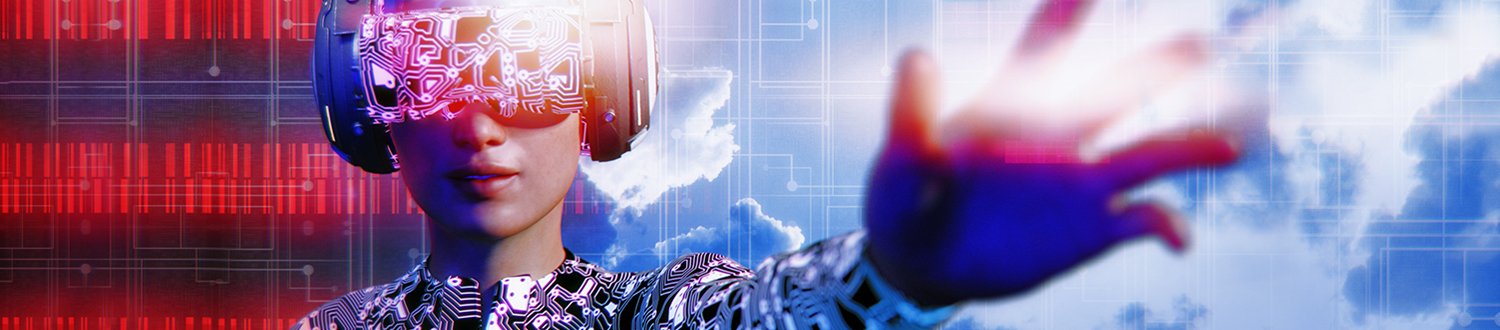
Research staff
Find out more about our research staff.
Research staff
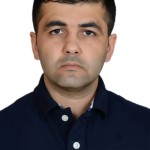
Dr Sarmad Al-Gawwam
Research Fellow in AI & Multimodality Analysis in Sleep
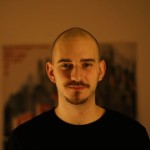
Davide Berghi
Research Engineer in audio-visual AI
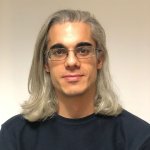
Gabriel Bibbo
Research Fellow in Sound Sensing
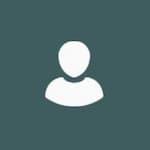
Craig Cieciura
Research Fellow A in Audio-Visual AI
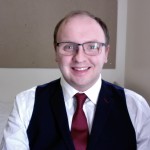
Jake Cumber
Postgraduate Research Student & Research Assistant
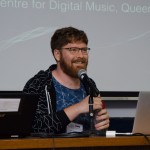
Dr Thomas Deacon
Research Fellow in Design Research for Sound Sensing
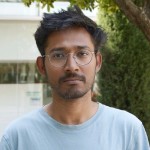
Dr Arka Ujjal Dey
Research Fellow
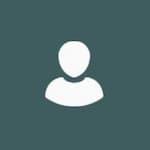
Dr Farshad Einabadi
Senior Research Engineer
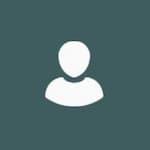
Dr Tarek Elsaleh
Research Software Engineer
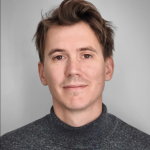
Dr Edward Fish
Research Fellow in Computer Vision and Deep Learning
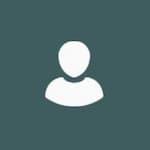
Sneha Hanumanthaiah
Research Software Engineer
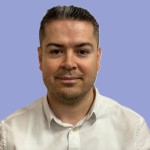
Dr Tahir Hassan
Research Fellow in Medical Image Analysis
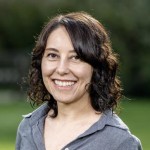
Dr Nimet Kaygusuz
Research Fellow in Vision-Based Localisation, Robotics and AI
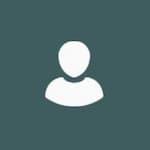
Dr Mohamed Lakhal
Research Fellow
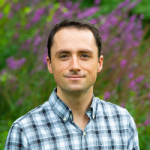
Dr Charles Malleson
Research Fellow in Computer Vision, CVSSP, Leverhulme Early Career Fellow
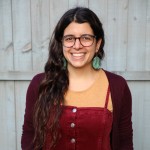
Dr Violeta Menendez Gonzalez
Senior Research Software Engineer in AI in Media Production for CoSTAR National Lab
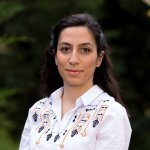
Dr Ozge Mercanoglu Sincan
Research Fellow in Computer Vision and Deep Learning
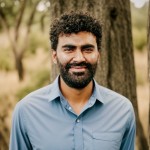
Asmar Nadeem
Research Fellow in Computer Vision, Spatial Audio
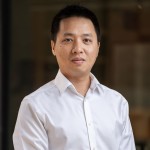
Dr Cuong Nguyen
Research Fellow in Medical Image Analysis
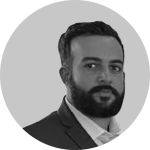
Dr Taran Rai
Data Scientist
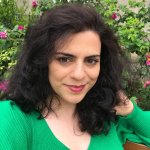
Faegheh Sardari
Research Fellow in Computer Vision and Audio-Visual AI
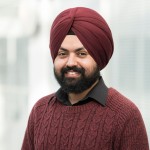
Dr Arshdeep Singh
UKAN+ Early Career Acoustic Champion, Research Fellow A in AI4S Project + Sustainability Fellow at the Institute for Sustainability
Peipei (Paul) Wu
Research Software Engineer