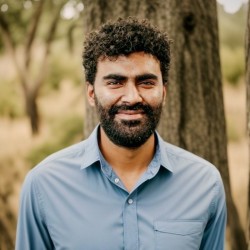
Asmar Nadeem
Academic and research departments
Centre for Vision, Speech and Signal Processing (CVSSP), School of Computer Science and Electronic Engineering.About
My research project
Audio-visual object-based dynamic scene representation from monocular videoThis project will investigate the transformation of monocular audio and visual video into a spatially localised object-based audio-visual representation.
Self-supervised and weakly supervised deep learning will be investigated for the transformation of general scenes into semantically labelled and localised objects.
Learning on in-the-wild and BBC archive datasets will be investigated to support the generalisation to complex scenes. Specific use-cases such as sports and programme recommendation will also be investigated for evaluation in constrained contexts. The approach will be evaluated on both live and legacy content.
Supervisors
This project will investigate the transformation of monocular audio and visual video into a spatially localised object-based audio-visual representation.
Self-supervised and weakly supervised deep learning will be investigated for the transformation of general scenes into semantically labelled and localised objects.
Learning on in-the-wild and BBC archive datasets will be investigated to support the generalisation to complex scenes. Specific use-cases such as sports and programme recommendation will also be investigated for evaluation in constrained contexts. The approach will be evaluated on both live and legacy content.
Publications
Leveraging machine learning techniques, in the context of object-based media production, could enable provision of personalized media experiences to diverse audiences. To fine-tune and evaluate techniques for personalization applications, as well as more broadly, datasets which bridge the gap between research and production are needed. We introduce and publicly release such a dataset, themed around a UK weather forecast and shot against a blue-screen background, of three professional actors/presenters – one male and one female (English) and one female (British Sign Language). Scenes include both production and research-oriented examples, with a range of dialogue, motions, and actions. Capture techniques consisted of a synchronized 4K resolution 16-camera array, production-typical microphones plus professional audio mix, a 16-channel microphone array with collocated Grasshopper3 camera, and a photogrammetry array. We demonstrate applications relevant to virtual production and creation of personalized media including neural radiance fields, shadow casting, action/event detection, speaker source tracking and video captioning.
Leveraging machine learning techniques, in the context of object-based media production, could enable provision of personalized media experiences to diverse audiences. To fine-tune and evaluate techniques for personalization applications, as well as more broadly, datasets which bridge the gap between research and production are needed. We introduce and publicly release such a dataset, themed around a UK weather forecast and shot against a blue-screen background, of three professional actors/presenters – one male and one female (English) and one female (British Sign Language). Scenes include both production and research-oriented examples, with a range of dialogue, motions, and actions. Capture techniques consisted of a synchronized 4K resolution 16-camera array, production-typical microphones plus professional audio mix, a 16-channel microphone array with collocated Grasshopper3 camera, and a photogrammetry array. We demonstrate applications relevant to virtual production and creation of personalized media including neural radiance fields, shadow casting, action/event detection, speaker source tracking and video captioning.
In the context of Audio Visual Question Answering (AVQA) tasks, the audio and visual modalities could be learnt on three levels: 1) Spatial, 2) Temporal, and 3) Semantic. Existing AVQA methods suffer from two major shortcomings; the audiovisual (AV) information passing through the network isn't aligned on Spatial and Temporal levels; and, intermodal (audio and visual) Semantic information is often not balanced within a context; this results in poor performance. In this paper, we propose a novel end-to-end Contextual Multi-modal Alignment (CAD) network that addresses the challenges in AVQA methods by i) introducing a parameter-free stochastic Contextual block that ensures robust audio and visual alignment on the Spatial level; ii) proposing a pre-training technique for dynamic audio and visual alignment on Temporal level in a self-supervised setting , and iii) introducing a cross-attention mechanism to balance audio and visual information on Semantic level. The proposed novel CAD network improves the overall performance over the state-of-the-art methods on average by 9.4% on the MUSIC-AVQA dataset. We also demonstrate that our proposed contributions to AVQA can be added to the existing methods to improve their performance without additional complexity requirements.
Exploiting both audio and visual modalities for video classification is a challenging task, as the existing methods require large model architectures, leading to high computational complexity and resource requirements. Smaller architectures, on the other hand, struggle to achieve optimal performance. In this paper, we propose Attend Fusion, an audio-visual (AV) fusion approach that introduces a compact model architecture specifically designed to capture intricate audio-visual relationships in video data. Through extensive experiments on the challenging YouTube-8M dataset, we demonstrate that Attend-Fusion achieves an F1 score of 75.64% with only 72M parameters, which is comparable to the performance of larger baseline models such as Fully-Connected Late Fusion (75.96% F1 score, 341M parameters). Attend-Fusion achieves similar performance to the larger baseline model while reducing the model size by nearly 80%, highlighting its efficiency in terms of model complexity. Our work demonstrates that the Attend-Fusion model effectively combines audio and visual information for video classification, achieving competitive performance with significantly reduced model size. This approach opens new possibilities for deploying high-performance video understanding systems in resource-constrained environments across various applications.
Generating grammatically and semantically correct captions in video captioning is a challenging task.. The captions generated from the existing methods are either word-by-word that do not align with grammatical structure or miss key information from the input videos. To address these issues, we introduce a novel global-local fusion network, with a Global-Local Fusion Block (GLFB) that encodes and fuses features from different parts of speech (POS) components with visual-spatial features. We use novel combinations of different POS components - 'determinant + subject', 'auxiliary verb', 'verb', and 'determinant + object' for supervision of the POS blocks - Det + Subject, Aux Verb, Verb, and Det + Object respectively. The novel global-local fusion network together with POS blocks helps align the visual features with language description to generate grammatically and semantically correct captions. Extensive qualitative and quantitative experiments on benchmark MSVD and MSRVTT datasets demonstrate that the proposed approach generates more grammatically and semantically correct captions compared to the existing methods, achieving the new state-of-the-art. Ablations on the POS blocks and the GLFB demonstrate the impact of the contributions on the proposed method.
In the context of Audio Visual Question Answering (AVQA) tasks, the audio visual modalities could be learnt on three levels: 1) Spatial, 2) Temporal, and 3) Semantic. Existing AVQA methods suffer from two major shortcomings; the audio-visual (AV) information passing through the network isn't aligned on Spatial and Temporal levels; and, inter-modal (audio and visual) Semantic information is often not balanced within a context; this results in poor performance. In this paper, we propose a novel end-to-end Contextual Multi-modal Alignment (CAD) network that addresses the challenges in AVQA methods by i) introducing a parameter-free stochastic Contextual block that ensures robust audio and visual alignment on the Spatial level; ii) proposing a pre-training technique for dynamic audio and visual alignment on Temporal level in a self-supervised setting, and iii) introducing a cross-attention mechanism to balance audio and visual information on Semantic level. The proposed novel CAD network improves the overall performance over the state-of-the-art methods on average by 9.4% on the MUSIC-AVQA dataset. We also demonstrate that our proposed contributions to AVQA can be added to the existing methods to improve their performance without additional complexity requirements.
Generating grammatically and semantically correct captions in video captioning is a challenging task. The captions generated from the existing methods are either word-by-word that do not align with grammatical structure or miss key information from the input videos. To address these issues, we introduce a novel global-local fusion network, with a Global-Local Fusion Block (GLFB) that encodes and fuses features from different parts of speech (POS) components with visual-spatial features. We use novel combinations of different POS components - 'determinant + subject', 'auxiliary verb', 'verb', and 'determinant + object' for supervision of the POS blocks - Det + Subject, Aux Verb, Verb, and Det + Object respectively. The novel global-local fusion network together with POS blocks helps align the visual features with language description to generate grammatically and semantically correct captions. Extensive qualitative and quantitative experiments on benchmark MSVD and MSRVTT datasets demonstrate that the proposed approach generates more grammatically and semantically correct captions compared to the existing methods, achieving the new state-of-the-art. Ablations on the POS blocks and the GLFB demonstrate the impact of the contributions on the proposed method.