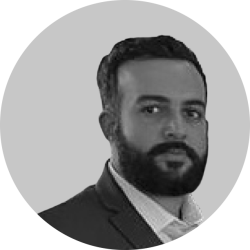
Dr Taran Rai
Academic and research departments
Centre for Vision, Speech and Signal Processing (CVSSP), School of Veterinary Medicine.Publications
The effectiveness of untrained convolutional layers for feature extraction in a computational pathology task using real-world data from a necrosis detection dataset is investigated. The study aims to determine whether ImageNet pretrained layers from deep CNNs combined with frozen untrained weights are sufficient for effective necrosis detection in canine Perivascular Wall Tumour (cPWT) whole slide images. Additionally, the authors investigate the impact of pruning CNNs, and whether it can be effective for necrosis detection as this technique can contribute towards reducing memory requirements and improve inference speed in diagnostic settings. The study found that fine-tuning the last (deepest) layers of a pretrained ImageNet model for necrosis detection in cPWT produces the highest test F1-score (0.715) when compared to alternative set ups. This score is further improved to 0.754 when the results are optimised using an optimal threshold predetermined on maximising the validation set F1-score. Resetting weights (untrained) and freezing the last few convolutional layers in the last dense block also demonstrated some capability in necrosis detection with an optimised F1-score of 0.747, still outperforming models trained from scratch as well as an ImageNet pretrained feature extraction model. Pruning the fine-tuned model using lower thresholds also showed the potential to improve performance, however thresholds higher than 0.40 negatively impacted performance.
Assessment of histology presented within tissue slides is an essential expert task undertaken by pathologists to determine diagnosis and the aggressiveness of a tumour thus leading to defining appropriate treatment options and determining prognosis for the assumed sick patient. Necrosis and mitosis seen in histological slides are both major criteria that contribute towards the scoring of histological tumour grade in canine Soft Tissue Sarcoma (cSTS). However, conventional manual assessment using traditional pathology approaches typically suffers from inter and intra-observer reproducibility thus impacting grading precision. The digitisation of histological slides into Whole Slide Images (WSI) has further advanced the field of digital pathology, which has further enabled a paradigm shift to digital workflows and the use of AI to address such inter- and intra-observer variations. The focus of this thesis is on developing computational methods to aid workflow for necrosis and mitosis detection. The exemplar used here is a subtype of cSTS known as canine Perivascular Wall Tumours (cPWT). This thesis focuses on two different approaches for necrosis and mitosis detection re- spectively. Using deep learning, we describe a novel approach for automating necrosis detection in WSIs, tested on a cSTS dataset consisting of cPWTs. Often considered a texture classification problem, blind to location, a patch-based deep learning approach was developed where different variations of training a DenseNet-161 deep learning Convolutional Neural Network (CNN) architecture were investigated as well as a stacking ensemble. An optimised DenseNet-161 with post-processing produced a hold-out test F1-score of 0.708 using a novel F1 score optimisation approach, demonstrating state-of-the-art performance. This represents a novel first-time automated necrosis detection method in the cSTS domain as well specifically in detecting necrosis in cPWTs demonstrating a significant step forward in reproducible and reliable necrosis assessment for improving the precision of tumour grading. Mitosis detection is often considered an object detection problem and works well in a multi-stage approach. Consequently, we compiled in essence an ablation study, breaking down the many steps used in a multi-stage approach. Generating mitosis annotations is a long and arduous process open to major inter and intra-observer variability. Therefore, we performed a two-step algorithm-aided annotation process; the second step using a pretrained Faster R-CNN model further trained on the initial annotations. The collaborating pathologists reviewed the false positive mitosis candidates and determined whether these were overlooked mitosis candidates thus refining the dataset. We then trained our Faster R-CNN model on this refined dataset. Taking inspiration from our necrosis work, we applied an optimal decision threshold based on maximising the F1-score. The optimal decision threshold was predetermined using the validation set and produced our best F1-score of 0.75 which is competitive with state-of-the-art in the canine mitosis detection domain. Further stages were added to the approach where ImageNet pretrained bottleneck features extracted from mitosis candidates were fed into a logistic regression model, with little improvement, suggesting that the difference between true positive and high probability threshold false positive mitosis candidates suggests a more complex approach may be required. Further work should include investigating alternative models such as an EfficientNet for necrosis detection or a Cascade R-CNN for mitosis detection, the pruning of models to create lightweight models for inference and exploring inter-species datasets from different centres to boost the size of limited datasets, thus tackling the domain shift problem in real-world settings and going further towards a One Health, One Medicine approach.
Necrosis seen in histopathology Whole Slide Images is a major criterion that contributes towards scoring tumour grade which then determines treatment options. However conventional manual assessment suffers from inter-operator reproducibility impacting grading precision. To address this, automatic necrosis detection using AI may be used to assess necrosis for final scoring that contributes towards the final clinical grade. Using deep learning AI, we describe a novel approach for automating necrosis detection in Whole Slide Images, tested on a canine Soft Tissue Sarcoma (cSTS) data set consisting of canine Perivascular Wall Tumours (cPWTs). A patch-based deep learning approach was developed where different variations of training a DenseNet-161 Convolutional Neural Network architecture were investigated as well as a stacking ensemble. An optimised DenseNet-161 with post-processing produced a hold-out test F1-score of 0.708 demonstrating state-of-the-art performance. This represents a novel first-time automated necrosis detection method in the cSTS domain as well specifically in detecting necrosis in cPWTs demonstrating a significant step forward in reproducible and reliable necrosis assessment for improving the precision of tumour grading.