. We train AudioSep on large-scale multimodal datasets and extensively evaluate its capabilities on numerous tasks including audio event separation, musical instrument separation, and speech enhancement. AudioSep demonstrates strong separation performance and impressive zero-shot generalization ability using audio captions or text labels as queries, substantially outperforming previous audio-queried and language-queried sound separation models. For reproducibility of this work, we will release the source code, evaluation benchmark and pre-trained model at: https://github.com/Audio-AGI/AudioSep.
The goal of Unsupervised Anomaly Detection (UAD) is to detect anomalous signals under the condition that only non-anomalous (normal) data is available beforehand. In UAD under Domain-Shift Conditions (UAD-S), data is further exposed to contextual changes that are usually unknown beforehand. Motivated by the difficulties encountered in the UAD-S task presented at the 2021 edition of the Detection and Classification of Acoustic Scenes and Events (DCASE) challenge, we visually inspect Uniform Manifold Approximations and Projections (UMAPs) for log-STFT, log-mel and pretrained Look, Listen and Learn (L3) representations of the DCASE UAD-S dataset. In our exploratory investigation, we look for two qualities, Separability (SEP) and Discriminative Support (DSUP), and formulate several hypotheses that could facilitate diagnosis and developement of further representation and detection approaches. Particularly, we hypothesize that input length and pretraining may regulate a relevant tradeoff between SEP and DSUP. Our code as well as the resulting UMAPs and plots are publicly available. Accepted at the DCASE2021 Workshop
The advancement of audio-language (AL) multi-modal learning tasks has been significant in recent years, yet the limited size of existing audio-language datasets poses challenges for researchers due to the costly and time-consuming collection process. To address this data scarcity issue, we introduce WavCaps, the first large-scale weakly-labelled audio captioning dataset, comprising approximately 400k audio clips with paired captions. We sourced audio clips and their raw descriptions from web sources and a sound event detection dataset. However, the online-harvested raw descriptions are highly noisy and unsuitable for direct use in tasks such as automated audio captioning. To overcome this issue, we propose a three-stage processing pipeline for filtering noisy data and generating high-quality captions, where ChatGPT, a large language model, is leveraged to filter and transform raw descriptions automatically. We conduct a comprehensive analysis of the characteristics of WavCaps dataset and evaluate it on multiple downstream audio-language multimodal learning tasks. The systems trained on WavCaps outperform previous state-of-the-art (SOTA) models by a significant margin. Our aspiration is for the WavCaps dataset we have proposed to facilitate research in audio-language multimodal learning and demonstrate the potential of utilizing large language models (LLMs) to enhance academic research. Our dataset and codes are available at https://github.com/XinhaoMei/WavCaps.
Environment shifts and conflicts present significant challenges for learning-based sound event localization and detection (SELD) methods. SELD systems, when trained in particular acoustic settings, often show restricted generalization capabilities for diverse acoustic environments. Furthermore, it is notably costly to obtain annotated samples for spatial sound events. Deploying a SELD system in a new environment requires extensive time for re-training and fine-tuning. To overcome these challenges, we propose environment-adaptive Meta-SELD, designed for efficient adaptation to new environments using minimal data. Our method specifically utilizes computationally synthesized spatial data and employs Model-Agnostic MetaLearning (MAML) on a pre-trained, environment-independent model. The method then utilizes fast adaptation to unseen realworld environments using limited samples from the respective environments. Inspired by the Learning-to-Forget approach, we introduce the concept of selective memory as a strategy for resolving conflicts across environments. This approach involves selectively memorizing target-environment-relevant information and adapting to the new environments through the selective attenuation of model parameters. In addition, we introduce environment representations to characterize different acoustic settings, enhancing the adaptability of our attenuation approach to various environments. We evaluate our proposed method on the development set of the Sony-TAu Realistic Spatial Soundscapes 2023 (STARSS23) dataset and computationally synthesized scenes. Experimental results demonstrate the superior performance of the proposed method compared to conventional supervised learning methods, particularly in localization
Sound event detection (SED) methods typically rely on either strongly labelled data or weakly labelled data. As an alternative, sequentially labelled data (SLD) was proposed. In SLD, the events and the order of events in audio clips are known, without knowing the occurrence time of events. This paper proposes a connectionist temporal classification (CTC) based SED system that uses SLD instead of strongly labelled data, with a novel unsupervised clustering stage. Experiments on 41 classes of sound events show that the proposed two-stage method trained on SLD achieves performance comparable to the previous state-of-the-art SED system trained on strongly labelled data, and is far better than another state-of-the-art SED system trained on weakly labelled data, which indicates the effectiveness of the proposed two-stage method trained on SLD without any onset/offset time of sound events.
Universal sound separation (USS) is a task of separating mixtures of arbitrary sound sources. Typically, universal separation models are trained from scratch in a supervised manner, using labeled data. Self-supervised learning (SSL) is an emerging deep learning approach that leverages unlabeled data to obtain task-agnostic representations, which can benefit many downstream tasks. In this paper, we propose integrating a self-supervised pre-trained model, namely the audio masked autoencoder (A-MAE), into a universal sound separation system to enhance its separation performance. We employ two strategies to utilize SSL embeddings: freezing or updating the parameters of A-MAE during fine-tuning. The SSL embeddings are concate-nated with the short-time Fourier transform (STFT) to serve as input features for the separation model. We evaluate our methods on the AudioSet dataset, and the experimental results indicate that the proposed methods successfully enhance the separation performance of a state-of-the-art ResUNet-based USS model.
A method is proposed for instrument recognition in polyphonic music which combines two independent detector systems. A polyphonic musical instrument recognition system using a missing feature approach and an automatic music transcription system based on shift invariant probabilistic latent component analysis that includes instrument assignment. We propose a method to integrate the two systems by fusing the instrument contributions estimated by the first system onto the transcription system in the form of Dirichlet priors. Both systems, as well as the integrated system are evaluated using a dataset of continuous polyphonic music recordings. Detailed results that highlight a clear improvement in the performance of the integrated system are reported for different training conditions.
We outline a set of audio effects that use rhythmic analysis, in particular the extraction of beat and tempo information, to automatically synchronise temporal parameters to the input signal. We demonstrate that this analysis, known as beat-tracking, can be used to create adaptive parameters that adjust themselves according to changes in the properties of the input signal. We present common audio effects such as delay, tremolo and auto-wah augmented in this fashion and discuss their real-time implementation as Audio Unit plug-ins and objects for Max/MSP.
Audio source separation is a very challenging problem, and many different approaches have been proposed in attempts to solve it. We consider the problem of separating sources from two-channel instantaneous audio mixtures. One approach to this is to transform the mixtures into the time-frequency domain to obtain approximately disjoint representations of the sources, and then separate the sources using time-frequency masking. We focus on demixing the sources by binary masking, and assume that the mixing parameters are known. In this paper, we investigate the application of cosine packet (CP) trees as a foundation for the transform. We determine an appropriate transform by applying a computationally efficient best basis algorithm to a set of possible local cosine bases organised in a tree structure. We develop a heuristically motivated cost function which maximises the energy of the transform coefficients associated with a particular source. Finally, we evaluate objectively our proposed transform method by comparing it against fixed-basis transforms such as the short-time Fourier transform (STFT) and modified discrete cosine transform (MDCT). Evaluation results indicate that our proposed transform method outperforms MDCT and is competitive with the STFT, and informal listening tests suggest that the proposed method exhibits less objectionable noise than the STFT.
© 2015 Taylor & Francis. This paper presents a Bayesian probabilistic framework for real-time alignment of a recording or score with a live performance using an event-based approach. Multitrack audio files are processed using existing onset detection and harmonic analysis algorithms to create a representation of a musical performance as a sequence of time-stamped events. We propose the use of distributions for the position and relative speed which are sequentially updated in real-time according to Bayes’ theorem. We develop the methodology for this approach by describing its application in the case of matching a single MIDI track and then extend this to the case of multitrack recordings. An evaluation is presented that contrasts ourmultitrack alignment method with state-of-the-art alignment techniques.
One approach to Automatic Music Transcription (AMT) is to decompose a spectrogram with a dictionary matrix that contains a pitch-labelled note spectrum atom in each column. AMT performance is typically measured using frame-based comparison, while an alternative perspective is to use an event-based analysis. We have previously proposed an AMT system, based on the use of structured sparse representations. The method is described and experimental results are given, which are seen to be promising. An inspection of the graphical AMT output known as a piano roll may lead one to think that the performance may be slightly better than is suggested by the AMT metrics used. This leads us to perform an oracle analysis of the AMT system, with some interesting outcomes which may have implications for decomposition based AMT in general.
We are looking to use pitch estimators to provide an accurate high-resolution pitch track for resynthesis of musical audio. We found that current evaluation measures such as gross error rate (GER) are not suitable for algorithm selection. In this paper we examine the issues relating to evaluating pitch estimators and use these insights to improve performance of existing algorithms such as the well-known YIN pitch estimation algorithm.
This study concludes a tripartite investigation into the indirect visibility of the moving tongue in human speech as reflected in co-occurring changes of the facial surface. We were in particular interested in how the shared information is distributed over the range of contributing frequencies. In the current study we examine the degree to which tongue movements during speech can be reliably estimated from face motion using artificial neural networks. We simultaneously acquired data for both movement types; tongue movements were measured with Electromagnetic Articulography (EMA), face motion with a passive marker-based motion capture system. A multiresolution analysis using wavelets provided the desired decomposition into frequency subbands. In the two earlier studies of the project we established linear and non-linear relations between lingual and facial speech motions, as predicted and compatible with previous research in auditory-visual speech. The results of the current study using a Deep Neural Network (DNN) for prediction show that a substantive amount of variance can be recovered (between 13.9 and 33.2% dependent on the speaker and tongue sensor location). Importantly, however, the recovered variance values and the root mean squared error values of the Euclidean distances between the measured and the predicted tongue trajectories are in the range of the linear estimations of our earlier study.
Non-negative Matrix Factorisation (NMF) is a popular tool in which a ‘parts-based’ representation of a non-negative matrix is sought. NMF tends to produce sparse decompositions. This sparsity is a desirable property in many applications, and Sparse NMF (S-NMF) methods have been proposed to enhance this feature. Typically these enforce sparsity through use of a penalty term, and a `1 norm penalty term is often used. However an `1 penalty term may not be appropriate in a non-negative framework. In this paper the use of a `0 norm penalty for NMF is proposed, approximated using backwards elimination from an initial NNLS decomposition. Dictionary recovery experiments using overcomplete dictionaries show that this method outperforms both NMF and a state of the art S-NMF method, in particular when the dictionary to be learnt is dense.
Harmonic progression is one of the cornerstones of tonal music composition and is thereby essential to many musical styles and traditions. Previous studies have shown that musical genres and composers could be discriminated based on chord progressions modeled as chord n-grams. These studies were however conducted on small-scale datasets and using symbolic music transcriptions. In this work, we apply pattern mining techniques to over 200,000 chord progression sequences out of 1,000,000 extracted from the I Like Music (ILM) commercial music audio collection. The ILM collection spans 37 musical genres and includes pieces released between 1907 and 2013. We developed a single program multiple data parallel computing approach whereby audio feature extraction tasks are split up and run simultaneously on multiple cores. An audio-based chord recognition model (Vamp plugin Chordino) was used to extract the chord progressions from the ILM set. To keep low-weight feature sets, the chord data were stored using a compact binary format. We used the CM-SPADE algorithm, which performs a vertical mining of sequential patterns using co-occurence information, and which is fast and efficient enough to be applied to big data collections like the ILM set. In order to derive key-independent frequent patterns, the transition between chords are modeled by changes of qualities (e.g. major, minor, etc.) and root keys (e.g. fourth, fifth, etc.). The resulting key-independent chord progression patterns vary in length (from 2 to 16) and frequency (from 2 to 19,820) across genres. As illustrated by graphs generated to represent frequent 4-chord progressions, some patterns like circleof- fifths movements are well represented in most genres but in varying degrees. These large-scale results offer the opportunity to uncover similarities and discrepancies between sets of musical pieces and therefore to build classifiers for search and recommendation. They also support the empirical testing of music theory. It is however more difficult to derive new hypotheses from such dataset due to its size. This can be addressed by using pattern detection algorithms or suitable visualisation which we present in a companion study.
Acoustic Event Detection (AED) is an important task of machine listening which, in recent years, has been addressed using common machine learning methods like Non-negative Matrix Factorization (NMF) or deep learning. However, most of these approaches do not take into consideration the way that human auditory system detects salient sounds. In this work, we propose a method for AED using weakly labeled data that combines a Non-negative Matrix Factorization model with a salience model based on predictive coding in the form of Kalman filters. We show that models of auditory perception, particularly auditory salience, can be successfully incorporated into existing AED methods and improve their performance on rare event detection. We evaluate the method on the Task2 of DCASE2017 Challenge.
Acoustic Scene Classification (ASC) is a task that classifies a scene according to environmental acoustic signals. Audios collected from different cities and devices often exhibit biases in feature distributions, which may negatively impact ASC performance. Taking the city and device of the audio collection as two types of data domain, this paper attempts to disentangle the audio features of each domain to remove the related feature biases. A dual-alignment framework is proposed to generalize the ASC system on new devices or cities, by aligning boundaries across domains and decision boundaries within each domain. During the alignment, the maximum classifier discrepancy and gradient reversed layer are used for the feature disentanglement of scene, city and device, while four candidate domain classifiers are proposed to explore the optimal solution of feature disentanglement. To evaluate the dual-alignment framework, three experiments of biased ASC tasks are designed: 1) cross-city ASC in new cities; 2) cross-device ASC in new devices; 3) cross-city-device ASC in new cities and new devices. Results demonstrate the superiority of the proposed framework, showcasing performance improvements of 0.9%, 19.8%, and 10.7% on classification accuracy, respectively. The effectiveness of the proposed feature disentanglement approach is further evaluated in both biased and unbiased ASC problems, and the results demonstrate that better-disentangled audio features can lead to a more robust ASC system across different devices and cities. This paper advocates for the integration of feature disentanglement in ASC systems to achieve more reliable performance.
We present a new method for score-informed source separation, combining ideas from two previous approaches: one based on paramet- ric modeling of the score which constrains the NMF updating process, the other based on PLCA that uses synthesized scores as prior probability distributions. We experimentally show improved separation results using the BSS EVAL and PEASS toolkits, and discuss strengths and weaknesses compared with the previous PLCA-based approach.
The goal of Acoustic Scene Classification (ASC) is to recognise the environment in which an audio waveform has been recorded. Recently, deep neural networks have been applied to ASC and have achieved state-of-the-art performance. However, few works have investigated how to visualise and understand what a neural network has learnt from acoustic scenes. Previous work applied local pooling after each convolutional layer, therefore reduced the size of the feature maps. In this paper, we suggest that local pooling is not necessary, but the size of the receptive field is important. We apply atrous Convolutional Neural Networks (CNNs) with global attention pooling as the classification model. The internal feature maps of the attention model can be visualised and explained. On the Detection and Classification of Acoustic Scenes and Events (DCASE) 2018 dataset, our proposed method achieves an accuracy of 72.7 %, significantly outperforming the CNNs without dilation at 60.4 %. Furthermore, our results demonstrate that the learnt feature maps contain rich information on acoustic scenes in the time-frequency domain.
We consider the separation of sources when only one movable sensor is available to record a set of mixtures at distinct locations. A single mixture signal is acquired, which is firstly segmented. Then, based on the assumption that the underlying sources are temporally periodic, we align the resulting signals and form a measurement vector on which source separation can be performed. We demonstrate that this approach can successfully recover the original sources both when working with simulated data, and for a real problem of heart sound separation. © 2011 University of Zagreb.
—Audio pattern recognition is an important research topic in the machine learning area, and includes several tasks such as audio tagging, acoustic scene classification, music classification , speech emotion classification and sound event detection. Recently, neural networks have been applied to tackle audio pattern recognition problems. However, previous systems are built on specific datasets with limited durations. Recently, in computer vision and natural language processing, systems pretrained on large-scale datasets have generalized well to several tasks. However, there is limited research on pretraining systems on large-scale datasets for audio pattern recognition. In this paper, we propose pretrained audio neural networks (PANNs) trained on the large-scale AudioSet dataset. These PANNs are transferred to other audio related tasks. We investigate the performance and computational complexity of PANNs modeled by a variety of convolutional neural networks. We propose an architecture called Wavegram-Logmel-CNN using both log-mel spectrogram and waveform as input feature. Our best PANN system achieves a state-of-the-art mean average precision (mAP) of 0.439 on AudioSet tagging, outperforming the best previous system of 0.392. We transfer PANNs to six audio pattern recognition tasks, and demonstrate state-of-the-art performance in several of those tasks. We have released the source code and pretrained models of PANNs: https://github.com/qiuqiangkong/audioset_tagging_cnn.
For intelligent systems to make best use of the audio modality, it is important that they can recognize not just speech and music, which have been researched as specific tasks, but also general sounds in everyday environments. To stimulate research in this field we conducted a public research challenge: the IEEE Audio and Acoustic Signal Processing Technical Committee challenge on Detection and Classification of Acoustic Scenes and Events (DCASE). In this paper, we report on the state of the art in automatically classifying audio scenes, and automatically detecting and classifying audio events. We survey prior work as well as the state of the art represented by the submissions to the challenge from various research groups. We also provide detail on the organization of the challenge, so that our experience as challenge hosts may be useful to those organizing challenges in similar domains. We created new audio datasets and baseline systems for the challenge; these, as well as some submitted systems, are publicly available under open licenses, to serve as benchmarks for further research in general-purpose machine listening.
Techniques based on non-negative matrix factorization (NMF) have been successfully used to decompose a spectrogram of a music recording into a dictionary of templates and activations. While advanced NMF variants often yield robust signal models, there are usually some inaccuracies in the factorization since the underlying methods are not prepared for phase cancellations that occur when sounds with similar frequency are mixed. In this paper, we present a novel method that takes phase cancellations into account to refine dictionaries learned by NMF-based methods. Our approach exploits the fact that advanced NMF methods are often robust enough to provide information about how sound sources interact in a spectrogram, where they overlap, and thus where phase cancellations could occur. Using this information, the distances used in NMF are weighted entry-wise to attenuate the influence of regions with phase cancellations. Experiments on full-length, polyphonic piano recordings indicate that our method can be successfully used to refine NMF-based dictionaries.
For dictionary-based decompositions of certain types, it has been observed that there might be a link between sparsity in the dictionary and sparsity in the decomposition. Sparsity in the dictionary has also been associated with the derivation of fast and efficient dictionary learning algorithms. Therefore, in this paper we present a greedy adaptive dictionary learning algorithm that sets out to find sparse atoms for speech signals. The algorithm learns the dictionary atoms on data frames taken from a speech signal. It iteratively extracts the data frame with minimum sparsity index, and adds this to the dictionary matrix. The contribution of this atom to the data frames is then removed, and the process is repeated. The algorithm is found to yield a sparse signal decomposition, supporting the hypothesis of a link between sparsity in the decomposition and dictionary. The algorithm is applied to the problem of speech representation and speech denoising, and its performance is compared to other existing methods. The method is shown to find dictionary atoms that are sparser than their time-domain waveform, and also to result in a sparser speech representation. In the presence of noise, the algorithm is found to have similar performance to the well established principal component analysis. © 2011 IEEE.
Current research on audio source separation provides tools to estimate the signals contributed by different instruments in polyphonic music mixtures. Such tools can be already incorporated in music production and post-production workflows. In this paper, we describe recent experiments where audio source separation is applied to remixing and upmixing existing mono and stereo music content
It is now commonplace to capture and share images in photography as triggers for memory. In this paper we explore the possibility of using sound in the same sort of way, in a practice we call audiography. We report an initial design activity to create a system called Audio Memories comprising a ten second sound recorder, an intelligent archive for auto-classifying sound clips, and a multi-layered sound player for the social sharing of audio souvenirs around a table. The recorder and player components are essentially user experience probes that provide tangible interfaces for capturing and interacting with audio memory cues. We discuss our design decisions and process in creating these tools that harmonize user interaction and machine listening to evoke rich memories and conversations in an exploratory and open-ended way.
We introduce an information theoretic measure of statistical structure, called 'binding information', for sets of random variables, and compare it with several previously proposed measures including excess entropy, Bialek et al.'s predictive information, and the multi-information. We derive some of the properties of the binding information, particularly in relation to the multi-information, and show that, for finite sets of binary random variables, the processes which maximises binding information are the 'parity' processes. Finally we discuss some of the implications this has for the use of the binding information as a measure of complexity.
Deep learning techniques have been used recently to tackle the audio source separation problem. In this work, we propose to use deep fully convolutional denoising autoencoders (CDAEs) for monaural audio source separation. We use as many CDAEs as the number of sources to be separated from the mixed signal. Each CDAE is trained to separate one source and treats the other sources as background noise. The main idea is to allow each CDAE to learn suitable spectral-temporal filters and features to its corresponding source. Our experimental results show that CDAEs perform source separation slightly better than the deep feedforward neural networks (FNNs) even with fewer parameters than FNNs.
A method based on Deep Neural Networks (DNNs) and time-frequency masking has been recently developed for binaural audio source separation. In this method, the DNNs are used to predict the Direction Of Arrival (DOA) of the audio sources with respect to the listener which is then used to generate soft time-frequency masks for the recovery/estimation of the individual audio sources. In this paper, an algorithm called ‘dropout’ will be applied to the hidden layers, affecting the sparsity of hidden units activations: randomly selected neurons and their connections are dropped during the training phase, preventing feature co-adaptation. These methods are evaluated on binaural mixtures generated with Binaural Room Impulse Responses (BRIRs), accounting a certain level of room reverberation. The results show that the proposed DNNs system with randomly deleted neurons is able to achieve higher SDRs performances compared to the baseline method without the dropout algorithm.
Foley sound generation aims to synthesise the background sound for multimedia content. Previous models usually employ a large development set with labels as input (e.g., single numbers or one-hot vector). In this work, we propose a diffusion model based system for Foley sound generation with text conditions. To alleviate the data scarcity issue, our model is initially pre-trained with large-scale datasets and fine-tuned to this task via transfer learning using the contrastive language-audio pertaining (CLAP) technique. We have observed that the feature embedding extracted by the text encoder can significantly affect the performance of the generation model. Hence, we introduce a trainable layer after the encoder to improve the text embedding produced by the encoder. In addition, we further refine the generated waveform by generating multiple candidate audio clips simultaneously and selecting the best one, which is determined in terms of the similarity score between the embedding of the candidate clips and the embedding of the target text label. Using the proposed method, our system ranks \({1}^{st}\) among the systems submitted to DCASE Challenge 2023 Task 7. The results of the ablation studies illustrate that the proposed techniques significantly improve sound generation performance. The codes for implementing the proposed system are available online.
Foley sound presents the background sound for multimedia content and the generation of Foley sound involves computationally modelling sound effects with specialized techniques. In this work, we proposed a system for DCASE 2023 challenge task 7: Foley Sound Synthesis. The proposed system is based on AudioLDM, which is a diffusion-based text-to-audio generation model. To alleviate the data-hungry problem, the system first trained with large-scale datasets and then downstreamed into this DCASE task via transfer learning. Through experiments, we found out that the feature extracted by the encoder can significantly affect the performance of the generation model. Hence, we improve the results by leveraging the input label with related text embedding features obtained by a significant language model, i.e., contrastive language-audio pertaining (CLAP). In addition, we utilize a filtering strategy to further refine the output, i.e. by selecting the best results from the candidate clips generated in terms of the similarity score between the sound and target labels. The overall system achieves a Frechet audio distance (FAD) score of 4.765 on average among all seven different classes, substantially outperforming the baseline system which performs a FAD score of 9.7.
Sounds carry an abundance of information about activities and events in our everyday environment, such as traffic noise, road works, music, or people talking. Recent machine learning methods, such as convolutional neural networks (CNNs), have been shown to be able to automatically recognize sound activities, a task known as audio tagging. One such method, pre-trained audio neural networks (PANNs), provides a neural network which has been pre-trained on over 500 sound classes from the publicly available AudioSet dataset, and can be used as a baseline or starting point for other tasks. However, the existing PANNs model has a high computational complexity and large storage requirement. This could limit the potential for deploying PANNs on resource-constrained devices, such as on-the-edge sound sensors, and could lead to high energy consumption if many such devices were deployed. In this paper, we reduce the computational complexity and memory requirement of the PANNs model by taking a pruning approach to eliminate redundant parameters from the PANNs model. The resulting Efficient PANNs (E-PANNs) model, which requires 36% less computations and 70% less memory, also slightly improves the sound recognition (audio tagging) performance. The code for the E-PANNs model has been released under an open source license.
To assist with the development of intelligent mixing systems, it would be useful to be able to extract the loudness balance of sources in an existing musical mixture. The relative-to-mix loudness level of four instrument groups was predicted using the sources extracted by 12 audio source separation algorithms. The predictions were compared with the ground truth loudness data of the original unmixed stems obtained from a recent dataset involving 100 mixed songs. It was found that the best source separation system could predict the relative loudness of each instrument group with an average root-mean-square error of 1.2 LU, with superior performance obtained on vocals.
Analysing expressive timing in performed music can help machine to perform various perceptual tasks such as identifying performers and understand music structures in classical music. A hierarchical structure is commonly used for expressive timing analysis. This paper provides a statistical demonstration to support the use of hierarchical structure in expressive timing analysis by presenting two groups of model selection tests. The first model selection test uses expressive timing to determine the location of music structure boundaries. The second model selection test is matching a piece of performance with the same performer playing another given piece. Comparing the results of model selection tests, the preferred hierarchical structures in these two model selection tests are not the same. While determining music structure boundaries demands a hierarchical structure with more levels in the expressive timing analysis, a hierarchical structure with less levels helps identifying the dedicated performer in most cases.
Humans are able to identify a large number of environmental sounds and categorise them according to high-level semantic categories, e.g. urban sounds or music. They are also capable of generalising from past experience to new sounds when applying these categories. In this paper we report on the creation of a data set that is structured according to the top-level of a taxonomy derived from human judgements and the design of an associated machine learning challenge, in which strong generalisation abilities are required to be successful. We introduce a baseline classification system, a deep convolutional network, which showed strong performance with an average accuracy on the evaluation data of 80.8%. The result is discussed in the light of two alternative explanations: An unlikely accidental category bias in the sound recordings or a more plausible true acoustic grounding of the high-level categories.
Automatic species classification of birds from their sound is a computational tool of increasing importance in ecology, conservation monitoring and vocal communication studies. To make classification useful in practice, it is crucial to improve its accuracy while ensuring that it can run at big data scales. Many approaches use acoustic measures based on spectrogram-type data, such as the Mel-frequency cepstral coefficient (MFCC) features which represent a manually-designed summary of spectral information. However, recent work in machine learning has demonstrated that features learnt automatically from data can often outperform manually-designed feature transforms. Feature learning can be performed at large scale and "unsupervised", meaning it requires no manual data labelling, yet it can improve performance on "supervised" tasks such as classification. In this work we introduce a technique for feature learning from large volumes of bird sound recordings, inspired by techniques that have proven useful in other domains. We experimentally compare twelve different feature representations derived from the Mel spectrum (of which six use this technique), using four large and diverse databases of bird vocalisations, classified using a random forest classifier. We demonstrate that in our classification tasks, MFCCs can often lead to worse performance than the raw Mel spectral data from which they are derived. Conversely, we demonstrate that unsupervised feature learning provides a substantial boost over MFCCs and Mel spectra without adding computational complexity after the model has been trained. The boost is particularly notable for single-label classification tasks at large scale. The spectro-temporal activations learned through our procedure resemble spectro-temporal receptive fields calculated from avian primary auditory forebrain. However, for one of our datasets, which contains substantial audio data but few annotations, increased performance is not discernible. We study the interaction between dataset characteristics and choice of feature representation through further empirical analysis.
We describe our method for automatic bird species classification, which uses raw audio without segmentation and without using any auxiliary metadata. It successfully classifies among 501 bird categories, and was by far the highest scoring audio-only bird recognition algorithm submitted to BirdCLEF 2014. Our method uses unsupervised feature learning, a technique which learns regularities in spectro-temporal content without reference to the training labels, which helps a classifier to generalise to further content of the same type. Our strongest submission uses two layers of feature learning to capture regularities at two different time scales.
In this paper, we present a gated convolutional neural network and a temporal attention-based localization method for audio classification, which won the 1st place in the large-scale weakly supervised sound event detection task of Detection and Classification of Acoustic Scenes and Events (DCASE) 2017 challenge. The audio clips in this task, which are extracted from YouTube videos, are manually labelled with one or more audio tags, but without time stamps of the audio events, hence referred to as weakly labelled data. Two subtasks are defined in this challenge including audio tagging and sound event detection using this weakly labelled data. We propose a convolutional recurrent neural network (CRNN) with learnable gated linear units (GLUs) non-linearity applied on the log Mel spectrogram. In addition, we propose a temporal attention method along the frames to predict the locations of each audio event in a chunk from the weakly labelled data. The performances of our systems were ranked the 1st and the 2nd as a team in these two sub-tasks of DCASE 2017 challenge with F value 55.6% and Equal error 0.73, respectively.
Non-negative Matrix Factorisation (NMF) is a popular tool in musical signal processing. However, problems using this methodology in the context of Automatic Music Transcription (AMT) have been noted resulting in the proposal of supervised and constrained variants of NMF for this purpose. Group sparsity has previously been seen to be effective for AMT when used with stepwise methods. In this paper group sparsity is introduced to supervised NMF decompositions and a dictionary tuning approach to AMT is proposed based upon group sparse NMF using the β-divergence. Experimental results are given showing improved AMT results over the state-of-the-art NMF-based AMT system.
Radio production involves editing speech-based audio using tools that represent sound using simple waveforms. Semantic speech editing systems allow users to edit audio using an automatically generated transcript, which has the potential to improve the production workflow. To investigate this, we developed a semantic audio editor based on a pilot study. Through a contextual qualitative study of five professional radio producers at the BBC, we examined the existing radio production process and evaluated our semantic editor by using it to create programmes that were later broadcast. We observed that the participants in our study wrote detailed notes about their recordings and used annotation to mark which parts they wanted to use. They collaborated closely with the presenter of their programme to structure the contents and write narrative elements. Participants reported that they often work away from the office to avoid distractions, and print transcripts so they can work away from screens. They also emphasised that listening is an important part of production, to ensure high sound quality. We found that semantic speech editing with automated speech recognition can be used to improve the radio production workflow, but that annotation, collaboration, portability and listening were not well supported by current semantic speech editing systems. In this paper, we make recommendations on how future semantic speech editing systems can better support the requirements of radio production.
In this paper we present a model for beat-synchronous analysis of musical audio signals. Introducing a real-time beat tracking model with performance comparable to offline techniques, we discuss its application to the analysis of musical performances segmented by beat. We discuss the various design choices for beat-synchronous analysis and their implications for real-time implementations before presenting some beat-synchronous harmonic analysis examples. We make available our beat tracker and beat-synchronous analysis techniques as externals for Max/MSP.
Despite recent progress in text-to-audio (TTA) generation, we show that the state-of-the-art models, such as AudioLDM, trained on datasets with an imbalanced class distribution, such as AudioCaps, are biased in their generation performance. Specifically, they excel in generating common audio classes while underperforming in the rare ones, thus degrading the overall generation performance. We refer to this problem as long-tailed text-to-audio generation. To address this issue, we propose a simple retrieval-augmented approach for TTA models. Specifically, given an input text prompt, we first leverage a Contrastive Language Audio Pretraining (CLAP) model to retrieve relevant text-audio pairs. The features of the retrieved audio-text data are then used as additional conditions to guide the learning of TTA models. We enhance AudioLDM with our proposed approach and denote the resulting augmented system as Re-AudioLDM. On the AudioCaps dataset, Re-AudioLDM achieves a state-of-the-art Frechet Audio Distance (FAD) of 1.37, outperforming the existing approaches by a large margin. Furthermore, we show that Re-AudioLDM can generate realistic audio for complex scenes, rare audio classes, and even unseen audio types, indicating its potential in TTA tasks.
Polyphonic sound event localization and detection is not only detecting what sound events are happening but localizing corresponding sound sources. This series of tasks was first introduced in DCASE 2019 Task 3. In 2020, the sound event localization and detection task introduces additional challenges in moving sound sources and overlapping-event cases, which include two events of the same type with two different direction-of-arrival (DoA) angles. In this paper, a novel event-independent network for polyphonic sound event lo-calization and detection is proposed. Unlike the two-stage method we proposed in DCASE 2019 Task 3, this new network is fully end-to-end. Inputs to the network are first-order Ambisonics (FOA) time-domain signals, which are then fed into a 1-D convolutional layer to extract acoustic features. The network is then split into two parallel branches. The first branch is for sound event detection (SED), and the second branch is for DoA estimation. There are three types of predictions from the network, SED predictions, DoA predictions , and event activity detection (EAD) predictions that are used to combine the SED and DoA features for onset and offset estimation. All of these predictions have the format of two tracks indicating that there are at most two overlapping events. Within each track, there could be at most one event happening. This architecture introduces a problem of track permutation. To address this problem, a frame-level permutation invariant training method is used. Experimental results show that the proposed method can detect polyphonic sound events and their corresponding DoAs. Its performance on the Task 3 dataset is greatly increased as compared with that of the baseline method. Index Terms— Sound event localization and detection, direction of arrival, event-independent, permutation invariant training.
This paper describes work aimed at creating an efficient, real-time, robust and high performance chord recognition system for use on a single instrument in a live performance context. An improved chroma calculation method is combined with a classification technique based on masking out expected note positions in the chromagram and minimising the residual energy. We demonstrate that our approach can be used to classify a wide range of chords, in real-time, on a frame by frame basis. We present these analysis techniques as externals for Max/MSP. © July 2009- All copyright remains with the individual authors.
Music remixing is difficult when the original multitrack recording is not available. One solution is to estimate the elements of a mixture using source separation. However, existing techniques suffer from imperfect separation and perceptible artifacts on single separated sources. To investigate their influence on a remix, five state-of-the-art source separation algorithms were used to remix six songs by increasing the level of the vocals. A listening test was conducted to assess the remixes in terms of loudness balance and sound quality. The results show that some source separation algorithms are able to increase the level of the vocals by up to 6 dB at the cost of introducing a small but perceptible degradation in sound quality.
Non-negative Matrix Factorization (NMF) is a well established tool for audio analysis. However, it is not well suited for learning on weakly labeled data, i.e. data where the exact timestamp of the sound of interest is not known. In this paper we propose a novel extension to NMF, that allows it to extract meaningful representations from weakly labeled audio data. Recently, a constraint on the activation matrix was proposed to adapt for learning on weak labels. To further improve the method we propose to add an orthogonality regularizer of the dictionary in the cost function of NMF. In that way we obtain appropriate dictionaries for the sounds of interest and background sounds from weakly labeled data. We demonstrate that the proposed Orthogonality-Regularized Masked NMF (ORM-NMF) can be used for Audio Event Detection of rare events and evaluate the method on the development data from Task2 of DCASE2017 Challenge.
The sources separated by most single channel audio source separation techniques are usually distorted and each separated source contains residual signals from the other sources. To tackle this problem, we propose to enhance the separated sources by decreasing the distortion and interference between the separated sources using deep neural networks (DNNs). Two different DNNs are used in this work. The first DNN is used to separate the sources from the mixed signal. The second DNN is used to enhance the ...
In this paper we present the supervised iterative projections and rotations (S-IPR) algorithm, a method to optimise a set of discriminative subspaces for supervised classification. We show how the proposed technique is based on our previous unsupervised iterative projections and rotations (IPR) algorithm for incoherent dictionary learning, and how projecting the features onto the learned sub-spaces can be employed as a feature transform algorithm in the context of classification. Numerical experiments on the FISHERIRIS and on the USPS datasets, and a comparison with the PCA and LDA methods for feature transform demonstrates the value of the proposed technique and its potential as a tool for machine learning. © 2013 IEEE.
The recent (2013) bird species recognition challenges organised by the SABIOD project attracted some strong performances from automatic classifiers applied to short audio excerpts from passive acoustic monitoring stations. Can such strong results be achieved for dawn chorus field recordings in audio archives? The question is important because archives (such as the British Library Sound Archive) hold thousands such recordings, covering many decades and many countries, but they are mostly unlabelled. Automatic labelling holds the potential to unlock their value to ecological studies. Audio in such archives is quite different from passive acoustic monitoring data: importantly, the recording conditions vary randomly (and are usually unknown), making the scenario a ”cross-condition” rather than ”single-condition” train/test task. Dawn chorus recordings are generally long, and the annotations often indicate which birds are in a 20-minute recording but not within which 5-second segments they are active. Further, the amount of annotation available is very small. We report on experiments to evaluate a variety of classifier configurations for automatic multilabel species annotation in dawn chorus archive recordings. The audio data is an order of magnitude larger than the SABIOD challenges, but the ground-truth data is an order of magnitude smaller. We report some surprising findings, including clear variation in the bene- fits of some analysis choices (audio features, pooling techniques noise-robustness techniques) as we move to handle the specific multi-condition case relevant for audio archives.
Clipping, or saturation, is a common nonlinear distortion in signal processing. Recently, declipping techniques have been proposed based on sparse decomposition of the clipped signals on a fixed dictionary, with additional constraints on the amplitude of the clipped samples. Here we propose a dictionary learning approach, where the dictionary is directly learned from the clipped measurements. We propose a soft-consistency metric that minimizes the distance to a convex feasibility set, and takes into account our knowledge about the clipping process. We then propose a gradient descent-based dictionary learning algorithm that minimizes the proposed metric, and is thus consistent with the clipping measurement. Experiments show that the proposed algorithm outperforms other dictionary learning algorithms applied to clipped signals. We also show that learning the dictionary directly from the clipped signals outperforms consistent sparse coding with a fixed dictionary.
Segmenting note objects in a real time context is useful for live performances, audio broadcasting, or object-based coding. This temporal segmentation relies upon the correct detection of onsets and offsets of musical notes, an area of much research over recent years. However the low-latency requirements of real-time systems impose new, tight constraints on this process. In this paper, we present a system for the segmentation of note objects with very short delays, using recent developments in onset detection, specially modi ed to work in a real-time context. A portable and open C implementation is presented.
Sparse coding and dictionary learning are popular techniques for linear inverse problems such as denoising or inpainting. However in many cases, the measurement process is nonlinear, for example for clipped, quantized or 1-bit measurements. These problems have often been addressed by solving constrained sparse coding problems, which can be difficult to solve, and assuming that the sparsifying dictionary is known and fixed. Here we propose a simple and unified framework to deal with nonlinear measurements. We propose a cost function that minimizes the distance to a convex feasibility set, which models our knowledge about the nonlinear measurement. This provides an unconstrained, convex, and differentiable cost function that is simple to optimize, and generalizes the linear least squares cost commonly used in sparse coding. We then propose proximal based sparse coding and dictionary learning algorithms, that are able to learn directly from nonlinearly corrupted signals. We show how the proposed framework and algorithms can be applied to clipped, quantized and 1-bit data.
Text-to-audio (TTA) system has recently gained attention for its ability to synthesize general audio based on text descriptions. However, previous studies in TTA have limited generation quality with high computational costs. In this study, we propose AudioLDM, a TTA system that is built on a latent space to learn the continuous audio representations from contrastive language-audio pretraining (CLAP) latents. The pretrained CLAP models enable us to train LDMs with audio embedding while providing text embedding as a condition during sampling. By learning the latent representations of audio signals and their compositions without modeling the cross-modal relationship, AudioLDM is advantageous in both generation quality and computational efficiency. Trained on AudioCaps with a single GPU, AudioLDM achieves state-of-the-art TTA performance measured by both objective and subjective metrics (e.g., frechet distance). Moreover, AudioLDM is the first TTA system that enables various text-guided audio manipulations (e.g., style transfer) in a zero-shot fashion. Our implementation and demos are available at https://audioldm.github.io.
Audio tagging aims to assign one or several tags to an audio clip. Most of the datasets are weakly labelled, which means only the tags of the clip are known, without knowing the occurrence time of the tags. The labeling of an audio clip is often based on the audio events in the clip and no event level label is provided to the user. Previous works have used the bag of frames model assume the tags occur all the time, which is not the case in practice. We propose a joint detection-classification (JDC) model to detect and classify the audio clip simultaneously. The JDC model has the ability to attend to informative and ignore uninformative sounds. Then only informative regions are used for classification. Experimental results on the “CHiME Home” dataset show that the JDC model reduces the equal error rate (EER) from 19.0% to 16.9%. More interestingly, the audio event detector is trained successfully without needing the event level label.
Bird audio detection (BAD) aims to detect whether there is a bird call in an audio recording or not. One difficulty of this task is that the bird sound datasets are weakly labelled, that is only the presence or absence of a bird in a recording is known, without knowing when the birds call. We propose to apply joint detection and classification (JDC) model on the weakly labelled data (WLD) to detect and classify an audio clip at the same time. First, we apply VGG like convolutional neural network (CNN) on mel spectrogram as baseline. Then we propose a JDC-CNN model with VGG as a classifier and CNN as a detector. We report the denoising method including optimally-modified log-spectral amplitude (OM-LSA), median filter and spectral spectrogram will worse the classification accuracy on the contrary to previous work. JDC-CNN can predict the time stamps of the events from weakly labelled data, so is able to do sound event detection from WLD. We obtained area under curve (AUC) of 95.70% on the development data and 81.36% on the unseen evaluation data, which is nearly comparable to the baseline CNN model.
Sound event localization and detection (SELD) is a joint task of sound event detection and direction-of-arrival estimation. In DCASE 2022 Task 3, types of data transform from computationally generated spatial recordings to recordings of real-sound scenes. Our system submitted to the DCASE 2022 Task 3 is based on our previous proposed Event-Independent Network V2 (EINV2) with a novel data augmentation method. Our method employs EINV2 with a track-wise output format, permutation-invariant training, and a soft parameter-sharing strategy, to detect different sound events of the same class but in different locations. The Conformer structure is used for extending EINV2 to learn local and global features. A data augmentation method, which contains several data augmentation chains composed of stochastic combinations of several different data augmentation operations, is utilized to generalize the model. To mitigate the lack of real-scene recordings in the development dataset and the presence of sound events being unbalanced, we exploit FSD50K, AudioSet, and TAU Spatial Room Impulse Response Database (TAU-SRIR DB) to generate simulated datasets for training. We present results on the validation set of Sony-TAu Realistic Spatial Soundscapes 2022 (STARSS22) in detail. Experimental results indicate that the ability to generalize to different environments and unbalanced performance among different classes are two main challenges. We evaluate our proposed method in Task 3 of the DCASE 2022 challenge and obtain the second rank in the teams ranking.
We propose the audio inpainting framework that recovers portions of audio data distorted due to impairments such as impulsive noise, clipping, and packet loss. In this framework, the distorted data are treated as missing and their location is assumed to be known. The signal is decomposed into overlapping time-domain frames and the restoration problem is then formulated as an inverse problem per audio frame. Sparse representation modeling is employed per frame, and each inverse problem is solved using the Orthogonal Matching Pursuit algorithm together with a discrete cosine or a Gabor dictionary. The Signal-to-Noise Ratio performance of this algorithm is shown to be comparable or better than state-of-the-art methods when blocks of samples of variable durations are missing. We also demonstrate that the size of the block of missing samples, rather than the overall number of missing samples, is a crucial parameter for high quality signal restoration. We further introduce a constrained Matching Pursuit approach for the special case of audio declipping that exploits the sign pattern of clipped audio samples and their maximal absolute value, as well as allowing the user to specify the maximum amplitude of the signal. This approach is shown to outperform state-of-the-art and commercially available methods for audio declipping in terms of Signal-to-Noise Ratio
This article deals with learning dictionaries for sparse approximation whose atoms are both adapted to a training set of signals and mutually incoherent. To meet this objective, we employ a dictionary learning scheme consisting of sparse approximation followed by dictionary update and we add to the latter a decorrelation step in order to reach a target mutual coherence level. This step is accomplished by an iterative projection method complemented by a rotation of the dictionary. Experiments on musical audio data and a comparison with the method of optimal coherence-constrained directions (mocod) and the incoherent k-svd (ink-svd) illustrate that the proposed algorithm can learn dictionaries that exhibit a low mutual coherence while providing a sparse approximation with better signal-to-noise ratio (snr) than the benchmark techniques
In this paper, we introduce the task of language-queried audio source separation (LASS), which aims to separate a target source from an audio mixture based on a natural language query of the target source (e.g., “a man tells a joke followed by people laughing”). A unique challenge in LASS is associated with the complexity of natural language description and its relation with the audio sources. To address this issue, we proposed LASSNet, an end-to-end neural network that is learned to jointly process acoustic and linguistic information, and separate the target source that is consistent with the language query from an audio mixture. We evaluate the performance of our proposed system with a dataset created from the AudioCaps dataset. Experimental results show that LASS-Net achieves considerable improvements over baseline methods. Furthermore, we observe that LASS-Net achieves promising generalization results when using diverse human-annotated descriptions as queries, indicating its potential use in real-world scenarios. The separated audio samples and source code are available at https://liuxubo717.github.io/LASS-demopage.
Assuming that a set of source signals is sparsely representable in a given dictionary, we show how their sparse recovery fails whenever we can only measure a convolved observation of them. Starting from this motivation, we develop a block coordinate descent method which aims to learn a convolved dictionary and provide a sparse representation of the observed signals with small residual norm. We compare the proposed approach to the K-SVD dictionary learning algorithm and show through numerical experiment on synthetic signals that, provided some conditions on the problem data, our technique converges in a fixed number of iterations to a sparse representation with smaller residual norm.
Neuroevolution techniques combine genetic algorithms with artificial neural networks, some of them evolving network topology along with the network weights. One of these latter techniques is the NeuroEvolution of Augmenting Topologies (NEAT) algorithm. For this pilot study we devised an extended variant (joint NEAT, J-NEAT), introducing dynamic cooperative co-evolution, and applied it to sound event detection in real life audio (Task 3) in the DCASE 2017 challenge. Our research question was whether small networks could be evolved that would be able to compete with the much larger networks now typical for classification and detection tasks. We used the wavelet-based deep scattering transform and k-means clustering across the resulting scales (not across samples) to provide J-NEAT with a compact representation of the acoustic input. The results show that for the development data set J-NEAT was capable of evolving small networks that match the performance of the baseline system in terms of the segment-based error metrics, while exhibiting a substantially better event-related error rate. In the challenge, J-NEAT took first place overall according to the F1 error metric with an F1 of 44:9% and achieved rank 15 out of 34 on the ER error metric with a value of 0:891. We discuss the question of evolving versus learning for supervised tasks.
In this paper, we propose two methods for polyphonic Acoustic Event Detection (AED) in real life environments. The first method is based on Coupled Sparse Non-negative Matrix Factorization (CSNMF) of spectral representations and their corresponding class activity annotations. The second method is based on Multi-class Random Forest (MRF) classification of time-frequency patches. We compare the performance of the two methods on a recently published dataset TUT Sound Events 2016 containing data from home and residential area environments. Both methods show comparable performance to the baseline system proposed for DCASE 2016 Challenge on the development dataset with MRF outperforming the baseline on the evaluation dataset.
Audio source separation is a difficult machine learning problem and performance is measured by comparing extracted signals with the component source signals. However, if separation is motivated by the ultimate goal of re-mixing then complete separation is not necessary and hence separation difficulty and separation quality are dependent on the nature of the re-mix. Here, we use a convolutional deep neural network (DNN), trained to estimate 'ideal' binary masks for separating voice from music, to perform re-mixing of the vocal balance by operating directly on the individual magnitude components of the musical mixture spectrogram. Our results demonstrate that small changes in vocal gain may be applied with very little distortion to the ultimate re-mix. Our method may be useful for re-mixing existing mixes.
We introduce `PaperClip' - a novel digital pen interface for semantic editing of speech recordings for radio production. We explain how we designed and developed our system, then present the results of a contextual qualitative user study of eight professional radio producers that compared editing using PaperClip to a screen-based interface and normal paper. As in many other paper-versus-screen studies, we found no overall preferences but rather advantages and disadvantages of both in different contexts. We discuss these relative benefits and make recommendations for future development.
Clipping is a common type of distortion in which the amplitude of a signal is truncated if it exceeds a certain threshold. Sparse representation has underpinned several algorithms developed recently for reconstruction of the original signal from clipped observations. However, these declipping algorithms are often built on a synthesis model, where the signal is represented by a dictionary weighted by sparse coding coefficients. In contrast to these works, we propose a sparse analysis-model-based declipping (SAD) method, where the declipping model is formulated on an analysis (i.e. transform) dictionary, and additional constraints characterizing the clipping process. The analysis dictionary is updated using the Analysis SimCO algorithm, and the signal is recovered by using a least-squares based method or a projected gradient descent method, incorporating the observable signal set. Numerical experiments on speech and music are used to demonstrate improved performance in signal to distortion ratio (SDR) compared to recent state-of-the-art methods including A-SPADE and ConsDL.
In this article we present the supervised iterative projections and rotations (s-ipr) algorithm, a method for learning discriminative incoherent subspaces from data. We derive s-ipr as a supervised extension of our previously proposed iterative projections and rotations (ipr) algorithm for incoherent dictionary learning, and we employ it to learn incoherent sub-spaces that model signals belonging to different classes. We test our method as a feature transform for supervised classification, first by visualising transformed features from a synthetic dataset and from the ‘iris’ dataset, then by using the resulting features in a classification experiment.
Digital music libraries and collections are growing quickly and are increasingly made available for research. We argue that the use of large data collections will enable a better understanding of music performance and music in general, which will benefit areas such as music search and recommendation, music archiving and indexing, music production and education. However, to achieve these goals it is necessary to develop new musicological research methods, to create and adapt the necessary technological infrastructure, and to find ways of working with legal limitations. Most of the necessary basic technologies exist, but they need to be brought together and applied to musicology. We aim to address these challenges in the Digital Music Lab project, and we feel that with suitable methods and technology Big Music Data can provide new opportunities to musicology.
The audio spectrogram is a time-frequency representation that has been widely used for audio classification. One of the key attributes of the audio spectrogram is the temporal resolution, which depends on the hop size used in the Short-Time Fourier Transform (STFT). Previous works generally assume the hop size should be a constant value (e.g., 10 ms). However, a fixed temporal resolution is not always optimal for different types of sound. The temporal resolution affects not only classification accuracy but also computational cost. This paper proposes a novel method, DiffRes, that enables differentiable temporal resolution modeling for audio classification. Given a spectrogram calculated with a fixed hop size, DiffRes merges non-essential time frames while preserving important frames. DiffRes acts as a "drop-in" module between an audio spectrogram and a classifier and can be jointly optimized with the classification task. We evaluate DiffRes on five audio classification tasks, using mel-spectrograms as the acoustic features, followed by off-the-shelf classifier backbones. Compared with previous methods using the fixed temporal resolution, the DiffRes-based method can achieve the equivalent or better classification accuracy with at least 25% computational cost reduction. We further show that DiffRes can improve classification accuracy by increasing the temporal resolution of input acoustic features, without adding to the computational cost.
Polyphonic music transcription is a challenging problem, requiring the identification of a collection of latent pitches which can explain an observed music signal. Many state-of-the-art methods are based on the Non-negative Matrix Factorization (NMF) framework, which itself can be cast as a latent variable model. However, the basic NMF algorithm fails to consider many important aspects of music signals such as lowrank or hierarchical structure and temporal continuity. In this work we propose a probabilistic model to address some of the shortcomings of NMF. Probabilistic Latent Component Analysis (PLCA) provides a probabilistic interpretation of NMF and has been widely applied to problems in audio signal processing. Based on PLCA, we propose an algorithm which represents signals using a collection of low-rank dictionaries built from a base pitch dictionary. This allows each dictionary to specialize to a given chord or interval template which will be used to represent collections of similar frames. Experiments on a standard music transcription data set show that our method can successfully decompose signals into a hierarchical and smooth structure, improving the quality of the transcription.
We present a method to develop low-complexity convolu-tional neural networks (CNNs) for acoustic scene classification (ASC). The large size and high computational complexity of typical CNNs is a bottleneck for their deployment on resource-constrained devices. We propose a passive filter pruning framework , where a few convolutional filters from the CNNs are eliminated to yield compressed CNNs. Our hypothesis is that similar filters produce similar responses and give redundant information allowing such filters to be eliminated from the network. To identify similar filters, a cosine distance based greedy algorithm is proposed. A fine-tuning process is then performed to regain much of the performance lost due to filter elimination. To perform efficient fine-tuning, we analyze how the performance varies as the number of fine-tuning training examples changes. An experimental evaluation of the proposed framework is performed on the publicly available DCASE 2021 Task 1A base-line network trained for ASC. The proposed method is simple, reduces computations per inference by 27%, with 25% fewer parameters, with less than 1% drop in accuracy.
Recently, Convolutional Neural Networks (CNNs) have been successfully applied to ASC. However, the data distributions of the audio signals recorded with multiple devices are different. There has been little research on the training of robust neural networks on acoustic scene datasets recorded with multiple devices, and on explaining the operation of the internal layers of the neural networks. In this article, we focus on training and explaining device-robust CNNs on multi-device acoustic scene data. We propose conditional atrous CNNs with attention for multi-device ASC. Our proposed system contains an ASC branch and a device classification branch, both modelled by CNNs. We visualise and analyse the intermediate layers of the atrous CNNs. A time-frequency attention mechanism is employed to analyse the contribution of each time-frequency bin of the feature maps in the CNNs. On the Detection and Classification of Acoustic Scenes and Events (DCASE) 2018 ASC dataset, recorded with three devices, our proposed model performs significantly better than CNNs trained on single-device data.
A method based on local spectral features and missing feature techniques is proposed for the recognition of harmonic sounds in mixture signals. A mask estimation algorithm is proposed for identifying spectral regions that contain reliable information for each sound source and then bounded marginalization is employed to treat the feature vector elements that are determined as unreliable. The proposed method is tested on musical instrument sounds due to the extensive availability of data but it can be applied on other sounds (i.e. animal sounds, environmental sounds), whenever these are harmonic. In simulations the proposed method clearly outperformed a baseline method for mixture signals.
Combining different models is a common strategy to build a good audio source separation system. In this work, we combine two powerful deep neural networks for audio single channel source separation (SCSS). Namely, we combine fully convolutional neural networks (FCNs) and recurrent neural networks, specifically, bidirectional long short-term memory recurrent neural networks (BLSTMs). FCNs are good at extracting useful features from the audio data and BLSTMs are good at modeling the temporal structure of the audio signals. Our experimental results show that combining FCNs and BLSTMs achieves better separation performance than using each model individually.
Harmonie sinusoidal models are a fundamental tool for audio signal analysis. Bayesian harmonic models guarantee a good resynthesis quality and allow joint use of learnt parameter priors and auditory motivated distortion measures. However inference algorithms based on Monte Carlo sampling are rather slow for realistic data. In this paper, we investigate fast inference algorithms based on approximate factorization of the joint posterior into a product of independent distributions on small subsets of parameters. We discuss the conditions under which these approximations hold true and evaluate their performance experimentally. We suggest how they could be used together with Monte Carlo algorithms for a faster sampling-based inference. © 2006 IEEE.
Given a musical audio recording, the goal of music transcription is to determine a score-like representation of the piece underlying the recording. Most current transcription methods employ variants of non-negative matrix factorization (NMF), which often fails to robustly model instruments producing non-stationary sounds. Using entire time-frequency patterns to represent sounds, non-negative matrix deconvolution (NMD) can capture certain types of nonstationary behavior but is only applicable if all sounds have the same length. In this paper, we present a novel method that combines the non-stationarity modeling capabilities available with NMD with the variable note lengths possible with NMF. Identifying frames in NMD patterns with states in a dynamical system, our method iteratively generates sound-object candidates separately for each pitch, which are then combined in a global optimization. We demonstrate the transcription capabilities of our method using piano pieces assuming the availability of single note recordings as training data.
In this paper we discuss how software development can be improved in the audio and music research community by implementing tighter and more effective development feedback loops. We suggest first that researchers in an academic environment can benefit from the straightforward application of peer code review, even for ad-hoc research software; and second, that researchers should adopt automated software unit testing from the start of research projects. We discuss and illustrate how to adopt both code reviews and unit testing in a research environment. Finally, we observe that the use of a software version control system provides support for the foundations of both code reviews and automated unit tests. We therefore also propose that researchers should use version control with all their projects from the earliest stage.
We consider the task of inferring associations between two differently-distributed and unlabelled sets of timbre data. This arises in applications such as concatenative synthesis/ audio mosaicing in which one audio recording is used to control sound synthesis through concatenating fragments of an unrelated source recording. Timbre is a multidimensional attribute with interactions between dimensions, so it is non-trivial to design a search process which makes best use of the timbral variety available in the source recording. We must be able to map from control signals whose timbre features have different distributions from the source material, yet labelling large collections of timbral sounds is often impractical, so we seek an unsupervised technique which can infer relationships between distributions. We present a regression tree technique which learns associations between two unlabelled multidimensional distributions, and apply the technique to a simple timbral concatenative synthesis system. We demonstrate numerically that the mapping makes better use of the source material than a nearest-neighbour search. © 2010 Dan Stowell et al.
Sound event detection (SED) is a task to detect sound events in an audio recording. One challenge of the SED task is that many datasets such as the Detection and Classification of Acoustic Scenes and Events (DCASE) datasets are weakly labelled. That is, there are only audio tags for each audio clip without the onset and offset times of sound events. We compare segment-wise and clip-wise training for SED that is lacking in previous works. We propose a convolutional neural network transformer (CNN-Transfomer) for audio tagging and SED, and show that CNN-Transformer performs similarly to a convolutional recurrent neural network (CRNN). Another challenge of SED is that thresholds are required for detecting sound events. Previous works set thresholds empirically, and are not an optimal approaches. To solve this problem, we propose an automatic threshold optimization method. The first stage is to optimize the system with respect to metrics that do not depend on thresholds, such as mean average precision (mAP). The second stage is to optimize the thresholds with respect to metrics that depends on those thresholds. Our proposed automatic threshold optimization system achieves a state-of-the-art audio tagging F1 of 0.646, outperforming that without threshold optimization of 0.629, and a sound event detection F1 of 0.584, outperforming that without threshold optimization of 0.564.
Public evaluation campaigns and datasets promote active development in target research areas, allowing direct comparison of algorithms. The second edition of the challenge on Detection and Classification of Acoustic Scenes and Events (DCASE 2016) has offered such an opportunity for development of state-of-the-art methods, and succeeded in drawing together a large number of participants from academic and industrial backgrounds. In this paper, we report on the tasks and outcomes of the DCASE 2016 challenge. The challenge comprised four tasks: acoustic scene classification, sound event detection in synthetic audio, sound event detection in real-life audio, and domestic audio tagging. We present in detail each task and analyse the submitted systems in terms of design and performance. We observe the emergence of deep learning as the most popular classification method, replacing the traditional approaches based on Gaussian mixture models and support vector machines. By contrast, feature representations have not changed substantially throughout the years, as mel frequency-based representations predominate in all tasks. The datasets created for and used in DCASE 2016 are publicly available and are a valuable resource for further research.
Convolutional neural networks (CNNs) are popular in high-performing solutions to many real-world problems, such as audio classification. CNNs have many parameters and filters, with some having a larger impact on the performance than others. This means that networks may contain many unnecessary filters, increasing a CNN's computation and memory requirements while providing limited performance benefits. To make CNNs more efficient, we propose a pruning framework that eliminates filters with the highest " commonality ". We measure this commonality using the graph-theoretic concept of centrality. We hypothesise that a filter with a high centrality should be eliminated as it represents commonality and can be replaced by other filters without affecting the performance of a network much. An experimental evaluation of the proposed framework is performed on acoustic scene classification and audio tagging. On the DCASE 2021 Task 1A baseline network, our proposed method reduces computations per inference by 71% with 50% fewer parameters with less than a two percentage point drop in accuracy compared to the original network. For large-scale CNNs such as PANNs designed for audio tagging, our method reduces computations per inference by 24% with 41% fewer parameters at a slight improvement in performance.
Audio source separation aims to extract individual sources from mixtures of multiple sound sources. Many techniques have been developed such as independent compo- nent analysis, computational auditory scene analysis, and non-negative matrix factorisa- tion. A method based on Deep Neural Networks (DNNs) and time-frequency (T-F) mask- ing has been recently developed for binaural audio source separation. In this method, the DNNs are used to predict the Direction Of Arrival (DOA) of the audio sources with respect to the listener which is then used to generate soft T-F masks for the recovery/estimation of the individual audio sources.
This chapter discusses the role of information theory for analysis of neural networks using differential geometric ideas. Information theory is useful for understanding preprocessing, in terms of predictive coding in the retina and principal component analysis and decorrelation processing in early visual cortex. The chapter introduces some concepts from information theory. In particular, the entropy of a random variable and the mutual information between two random variables are focused. One of the major uses for information theory has been in interpretation and guidance for unsupervised neural networks: networks that are not provided with a teacher or target output that they are to emulate. The chapter describes how information relates to the more familiar supervised learning schemes, and discusses the use of error back propagation (BackProp) to minimize mean squared error (MSE) in a multi-layer perceptron (MLP). Other distortion measures are possible in place of MSE. In particular, the information theoretic cross-entropy distortion has been focused in the chapter.
Most sound scenes result from the superposition of several sources, which can be separately perceived and analyzed by human listeners. Source separation aims to provide machine listeners with similar skills by extracting the sounds of individual sources from a given scene. Existing separation systems operate either by emulating the human auditory system or by inferring the parameters of probabilistic sound models. In this chapter, the authors focus on the latter approach and provide a joint overview of established and recent models, including independent component analysis, local time-frequency models and spectral template-based models. They show that most models are instances of one of the following two general paradigms: linear modeling or variance modeling. They compare the merits of either paradigm and report objective performance figures. They also,conclude by discussing promising combinations of probabilistic priors and inference algorithms that could form the basis of future state-of-the-art systems.
Sub-Nyquist spectrum sensing and learning are investigated from theory to practice as a promising approach enabling cognitive and intelligent radios and wireless systems to work in GHz channel bandwidth. These techniques would be helpful for future electromagnetic spectrum sensing in sub-6 GHz, millimetre-wave, and Terahertz frequency bands. However, challenges such as computation complexity, real-time processing and theoretical sampling limits still exist. We issued a challenge with a reference sub-Nyquist algorithm, open data set and awards up to 10,000 USD to stimulate novel approaches and designs on sub-Nyquist spectrum sensing and learning algorithms to promote relative research and facilitate the theory-to-practice process of promising ideas.
Automatic Music Transcription is often performed by decomposing a spectrogram over a dictionary of note specific atoms. Several note template atoms may be used to represent one note, and a group structure may be imposed on the dictionary. We propose a group sparse algorithm based on a multiplicative update and thresholding and show transcription results on a challenging dataset.
A novel algorithm to separate S2 heart sounds into their aortic and pulmonary components is proposed. This approach is based on the assumption that, in different heartbeats of a given recording, aortic and pulmonary components maintain the same waveform but with different relative delays, which are induced by the variation of the thoracic pressure at different respiration phases. The proposed algorithm then retrieves the aortic and pulmonary components as the solution of an optimization problem which is approximated via alternating optimization. The proposed approach is shown to provide reconstructions of aortic and pulmonary components with normalized root mean-squared error consistently below 10% in various operational regimes.
Untwist is a new open source toolbox for audio source separation. The library provides a self-contained objectoriented framework including common source separation algorithms as well as input/output functions, data management utilities and time-frequency transforms. Everything is implemented in Python, facilitating research, experimentation and prototyping across platforms. The code is available on github 1.
This book constitutes the proceedings of the 14th International Conference on Latent Variable Analysis and Signal Separation, LVA/ICA 2018, held in Guildford, UK, in July 2018.The 52 full papers were carefully reviewed and selected from 62 initial submissions. As research topics the papers encompass a wide range of general mixtures of latent variables models but also theories and tools drawn from a great variety of disciplines such as structured tensor decompositions and applications; matrix and tensor factorizations; ICA methods; nonlinear mixtures; audio data and methods; signal separation evaluation campaign; deep learning and data-driven methods; advances in phase retrieval and applications; sparsity-related methods; and biomedical data and methods.
This work presents a geometrical analysis of the Large Step Gradient Descent (LGD) dictionary learning algorithm. LGD updates the atoms of the dictionary using a gradient step with a step size equal to twice the optimal step size. We show that the large step gradient descent can be understood as a maximal exploration step where one goes as far away as possible without increasing the the error. We also show that the LGD iteration is monotonic when the algorithm used for the sparse approximation step is close enough to orthogonal.
Large Language Models (LLMs) have shown great promise in integrating diverse expert models to tackle intricate language and vision tasks. Despite their significance in advancing the field of Artificial Intelligence Generated Content (AIGC), their potential in intelligent audio content creation remains unexplored. In this work, we tackle the problem of creating audio content with storylines encompassing speech, music, and sound effects, guided by text instructions. We present WavJourney, a system that leverages LLMs to connect various audio models for audio content generation. Given a text description of an auditory scene, WavJourney first prompts LLMs to generate a structured script dedicated to audio storytelling. The audio script incorporates diverse audio elements, organized based on their spatio-temporal relationships. As a conceptual representation of audio, the audio script provides an interactive and interpretable rationale for human engagement. Afterward, the audio script is fed into a script compiler, converting it into a computer program. Each line of the program calls a task-specific audio generation model or computational operation function (e.g., concatenate, mix). The computer program is then executed to obtain an explainable solution for audio generation. We demonstrate the practicality of WavJourney across diverse real-world scenarios, including science fiction, education, and radio play. The explainable and interactive design of WavJourney fosters human-machine co-creation in multi-round dialogues, enhancing creative control and adaptability in audio production. WavJourney audiolizes the human imagination, opening up new avenues for creativity in multimedia content creation.
Contrastive language-audio pretraining (CLAP) has been developed to align the representations of audio and language, achieving remarkable performance in retrieval and classification tasks. However, current CLAP struggles to capture temporal information within audio and text features, presenting substantial limitations for tasks such as audio retrieval and generation. To address this gap, we introduce T-CLAP, a temporal-enhanced CLAP model. We use Large Language Models (LLMs) and mixed-up strategies to generate temporal-contrastive captions for audio clips from extensive audio-text datasets. Subsequently, a new temporal-focused contrastive loss is designed to fine-tune the CLAP model by incorporating these synthetic data. We conduct comprehensive experiments and analysis in multiple downstream tasks. T-CLAP shows improved capability in capturing the temporal relationship of sound events and outperforms state-of-the-art models by a significant margin. Our code and models will be released soon.
Transformers, which were originally developed for natural language processing, have recently generated significant interest in the computer vision and audio communities due to their flexibility in learning long-range relationships. Constrained by the data hungry nature of transformers and the limited amount of labelled data, most transformer-based models for audio tasks are finetuned from ImageNet pretrained models, despite the huge gap between the domain of natural images and audio. This has motivated the research in self-supervised pretraining of audio transformers, which reduces the dependency on large amounts of labeled data and focuses on extracting concise representations of audio spectrograms. In this paper, we propose L ocal- G lobal A udio S pectrogram v I sion T ransformer, namely ASiT, a novel self-supervised learning framework that captures local and global contextual information by employing group masked model learning and self-distillation. We evaluate our pretrained models on both audio and speech classification tasks, including audio event classification, keyword spotting, and speaker identification. We further conduct comprehensive ablation studies, including evaluations of different pretraining strategies. The proposed ASiT framework significantly boosts the performance on all tasks and sets a new state-of-the-art performance in five audio and speech classification tasks, outperforming recent methods, including the approaches that use additional datasets for pretraining.
Audio source separation models are typically evaluated using objective separation quality measures, but rigorous statistical methods have yet to be applied to the problem of model comparison. As a result, it can be difficult to establish whether or not reliable progress is being made during the development of new models. In this paper, we provide a hypothesis-driven statistical analysis of the results of the recent source separation SiSEC challenge involving twelve competing models tested on separation of voice and accompaniment from fifty pieces of "professionally produced" contemporary music. Using non-parametric statistics, we establish reliable evidence for meaningful conclusions about the performance of the various models.
In deep neural networks with convolutional layers, all the neurons in each layer typically have the same size receptive fields (RFs) with the same resolution. Convolutional layers with neurons that have large RF capture global information from the input features, while layers with neurons that have small RF size capture local details with high resolution from the input features. In this work, we introduce novel deep multi-resolution fully convolutional neural networks (MR-FCN), where each layer has a range of neurons with different RF sizes to extract multi- resolution features that capture the global and local information from its input features. The proposed MR-FCN is applied to separate the singing voice from mixtures of music sources. Experimental results show that using MR-FCN improves the performance compared to feedforward deep neural networks (DNNs) and single resolution deep fully convolutional neural networks (FCNs) on the audio source separation problem.
Audio captioning aims to generate text descriptions of audio clips. In the real world, many objects produce similar sounds. How to accurately recognize ambiguous sounds is a major challenge for audio captioning. In this work, inspired by inherent human multimodal perception, we propose visually-aware audio captioning, which makes use of visual information to help the description of ambiguous sounding objects. Specifically , we introduce an off-the-shelf visual encoder to extract video features and incorporate the visual features into an audio captioning system. Furthermore, to better exploit complementary audiovisual contexts, we propose an audiovisual attention mechanism that adaptively integrates audio and visual context and removes the redundant information in the latent space. Experimental results on AudioCaps, the largest audio captioning dataset, show that our proposed method achieves state-of-the-art results on machine translation metrics.
Automated audio captioning (AAC) which generates textual descriptions of audio content. Existing AAC models achieve good results but only use the high-dimensional representation of the encoder. There is always insufficient information learning of high-dimensional methods owing to high-dimensional representations having a large amount of information. In this paper, a new encoder-decoder model called the Low-and High-Dimensional Feature Fusion (LHDFF) is proposed. LHDFF uses a new PANNs encoder called Residual PANNs (RPANNs) to fuse low-and high-dimensional features. Low-dimensional features contain limited information about specific audio scenes. The fusion of low-and high-dimensional features can improve model performance by repeatedly emphasizing specific audio scene information. To fully exploit the fused features, LHDFF uses a dual transformer decoder structure to generate captions in parallel. Experimental results show that LHDFF outperforms existing audio captioning models.
Following the successful application of AI and machine learning technologies to the recognition of speech and images, computer systems can now automatically analyse and recognise everyday real-world sound scenes and events. This new technology presents promising potential applications in environmental sensing and urban living. Specifically, urban soundscape analysis could be used to monitor and improve soundscapes experienced for people in towns and cities, helping to identify and formulate strategies for enhancing quality of life through future urban planning and development.
Deep neural networks (DNNs) are usually used for single channel source separation to predict either soft or binary time frequency masks. The masks are used to separate the sources from the mixed signal. Binary masks produce separated sources with more distortion and less interference than soft masks. In this paper, we propose to use another DNN to combine the estimates of binary and soft masks to achieve the advantages and avoid the disadvantages of using each mask individually. We aim to achieve separated sources with low distortion and low interference between each other. Our experimental results show that combining the estimates of binary and soft masks using DNN achieves lower distortion than using each estimate individually and achieves as low interference as the binary mask.
In this article, we present an account of the state of the art in acoustic scene classification (ASC), the task of classifying environments from the sounds they produce. Starting from a historical review of previous research in this area, we define a general framework for ASC and present different implementations of its components. We then describe a range of different algorithms submitted for a data challenge that was held to provide a general and fair benchmark for ASC techniques. The data set recorded for this purpose is presented along with the performance metrics that are used to evaluate the algorithms and statistical significance tests to compare the submitted methods.
This paper investigates the Audio Set classification. Audio Set is a large scale weakly labelled dataset (WLD) of audio clips. In WLD only the presence of a label is known, without knowing the happening time of the labels. We propose an attention model to solve this WLD problem and explain the attention model from a novel probabilistic perspective. Each audio clip in Audio Set consists of a collection of features. We call each feature as an instance and the collection as a bag following the terminology in multiple instance learning. In the attention model, each instance in the bag has a trainable probability measure for each class. The classification of the bag is the expectation of the classification output of the instances in the bag with respect to the learned probability measure. Experiments show that the proposed attention model achieves a mAP of 0.327 on Audio Set, outperforming the Google’s baseline of 0.314.
Recent theoretical analyses of a class of unsupervized Hebbian principal component algorithms have identified its local stability conditions. The only locally stable solution for the subspace P extracted by the network is the principal component subspace P∗. In this paper we use the Lyapunov function approach to discover the global stability characteristics of this class of algorithms. The subspace projection error, least mean squared projection error, and mutual information I are all Lyapunov functions for convergence to the principal subspace, although the various domains of convergence indicated by these Lyapunov functions leave some of P-space uncovered. A modification to I yields a principal subspace information Lyapunov function I′ with a domain of convergence that covers almost all of P-space. This shows that this class of algorithms converges to the principal subspace from almost everywhere.
Perceptual measurements have typically been recognized as the most reliable measurements in assessing perceived levels of reverberation. In this paper, a combination of blind RT60 estimation method and a binaural, nonlinear auditory model is employed to derive signal-based measures (features) that are then utilized in predicting the perceived level of reverberation. Such measures lack the excess of effort necessary for calculating perceptual measures; not to mention the variations in either stimuli or assessors that may cause such measures to be statistically insignificant. As a result, the automatic extraction of objective measurements that can be applied to predict the perceived level of reverberation become of vital significance. Consequently, this work is aimed at discovering measurements such as clarity, reverberance, and RT60 which can automatically be derived directly from audio data. These measurements along with labels from human listening tests are then forwarded to a machine learning system seeking to build a model to estimate the perceived level of reverberation, which is labeled by an expert, autonomously. The data has been labeled by an expert human listener for a unilateral set of files from arbitrary audio source types. By examining the results, it can be observed that the automatically extracted features can aid in estimating the perceptual rates.
Automatic and fast tagging of natural sounds in audio collections is a very challenging task due to wide acoustic variations, the large number of possible tags, the incomplete and ambiguous tags provided by different labellers. To handle these problems, we use a co-regularization approach to learn a pair of classifiers on sound and text. The first classifier maps low-level audio features to a true tag list. The second classifier maps actively corrupted tags to the true tags, reducing incorrect mappings caused by low-level acoustic variations in the first classifier, and to augment the tags with additional relevant tags. Training the classifiers is implemented using marginal co-regularization, pair of which draws the two classifiers into agreement by a joint optimization. We evaluate this approach on two sound datasets, Freefield1010 and Task4 of DCASE2016. The results obtained show that marginal co-regularization outperforms the baseline GMM in both ef- ficiency and effectiveness.
Situated in the domain of urban sound scene classification by humans and machines, this research is the first step towards mapping urban noise pollution experienced indoors and finding ways to reduce its negative impact in peoples’ homes. We have recorded a sound dataset, called Open-Window, which contains recordings from three different locations and four different window states; two stationary states (open and close) and two transitional states (open to close and close to open). We have then built our machine recognition base lines for different scenarios (open set versus closed set) using a deep learning framework. The human listening test is also performed to be able to compare the human and machine performance for detecting the window state just using the acoustic cues. Our experimental results reveal that when using a simple machine baseline system, humans and machines are achieving similar average performance for closed set experiments.
Deep neural networks have recently achieved break-throughs in sound generation. Despite the outstanding sample quality, current sound generation models face issues on small-scale datasets (e.g., overfitting), significantly limiting performance. In this paper, we make the first attempt to investigate the benefits of pre-training on sound generation with AudioLDM, the cutting-edge model for audio generation, as the backbone. Our study demonstrates the advantages of the pre-trained AudioLDM, especially in data-scarcity scenarios. In addition, the baselines and evaluation protocol for sound generation systems are not consistent enough to compare different studies directly. Aiming to facilitate further study on sound generation tasks, we benchmark the sound generation task on various frequently-used datasets. We hope our results on transfer learning and benchmarks can provide references for further research on conditional sound generation.
Sound event detection (SED) and localization refer to recognizing sound events and estimating their spatial and temporal locations. Using neural networks has become the prevailing method for SED. In the area of sound localization, which is usually performed by estimating the direction of arrival (DOA), learning-based methods have recently been developed. In this paper, it is experimentally shown that the trained SED model is able to contribute to the direction of arrival estimation (DOAE). However, joint training of SED and DOAE degrades the performance of both. Based on these results, a two-stage polyphonic sound event detection and localization method is proposed. The method learns SED first, after which the learned feature layers are transferred for DOAE. It then uses the SED ground truth as a mask to train DOAE. The proposed method is evaluated on the DCASE 2019 Task 3 dataset, which contains different overlapping sound events in different environments. Experimental results show that the proposed method is able to improve the performance of both SED and DOAE, and also performs significantly better than the baseline method.
Audio super-resolution is a fundamental task that predicts high-frequency components for low-resolution audio, enhancing audio quality in digital applications. Previous methods have limitations such as the limited scope of audio types (e.g., music, speech) and specific bandwidth settings they can handle (e.g., 4kHz to 8kHz). In this paper, we introduce a diffusion-based generative model, AudioSR, that is capable of performing robust audio super-resolution on versatile audio types, including sound effects, music, and speech. Specifically, AudioSR can upsample any input audio signal within the bandwidth range of 2kHz to 16kHz to a high-resolution audio signal at 24kHz bandwidth with a sampling rate of 48kHz. Extensive objective evaluation on various audio super-resolution benchmarks demonstrates the strong result achieved by the proposed model. In addition, our subjective evaluation shows that AudioSR can acts as a plug-and-play module to enhance the generation quality of a wide range of audio generative models, including AudioLDM, Fastspeech2, and MusicGen. Our code and demo are available at https://audioldm.github.io/audiosr.
Probabilistic approaches to tracking often use single-source Bayesian models; applying these to multi-source tasks is problematic. We apply a principled multi-object tracking implementation, the Gaussian mixture probability hypothesis density filter, to track multiple sources having fixed pitch plus vibrato. We demonstrate high-quality ltering in a synthetic experiment, and nd improved tracking using a richer feature set which captures underlying dynamics. Our implementation is available as open-source Python code.
Audio tagging is the task of predicting the presence or absence of sound classes within an audio clip. Previous work in audio tagging focused on relatively small datasets limited to recognising a small number of sound classes. We investigate audio tagging on AudioSet, which is a dataset consisting of over 2 million audio clips and 527 classes. AudioSet is weakly labelled, in that only the presence or absence of sound classes is known for each clip, while the onset and offset times are unknown. To address the weakly-labelled audio tagging problem, we propose attention neural networks as a way to attend the most salient parts of an audio clip. We bridge the connection between attention neural networks and multiple instance learning (MIL) methods, and propose decision-level and feature-level attention neural networks for audio tagging. We investigate attention neural networks modelled by different functions, depths and widths. Experiments on AudioSet show that the feature-level attention neural network achieves a state-of-the-art mean average precision (mAP) of 0.369, outperforming the best multiple instance learning (MIL) method of 0.317 and Google’s deep neural network baseline of 0.314. In addition, we discover that the audio tagging performance on AudioSet embedding features has a weak correlation with the number of training examples and the quality of labels of each sound class.
Source separation (SS) aims to separate individual sources from an audio recording. Sound event detection (SED) aims to detect sound events from an audio recording. We propose a joint separation-classification (JSC) model trained only on weakly labelled audio data, that is, only the tags of an audio recording are known but the time of the events are unknown. First, we propose a separation mapping from the time-frequency (T-F) representation of an audio to the T-F segmentation masks of the audio events. Second, a classification mapping is built from each T-F segmentation mask to the presence probability of each audio event. In the source separation stage, sources of audio events and time of sound events can be obtained from the T-F segmentation masks. The proposed method achieves an equal error rate (EER) of 0.14 in SED, outperforming deep neural network baseline of 0.29. Source separation SDR of 8.08 dB is obtained by using global weighted rank pooling (GWRP) as probability mapping, outperforming the global max pooling (GMP) based probability mapping giving SDR at 0.03 dB. Source code of our work is published.
In this paper, we consider the dictionary learning problem for the sparse analysis model. A novel algorithm is proposed by adapting the simultaneous codeword optimization (SimCO) algorithm, based on the sparse synthesis model, to the sparse analysis model. This algorithm assumes that the analysis dictionary contains unit ℓ2-norm atoms and learns the dictionary by optimization on manifolds. This framework allows multiple dictionary atoms to be updated simultaneously in each iteration. However, similar to several existing analysis dictionary learning algorithms, dictionaries learned by the proposed algorithm may contain similar atoms, leading to a degenerate (coherent) dictionary. To address this problem, we also consider restricting the coherence of the learned dictionary and propose Incoherent Analysis SimCO by introducing an atom decorrelation step following the update of the dictionary. We demonstrate the competitive performance of the proposed algorithms using experiments with synthetic data
Perceptual measures are usually considered more reliable than instrumental measures for evaluating the perceived level of reverberation. However, such measures are time consuming and expensive, and, due to variations in stimuli or assessors, the resulting data is not always statistically significant. Therefore, an (objective) measure of the perceived level of reverberation becomes desirable. In this paper, we develop a new method to predict the level of reverberation from audio signals by relating the perceptual listening test results with those obtained from a machine learned model. More specifically, we compare the use of a multiple stimuli test for within and between class architectures to evaluate the perceived level of reverberation. An expert set of 16 human listeners rated the perceived level of reverberation for a same set of files from different audio source types. We then train a machine learning model using the training data gathered for the same set of files and a variety of reverberation related features extracted from the data such as reverberation time, and direct to reverberation ratio. The results suggest that the machine learned model offers an accurate prediction of the perceptual scores.
Most single channel audio source separation (SCASS) approaches produce separated sources accompanied by interference from other sources and other distortions. To tackle this problem, we propose to separate the sources in two stages. In the first stage, the sources are separated from the mixed signal. In the second stage, the interference between the separated sources and the distortions are reduced using deep neural networks (DNNs). We propose two methods that use DNNs to improve the quality of the separated sources in the second stage. In the first method, each separated source is improved individually using its own trained DNN, while in the second method all the separated sources are improved together using a single DNN. To further improve the quality of the separated sources, the DNNs in the second stage are trained discriminatively to further decrease the interference and the distortions of the separated sources. Our experimental results show that using two stages of separation improves the quality of the separated signals by decreasing the interference between the separated sources and distortions compared to separating the sources using a single stage of separation.
Audio source separation models are typically evaluated using objective separation quality measures, but rigorous statistical methods have yet to be applied to the problem of model comparison. As a result, it can be difficult to establish whether or not reliable progress is being made during the development of new models. In this paper, we provide a hypothesis-driven statistical analysis of the results of the recent source separation SiSEC challenge involving twelve competing models tested on separation of voice and accompaniment from fifty pieces of “professionally produced” contemporary music. Using nonparametric statistics, we establish reliable evidence for meaningful conclusions about the performance of the various models.
In this technique report, we present a bunch of methods for the task 4 of Detection and Classification of Acoustic Scenes and Events 2017 (DCASE2017) challenge. This task evaluates systems for the large-scale detection of sound events using weakly labeled training data. The data are YouTube video excerpts focusing on transportation and warnings due to their industry applications. There are two tasks, audio tagging and sound event detection from weakly labeled data. Convolutional neural network (CNN) and gated recurrent unit (GRU) based recurrent neural network (RNN) are adopted as our basic framework. We proposed a learnable gating activation function for selecting informative local features. Attention-based scheme is used for localizing the specific events in a weakly-supervised mode. A new batch-level balancing strategy is also proposed to tackle the data unbalancing problem. Fusion of posteriors from different systems are found effective to improve the performance. In a summary, we get 61% F-value for the audio tagging subtask and 0.73 error rate (ER) for the sound event detection subtask on the development set. While the official multilayer perceptron (MLP) based baseline just obtained 13.1% F-value for the audio tagging and 1.02 for the sound event detection.
The analysis of large datasets of music audio and other representations entails the need for techniques that support musicologists and other users in interpreting extracted data. We explore and develop visualisation techniques of chord sequence patterns mined from a corpus of over one million tracks. The visualisations use different representations of root movements and chord qualities with geometrical representations, and mostly colour mappings for pattern support. The presented visualisations are being developed in close collaboration with musicologists and can help gain insights into the differences of musical genres and styles as well as support the development of new classification methods.
This paper presents two new algorithms for wideband spectrum sensing at sub-Nyquist sampling rates, for both single nodes and cooperative multiple nodes. In single-node spectrum sensing, a two-phase spectrum sensing algorithm based on compressive sensing is proposed to reduce the computational complexity and improve the robustness at secondary users (SUs). In the cooperative multiple nodes case, the signals received at SUs exhibit a sparsity property that yields a low-rank matrix of compressed measurements at the fusion center. This therefore leads to a two-phase cooperative spectrum sensing algorithm for cooperative multiple SUs based on low-rank matrix completion. In addition, the two proposed spectrum sensing algorithms are evaluated on the TV white space (TVWS), in which pioneering work aimed at enabling dynamic spectrum access into practice has been promoted by both the Federal Communications Commission and the U.K. Office of Communications. The proposed algorithms are tested on the real-time signals after they have been validated by the simulated signals in TVWS. The numerical results show that our proposed algorithms are more robust to channel noise and have lower computational complexity than the state-of-the-art algorithms.
We describe a new method for preprocessing STFT phasevocoder frames for improved performance in real-time onset detection, which we term "adaptive whitening". The procedure involves normalising the magnitude of each bin according to a recent maximum value for that bin, with the aim of allowing each bin to achieve a similar dynamic range over time, which helps to mitigate against the influence of spectral roll-off and strongly-varying dynamics. Adaptive whitening requires no training, is relatively lightweight to compute, and can run in real-time. Yet it can improve onset detector performance by more than ten percentage points (peak F-measure) in some cases, and improves the performance of most of the onset detectors tested. We present results demonstrating that adaptive whitening can significantly improve the performance of various STFT-based onset detection functions, including functions based on the power, spectral flux, phase deviation, and complex deviation measures. Our results find the process to be especially beneficial for certain types of audio signal (e.g. complex mixtures such as pop music).
Convolutional neural networks (CNNs) have shown state-of-the-art performance in various applications. However, CNNs are resource-hungry due to their requirement of high computational complexity and memory storage. Recent efforts toward achieving computational efficiency in CNNs involve filter pruning methods that eliminate some of the filters in CNNs based on the \enquote{importance} of the filters. The majority of existing filter pruning methods are either "active", which use a dataset and generate feature maps to quantify filter importance, or "passive", which compute filter importance using entry-wise norm of the filters without involving data. Under a high pruning ratio where large number of filters are to be pruned from the network, the entry-wise norm methods eliminate relatively smaller norm filters without considering the significance of the filters in producing the node output, resulting in degradation in the performance. To address this, we present a passive filter pruning method where the filters are pruned based on their contribution in producing output by considering the operator norm of the filters. The proposed pruning method generalizes better across various CNNs compared to that of the entry-wise norm-based pruning methods. In comparison to the existing active filter pruning methods, the proposed pruning method is at least 4.5 times faster in computing filter importance and is able to achieve similar performance compared to that of the active filter pruning methods. The efficacy of the proposed pruning method is evaluated on audio scene classification and image classification using various CNNs architecture such as VGGish, DCASE21_Net, VGG-16 and ResNet-50.
In this paper, a system for overlapping acoustic event detection is proposed, which models the temporal evolution of sound events. The system is based on probabilistic latent component analysis, supporting the use of a sound event dictionary where each exemplar consists of a succession of spectral templates. The temporal succession of the templates is controlled through event class-wise Hidden Markov Models (HMMs). As input time/frequency representation, the Equivalent Rectangular Bandwidth (ERB) spectrogram is used. Experiments are carried out on polyphonic datasets of office sounds generated using an acoustic scene simulator, as well as real and synthesized monophonic datasets for comparative purposes. Results show that the proposed system outperforms several state-of-the-art methods for overlapping acoustic event detection on the same task, using both frame-based and event-based metrics, and is robust to varying event density and noise levels.
In the context of the Internet of Things (IoT), sound sensing applications are required to run on embedded platforms where notions of product pricing and form factor impose hard constraints on the available computing power. Whereas Automatic Environmental Sound Recognition (AESR) algorithms are most often developed with limited consideration for computational cost, this article seeks which AESR algorithm can make the most of a limited amount of computing power by comparing the sound classification performance as a function of its computational cost. Results suggest that Deep Neural Networks yield the best ratio of sound classification accuracy across a range of computational costs, while Gaussian Mixture Models offer a reasonable accuracy at a consistently small cost, and Support Vector Machines stand between both in terms of compromise between accuracy and computational cost.
We present a method for real-time pitch-tracking which generates an estimation of the relative amplitudes of the partials relative to the fundamental for each detected note. We then employ a subtraction method, whereby lower fundamentals in the spectrum are accounted for when looking at higher fundamental notes. By tracking notes which are playing, we look for note off events and continually update our expected partial weightings for each note. The resulting algorithm makes use of these relative partial weightings within its decision process. We have evaluated the system against a data set and compared it with specialised offline pitch-trackers. © July 2009- All copyright remains with the individual authors.
This paper proposes sound event localization and detection methods from multichannel recording. The proposed system is based on two Convolutional Recurrent Neural Networks (CRNNs) to perform sound event detection (SED) and time difference of arrival (TDOA) estimation on each pair of microphones in a microphone array. In this paper, the system is evaluated with a four-microphone array, and thus combines the results from six pairs of microphones to provide a final classification and a 3-D direction of arrival (DOA) estimate. Results demonstrate that the proposed approach outperforms the DCASE 2019 baseline system.
The Detection and Classification of Acoustic Scenes and Events (DCASE) consists of five audio classification and sound event detectiontasks: 1)Acousticsceneclassification,2)General-purposeaudio tagging of Freesound, 3) Bird audio detection, 4) Weakly-labeled semi-supervised sound event detection and 5) Multi-channel audio classification. In this paper, we create a cross-task baseline system for all five tasks based on a convlutional neural network (CNN): a “CNN Baseline” system. We implemented CNNs with 4 layers and 8 layers originating from AlexNet and VGG from computer vision. We investigated how the performance varies from task to task with the same configuration of neural networks. Experiments show that deeper CNN with 8 layers performs better than CNN with 4 layers on all tasks except Task 1. Using CNN with 8 layers, we achieve an accuracy of 0.680 on Task 1, an accuracy of 0.895 and a mean average precision (MAP) of 0.928 on Task 2, an accuracy of 0.751 andanareaunderthecurve(AUC)of0.854onTask3,asoundevent detectionF1scoreof20.8%onTask4,andanF1scoreof87.75%on Task 5. We released the Python source code of the baseline systems under the MIT license for further research.
Live performance situations can lead to degradations in the vocal signal from a typical microphone, such as ambient noise or echoes due to feedback. We investigate the robustness of continuousvalued timbre features measured on vocal signals (speech, singing, beatboxing) under simulated degradations. We also consider nonparametric dependencies between features, using information theoretic measures and a feature-selection algorithm. We discuss how robustness and independence issues reflect on the choice of acoustic features for use in constructing a continuous-valued vocal timbre space. While some measures (notably spectral crest factors) emerge as good candidates for such a task, others are poor, and some features such as ZCR exhibit an interaction with the type of voice signal being analysed.
Significant amounts of user-generated audio content, such as sound effects, musical samples and music pieces, are uploaded to online repositories and made available under open licenses. Moreover, a constantly increasing amount of multimedia content, originally released with traditional licenses, is becoming public domain as its license expires. Nevertheless, the creative industries are not yet using much of all this content in their media productions. There is still a lack of familiarity and understanding of the legal context of all this open content, but there are also problems related with its accessibility. A big percentage of this content remains unreachable either because it is not published online or because it is not well organised and annotated. In this paper we present the Audio Commons Initiative, which is aimed at promoting the use of open audio content and at developing technologies with which to support the ecosystem composed by content repositories, production tools and users. These technologies should enable the reuse of this audio material, facilitating its integration in the production workflows used by the creative industries. This is a position paper in which we describe the core ideas behind this initiative and outline the ways in which we plan to address the challenges it poses.
The workplace is a site for the rapid development and deployment of Artificial Intelligence (AI) systems. However, our research suggests that their adoption could already be hindered by critical issues such as trust, privacy, and security. This paper examines the integration of AI-enabled sound technologies in the workplace, with a focus on enhancing well-being and productivity through a soundscape approach while addressing ethical concerns. To explore these concepts, we used scenario-based design and structured feedback sessions with knowledge workers from open-plan offices and those working from home. To do this, we present initial design concepts for AI sound analysis and control systems. Based on the perspectives gathered, we present user requirements and concerns, particularly regarding privacy and the potential for workplace surveillance, emphasising the need for user consent and levels of transparency in AI deployments. Navigating these ethical considerations is a key implication of the study. We advocate for novel ways to incorporate people’s involvement in the design process through co-design and serious games to shape the future of AI audio technologies in the workplace.
Although audio generation shares commonalities across different types of audio, such as speech, music, and sound effects, designing models for each type requires careful consideration of specific objectives and biases that can significantly differ from those of other types. To bring us closer to a unified perspective of audio generation, this paper proposes a framework that utilizes the same learning method for speech, music, and sound effect generation. Our framework introduces a general representation of audio, called "language of audio" (LOA). Any audio can be translated into LOA based on AudioMAE, a self-supervised pre-trained representation learning model. In the generation process, we translate any modalities into LOA by using a GPT-2 model, and we perform self-supervised audio generation learning with a latent diffusion model conditioned on LOA. The proposed framework naturally brings advantages such as in-context learning abilities and reusable self-supervised pretrained AudioMAE and latent diffusion models. Experiments on the major benchmarks of text-to-audio, text-to-music, and text-to-speech demonstrate state-of-the-art or competitive performance against previous approaches. Our code, pretrained model, and demo are available at https://audioldm.github.io/audioldm2.
Many people listen to recorded music as part of their everyday lives, for example from radio or TV programmes, CDs, downloads or increasingly from online streaming services. Sometimes we might want to remix the balance within the music, perhaps to make the vocals louder or to suppress an unwanted sound, or we might want to upmix a 2-channel stereo recording to a 5.1- channel surround sound system. We might also want to change the spatial location of a musical instrument within the mix. All of these applications are relatively straightforward, provided we have access to separate sound channels (stems) for each musical audio object. However, if we only have access to the final recording mix, which is usually the case, this is much more challenging. To estimate the original musical sources, which would allow us to remix, suppress or upmix the sources, we need to perform musical source separation (MSS). In the general source separation problem, we are given one or more mixture signals that contain different mixtures of some original source signals. This is illustrated in Figure 1 where four sources, namely vocals, drums, bass and guitar, are all present in the mixture. The task is to recover one or more of the source signals given the mixtures. In some cases, this is relatively straightforward, for example, if there are at least as many mixtures as there are sources, and if the mixing process is fixed, with no delays, filters or non-linear mastering [1]. However, MSS is normally more challenging. Typically, there may be many musical instruments and voices in a 2-channel recording, and the sources have often been processed with the addition of filters and reverberation (sometimes nonlinear) in the recording and mixing process. In some cases, the sources may move, or the production parameters may change, meaning that the mixture is time-varying. All of these issues make MSS a very challenging problem. Nevertheless, musical sound sources have particular properties and structures that can help us. For example, musical source signals often have a regular harmonic structure of frequencies at regular intervals, and can have frequency contours characteristic of each musical instrument. They may also repeat in particular temporal patterns based on the musical structure. In this paper we will explore the MSS problem and introduce approaches to tackle it. We will begin by introducing characteristics of music signals, we will then give an introduction to MSS, and finally consider a range of MSS models. We will also discuss how to evaluate MSS approaches, and discuss limitations and directions for future research
We have previously proposed a structured sparse approach to piano transcription with promising results recorded on a challenging dataset. The approach taken was measured in terms of both frame-based and onset-based metrics. Close inspection of the results revealed problems in capturing frames displaying low-energy of a given note, for example in sustained notes. Further problems were also noticed in the onset detection, where for many notes seen to be active in the output trancription an onset was not detected. A brief description of the approach is given here, and further analysis of the system is given by considering an oracle transcription, derived from the ground truth piano roll and the given dictionary of spectral template atoms, which gives a clearer indication of the problems which need to be overcome in order to improve the proposed approach.
Automated audio captioning (AAC) aims to generate textual descriptions for a given audio clip. Despite the existing AAC models obtaining promising performance, they struggle to capture intricate audio patterns due to only using a high-dimensional representation. In this paper, we propose a new encoder-decoder model for AAC, called the Pyramid Feature Fusion and Cross Context Attention Network (PFCA-Net). In PFCA-Net, the encoder is constructed using a pyramid network, facilitating the extraction of audio features across multiple scales. It achieves this by combining top-down and bottom-up connections to fuse features across scales, resulting in feature maps at various scales. In the decoder, cross-content attention is designed to fuse the different scale features which allows the propagation of information from a low-scale to a high-scale. Experimental results show that PFCA-Net achieves considerable improvement over existing models.
Automatic music transcription (AMT) can be performed by deriving a pitch-time representation through decomposition of a spectrogram with a dictionary of pitch-labelled atoms. Typically, non-negative matrix factorisation (NMF) methods are used to decompose magnitude spectrograms. One atom is often used to represent each note. However, the spectrum of a note may change over time. Previous research considered this variability using different atoms to model specific parts of a note, or large dictionaries comprised of datapoints from the spectrograms of full notes. In this paper, the use of subspace modelling of note spectra is explored, with group sparsity employed as a means of coupling activations of related atoms into a pitched subspace. Stepwise and gradient-based methods for non-negative group sparse decompositions are proposed. Finally, a group sparse NMF approach is used to tune a generic harmonic subspace dictionary, leading to improved NMF-based AMT results.
Time-scale transformations of audio signals have traditionally relied exclusively upon manipulations of tempo. We present a novel technique for automatic mixing and synchronization between two musical signals. In this transformation, the original signal assumes the tempo, meter, and rhythmic structure of the model signal, while the extracted downbeats and salient intra-measure infrastructure of the original are maintained.
In this paper, a system for polyphonic sound event detection and tracking is proposed, based on spectrogram factorisation techniques and state space models. The system extends probabilistic latent component analysis (PLCA) and is modelled around a 4-dimensional spectral template dictionary of frequency, sound event class, exemplar index, and sound state. In order to jointly track multiple overlapping sound events over time, the integration of linear dynamical systems (LDS) within the PLCA inference is proposed. The system assumes that the PLCA sound event activation is the (noisy) observation in an LDS, with the latent states corresponding to the true event activations. LDS training is achieved using fully observed data, making use of ground truth-informed event activations produced by the PLCA-based model. Several LDS variants are evaluated, using polyphonic datasets of office sounds generated from an acoustic scene simulator, as well as real and synthesized monophonic datasets for comparative purposes. Results show that the integration of LDS tracking within PLCA leads to an improvement of +8.5-10.5% in terms of frame-based F-measure as compared to the use of the PLCA model alone. In addition, the proposed system outperforms several state-of-the-art methods for the task of polyphonic sound event detection.
In this paper, we propose a divide-and-conquer approach using two generative adversarial networks (GANs) to explore how a machine can draw colorful pictures (bird) using a small amount of training data. In our work, we simulate the procedure of an artist drawing a picture, where one begins with drawing objects’ contours and edges and then paints them different colors. We adopt two GAN models to process basic visual features including shape, texture and color. We use the first GAN model to generate object shape, and then paint the black and white image based on the knowledge learned using the second GAN model. We run our experiments on 600 color images. The experimental results show that the use of our approach can generate good quality synthetic images, comparable to real ones.
Though many past works have tried to cluster expressive timing within a phrase, there have been few attempts to cluster features of expressive timing with constant dimensions regardless of phrase lengths. For example, used as a way to represent expressive timing, tempo curves can be regressed by a polynomial function such that the number of regressed polynomial coefficients remains constant with a given order regardless of phrase lengths. In this paper, clustering the regressed polynomial coefficients is proposed for expressive timing analysis. A model selection test is presented to compare Gaussian Mixture Models (GMMs) fitting regressed polynomial coefficients and fitting expressive timing directly. As there are no expected results of clustering expressive timing, the proposed method is demonstrated by how well the expressive timing are approximated by the centroids of GMMs. The results show that GMMs fitting the regressed polynomial coefficients outperform GMMs fitting expressive timing directly. This conclusion suggests that it is possible to use regressed polynomial coefficients to represent expressive timing within a phrase and cluster expressive timing within phrases of different lengths.
The choice of data augmentation is pivotal in contrastive self-supervised learning. Current augmentation techniques for audio data, such as the widely used Random Resize Crop (RRC), underperform in pitch-sensitive music tasks and lack generalisation across various types of audio. This study aims to address these limitations by introducing Neural Compression Augmentation (NCA), an approach based on lossy neural compression. We use the Audio Barlow Twins (ABT), a contrastive self-supervised framework for audio, as our backbone. We experiment with both NCA and several baseline augmentation methods in the augmentation block of ABT and train the models on AudioSet. Experimental results show that models integrated with NCA considerably surpass the original performance of ABT, especially in the music tasks of the HEAR benchmark, demonstrating the effectiveness of compression-based augmentation for audio contrastive self-supervised learning.
When working with generative systems, designers enter into a loop of discrete steps; external evaluations of the output feedback into the system, and new outputs are subsequently reevaluated. In such systems, interacting low level elements can engender a difficult to predict emergence of macro-level characteristics. Furthermore, the state space of some systems can be vast. Consequently, designers generally rely on trial-and-error, experience or intuition in selecting parameter values to develop the aesthetic aspects of their designs. We investigate an alternative means of exploring the state spaces of generative visual systems by using a gaze- contingent display. A user's gaze continuously controls and directs an evolution of visual forms and patterns on screen. As time progresses and the viewer and system remain coupled in this evolution, a population of generative artefacts tends towards an area of their state space that is 'of interest', as defined by the eye tracking data. The evaluation-feedback loop is continuous and uninterrupted, gaze the guiding feedback mechanism in the exploration of state space.
We explore the use of geometrical methods to tackle the non-negative independent component analysis (non-negative ICA) problem, without assuming the reader has an existing background in differential geometry. We concentrate on methods that achieve this by minimizing a cost function over the space of orthogonal matrices. We introduce the idea of the manifold and Lie group SO(n) of special orthogonal matrices that we wish to search over, and explain how this is related to the Lie algebra so(n) of skew-symmetric matrices. We describe how familiar optimization methods such as steepest-descent and conjugate gradients can be transformed into this Lie group setting, and how the Newton update step has an alternative Fourier version in SO(n). Finally we introduce the concept of a toral subgroup generated by a particular element of the Lie group or Lie algebra, and explore how this commutative subgroup might be used to simplify searches on our constraint surface. No proofs are presented in this article.
MIDI renderings of audio are traditionally regarded as lifeless and unnatural - lacking in expression. However, MIDI is simply a protocol for controlling a synthesizer. Lack of expression is caused by either an expressionless synthesizer or by the difficulty in setting the MIDI parameters to provide expressive output. We have developed a system to construct an expressive MIDI representation of an audio signal, i.e. an audio representation which uses tailored pitch variations in addition to the note base pitch parameters which audio-to-MIDI systems usually attempt. A pitch envelope is estimated from the original audio, and a genetic algorithm is then used to estimate pitch modulator parameters from that envelope. These pitch modulations are encoded in a MIDI file and rerendered using a sampler. We present some initial comparisons between the final output audio and the estimated pitch envelopes.
The Signal Separation Evaluation Campaign (SiSEC) is a large-scale regular event aimed at evaluating current progress in source separation through a systematic and reproducible comparison of the participants’ algorithms, providing the source separation community with an invaluable glimpse of recent achievements and open challenges. This paper focuses on the music separation task from SiSEC 2018, which compares algorithms aimed at recovering instrument stems from a stereo mix. In this context, we conducted a subjective evaluation whereby 34 listeners picked which of six competing algorithms, with high objective performance scores, best separated the singing-voice stem from 13 professionally mixed songs. The subjective results reveal strong differences between the algorithms, and highlight the presence of song-dependent performance for state-of-the-art systems. Correlations between the subjective results and the scores of two popular performance metrics are also presented.
Humans are able to identify a large number of environmental sounds and categorise them according to high-level semantic categories, e.g. urban sounds or music. They are also capable of generalising from past experience to new sounds when applying these categories. In this paper we report on the creation of a data set that is structured according to the top-level of a taxonomy derived from human judgements and the design of an associated machine learning challenge, in which strong generalisation abilities are required to be successful. We introduce a baseline classification system, a deep convolutional network, which showed strong performance with an average accuracy on the evaluation data of 80.8%. The result is discussed in the light of two alternative explanations: An unlikely accidental category bias in the sound recordings or a more plausible true acoustic grounding of the high-level categories.
As consumers move increasingly to multichannel and surround-sound reproduction of sound, and also perhaps wish to remix their music to suit their own tastes, there will be an increasing need for high quality automatic source separation to recover sound sources from legacy mono or 2-channel stereo recordings. In this Contribution, we will give an overview of some for audio source separation, and some of the remaining research challenges in this area.
This paper describes an algorithm for real-time beat tracking with a visual interface. Multiple tempo and phase hypotheses are represented by a comb filter matrix. The user can interact by specifying the tempo and phase to be tracked by the algorithm, which will seek to find a continuous path through the space. We present results from evaluating the algorithm on the Hainsworth database and offer a comparison with another existing real-time beat tracking algorithm and offline algorithms.
Audio editing is performed at scale in the production of radio, but often the tools used are poorly targeted toward the task at hand. There are a number of audio analysis techniques that have the potential to aid radio producers, but without a detailed understanding of their process and requirements, it can be difficult to apply these methods. To aid this understanding, a study of radio production practice was conducted on three varied case studies—a news bulletin, drama, and documentary. It examined the audio/metadata workflow, the roles and motivations of the producers, and environmental factors. The study found that producers prefer to interact with higher-level representations of audio content like transcripts and enjoy working on paper. The study also identified opportunities to improve the work flow with tools that link audio to text, highlight repetitions, compare takes, and segment speakers.
Supervised multi-channel audio source separation requires extracting useful spectral, temporal, and spatial features from the mixed signals. The success of many existing systems is therefore largely dependent on the choice of features used for training. In this work, we introduce a novel multi-channel, multiresolution convolutional auto-encoder neural network that works on raw time-domain signals to determine appropriate multiresolution features for separating the singing-voice from stereo music. Our experimental results show that the proposed method can achieve multi-channel audio source separation without the need for hand-crafted features or any pre- or post-processing.
An increasing number of researchers work in computational auditory scene analysis (CASA). However, a set of tasks, each with a well-defined evaluation framework and commonly used datasets do not yet exist. Thus, it is difficult for results and algorithms to be compared fairly, which hinders research on the field. In this paper we will introduce a newly-launched public evaluation challenge dealing with two closely related tasks of the field: acoustic scene classification and event detection. We give an overview of the tasks involved; describe the processes of creating the dataset; and define the evaluation metrics. Finally, illustrations on results for both tasks using baseline methods applied on this dataset are presented, accompanied by open-source code. © 2013 EURASIP.
We investigate the conditions for which nonnegative matrix factorization (NMF) is unique and introduce several theorems which can determine whether the decomposition is in fact unique or not. The theorems are illustrated by several examples showing the use of the theorems and their limitations. We have shown that corruption of a unique NMF matrix by additive noise leads to a noisy estimation of the noise-free unique solution. Finally, we use a stochastic view of NMF to analyze which characterization of the underlying model will result in an NMF with small estimation errors.
Phrases are common musical units akin to that in speech and text. In music performance, performers often change the way they vary the tempo from one phrase to the next in order to choreograph patterns of repetition and contrast. This activity is commonly referred to as expressive music performance. Despite its importance, expressive performance is still poorly understood. No formal models exist that would explain, or at least quantify and characterise, aspects of commonalities and differences in performance style. In this paper we present such a model for tempo variation between phrases in a performance. We demonstrate that the model provides a good fit with a performance database of 25 pieces and that perceptually important information is not lost through the modelling process.
In this paper we address the issue of causal rhythmic analysis, primarily towards predicting the locations of musical beats such that they are consistent with a musical audio input. This will be a key component required for a system capable of automatic accompaniment with a live musician. We are implementing our approach as part of the aubio real-time audio library. While performance for this causal system is reduced in comparison to our previous non-causal system, it is still suitable for our intended purpose.
The Serendiptichord is a wearable instrument, resulting from a collaboration crossing fashion, technology, music and dance. This paper reflects on the collaborative process and how defining both creative and research roles for each party led to a successful creative partnership built on mutual respect and open communication. After a brief snapshot of the instrument in performance, the instrument is considered within the context of dance-driven interactive music systems followed by a discussion on the nature of the collaboration and its impact upon the design process and final piece. © 2013 ISAST.
We describe an inference task in which a set of timestamped event observations must be clustered into an unknown number of temporal sequences with independent and varying rates of observations. Various existing approaches to multi-object tracking assume a fixed number of sources and/or a fixed observation rate; we develop an approach to inferring structure in timestamped data produced by a mixture of an unknown and varying number of similar Markov renewal processes, plus independent clutter noise. The inference simultaneously distinguishes signal from noise as well as clustering signal observations into separate source streams. We illustrate the technique via synthetic experiments as well as an experiment to track a mixture of singing birds. Source code is available.
We address the problem of decomposing several consecutive sparse signals, such as audio time frames or image patches. A typical approach is to process each signal sequentially and independently, with an arbitrary sparsity level fixed for each signal. Here, we propose to process several frames simultaneously, allowing for more flexible sparsity patterns to be considered. We propose a multivariate sparse coding approach, where sparsity is enforced on average across several frames. We propose a Multivariate Iterative Hard Thresholding to solve this problem. The usefulness of the proposed approach is demonstrated on audio coding and denoising tasks. Experiments show that the proposed approach leads to better results when the signal contains both transients and tonal components.
* Birdsong often contains large amounts of rapid frequency modulation (FM). It is believed that the use or otherwise of FM is adaptive to the acoustic environment and also that there are specific social uses of FM such as trills in aggressive territorial encounters. Yet temporal fine detail of FM is often absent or obscured in standard audio signal analysis methods such as Fourier analysis or linear prediction. Hence, it is important to consider high-resolution signal processing techniques for analysis of FM in bird vocalizations. If such methods can be applied at big data scales, this offers a further advantage as large data sets become available. * We introduce methods from the signal processing literature which can go beyond spectrogram representations to analyse the fine modulations present in a signal at very short time-scales. Focusing primarily on the genus Phylloscopus, we investigate which of a set of four analysis methods most strongly captures the species signal encoded in birdsong. We evaluate this through a feature selection technique and an automatic classification experiment. In order to find tools useful in practical analysis of large data bases, we also study the computational time taken by the methods, and their robustness to additive noise and MP3 compression. * We find three methods which can robustly represent species-correlated FM attributes and can be applied to large data sets, and that the simplest method tested also appears to perform the best. We find that features representing the extremes of FM encode species identity supplementary to that captured in frequency features, whereas bandwidth features do not encode additional information. * FM analysis can extract information useful for bioacoustic studies, in addition to measures more commonly used to characterize vocalizations. Further, it can be applied efficiently across very large data sets and archives.
In a cognitive radio (CR) system, cooperative spectrum sensing (CSS) is the key to improving sensing performance in deep fading channels. In CSS networks, signals received at the secondary users (SUs) are sent to a fusion center to make a final decision of the spectrum occupancy. In this process, the presence of malicious users sending false sensing samples can severely degrade the performance of the CSS network. In this paper, with the compressive sensing (CS) technique being implemented at each SU, we build a CSS network with double sparsity property. A new malicious user detection scheme is proposed by utilizing the adaptive outlier pursuit (AOP) based low-rank matrix completion in the CSS network. In the proposed scheme, the malicious users are removed in the process of signal recovery at the fusion center. The numerical analysis of the proposed scheme is carried out and compared with an existing malicious user detection algorithm.
Source separation evaluation is typically a top-down process, starting with perceptual measures which capture fitness-for-purpose and followed by attempts to find physical (objective) measures that are predictive of the perceptual measures. In this paper, we take a contrasting bottom-up approach. We begin with the physical measures provided by the Blind Source Separation Evaluation Toolkit (BSS Eval) and we then look for corresponding perceptual correlates. This approach is known as psychophysics and has the distinct advantage of leading to interpretable, psychophysical models. We obtained perceptual similarity judgments from listeners in two experiments featuring vocal sources within musical mixtures. In the first experiment, listeners compared the overall quality of vocal signals estimated from musical mixtures using a range of competing source separation methods. In a loudness experiment, listeners compared the loudness balance of the competing musical accompaniment and vocal. Our preliminary results provide provisional validation of the psychophysical approach
This paper presents a low-complexity framework for acoustic scene classification (ASC). Most of the frameworks designed for ASC use convolutional neural networks (CNNs) due to their learning ability and improved performance compared to hand-engineered features. However, CNNs are resource hungry due to their large size and high computational complexity. Therefore, CNNs are difficult to deploy on resource constrained devices. This paper addresses the problem of reducing the computational complexity and memory requirement in CNNs. We propose a low-complexity CNN architecture, and apply pruning and quantization to further reduce the parameters and memory. We then propose an ensemble framework that combines various low-complexity CNNs to improve the overall performance. An experimental evaluation of the proposed framework is performed on the publicly available DCASE 2022 Task 1 that focuses on ASC. The proposed ensemble framework has approximately 60K parameters, requires 19M multiply-accumulate operations and improves the performance by approximately 2-4 percentage points compared to the DCASE 2022 Task 1 baseline network .
Identification and extraction of singing voice from within musical mixtures is a key challenge in source separation and machine audition. Recently, deep neural networks (DNN) have been used to estimate 'ideal' binary masks for carefully controlled cocktail party speech separation problems. However, it is not yet known whether these methods are capable of generalizing to the discrimination of voice and non-voice in the context of musical mixtures. Here, we trained a convolutional DNN (of around a billion parameters) to provide probabilistic estimates of the ideal binary mask for separation of vocal sounds from real-world musical mixtures. We contrast our DNN results with more traditional linear methods. Our approach may be useful for automatic removal of vocal sounds from musical mixtures for 'karaoke' type applications.
In real rooms, recorded speech usually contains reverberation, which degrades the quality and intelligibility of the speech. It has proven effective to use neural networks to estimate complex ideal ratio masks (cIRMs) using mean square error (MSE) loss for speech dereverberation. However, in some cases, when using MSE loss to estimate complex-valued masks, phase may have a disproportionate effect compared to magnitude. We propose a new weighted magnitude-phase loss function, which is divided into a magnitude component and a phase component, to train a neural network to estimate complex ideal ratio masks. A weight parameter is introduced to adjust the relative contribution of magnitude and phase to the overall loss. We find that our proposed loss function outperforms the regular MSE loss function for speech dereverberation.
Automatic Music Transcription (AMT) is concerned with the problem of producing the pitch content of a piece of music given a recorded signal. Many methods rely on sparse or low rank models, where the observed magnitude spectra are represented as a linear combination of dictionary atoms corresponding to individual pitches. Some of the most successful approaches use Non-negative Matrix Decomposition (NMD) or Factorization (NMF), which can be used to learn a dictionary and pitch activation matrix from a given signal. Here we introduce a further refinement of NMD in which we assume the transcription itself is approximately low rank. The intuition behind this approach is that the total number of distinct activation patterns should be relatively small since the pitch content between adjacent frames should be similar. A rank penalty is introduced into the NMD objective function and solved using an iterative algorithm based on Singular Value thresholding. We find that the low rank assumption leads to a significant increase in performance compared to NMD using β-divergence on a standard AMT dataset.
Acoustic monitoring of bird species is an increasingly important field in signal processing. Many available bird sound datasets do not contain exact timestamp of the bird call but have a coarse weak label instead. Traditional Non-negative Matrix Factorization (NMF) models are not well designed to deal with weakly labeled data. In this paper we propose a novel Masked Non-negative Matrix Factorization (Masked NMF) approach for bird detection using weakly labeled data. During dictionary extraction we introduce a binary mask on the activation matrix. In that way we are able to control which parts of dictionary are used to reconstruct the training data. We compare our method with conventional NMF approaches and current state of the art methods. The proposed method outperforms the NMF baseline and offers a parsimonious model for bird detection on weakly labeled data. Moreover, to our knowledge, the proposed Masked NMF achieved the best result among non-deep learning methods on a test dataset used for the recent Bird Audio Detection Challenge.
Summary form only given. The authors examine the goals of early stages of a perceptual system, before the signal reaches the cortex, and describe its operation in information-theoretic terms. The effects of receptor adaptation, lateral inhibition, and decorrelation can all be seen as part of an optimization of information throughput, given that available resources such as average power and maximum firing rates are limited. The authors suggest a modification to Gabor functions which improves their performance as band-pass filters.
While deep learning is undoubtedly the predominant learning technique across speech processing, it is still not widely used in health-based applications. The corpora available for health-style recognition problems are often small, both concerning the total amount of data available and the number of individuals present. The Bipolar Disorder corpus, used in the 2018 Audio/Visual Emotion Challenge, contains only 218 audio samples from 46 individuals. Herein, we present a multi-instance learning framework aimed at constructing more reliable deep learning-based models in such conditions. First, we segment the speech files into multiple chunks. However, the problem is that each of the individual chunks is weakly labelled, as they are annotated with the label of the corresponding speech file, but may not be indicative of that label. We then train the deep learning-based (ensemble) multi-instance learning model, aiming at solving such a weakly labelled problem. The presented results demonstrate that this approach can improve the accuracy of feedforward, recurrent, and convolutional neural nets on the 3-class mania classification tasks undertaken on the Bipolar Disorder corpus.
Automated audio captioning (AAC) aims to describe the content of an audio clip using simple sentences. Existing AAC methods are developed based on an encoder-decoder architecture that success is attributed to the use of a pre-trained CNN10 called PANNs as the encoder to learn rich audio representations. AAC is a highly challenging task due to its high-dimensional talent space involves audio of various scenarios. Existing methods only use the high-dimensional representation of the PANNs as the input of the decoder. However, the low-dimension representation may retain as much audio information as the high-dimensional representation may be neglected. In addition, although the high-dimensional approach may predict the audio captions by learning from existing audio captions, which lacks robustness and efficiency. To deal with these challenges, a fusion model which integrates low- and high-dimensional features AAC framework is proposed. In this paper, a new encoder-decoder framework is proposed called the Low- and High-Dimensional Feature Fusion (LHDFF) model for AAC. Moreover, in LHDFF, a new PANNs encoder is proposed called Residual PANNs (RPANNs) by fusing the low-dimensional feature from the intermediate convolution layer output and the high-dimensional feature from the final layer output of PANNs. To fully explore the information of the low- and high-dimensional fusion feature and high-dimensional feature respectively, we proposed dual transformer decoder structures to generate the captions in parallel. Especially, a probabilistic fusion approach is proposed that can ensure the overall performance of the system is improved by concentrating on the respective advantages of the two transformer decoders. Experimental results show that LHDFF achieves the best performance on the Clotho and AudioCaps datasets compared with other existing models
Poor workplace soundscapes can negatively impact productivity and employee satisfaction. While current regulations and physical acoustic treatments are beneficial, the potential of AI sound systems to enhance worker wellbeing is not fully explored. This paper investigates the use of AI-enabled sound technologies in workplaces, aiming to boost wellbeing and productivity through a soundscape approach while addressing user concerns. To evaluate these systems, we used scenario-based design and focus groups with knowledge workers from open-plan offices and those working remotely. Participants were presented with initial design concepts for AI sound analysis and control systems. This paper outlines user requirements and recommendations gathered from these focus groups, with a specific emphasis on soundscape personalisation and the creation of relevant datasets.
Automated audio captioning is a cross-modal translation task for describing the content of audio clips with natural language sentences. This task has attracted increasing attention and substantial progress has been made in recent years. Captions generated by existing models are generally faithful to the content of audio clips, however, these machine-generated captions are often deterministic (e.g., generating a fixed caption for a given audio clip), simple (e.g., using common words and simple grammar), and generic (e.g., generating the same caption for similar audio clips). When people are asked to describe the content of an audio clip, different people tend to focus on different sound events and describe an audio clip diversely from various aspects using distinct words and grammar. We believe that an audio captioning system should have the ability to generate diverse captions, either for a fixed audio clip, or across similar audio clips. To this end, we propose an adversarial training framework based on a conditional generative adversarial network (C-GAN) to improve diversity of audio captioning systems. A caption generator and two hybrid discriminators compete and are learned jointly, where the caption generator can be any standard encoder-decoder captioning model used to generate captions, and the hybrid discriminators assess the generated captions from different criteria, such as their naturalness and semantics. We conduct experiments on the Clotho dataset. The results show that our proposed model can generate captions with better diversity as compared to state-of-the-art methods.
This paper presents a novel approach to make convolutional neural networks (CNNs) efficient by reducing their computational cost and memory footprint. Even though large-scale CNNs show state-of-the-art performance in many tasks, high computational costs and the requirement of a large memory footprint make them resource-hungry. Therefore, deploying large-scale CNNs on resource-constrained devices poses significant challenges. To address this challenge, we propose to use quaternion CNNs, where quaternion algebra enables the memory footprint to be reduced. Furthermore, we investigate methods to reduce the memory footprint and computational cost further through pruning the quaternion CNNs. Experimental evaluation of the audio tagging task involving the classification of 527 audio events from AudioSet shows that the quaternion algebra and pruning reduce memory footprint by 90% and computational cost by 70% compared to the original CNN model while maintaining similar performance.
Deep generative models have recently achieved impressive performance in speech and music synthesis. However, compared to the generation of those domain-specific sounds, generating general sounds (such as siren, gunshots) has received less attention , despite their wide applications. In previous work, the SampleRNN method was considered for sound generation in the time domain. However, SampleRNN is potentially limited in capturing long-range dependencies within sounds as it only back-propagates through a limited number of samples. In this work, we propose a method for generating sounds via neural discrete time-frequency representation learning, conditioned on sound classes. This offers an advantage in efficiently modelling long-range dependencies and retaining local fine-grained structures within sound clips. We evaluate our approach on the UrbanSound8K dataset, compared to SampleRNN, with the performance metrics measuring the quality and diversity of generated sounds. Experimental results show that our method offers comparable performance in quality and significantly better performance in diversity.
Proximal methods are an important tool in signal processing applications, where many problems can be characterized by the minimization of an expression involving a smooth fitting term and a convex regularization term – for example the classic ℓ1-Lasso. Such problems can be solved using the relevant proximal operator. Here we consider the use of proximal operators for the ℓp-quasinorm where 0 ≤ p ≤ 1. Rather than seek a closed form solution, we develop an iterative algorithm using a Majorization-Minimization procedure which results in an inexact operator. Experiments on image denoising show that for p ≤ 1 the algorithm is effective in the high-noise scenario, outperforming the Lasso despite the inexactness of the proximal step.
Acoustic scene generation (ASG) is a task to generate waveforms for acoustic scenes. ASG can be used to generate audio scenes for movies and computer games. Recently, neural networks such as SampleRNN have been used for speech and music generation. However, ASG is more challenging due to its wide variety. In addition, evaluating a generative model is also difficult. In this paper, we propose to use a conditional SampleRNN model to generate acoustic scenes conditioned on the input classes. We also propose objective criteria to evaluate the quality and diversity of the generated samples based on classification accuracy. The experiments on the DCASE 2016 Task 1 acoustic scene data show that with the generated audio samples, a classification accuracy of 65:5% can be achieved compared to samples generated by a random model of 6:7% and samples from real recording of 83:1%. The performance of a classifier trained only on generated samples achieves an accuracy of 51:3%, as opposed to an accuracy of 6:7% with samples generated by a random model.
Imagine standing on a street corner in the city. With your eyes closed, you can hear and recognize a succession of sounds: cars passing by, people speaking, their footsteps when they walk by, and the continuously falling rain. Recognition of all these sounds and interpretation of the perceived scene as a city street soundscape comes naturally to humans. It is, however, the result of years of “training”: encountering and learning associations between the vast variety of sounds in everyday life, the sources producing these sounds, and the names given to them. Our everyday environment consists of many sound sources that create a complex mixture audio signal. Human auditory perception is highly specialized in segregating the sound sources and directing attention to the sound source of interest. This phenomenon is called cocktail party effect, as an analogy to being able to focus on a single conversation in a noisy room. Perception groups the spectro-temporal information in acoustic signals into auditory objects such that sounds or groups of sounds are perceived as a coherent whole [1]. This determines for example a complex sequence of sounds to be perceived as a single sound event instance, be it “bird singing” or “footsteps”. The goal of automatic sound event detection (SED) methods is to recognize what is happening in an audio signal and when it is happening. In practice, the goal is to recognize at what temporal instances different sounds are active within an audio signal.
The sources separated by most single channel audio source separation techniques are usually distorted and each separated source contains residual signals from the other sources. To tackle this problem, we propose to enhance the separated sources to decrease the distortion and interference between the separated sources using deep neural networks (DNNs). Two different DNNs are used in this work. The first DNN is used to separate the sources from the mixed signal. The second DNN is used to enhance the separated signals. To consider the interactions between the separated sources, we propose to use a single DNN to enhance all the separated sources together. To reduce the residual signals of one source from the other separated sources (interference), we train the DNN for enhancement discriminatively to maximize the dissimilarity between the predicted sources. The experimental results show that using discriminative enhancement decreases the distortion and interference between the separated sources
We derive expressions for the predicitive information rate (PIR) for the class of autoregressive Gaussian processes AR(N), both in terms of the prediction coefficients and in terms of the power spectral density. The latter result suggests a duality between the PIR and the multi-information rate for processes with mutually inverse power spectra (i.e. with poles and zeros of the transfer function exchanged). We investigate the behaviour of the PIR in relation to the multi-information rate for some simple examples, which suggest, somewhat counter-intuitively, that the PIR is maximised for very `smooth' AR processes whose power spectra have multiple poles at zero frequency. We also obtain results for moving average Gaussian processes which are consistent with the duality conjectured earlier. One consequence of this is that the PIR is unbounded for MA(N) processes.
Given binaural features as input, such as interaural level difference and interaural phase difference, Deep Neural Networks (DNNs) have been recently used to localize sound sources in a mixture of speech signals and/or noise, and to create time-frequency masks for the estimation of the sound sources in reverberant rooms. Here, we explore a more advanced system, where feed-forward DNNs are replaced by Convolutional Neural Networks (CNNs). In addition, the adjacent frames of each time frame (occurring before and after this frame) are used to exploit contextual information, thus improving the localization and separation for each source. The quality of the separation results is evaluated in terms of Signal to Distortion Ratio (SDR).
Acoustic event detection for content analysis in most cases relies on lots of labeled data. However, manually annotating data is a time-consuming task, which thus makes few annotated resources available so far. Unlike audio event detection, automatic audio tagging, a multi-label acoustic event classification task, only relies on weakly labeled data. This is highly desirable to some practical applications using audio analysis. In this paper we propose to use a fully deep neural network (DNN) framework to handle the multi-label classification task in a regression way. Considering that only chunk-level rather than frame-level labels are available, the whole or almost whole frames of the chunk were fed into the DNN to perform a multi-label regression for the expected tags. The fully DNN, which is regarded as an encoding function, can well map the audio features sequence to a multi-tag vector. A deep pyramid structure was also designed to extract more robust high-level features related to the target tags. Further improved methods were adopted, such as the Dropout and background noise aware training, to enhance its generalization capability for new audio recordings in mismatched environments. Compared with the conventional Gaussian Mixture Model (GMM) and support vector machine (SVM) methods, the proposed fully DNN-based method could well utilize the long-term temporal information with the whole chunk as the input. The results show that our approach obtained a 15% relative improvement compared with the official GMM-based method of DCASE 2016 challenge.
Current performance evaluation for audio source separation depends on comparing the processed or separated signals with reference signals. Therefore, common performance evaluation toolkits are not applicable to real-world situations where the ground truth audio is unavailable. In this paper, we propose a performance evaluation technique that does not require reference signals in order to assess separation quality. The proposed technique uses a deep neural network (DNN) to map the processed audio into its quality score. Our experiment results show that the DNN is capable of predicting the sources-to-artifacts ratio from the blind source separation evaluation toolkit [1] for singing-voice separation without the need for reference signals.
Significant improvement has been achieved in automated audio captioning (AAC) with recent models. However, these models have become increasingly large as their performance is enhanced. In this work, we propose a knowledge distillation (KD) framework for AAC. Our analysis shows that in the encoder-decoder based AAC models, it is more effective to dis-till knowledge into the encoder as compared with the decoder. To this end, we incorporate encoder-level KD loss into training, in addition to the standard supervised loss and sequence-level KD loss. We investigate two encoder-level KD methods, based on mean squared error (MSE) loss and contrastive loss, respectively. Experimental results demonstrate that contrastive KD is more robust than MSE KD, exhibiting superior performance in data-scarce situations. By leveraging audio-only data into training in the KD framework, our student model achieves competitive performance, with an inference speed that is 19 times faster.
We present a method to develop low-complexity convolutional neural networks (CNNs) for acoustic scene classification (ASC). The large size and high computational complexity of typical CNNs is a bottleneck for their deployment on resource-constrained devices. We propose a passive filter pruning framework, where a few convolutional filters from the CNNs are eliminated to yield compressed CNNs. Our hypothesis is that similar filters produce similar responses and give redundant information allowing such filters to be eliminated from the network. To identify similar filters, a cosine distance based greedy algorithm is proposed. A fine-tuning process is then performed to regain much of the performance lost due to filter elimination. To perform efficient fine-tuning, we analyze how the performance varies as the number of fine-tuning training examples changes. An experimental evaluation of the proposed framework is performed on the publicly available DCASE 2021 Task 1A baseline network trained for ASC. The proposed method is simple, reduces computations per inference by 27%, with 25% fewer parameters, with less than 1% drop in accuracy.
An algorithm for blind source separation (BSS) of the second heart sound (S2) into aortic and pulmonary components is proposed. It recovers aortic (A2) and pulmonary (P2) waveforms, as well as their relative delays, by solving an alternating optimization problem on the set of S2 sounds, without the use of auxiliary ECG or respiration phase measurement data. This unsupervised and data-driven approach assumes that the A2 and P2 components maintain the same waveform across heartbeats and that the relative delay between onset of the components varies according to respiration phase. The proposed approach is applied to synthetic heart sounds and to real-world heart sounds from 43 patients. It improves over two state-of-the-art BSS approaches by 10% normalized root mean-squared error in the reconstruction of aortic and pulmonary components using synthetic heart sounds, demonstrates robustness to noise, and recovery of splitting delays. The detection of pulmonary hypertension (PH) in a Brazilian population is demonstrated by training a classifier on three scalar features from the recovered A2 and P2 waveforms, and this yields an auROC of 0.76.
Environmental audio tagging is a newly proposed task to predict the presence or absence of a specific audio event in a chunk. Deep neural network (DNN) based methods have been successfully adopted for predicting the audio tags in the domestic audio scene. In this paper, we propose to use a convolutional neural network (CNN) to extract robust features from mel-filter banks (MFBs), spectrograms or even raw waveforms for audio tagging. Gated recurrent unit (GRU) based recurrent neural networks (RNNs) are then cascaded to model the long-term temporal structure of the audio signal. To complement the input information, an auxiliary CNN is designed to learn on the spatial features of stereo recordings. We evaluate our proposed methods on Task 4 (audio tagging) of the Detection and Classification of Acoustic Scenes and Events 2016 (DCASE 2016) challenge. Compared with our recent DNN-based method, the proposed structure can reduce the equal error rate (EER) from 0.13 to 0.11 on the development set. The spatial features can further reduce the EER to 0.10. The performance of the end-to-end learning on raw waveforms is also comparable. Finally, on the evaluation set, we get the state-of-the-art performance with 0.12 EER while the performance of the best existing system is 0.15 EER.
The DCASE Challenge 2016 contains tasks for Acoustic Scene Classification (ASC), Acoustic Event Detection (AED), and audio tagging. Since 2006, Deep Neural Networks (DNNs) have been widely applied to computer visions, speech recognition and natural language processing tasks. In this paper, we provide DNN baselines for the DCASE Challenge 2016. In Task 1 we obtained accuracy of 81.0% using Mel + DNN against 77.2% by using Mel Frequency Cepstral Coefficients (MFCCs) + Gaussian Mixture Model (GMM). In Task 2 we obtained F value of 12.6% using Mel + DNN against 37.0% by using Constant Q Transform (CQT) + Nonnegative Matrix Factorization (NMF). In Task 3 we obtained F value of 36.3% using Mel + DNN against 23.7% by using MFCCs + GMM. In Task 4 we obtained Equal Error Rate (ERR) of 18.9% using Mel + DNN against 20.9% by using MFCCs + GMM. Therefore the DNN improves the baseline in Task 1, 3, and 4, although it is worse than the baseline in Task 2. This indicates that DNNs can be successful in many of these tasks, but may not always perform better than the baselines.
We introduce a free and open dataset of 7690 audio clips sampled from the field-recording tag in the Freesound audio archive. The dataset is designed for use in research related to data mining in audio archives of field recordings / soundscapes. Audio is standardised, and audio and metadata are Creative Commons licensed. We describe the data preparation process, characterise the dataset descriptively, and illustrate its use through an auto-tagging experiment.
In this paper we present a new method for musical audio source separation, using the information from the musical score to supervise the decomposition process. An original framework using nonnegative matrix factorization (NMF) is presented, where the components are initially learnt on synthetic signals with temporal and harmonic constraints. A new dataset of multitrack recordings with manually aligned MIDI scores is created (TRIOS), and we compare our separation results with other methods from the literature using the BSS EVAL and PEASS evaluation toolboxes. The results show a general improvement of the BSS EVAL metrics for the various instrumental configurations used.
Deep neural networks (DNNs) are often used to tackle the single channel source separation (SCSS) problem by predicting time-frequency masks. The predicted masks are then used to separate the sources from the mixed signal. Different types of masks produce separated sources with different levels of distortion and interference. Some types of masks produce separated sources with low distortion, while other masks produce low interference between the separated sources. In this paper, a combination of different DNNs’ predictions (masks) is used for SCSS to achieve better quality of the separated sources than using each DNN individually. We train four different DNNs by minimizing four different cost functions to predict four different masks. The first and second DNNs are trained to approximate reference binary and soft masks. The third DNN is trained to predict a mask from the reference sources directly. The last DNN is trained similarly to the third DNN but with an additional discriminative constraint to maximize the differences between the estimated sources. Our experimental results show that combining the predictions of different DNNs achieves separated sources with better quality than using each DNN individually
Audio tagging aims to perform multi-label classification on audio chunks and it is a newly proposed task in the Detection and Classification of Acoustic Scenes and Events 2016 (DCASE 2016) challenge. This task encourages research efforts to better analyze and understand the content of the huge amounts of audio data on the web. The difficulty in audio tagging is that it only has a chunk-level label without a frame-level label. This paper presents a weakly supervised method to not only predict the tags but also indicate the temporal locations of the occurred acoustic events. The attention scheme is found to be effective in identifying the important frames while ignoring the unrelated frames. The proposed framework is a deep convolutional recurrent model with two auxiliary modules: an attention module and a localization module. The proposed algorithm was evaluated on the Task 4 of DCASE 2016 challenge. State-of-the-art performance was achieved on the evaluation set with equal error rate (EER) reduced from 0.13 to 0.11, compared with the convolutional recurrent baseline system.
This chapter discusses some algorithms for the use of non-negativity constraints in unmixing problems, including positive matrix factorization, nonnegative matrix factorization (NMF), and their combination with other unmixing methods such as non-negative independent component analysis and sparse non-negative matrix factorization. The 2D models can be naturally extended to multiway array (tensor) decompositions, especially non-negative tensor factorization (NTF) and non-negative tucker decomposition (NTD). The standard NMF model has been extended in various ways, including semi-NMF, multilayer NMF, tri-NMF, orthogonal NMF, nonsmooth NMF, and convolutive NMF. When gradient descent is a simple procedure, convergence can be slow, and the convergence can be sensitive to the step size. This can be overcome by applying multiplicative update rules, which have proved particularly popular in NMF. These multiplicative update rules have proved to be attractive since they are simple, do not need the selection of an update parameter, and their multiplicative nature, and non-negative terms on the RHS ensure that the elements cannot become negative. © 2010 Elsevier Ltd. All rights reserved.
This book presents computational methods for extracting the useful information from audio signals, collecting the state of the art in the field of sound event and scene analysis. The authors cover the entire procedure for developing such methods, ranging from data acquisition and labeling, through the design of taxonomies used in the systems, to signal processing methods for feature extraction and machine learning methods for sound recognition. The book also covers advanced techniques for dealing with environmental variation and multiple overlapping sound sources, and taking advantage of multiple microphones or other modalities. The book gives examples of usage scenarios in large media databases, acoustic monitoring, bioacoustics, and context-aware devices. Graphical illustrations of sound signals and their spectrographic representations are presented, as well as block diagrams and pseudocode of algorithms. Gives an overview of methods for computational analysis of sounds scenes and events, allowing those new to the field to become fully informed; Covers all the aspects of the machine learning approach to computational analysis of sound scenes and events, ranging from data capture and labeling process to development of algorithms; Includes descriptions of algorithms accompanied by a website from which software implementations can be downloaded, facilitating practical interaction with the techniques.
This study investigates into the perceptual consequence of phase change in conventional magnitude-based source separation. A listening test was conducted, where the participants compared three different source separation scenarios, each with two phase retrieval cases: phase from the original mix or from the target source. The participants’ responses regarding their similarity to the reference showed that 1) the difference between the mix phase and the perfect target phase was perceivable in the majority of cases with some song-dependent exceptions, and 2) use of the mix phase degraded the perceived quality even in the case of perfect magnitude separation. The findings imply that there is room for perceptual improvement by attempting correct phase reconstruction, in addition to achieving better magnitude-based separation.
Polyphonic sound event localization and detection (SELD), which jointly performs sound event detection (SED) and direction-of-arrival (DoA) estimation, detects the type and occurrence time of sound events as well as their corresponding DoA angles simultaneously. We study the SELD task from a multi-task learning perspective. Two open problems are addressed in this paper. Firstly, to detect overlapping sound events of the same type but with different DoAs, we propose to use a trackwise output format and solve the accompanying track permutation problem with permutation-invariant training. Multi-head self-attention is further used to separate tracks. Secondly, a previous finding is that, by using hard parameter-sharing, SELD suffers from a performance loss compared with learning the subtasks separately. This is solved by a soft parameter-sharing scheme. We term the proposed method as Event Independent Network V2 (EINV2), which is an improved version of our previously-proposed method and an end-to-end network for SELD. We show that our proposed EINV2 for joint SED and DoA estimation outperforms previous methods by a large margin, and has comparable performance to state-of-the-art ensemble models. Index Terms— Sound event localization and detection, direction of arrival, event-independent, permutation-invariant training, multi-task learning.
In supervised machine learning, the assumption that training data is labelled correctly is not always satisfied. In this paper, we investigate an instance of labelling error for classification tasks in which the dataset is corrupted with out-of-distribution (OOD) instances: data that does not belong to any of the target classes, but is labelled as such. We show that detecting and relabelling certain OOD instances, rather than discarding them, can have a positive effect on learning. The proposed method uses an auxiliary classifier, trained on data that is known to be in-distribution, for detection and relabelling. The amount of data required for this is shown to be small. Experiments are carried out on the FSDnoisy18k audio dataset, where OOD instances are very prevalent. The proposed method is shown to improve the performance of convolutional neural networks by a significant margin. Comparisons with other noise-robust techniques are similarly encouraging.
General-purpose audio tagging refers to classifying sounds that are of a diverse nature, and is relevant in many applications where domain-specific information cannot be exploited. The DCASE 2018 challenge introduces Task 2 for this very problem. In this task, there are a large number of classes and the audio clips vary in duration. Moreover, a subset of the labels are noisy. In this paper, we propose a system to address these challenges. The basis of our system is an ensemble of convolutional neural networks trained on log-scaled mel spectrograms. We use preprocessing and data augmentation methods to improve the performance further. To reduce the effects of label noise, two techniques are proposed: loss function weighting and pseudo-labeling. Experiments on the private test set of this task show that our system achieves state-of-the-art performance with a mean average precision score of 0.951
There is some uncertainty as to whether objective metrics for predicting the perceived quality of audio source separation are sufficiently accurate. This issue was investigated by employing a revised experimental methodology to collect subjective ratings of sound quality and interference of singing-voice recordings that have been extracted from musical mixtures using state-of-the-art audio source separation. A correlation analysis between the experimental data and the measures of two objective evaluation toolkits, BSS Eval and PEASS, was performed to assess their performance. The artifacts-related perceptual score of the PEASS toolkit had the strongest correlation with the perception of artifacts and distortions caused by singing-voice separation. Both the source-to-interference ratio of BSS Eval and the interference-related perceptual score of PEASS showed comparable correlations with the human ratings of interference.
This paper studies the disjointness of the time-frequency representations of simultaneously playing musical instruments. As a measure of disjointness, we use the approximate W-disjoint orthogonality as proposed by Yilmaz and Rickard [1], which (loosely speaking) measures the degree of overlap of different sources in the time-frequency domain. The motivation for this study is to find a maximally disjoint representation in order to facilitate the separation and recognition of musical instruments in mixture signals. The transforms investigated in this paper include the short-time Fourier transform (STFT), constant-Q transform, modified discrete cosine transform (MDCT), and pitch-synchronous lapped orthogonal transforms. Simulation results are reported for a database of polyphonic music where the multitrack data (instrument signals before mixing) were available. Absolute performance varies depending on the instrument source in question, but on the average MDCT with 93 ms frame size performed best.
Single-channel signal separation and deconvolution aims to separate and deconvolve individual sources from a single-channel mixture and is a challenging problem in which no prior knowledge of the mixing filters is available. Both individual sources and mixing filters need to be estimated. In addition, a mixture may contain non-stationary noise which is unseen in the training set. We propose a synthesizing-decomposition (S-D) approach to solve the single-channel separation and deconvolution problem. In synthesizing, a generative model for sources is built using a generative adversarial network (GAN). In decomposition, both mixing filters and sources are optimized to minimize the reconstruction error of the mixture. The proposed S-D approach achieves a peak-to-noise-ratio (PSNR) of 18.9 dB and 15.4 dB in image inpainting and completion, outperforming a baseline convolutional neural network PSNR of 15.3 dB and 12.2 dB, respectively and achieves a PSNR of 13.2 dB in source separation together with deconvolution, outperforming a convolutive non-negative matrix factorization (NMF) baseline of 10.1 dB.
Source separation is the task of separating an audio recording into individual sound sources. Source separation is fundamental for computational auditory scene analysis. Previous work on source separation has focused on separating particular sound classes such as speech and music. Much previous work requires mixtures and clean source pairs for training. In this work, we propose a source separation framework trained with weakly labelled data. Weakly labelled data only contains the tags of an audio clip, without the occurrence time of sound events. We first train a sound event detection system with AudioSet. The trained sound event detection system is used to detect segments that are most likely to contain a target sound event. Then a regression is learnt from a mixture of two randomly selected segments to a target segment conditioned on the audio tagging prediction of the target segment. Our proposed system can separate 527 kinds of sound classes from AudioSet within a single system. A U-Net is adopted for the separation system and achieves an average SDR of 5.67 dB over 527 sound classes in AudioSet.
Sound Event Localization and Detection (SELD) is a task that involves detecting different types of sound events along with their temporal and spatial information, specifically, detecting the classes of events and estimating their corresponding direction of arrivals at each frame. In practice, real-world sound scenes might be complex as they may contain multiple overlapping events. For instance, in DCASE challenges task 3, each clip may involve simultaneous occurrences of up to five events. To handle multiple overlapping sound events, current methods prefer multiple output branches to estimate each event, which increases the size of the models. Therefore, current methods are often difficult to be deployed on the edge of sensor networks. In this paper, we propose a method called Probabilistic Localization and Detection of Independent Sound Events with Transformers (PLDISET), which estimates numerous events by using one output branch. The method has three stages. First, we introduce the track generation module to obtain various tracks from extracted features. Then, these tracks are fed into two transformers for sound event detection (SED) and localization, respectively. Finally, one output system, including a linear Gaussian system and regression network, is used to estimate each track. We give the evaluation resn results of our model on DCASE 2023 Task 3 development dataset.
Several probabilistic models involving latent components have been proposed for modeling time-frequency (TF) representations of audio signals such as spectrograms, notably in the nonnegative matrix factorization (NMF) literature. Among them, the recent high-resolution NMF (HR-NMF) model is able to take both phases and local correlations in each frequency band into account, and its potential has been illustrated in applications such as source separation and audio inpainting. In this paper, HR-NMF is extended to multichannel signals and to convolutive mixtures. The new model can represent a variety of stationary and non-stationary signals, including autoregressive moving average (ARMA) processes and mixtures of damped sinusoids. A fast variational expectation-maximization (EM) algorithm is proposed to estimate the enhanced model. This algorithm is applied to piano signals, and proves capable of accurately modeling reverberation, restoring missing observations, and separating pure tones with close frequencies.
The authors address the problem of audio source separation, namely, the recovery of audio signals from recordings of mixtures of those signals. The sparse component analysis framework is a powerful method for achieving this. Sparse orthogonal transforms, in which only few transform coefficients differ significantly from zero, are developed; once the signal has been transformed, energy is apportioned from each transform coefficient to each estimated source, and, finally, the signal is reconstructed using the inverse transform. The overriding aim of this chapter is to demonstrate how this framework, as exemplified here by two different decomposition methods which adapt to the signal to represent it sparsely, can be used to solve different problems in different mixing scenarios. To address the instantaneous (neither delays nor echoes) and underdetermined (more sources than mixtures) mixing model, a lapped orthogonal transform is adapted to the signal by selecting a basis from a library of predetermined bases. This method is highly related to the windowing methods used in the MPEG audio coding framework. In considering the anechoic (delays but no echoes) and determined (equal number of sources and mixtures) mixing case, a greedy adaptive transform is used based on orthogonal basis functions that are learned from the observed data, instead of being selected from a predetermined library of bases. This is found to encode the signal characteristics, by introducing a feedback system between the bases and the observed data. Experiments on mixtures of speech and music signals demonstrate that these methods give good signal approximations and separation performance, and indicate promising directions for future research. © 2011, IGI Global.
We present a new class of digital audio effects which can automatically relate parameter values to the tempo of a musical input in real-time. Using a beat tracking system as the front end, we demonstrate a tempo-dependent delay effect and a set of beat-synchronous low frequency oscillator (LFO) effects including auto-wah, tremolo and vibrato. The effects show better performance than might be expected as they are blind to certain beat tracker errors. All effects are implemented as VST plug-ins which operate in real-time, enabling their use both in live musical performance and the off-line modification of studio recordings.
For the task of sound source recognition, we introduce a novel data set based on 6.8 hours of domestic environment audio recordings. We describe our approach of obtaining annotations for the recordings. Further, we quantify agreement between obtained annotations. Finally, we report baseline results for sound source recognition using the obtained dataset. Our annotation approach associates each 4-second excerpt from the audio recordings with multiple labels, on a set of 7 labels associated with sound sources in the acoustic environment. With the aid of 3 human annotators, we obtain 3 sets of multi-label annotations, for 4378 4-second audio excerpts. We evaluate agreement between annotators by computing Jaccard indices between sets of label assignments. Observing varying levels of agreement across labels, with a view to obtaining a representation of ‘ground truth’ in annotations, we refine our dataset to obtain a set of multi-label annotations for 1946 audio excerpts. For the set of 1946 annotated audio excerpts, we predict binary label assignments using Gaussian mixture models estimated on MFCCs. Evaluated using the area under receiver operating characteristic curves, across considered labels we observe performance scores in the range 0.76 to 0.98
Universal source separation (USS) is a fundamental research task for computational auditory scene analysis, which aims to separate mono recordings into individual source tracks. There are three potential challenges awaiting the solution to the audio source separation task. First, previous audio source separation systems mainly focus on separating one or a limited number of specific sources. There is a lack of research on building a unified system that can separate arbitrary sources via a single model. Second, most previous systems require clean source data to train a separator, while clean source data are scarce. Third, there is a lack of USS system that can automatically detect and separate active sound classes in a hierarchical level. To use large-scale weakly labeled/unlabeled audio data for audio source separation, we propose a universal audio source separation framework containing: 1) an audio tagging model trained on weakly labeled data as a query net; and 2) a conditional source separation model that takes query net outputs as conditions to separate arbitrary sound sources. We investigate various query nets, source separation models, and training strategies and propose a hierarchical USS strategy to automatically detect and separate sound classes from the AudioSet ontology. By solely leveraging the weakly labelled AudioSet, our USS system is successful in separating a wide variety of sound classes, including sound event separation, music source separation, and speech enhancement. The USS system achieves an average signal-to-distortion ratio improvement (SDRi) of 5.57 dB over 527 sound classes of AudioSet; 10.57 dB on the DCASE 2018 Task 2 dataset; 8.12 dB on the MUSDB18 dataset; an SDRi of 7.28 dB on the Slakh2100 dataset; and an SSNR of 9.00 dB on the voicebank-demand dataset. We release the source code at https://github.com/bytedance/uss
We address the problem of recovering a sparse signal from clipped or quantized measurements. We show how these two problems can be formulated as minimizing the distance to a convex feasibility set, which provides a convex and differentiable cost function. We then propose a fast iterative shrinkage/thresholding algorithm that minimizes the proposed cost, which provides a fast and efficient algorithm to recover sparse signals from clipped and quantized measurements.
Recently, there has been increasing interest in building efficient audio neural networks for on-device scenarios. Most existing approaches are designed to reduce the size of audio neural networks using methods such as model pruning. In this work, we show that instead of reducing model size using complex methods, eliminating the temporal redundancy in the input audio features (e.g., mel-spectrogram) could be an effective approach for efficient audio classification. To do so, we proposed a family of simple pooling front-ends (SimPFs) which use simple non-parametric pooling operations to reduce the redundant information within the mel-spectrogram. We perform extensive experiments on four audio classification tasks to evaluate the performance of SimPFs. Experimental results show that SimPFs can achieve a reduction in more than half of the number of floating point operations (FLOPs) for off-the-shelf audio neural networks, with negligible degradation or even some improvements in audio classification performance.
Cardiovascular disease is one of the leading factors for death cause of human beings. In the past decade, heart sound classification has been increasingly studied for its feasibility to develop a non-invasive approach to monitor a subject’s health status. Particularly, relevant studies have benefited from the fast development of wearable devices and machine learning techniques. Nevertheless, finding and designing efficient acoustic properties from heart sounds is an expensive and time-consuming task. It is known that transfer learning methods can help extract higher representations automatically from the heart sounds without any human domain knowledge. However, most existing studies are based on models pre-trained on images, which may not fully represent the characteristics inherited from audio. To this end, we propose a novel transfer learning model pretrained on large scale audio data for a heart sound classification task. In this study, the PhysioNet CinC Challenge Dataset is used for evaluation. Experimental results demonstrate that, our proposed pre-trained audio models can outperform other popular models pre-trained by images by achieving the highest unweighted average recall at 89.7 %.
Sound event detection (SED) aims to detect when and recognize what sound events happen in an audio clip. Many supervised SED algorithms rely on strongly labelled data which contains the onset and offset annotations of sound events. However, many audio tagging datasets are weakly labelled, that is, only the presence of the sound events is known, without knowing their onset and offset annotations. In this paper, we propose a time-frequency (T-F) segmentation framework trained on weakly labelled data to tackle the sound event detection and separation problem. In training, a segmentation mapping is applied on a T-F representation, such as log mel spectrogram of an audio clip to obtain T-F segmentation masks of sound events. The T-F segmentation masks can be used for separating the sound events from the background scenes in the time-frequency domain. Then a classification mapping is applied on the T-F segmentation masks to estimate the presence probabilities of the sound events. We model the segmentation mapping using a convolutional neural network and the classification mapping using a global weighted rank pooling (GWRP). In SED, predicted onset and offset times can be obtained from the T-F segmentation masks. As a byproduct, separated waveforms of sound events can be obtained from the T-F segmentation masks. We remixed the DCASE 2018 Task 1 acoustic scene data with the DCASE 2018 Task 2 sound events data. When mixing under 0 dB, the proposed method achieved F1 scores of 0.534, 0.398 and 0.167 in audio tagging, frame-wise SED and event-wise SED, outperforming the fully connected deep neural network baseline of 0.331, 0.237 and 0.120, respectively. In T-F segmentation, we achieved an F1 score of 0.218, where previous methods were not able to do T-F segmentation.
Deep neural networks with convolutional layers usually process the entire spectrogram of an audio signal with the same time-frequency resolutions, number of filters, and dimensionality reduction scale. According to the constant-Q transform, good features can be extracted from audio signals if the low frequency bands are processed with high frequency resolution filters and the high frequency bands with high time resolution filters. In the spectrogram of a mixture of singing voices and music signals, there is usually more information about the voice in the low frequency bands than the high frequency bands. These raise the need for processing each part of the spectrogram differently. In this paper, we propose a multi-band multi-resolution fully convolutional neural network (MBR-FCN) for singing voice separation. The MBR-FCN processes the frequency bands that have more information about the target signals with more filters and smaller dimensionality reduction scale than the bands with less information. Furthermore, the MBR-FCN processes the low frequency bands with high frequency resolution filters and the high frequency bands with high time resolution filters. Our experimental results show that the proposed MBRFCN with very few parameters achieves better singing voice separation performance than other deep neural networks.
Envelope generator (EG) and Low Frequency Oscillator (LFO) parameters give a compact representation of audio pitch envelopes. By estimating these parameters from audio per-note, they could be used as part of an audio coding scheme. Recordings of various instruments and articulations were examined, and pitch envelopes found. Using an evolutionary algorithm, EG and LFO parameters for the envelopes were estimated. The resulting estimated envelopes are compared to both the original envelope, and to a fixed-pitch estimate. Envelopes estimated using EG+LFO can closely represent the envelope from the original audio and provide a more accurate estimate than the mean pitch.
For learning-based sound event localization and detection (SELD) methods, different acoustic environments in the training and test sets may result in large performance differences in the validation and evaluation stages. Different environments, such as different sizes of rooms, different reverberation times, and different background noise, may be reasons for a learning-based system to fail. On the other hand, acquiring annotated spatial sound event samples, which include onset and offset time stamps, class types of sound events, and direction-of-arrival (DOA) of sound sources is very expensive. In addition, deploying a SELD system in a new environment often poses challenges due to time-consuming training and fine-tuning processes. To address these issues, we propose Meta-SELD, which applies meta-learning methods to achieve fast adaptation to new environments. More specifically, based on Model Agnostic Meta-Learning (MAML), the proposed Meta-SELD aims to find good meta-initialized parameters to adapt to new environments with only a small number of samples and parameter updating iterations. We can then quickly adapt the meta-trained SELD model to unseen environments. Our experiments compare fine-tuning methods from pre-trained SELD models with our Meta-SELD on the Sony-TAU Realistic Spatial Soundscapes 2023 (STARSSS23) dataset. The evaluation results demonstrate the effectiveness of Meta-SELD when adapting to new environments.
We model expressive timing for a phrase in performed classical music as being dependent on two factors: the expressive timing in the previous phrase and the position of the phrase within the piece. We present a model selection test for evaluating candidate models that assert different dependencies for deciding the Cluster of Expressive Timing (CET) for a phrase. We use cross entropy and Kullback Leibler (KL) divergence to evaluate the resulting models: with these criteria we find that both the expressive timing in the previous phrase and the position of the phrase in the music score affect expressive timing in a phrase. The results show that the expressive timing in the previous phrase has a greater effect on timing choices than the position of the phrase, as the phrase position only impacts the choice of expressive timing in combination with the choice of expressive timing in the previous phrase.
Object-based coding of audio represents the signal as a parameter stream for a set of sound-producing objects. Encoding in this manner can provide a resolution-free representation of an audio signal. Given a robust estimation of the object-parameters and a multi-resolution synthesis engine, the signal can be "intelligently" upsampled, extending the bandwidth and getting best use out of a high-resolution signal-chain. We present some initial findings on extending bandwidth using harmonic models.
Sub-band methods are often used to address the problem of convolutive blind speech separation, as they offer the computational advantage of approximating convolutions by multiplications. The computational load, however, often remains quite high, because separation is performed on several sub-bands. In this paper, we exploit the well known fact that the high frequency content of speech signals typically conveys little information, since most of the speech power is found in frequencies up to 4kHz, and consider separation only in frequency bands below a certain threshold. We investigate the effect of changing the threshold, and find that separation performed only in the low frequencies can lead to the recovered signals being similar in quality to those extracted from all frequencies.
We introduce Harmonic Motion, a free open source toolkit for artists, musicians and designers working with gestural data. Extracting musically useful features from captured gesture data can be challenging, with projects often requiring bespoke processing techniques developed through iterations of tweaking equations involving a number of constant values – sometimes referred to as ‘magic numbers’. Harmonic Motion provides a robust interface for rapid prototyping of patches to process gestural data and a framework through which approaches may be encapsulated, reused and shared with others. In addition, we describe our design process in which both personal experience and a survey of potential users informed a set of specific goals for the software.
The Melody Triangle is an interface for the discovery of melodic materials, where the input positions within a triangle directly map to information theoretic properties of the output. A model of human expectation and surprise in the perception of music, information dynamics, is used to 'map out' a musical generative system's parameter space. This enables a user to explore the possibilities afforded by a generative algorithm, in this case Markov chains, not by directly selecting parameters, but by specifying the subjective predictability of the output sequence. We describe some of the relevant ideas from information dynamics and how the Melody Triangle is defined in terms of these. We describe its incarnation as a screen based performance tool and compositional aid for the generation of musical textures; the users control at the abstract level of randomness and predictability, and some pilot studies carried out with it. We also briefly outline a multi-user installation, where collaboration in a performative setting provides a playful yet informative way to explore expectation and surprise in music, and a forthcoming mobile phone version of the Melody Triangle.
Particle filters (PFs) have been widely used in speaker tracking due to their capability in modeling a non-linear process or a non-Gaussian environment. However, particle filters are limited by several issues. For example, pre-defined handcrafted measurements are often used which can limit the model performance. In addition, the transition and update models are often preset which make PF less flexible to be adapted to different scenarios. To address these issues, we propose an end-to-end differentiable particle filter framework by employing the multi-head attention to model the long-range dependencies. The proposed model employs the self-attention as the learned transition model and the cross-attention as the learned update model. To our knowledge, this is the first proposal of combining particle filter and transformer for speaker tracking, where the measurement extraction, transition and update steps are integrated into an end-to-end architecture. Experimental results show that the proposed model achieves superior performance over the recurrent baseline models.
Many performers of novel musical instruments find it diffi- cult to engage audiences beyond those in the field. Previous research points to a failure to balance complexity with usability, and a loss of transparency due to the detachment of the controller and sound generator. The issue is often exacerbated by an audiences lack of prior exposure to the instrument and its workings. However, we argue that there is a conflict underlying many novel musical instruments in that they are intended to be both a tool for creative expression and a creative work of art in themselves, resulting in incompatible requirements. By considering the instrument, the composition and the performance together as a whole with careful consideration of the rate of learning demanded of the audience, we propose that a lack of transparency can become an asset rather than a hindrance. Our approach calls for not only controller and sound generator to be designed in sympathy with each other, but composition, performance and physical form too. Identifying three design principles, we illustrate this approach with the Serendiptichord, a wearable instrument for dancers created by the authors.
Non-negative Matrix Factorisation (NMF) is a commonly used tool in many musical signal processing tasks, including Automatic Music Transcription (AMT). However unsupervised NMF is seen to be problematic in this context, and harmonically constrained variants of NMF have been proposed. While useful, the harmonic constraints may be constrictive in mixed signals. We have previously observed that recovery of overlapping signal elements using NMF is improved through introduction of a sparse coding step, and propose here the incorporation of a sparse coding step using the Hellinger distance into a NMF algorithm. Improved AMT results for unsupervised NMF are reported.
We propose a convolutional neural network (CNN) model based on an attention pooling method to classify ten different acoustic scenes, participating in the acoustic scene classification task of the IEEE AASPChallengeonDetectionandClassificationofAcousticScenes and Events (DCASE 2018), which includes data from one device (subtask A) and data from three different devices (subtask B). The log mel spectrogram images of the audio waves are first forwarded to convolutional layers, and then fed into an attention pooling layer to reduce the feature dimension and achieve classification. From attention perspective, we build a weighted evaluation of the features, instead of simple max pooling or average pooling. On the official development set of the challenge, the best accuracy of subtask A is 72.6%,whichisanimprovementof12.9%whencomparedwiththe official baseline (p < .001 in a one-tailed z-test). For subtask B, the best result of our attention-based CNN is a significant improvement of the baseline as well, in which the accuracies are 71.8%, 58.3%, and 58.3% for the three devices A to C (p < .001 for device A, p < .01 for device B, and p < .05 for device C).
The Melody Triangle is a smartphone application for Android that lets users easily create musical patterns and textures. The user creates melodies by specifying positions within a triangle, and these positions correspond to the information theoretic properties of generated musical sequences. A model of human expectation and surprise in the perception of music, information dynamics, is used to 'map out' a musical generative system's parameter space, in this case Markov chains. This enables a user to explore the possibilities afforded by Markov chains, not by directly selecting their parameters, but by specifying the subjective predictability of the output sequence. As users of the app find melodies and patterns they like, they are encouraged to press a 'like' button, where their setting are uploaded to our servers for analysis. Collecting the 'liked' settings of many users worldwide will allow us to elicit trends and commonalities in aesthetic preferences across users of the app, and to investigate how these might relate to the informationdynamic model of human expectation and surprise. We outline some of the relevant ideas from information dynamics and how the Melody Triangle is defined in terms of these. We then describe the Melody Triangle mobile application, how it is being used to collect research data and how the collected data will be evaluated.
In computational musicology research, clustering is a common approach to the analysis of expression. Our research uses mathematical model selection criteria to evaluate the performance of clustered and non-clustered models applied to intra-phrase tempo variations in classical piano performances. By engaging different standardisation methods for the tempo variations and engaging different types of covariance matrices, multiple pieces of performances are used for evaluating the performance of candidate models. The results of tests suggest that the clustered models perform better than the non-clustered models and the original tempo data should be standardised by the mean of tempo within a phrase.
We present a robust method for the detection of the first and second heart sounds (s1 and s2), without ECG reference, based on a music beat tracking algorithm. An intermediate representation of the input signal is first calculated by using an onset detection function based on complex spectral difference. A music beat tracking algorithm is then used to determine the location of the first heart sound. The beat tracker works in two steps, it first calculates the beat period and then finds the temporal beat alignment. Once the first sound is detected, inverse Gaussian weights are applied to the onset function on the detected positions and the algorithm is run again to find the second heart sound. At the last step s1 and s2 labels are attributed to the detected sounds. The algorithm was evaluated in terms of location accuracy as well as sensitivity and specificity and the results showed good results even in the presence of murmurs or noisy signals.
Binaural features of interaural level difference and interaural phase difference have proved to be very effective in training deep neural networks (DNNs), to generate timefrequency masks for target speech extraction in speech-speech mixtures. However, effectiveness of binaural features is reduced in more common speech-noise scenarios, since the noise may over-shadow the speech in adverse conditions. In addition, the reverberation also decreases the sparsity of binaural features and therefore adds difficulties to the separation task. To address the above limitations, we highlight the spectral difference between speech and noise spectra and incorporate the log-power spectra features to extend the DNN input. Tested on two different reverberant rooms at different signal to noise ratios (SNR), our proposed method shows advantages over the baseline method using only binaural features in terms of signal to distortion ratio (SDR) and Short-Time Perceptual Intelligibility (STOI).
Research work on automatic speech recognition and automatic music transcription has been around for several decades, supported by dedicated conferences or conference sessions. However, while individual researchers have been working on recognition of more general environmental sounds, until ten years ago there were no regular workshops or conference sessions where this research, or its researchers, could be found. There was also little available data for researchers to work on or to benchmark their work. In this paper we will outline how a new research community working on Detection and Classification of Acoustic Scenes and Events (DCASE) has grown over the last ten years, from two challenges on acoustic scene classification and sound event detection with a small workshop poster session, to an annual data challenge with six tasks and a dedicated annual workshop, attracting hundreds of delegates and strong industry interest. We will also describe how the analysis methods have evolved, from mel frequency cepstral coefficients (MFCCs) or cochelograms classified by support vector machines (SVMs) or hidden Markov models (HMMs), to deep learning methods such as transfer learning, transformers, and self-supervised learning. We will finish by suggesting some potential future directions for automatic sound recognition and the DCASE community.
Environmental audio tagging aims to predict only the presence or absence of certain acoustic events in the interested acoustic scene. In this paper we make contributions to audio tagging in two parts, respectively, acoustic modeling and feature learning. We propose to use a shrinking deep neural network (DNN) framework incorporating unsupervised feature learning to handle the multi-label classification task. For the acoustic modeling, a large set of contextual frames of the chunk are fed into the DNN to perform a multi-label classification for the expected tags, considering that only chunk (or utterance) level rather than frame-level labels are available. Dropout and background noise aware training are also adopted to improve the generalization capability of the DNNs. For the unsupervised feature learning, we propose to use a symmetric or asymmetric deep de-noising auto-encoder (syDAE or asyDAE) to generate new data-driven features from the logarithmic Mel-Filter Banks (MFBs) features. The new features, which are smoothed against background noise and more compact with contextual information, can further improve the performance of the DNN baseline. Compared with the standard Gaussian Mixture Model (GMM) baseline of the DCASE 2016 audio tagging challenge, our proposed method obtains a significant equal error rate (EER) reduction from 0.21 to 0.13 on the development set. The proposed asyDAE system can get a relative 6.7% EER reduction compared with the strong DNN baseline on the development set. Finally, the results also show that our approach obtains the state-of-the-art performance with 0.15 EER on the evaluation set of the DCASE 2016 audio tagging task while EER of the first prize of this challenge is 0.17.
Abstract Multilayer neural networks trained with backpropagation are in general not robust against the loss of a hidden neuron. In this paper we define a form of robustness called 1-node robustness and propose methods to improve it. One approach is based on a modification of the error function by the addition of a "robustness error". It leads to more robust networks but at the cost of a reduced accuracy. A second approach, "pruning-and-duplication", consists of duplicating the neurons whose loss is the most damaging for the network. Pruned neurons are used for the duplication. This procedure leads to robust and accurate networks at low computational cost. It may also prove benefical for generalisation. Both methods are evaluated on the XOR function.
Musical noise is a recurrent issue that appears in spectral techniques for denoising or blind source separation. Due to localised errors of estimation, isolated peaks may appear in the processed spectrograms, resulting in annoying tonal sounds after synthesis known as “musical noise”. In this paper, we propose a method to assess the amount of musical noise in an audio signal, by characterising the impact of these artificial isolated peaks on the processed sound. It turns out that because of the constraints between STFT coefficients, the isolated peaks are described as time-frequency “spots” in the spectrogram of the processed audio signal. The quantification of these “spots”, achieved through the adaptation of a method for localisation of significant STFT regions, allows for an evaluation of the amount of musical noise. We believe that this will pave the way to an objective measure and a better understanding of this phenomenon.
In cognitive radio networks, cooperative spectrum sensing (CSS) has been a promising approach to improve sensing performance by utilizing spatial diversity of participating secondary users (SUs). In current CSS networks, all cooperative SUs are assumed to be honest and genuine. However, the presence of malicious users sending out dishonest data can severely degrade the performance of CSS networks. In this paper, a framework with high detection accuracy and low costs of data acquisition at SUs is developed, with the purpose of mitigating the influences of malicious users. More specifically, a low-rank matrix completion based malicious user detection framework is proposed. In the proposed framework, in order to avoid requiring any prior information about the CSS network, a rank estimation algorithm and an estimation strategy for the number of corrupted channels are proposed. Numerical results show that the proposed malicious user detection framework achieves high detection accuracy with lower data acquisition costs in comparison with the conventional approach. After being validated by simulations, the proposed malicious user detection framework is tested on the real-world signals over TV white space spectrum.
We consider the extension of the greedy adaptive dictionary learning algorithm that we introduced previously, to applications other than speech signals. The algorithm learns a dictionary of sparse atoms, while yielding a sparse representation for the speech signals. We investigate its behavior in the analysis of music signals, and propose a different dictionary learning approach that can be applied to large data sets. This facilitates the application of the algorithm to problems that generate large amounts of data, such as multimedia of multi-channel application areas.
Research in audio source separation has progressed a long way, producing systems that are able to approximate the component signals of sound mixtures. In recent years, many efforts have focused on learning time-frequency masks that can be used to filter a monophonic signal in the frequency domain. Using current web audio technologies, time-frequency masking can be implemented in a web browser in real time. This allows applying source separation techniques to arbitrary audio streams, such as internet radios, depending on cross-domain security configurations. While producing good quality separated audio from monophonic music mixtures is still challenging, current methods can be applied to remixing scenarios, where part of the signal is emphasized or deemphasized. This paper describes a system for remixing musical audio on the web by applying time-frequency masks estimated using deep neural networks. Our example prototype, implemented in client-side Javascript, provides reasonable quality results for small modifications.
Dictionary Learning (DL) has seen widespread use in signal processing and machine learning. Given a data set, DL seeks to find a so-called ‘dictionary’ such that the data can be well represented by a sparse linear combination of dictionary elements. The representational power of DL may be extended by the use of kernel mappings, which implicitly map the data to some high dimensional feature space. In Kernel DL we wish to learn a dictionary in this underlying high-dimensional feature space, which can often model the data more accurately than learning in the original space. Kernel DL is more challenging than the linear case however since we no longer have access to the dictionary atoms directly – only their relationship to the data via the kernel matrix. One strategy is therefore to represent the dictionary as a linear combination of the input data whose coefficients can be learned during training [1], relying on the fact that any optimal dictionary lies in the span of the data. A difficulty in Kernel DL is that given a data set of size N, the full (N ×N) kernel matrix needs to be manipulated at each iteration and dealing with such a large dense matrix can be extremely slow for big datasets. Here, we impose an additional constraint of sparsity on the coefficients so that the learned dictionary is given by a sparse linear combination of the input data. This greatly speeds up learning, and furthermore the speed-up is greater for larger datasets and can be tuned via a dictionary-sparsity parameter. The proposed approach thus combines Kernel DL with the ‘double sparse’ DL model [2] in which the learned dictionary is given by a sparse reconstruction over some base dictionary (in this case, the data itself). We investigate the use of sparse Kernel DL as a feature learning step for a music transcription task and compare it to another Kernel DL approach based on the K-SVD algorithm [1] (which doesnt lead to sparse dictionaries in general), in terms of computation-time and performance. Initial experiments show that Sparse Kernel DL is significantly faster than the non-sparse Kernel DL approach (6× to 8× speed-up depending on the size of the training data and the sparsity level) while leading to similar performance.
We describe a novel musical instrument designed for use in contemporary dance performance. This instrument, the Serendiptichord, takes the form of a headpiece plus associated pods which sense movements of the dancer, together with associated audio processing software driven by the sensors. Movements such as translating the pods or shaking the trunk of the headpiece cause selection and modification of sampled sounds. We discuss how we have closely integrated physical form, sensor choice and positioning and software to avoid issues which otherwise arise with disconnection of the innate physical link between action and sound, leading to an instrument that non-musicians (in this case, dancers) are able to enjoy using immediately.
We address the problem of sparse signal reconstruction from a few noisy samples. Recently, a Covariance-Assisted Matching Pursuit (CAMP) algorithm has been proposed, improving the sparse coefficient update step of the classic Orthogonal Matching Pursuit (OMP) algorithm. CAMP allows the a-priori mean and covariance of the non-zero coefficients to be considered in the coefficient update step. In this paper, we analyze CAMP, which leads to a new interpretation of the update step as a maximum-a-posteriori (MAP) estimation of the non-zero coefficients at each step. We then propose to leverage this idea, by finding a MAP estimate of the sparse reconstruction problem, in a greedy OMP-like way. Our approach allows the statistical dependencies between sparse coefficients to be modelled, while keeping the practicality of OMP. Experiments show improved performance when reconstructing the signal from a few noisy samples.
The first generation of three-dimensional Electromagnetic Articulography devices (Carstens AG500) suffered from occasional critical tracking failures. Although now superseded by new devices, the AG500 is still in use in many speech labs and many valuable data sets exist. In this study we investigate whether deep neural networks (DNNs) can learn the mapping function from raw voltage amplitudes to sensor positions based on a comprehensive movement data set. This is compared to arriving sample by sample at individual position values via direct optimisation as used in previous methods. We found that with appropriate hyperparameter settings a DNN was able to approximate the mapping function with good accuracy, leading to a smaller error than the previous methods, but that the DNN-based approach was not able to solve the tracking problem completely.
In this paper, a method for separation of harmonic and percussive elements in music recordings is presented. The proposed method is based on a simple spectral peak detection step followed by a phase expectation analysis that discriminates between harmonic and percussive components. The proposed method was tested on a database of 10 audio tracks and has shown superior results to the reference state-of-the-art approach.
In this paper, we present a deep neural network (DNN)-based acoustic scene classification framework. Two hierarchical learning methods are proposed to improve the DNN baseline performance by incorporating the hierarchical taxonomy information of environmental sounds. Firstly, the parameters of the DNN are initialized by the proposed hierarchical pre-training. Multi-level objective function is then adopted to add more constraint on the cross-entropy based loss function. A series of experiments were conducted on the Task1 of the Detection and Classification of Acoustic Scenes and Events (DCASE) 2016 challenge. The final DNN-based system achieved a 22.9% relative improvement on average scene classification error as compared with the Gaussian Mixture Model (GMM)-based benchmark system across four standard folds.
At the University of Surrey (Guildford, UK), we have brought together research groups in different disciplines, with a shared interest in audio, to work on a range of collaborative research projects. In the Centre for Vision, Speech and Signal Processing (CVSSP) we focus on technologies for machine perception of audio scenes; in the Institute of Sound Recording (IoSR) we focus on research into human perception of audio quality; the Digital World Research Centre (DWRC) focusses on the design of digital technologies; while the Centre for Digital Economy (CoDE) focusses on new business models enabled by digital technology. This interdisciplinary view, across different traditional academic departments and faculties, allows us to undertake projects which would be impossible for a single research group. In this poster we will present an overview of some of these interdisciplinary projects, including projects in spatial audio, sound scene and event analysis, and creative commons audio.
This paper is devoted to enhancing rapid decision-making and identification of lactobacilli from dental plaque using statistical and neural network methods. Current techniques of identification such as clustering and principal component analysis are discussed with respect to the field of bacterial taxonomy. Decision-making using multilayer perceptron neural network and Kohonen self-organizing feature map is highlighted. Simulation work and corresponding results are presented with main emphasis on neural network convergence and identification capability using resubstitution, leave-one-out and cross validation techniques. Rapid analyses on two separate sets of bacterial data from dental plaque revealed accuracy of more than 90% in the identification process. The risk of misdiagnosis was estimated at 14% worst case. Test with unknown strains yields close correlation to cluster dendograms. The use of the AXEON VindAX simulator indicated close correlations of the results. The paper concludes that artificial neural networks are suitable for use in the rapid identification of dental bacteria.
We describe an information-theoretic approach to the analysis of music and other sequential data, which emphasises the predictive aspects of perception, and the dynamic process of forming and modifying expectations about an unfolding stream of data, characterising these using the tools of information theory: entropies, mutual informations, and related quantities. After reviewing the theoretical foundations, we discuss a few emerging areas of application, including musicological analysis, real-time beat-tracking analysis, and the generation of musical materials as a cognitively-informed compositional aid. © 2012 IEEE.
Automated audio captioning is a cross-modal translation task that aims to generate natural language descriptions for given audio clips. This task has received increasing attention with the release of freely available datasets in recent years. The problem has been addressed predominantly with deep learning techniques. Numerous approaches have been proposed, such as investigating different neural network architectures, exploiting auxiliary information such as keywords or sentence information to guide caption generation, and employing different training strategies, which have greatly facilitated the development of this field. In this paper, we present a comprehensive review of the published contributions in automated audio captioning, from a variety of existing approaches to evaluation metrics and datasets. We also discuss open challenges and envisage possible future research directions.
Polyphonic sound event localization and detection (SELD) aims at detecting types of sound events with corresponding temporal activities and spatial locations. In this paper, a track-wise ensemble event independent network with a novel data augmentation method is proposed. The proposed model is based on our previous proposed Event-Independent Network V2 and is extended by conformer blocks and dense blocks. The track-wise ensemble model with track-wise output format is proposed to solve an ensemble model problem for track-wise output format that track permutation may occur among different models. The data augmentation approach contains several data augmentation chains, which are composed of random combinations of several data augmentation operations. The method also utilizes log-mel spectrograms, intensity vectors, and Spatial Cues-Augmented Log-Spectrogram (SALSA) for different models. We evaluate our proposed method in the Task of the L3DAS22 challenge and obtain the top ranking solution with a location-dependent F-score to be 0.699. Source code is released 1 .
The instantaneous noise-free linear mixing model in independent component analysis is largely a solved problem under the usual assumption of independent nongaussian sources and full column rank mixing matrix. However, with some prior information on the sources, like positivity, new analysis and perhaps simplified solution methods may yet become possible. In this letter, we consider the task of independent component analysis when the independent sources are known to be nonnegative and well grounded, which means that they have a nonzero pdf in the region of zero. It can be shown that in this case, the solution method is basically very simple: an orthogonal rotation of the whitened observation vector into nonnegative outputs will give a positive permutation of the original sources. We propose a cost function whose minimum coincides with nonnegativity and derive the gradient algorithm under the whitening constraint, under which the separating matrix is orthogonal. We further prove that in the Stiefel manifold of orthogonal matrices, the cost function is a Lyapunov function for the matrix gradient flow, implying global convergence. Thus, this algorithm is guaranteed to find the nonnegative well-grounded independent sources. The analysis is complemented by a numerical simulation, which illustrates the algorithm.
This research investigates the potential of eye tracking as an interface to parameter search in visual design. We outline our experimental framework where a user's gaze acts as guiding feedback mechanism in an exploration of the state space of parametric designs. A small scale pilot study was carried out where participants in uence the evolution of generative patterns by looking at a screen while having their eyes tracked. Preliminary findings suggest that although our eye tracking system can be used to e ectively navigate small areas of a parametric design's state-space, there are challenges to overcome before such a system is practical in a design context. Finally we outline future directions of this research.
There have been a number of recent papers on information theory and neural networks, especially in a perceptual system such as vision. Some of these approaches are examined, and their implications for neural network learning algorithms are considered. Existing supervised learning algorithms such as Back Propagation to minimize mean squared error can be viewed as attempting to minimize an upper bound on information loss. By making an assumption of noise either at the input or the output to the system, unsupervised learning algorithms such as those based on Hebbian (principal component analysing) or anti-Hebbian (decorrelating) approaches can also be viewed in a similar light. The optimization of information by the use of interneurons to decorrelate output units suggests a role for inhibitory interneurons and cortical loops in biological sensory systems.
Sparse representations are becoming an increasingly useful tool in the analysis of musical audio signals. In this paper we will given an overview of work by ourselves and others in this area, to give a flavour of the work being undertaken, and to give some pointers for further information about this interesting and challenging research topic.