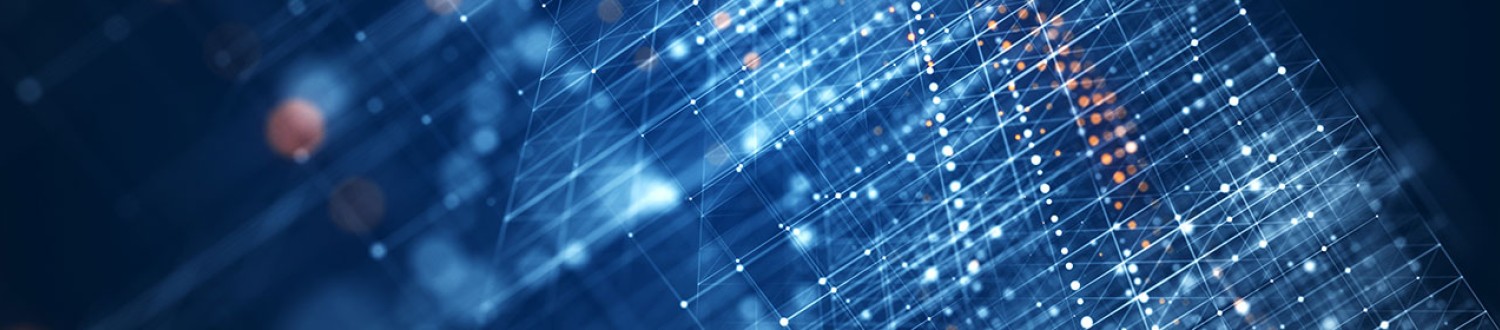
Mathematics and physics people
We are a friendly and diverse community of academic and research staff, tutors and administrators, supported by dedicated staff across the Faculty of Engineering and Physical Sciences and the University.
Our staff and students
Find out more about our staff and students and get in contact.