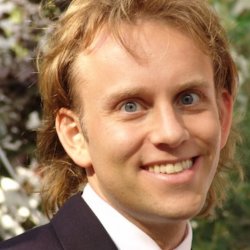
Dr Jonathan Nelson
About
Biography
I completed my PhD (2005) Cognitive Science at the University of California, San Diego (UCSD), with a dissertation on Optimal Experimental Design as a Theory of Perceptual and Cognitive Information Acquisition [http://www.jonathandnelson.com/papers/nelsonThesisCh1.pdf].
I did a postdoc (2005-2008) at the Salk Institute's Center for Neural Computation [http://cnl.salk.edu/] and UCSD's Computer Science and Engineering Department [https://cse.ucsd.edu/].
I worked from 2008-2017 as a research scientist at the Center for Adaptive Behavior and Cognition, at the Max Planck Institute for Human Development [https://www.mpib-berlin.mpg.de/en].
I am an affiliated researcher with the Berlin School of Mind and Brain [http://www.mind-and-brain.de/home/] at Humboldt University, where I occasionally teach PhD students about Cognitive Science.
I am also a guest researcher at the Max Planck Institute for Human Development [https://www.mpib-berlin.mpg.de/en].
University roles and responsibilities
- Serving on the FHMS Human Subjects Ethics Committee
Affiliations and memberships
ResearchResearch interests
Much of my research focuses on the psychology, mathematics, philosophy, and cognitive neuroscience of information. I like building (and playing!) games that teach key concepts in the mathematics of uncertainty, and hearing that children (after playing these games) tell their teacher "that was not math; that was a game". I also like "harnessing the creativity of the computer", so to speak, to figure out what various formal models entail, and when and why different models agree with or contradict each other, for instance about what exactly entropy is, or what would constitute a useful experiment in a probabilistic Bayesian framework.
I am interested in the relationship of perception (e.g. eye movements) and cognition, and more felt (gut feelings) vs more explicit representations. I also work on other themes in judgment and decision making and medical decision making, and on more applied aspects of decision making, such as figuring out which kind of information formats are most helpful for representing probabilities, relative to particular goals (see Wu et al., 2017).
Research collaborations
Since 2010 I have been leading a DFG (Deutsche Forschungsgemeinschaft)-funded project to develop integrative models of the value of information, that take into account psychological, mathematical, and philosophical considerations. This project is joint work with the philosopher Vincenzo Crupi (Turin), the mathematician and mathematics educator Laura Martignon (Ludwigsburg University of Education), and psychologists Björn Meder (Max Planck Institute for Human Development) and Katya Tentori (University of Trento). This project is part of the framework program on New Frameworks of Rationality (SPP 1516) , led by Markus Knauf (University of Giessen).
I have a number of projects with Flavia Filimon (Max Planck Institute for Human Development) and collaborators, to investigate the neural bases of valuation of information, the differences between learning probabilities through experience versus descriptively, the neural foundations of reaching movements, and multisensory representations for economic decision making tasks. Some collaborators on these projects include Gary Cottrell (University of California, San Diego), Gerd Gigerenzer (Max Planck Institute for Human Development), Amit Kothiyal (Max Planck Institute for Human Development), Terry Sejnowski (Salk Institute), Marty Sereno (San Diego State University), and Kirsten Volz (University of Tuebingen).
Research interests
Much of my research focuses on the psychology, mathematics, philosophy, and cognitive neuroscience of information. I like building (and playing!) games that teach key concepts in the mathematics of uncertainty, and hearing that children (after playing these games) tell their teacher "that was not math; that was a game". I also like "harnessing the creativity of the computer", so to speak, to figure out what various formal models entail, and when and why different models agree with or contradict each other, for instance about what exactly entropy is, or what would constitute a useful experiment in a probabilistic Bayesian framework.
I am interested in the relationship of perception (e.g. eye movements) and cognition, and more felt (gut feelings) vs more explicit representations. I also work on other themes in judgment and decision making and medical decision making, and on more applied aspects of decision making, such as figuring out which kind of information formats are most helpful for representing probabilities, relative to particular goals (see Wu et al., 2017).
Research collaborations
Since 2010 I have been leading a DFG (Deutsche Forschungsgemeinschaft)-funded project to develop integrative models of the value of information, that take into account psychological, mathematical, and philosophical considerations. This project is joint work with the philosopher Vincenzo Crupi (Turin), the mathematician and mathematics educator Laura Martignon (Ludwigsburg University of Education), and psychologists Björn Meder (Max Planck Institute for Human Development) and Katya Tentori (University of Trento). This project is part of the framework program on New Frameworks of Rationality (SPP 1516) , led by Markus Knauf (University of Giessen).
I have a number of projects with Flavia Filimon (Max Planck Institute for Human Development) and collaborators, to investigate the neural bases of valuation of information, the differences between learning probabilities through experience versus descriptively, the neural foundations of reaching movements, and multisensory representations for economic decision making tasks. Some collaborators on these projects include Gary Cottrell (University of California, San Diego), Gerd Gigerenzer (Max Planck Institute for Human Development), Amit Kothiyal (Max Planck Institute for Human Development), Terry Sejnowski (Salk Institute), Marty Sereno (San Diego State University), and Kirsten Volz (University of Tuebingen).
Teaching
- Convenor for Cognitive Psychology 1
- Contributor to Further Statistics.
Publications
Consider the task of selecting a medical test to determine whether a patient has a particular disease. Normatively, this requires taking into account (a) the prior probability of the disease, (b) the likelihood for each available test-of obtaining a positive result if the medical condition is present or absent, respectively, and (c) the utilities for both correct and incorrect treatment decisions based upon each possible test result. But these quantities may not be precisely known. Are there strategies that could help identify the test with the highest utility given incomplete information? Here, we consider the Likelihood Difference Heuristic (LDH), a simple heuristic that selects the test with the highest difference between the likelihood of obtaining a true positive and a false-positive test result, ignoring all other information. We prove that the LDH is optimal when the probability of the disease equals the therapeutic threshold, the probability for which treating the patient and not treating the patient have the same expected utility. By contrast, prominent models of the value of information from the literature, such as information gain, probability gain, and Bayesian diagnosticity, are not optimal under these circumstances. Further results show how, depending on the relationship of the therapeutic threshold and prior probability of the disease, it is possible to determine which likelihoods are more important for assessing tests' expected utilities. Finally, to illustrate the potential relevance for real-life contexts, we show how the LDH might be applied to choosing tests for screening of latent tuberculosis infection.
Research on Bayesian reasoning suggests that humans make good use of available information. Similarly, research on human information acquisition suggests that Optimal Experimental Design models predict human queries well. This perspective contrasts starkly with educational research on help seeking, which suggests that many students wait excessively long to ask for help, or even decline help when it is offered. We bring these lines of work together, exploring when people seek help as a function of problem state in the Entropy Mastermind code breaking game. The Entropy Mastermind game is a probabilistic version of the classic code breaking game, involving inductive, deductive and scientific reasoning. Whether help in the form of a hint was available was manipulated within subjects. Results showed that participants tended to ask for help late in the game play, often when they already had all the necessary information needed to crack the code. These results pose a challenge for some versions of Bayesian and Optimal Experimental Design frameworks. Possible theoretical frameworks to understand the results, including from computer science approaches to the Mastermind game, are considered.
From foraging for food to learning complex games, many aspects of human behaviour can be framed as a search problem with a vast space of possible actions. Under finite search horizons, optimal solutions are generally unobtainable. Yet, how do humans navigate vast problem spaces, which require intelligent exploration of unobserved actions? Using various bandit tasks with up to 121 arms, we study how humans search for rewards under limited search horizons, in which the spatial correlation of rewards (in both generated and natural environments) provides traction for generalization. Across various different probabilistic and heuristic models, we find evidence that Gaussian process function learning—combined with an optimistic upper confidence bound sampling strategy—provides a robust account of how people use generalization to guide search. Our modelling results and parameter estimates are recoverable and can be used to simulate human-like performance, providing insights about human behaviour in complex environments.
A crowd of pedestrians is a complex system that exhibits a rich variety of self-organized collective behaviors, such as lane formation, stop-and-go waves, or crowd turbulence. Understanding the mechanisms of crowd dynamics requires establishing a link between the local behavior of pedestrians during interactions, and the global dynamics of the crowd at high density. For this, the elaboration of a model is necessary. In this contribution, we will make a distinction between two kinds of modelling methods: outcome models that are often based on analogies with Newtonian mechanics, and process models based on concepts of cognitive science. While outcome models describe directly the movements of a pedestrian by means of repulsive forces or probabilities to move from one place to another, process models generate the movement from the bottom-up by describing the underlying cognitive process used by the pedestrian during navigation. Here, we will describe and compare two representatives of outcome and process models, namely the social force model on the one hand, and the heuristic model on the other hand. In particular, we will describe the strength and the limitations of each approach, and discuss possible future improvements for process models.
This paper investigates the role of the assumption of class-conditional independence of object features in human classification learning. This assumption holds that object feature values are statistically independent of each other, given knowledge of the object's true category. Treating features as class-conditionally independent can in many situations substantially facilitate learning and categorization even if the assumption is not perfectly true. Using optimal experimental design principles, we designed a task to test whether people have this default assumption when learning to categorize. Results provide some supporting evidence, although the data are mixed. What is clear is that classification behavior adapts to the structure of the environment: a category structure that is unlearnable under the assumption of class-conditional independence is learned by all participants.
Do dopaminergic reward structures represent the expected utility of information similarly to a reward? Optimal experimental design models from Bayesian decision theory and statistics have proposed a theoretical framework for quantifying the expected value of information that might result from a query. In particular, this formulation quantifies the value of information before the answer to that query is known, in situations where payoffs are unknown and the goal is purely epistemic: That is, to increase knowledge about the state of the world. Whether and how such a theoretical quantity is represented in the brain is unknown. Here we use an event-related functional MRI (fMRI) task design to disentangle information expectation, information revelation and categorization outcome anticipation, and response-contingent reward processing in a visual probabilistic categorization task. We identify a neural signature corresponding to the expectation of information, involving the left lateral ventral striatum. Moreover, we show a temporal dissociation in the activation of different reward-related regions, including the nucleus accumbens, medial prefrontal cortex, and orbitofrontal cortex, during information expectation versus reward-related processing.
Searching for information is critical in many situations. In medicine, for instance, careful choice of a diagnostic test can help narrow down the range of plausible diseases that the patient might have. In a probabilistic framework, test selection is often modeled by assuming that people’s goal is to reduce uncertainty about possible states of the world. In cognitive science, psychology, and medical decision making, Shannon entropy is the most prominent and most widely used model to formalize probabilistic uncertainty and the reduction thereof. However, a variety of alternative entropy metrics (Hartley, Quadratic, Tsallis, Renyi, and more) are popular in the social and the natural sciences, computer science, and philosophy of science. Particular entropy measures have been predominant in particular research areas, and it is often an open issue whether these divergences emerge from different theoretical and practical goals or are merely due to historical accident. Cutting across disciplinary boundaries, we show that several entropy and entropy reduction measures arise as special cases in a unified formalism, the Sharma–Mittal framework. Using mathematical results, computer simulations, and analyses of published behavioral data, we discuss four key questions: How do various entropy models relate to each other? What insights can be obtained by considering diverse entropy models within a unified framework? What is the psychological plausibility of different entropy models? What new questions and insights for research on human information acquisition follow? Our work provides several new pathways for theoretical and empirical research, reconciling apparently conflicting approaches and empirical findings within a comprehensive and unified information-theoretic formalism.
Judgment and Decision Making Under Uncertainty: Descriptive, Normative, and Prescriptive Perspectives was motivated by our interest in better understanding why people judge and decide as they do (descriptive perspective), how they ideally ought to judge and decide (normative perspective), and how their judgment and decision-making processes might be improved in practice (prescriptive perspective). We sought papers that addressed some aspect of judgment and decision making from one or more of these three theoretical perspectives. We further sought contributions that examined judgment and decision making under conditions of uncertainty, which we intentionally left loosely defined. Our focus on uncertainty reflects the fact that the vast majority of decisions people make in life are not made under conditions of complete certainty, and the uncertainties may be more or less well-defined. Indeed, different components of a single judgment or decision may have multiple uncertainties associated with it, some of which may be fuzzier than others. Following our call for papers, we received 32 submissions, 17 of which were accepted. The latter set comprises this book. There are 11 original research articles, 2 hypothesis and theory articles, 2 perspectives, and 1 book review and systematic review each.
Previous research shows that variation in coherence (i.e., degrees of respect for axioms of probability calculus), when used as a basis for performance-weighted aggregation, can improve the accuracy of probability judgments. However, many aspects of coherence-weighted aggregation remain a mystery, including both prescriptive issues (e.g., how best to use coherence measures) and theoretical issues (e.g., why coherence-weighted aggregation is effective). Using data from six experiments in two earlier studies ( N = 58, N = 2,858) employing either general-knowledge or statistical information integration tasks, we addressed many of these issues. Of prescriptive relevance, we examined the effectiveness of coherence-weighted aggregation as a function of judgment elicitation method, group size, weighting function, and the bias of the function’s tuning parameter. Of descriptive relevance, we propose that coherence-weighted aggregation can improve accuracy via two distinct, task-dependent routes: a causal route in which the bases for scoring accuracy depend on conformity to coherence principles (e.g., Bayesian information integration) and a diagnostic route in which coherence serves as a cue to correct knowledge. The findings provide support for the efficacy of both routes, but they also highlight why coherence weighting, especially the most biased forms, sometimes imposes costs to accuracy. We conclude by sketching a decision–theoretic approach to how aggregators can sensibly leverage the wisdom of the coherent within the crowd. (PsycInfo Database Record (c) 2023 APA, all rights reserved) (Source: journal abstract)
How do children and adults search for information when stepwise-optimal strategies fail to identify the most efficient query? The value of questions is often measured in terms of stepwise information gain (expected reduction of entropy on the next time step) or other stepwise-optimal methods. However, such myopic models are not guaranteed to identify the most efficient sequence of questions, that is, the shortest path to the solution. In two experiments we contrast stepwise methods with globally optimal strategies and study how younger children (around age 8, N = 52), older children (around age 10, N = 99), and adults (N = 101) search in a 20-questions game where planning ahead is required to identify the most efficient first question. Children searched as efficiently as adults, but also as myopically. Both children and adults tended to rely on heuristic stepwise-optimal strategies, focusing primarily on questions’ implications for the next time step, rather than planning ahead.
What drives people’s exploration in complex scenarios where they have to actively acquire information? How do people adapt their selection of queries to the environment? We explore these questions using Entropy Mastermind, a novel variant of the Mastermind code-breaking game, in which participants have to guess a secret code by making useful queries. Participants solved games more efficiently if the entropy of the game environment was low; moreover, people adapted their initial queries to the scenario they were in. We also investigated whether it would be possible to predict participants’ queries within the generalized Sharma-Mittal information-theoretic framework. Although predicting individual queries was difficult, the modeling framework offered important insights on human behavior. Entropy Mastermind opens up rich possibilities for modeling and behavioral research.
Conceptual descriptions and measures of information and entropy were established in the twentieth century with the emergence of a science of communication and information. Today these concepts have come to pervade modern science and society, and are increasingly being recommended as topics for science and mathematics education. We introduce a set of playful activities aimed at fostering intuitions about entropy and describe a primary school intervention that was conducted according to this plan. Fourth grade schoolchildren (8-10 years) played a version of Entropy Mastermind with jars and colored marbles, in which a hidden code to be deciphered was generated at random from an urn with a known, visually presented probability distribution of marble colors. Children prepared urns according to specified recipes, drew marbles from the urns, generated codes and guessed codes. Despite not being formally instructed in probability or entropy, children were able to estimate and compare the difficulty of different probability distributions used for generating possible codes.
The ability to act on the world with the goal of gaining information is core to human adaptability and intelligence. Perhaps the most successful and influential account of such abilities is the Optimal Experiment Design (OED) hypothesis, which argues that humans intuitively perform experiments on the world similar to the way an effective scientist plans an experiment. The widespread application of this theory within many areas of psychology calls for a critical evaluation of the theory’s core claims. Despite many successes, we argue that the OED hypothesis remains lacking as a theory of human inquiry and that research in the area often fails to confront some of the most interesting and important questions. In this critical review, we raise and discuss nine open questions about the psychology of human inquiry.
Humans excel in categorization. Yet from a computational standpoint, learning a novel probabilistic classification task involves severe computational challenges. The present paper investigates one way to address these challenges: assuming class-conditional independence of features. This feature independence assumption simplifies the inference problem, allows for informed inferences about novel feature combinations, and performs robustly across different statistical environments. We designed a new Bayesian classification learning model (the dependence-independence structure and category learning model, DISC-LM) that incorporates varying degrees of prior belief in class-conditional independence, learns whether or not independence holds, and adapts its behavior accordingly. Theoretical results from two simulation studies demonstrate that classification behavior can appear to start simple, yet adapt effectively to unexpected task structures. Two experiments — designed using optimal experimental design principles — were conducted with human learners. Classification decisions of the majority of participants were best accounted for by a version of the model with very high initial prior belief in class-conditional independence, before adapting to the true environmental structure. Class-conditional independence may be a strong and useful default assumption in category learning tasks.
Gute Fragen zu stellen, ist eine grundlegende Kompetenz für das Lösen alltäglicher Aufgaben. Der vorliegende Beitrag skiz-ziert eine spielerische Möglichkeit, anhand von gut überlegten Fragen zu verschiedenen Merkmalen und Fabelwesen erste Kenntnisse über Information, sowie einfache Strategien (Heuristiken) der Informationssuche von Schüler/inne/n zu vermit-teln. Dabei geht es auch darum, elementare Kompetenzen im Umgang mit Kodierung und Dekodierung von Information von Kindern zu fördern.
While the influence of presentation formats have been widely studied in Bayesian reasoning tasks, we present the first systematic investigation of how presentation formats influence information search decisions. Four experiments were conducted across different probabilistic environments, where subjects (N 2,858) chose between 2 possible search queries, each with binary probabilistic outcomes, with the goal of maximizing classification accuracy. We studied 14 different numerical and visual formats for presenting information about the search environment, constructed across 6 design features that have been prominently related to improvements in Bayesian reasoning accuracy (natural frequencies, posteriors, complement, spatial extent, countability, and part-to-whole information). The posterior variants of the icon array and bar graph formats led to the highest proportion of correct responses, and were substantially better than the standard probability format. Results suggest that presenting information in terms of posterior probabilities and visualizing natural frequencies using spatial extent (a perceptual feature) were especially helpful in guiding search decisions, although environments with a mixture of probabilistic and certain outcomes were challenging across all formats. Subjects who made more accurate probability judgments did not perform better on the search task, suggesting that simple decision heuristics may be used to make search decisions without explicitly applying Bayesian inference to compute probabilities. We propose a new take-the-difference (TTD) heuristic that identifies the accuracy-maximizing query without explicit computation of posterior probabilities.
Anxiety is one of the most prevalent mental health problems; it is known to impede cognitive functioning. It is believed to alter preferences for feedback-based learning in anxious and non-anxious learners. Thus, the present study measured feedback processing in adults ( N = 30) with and without anxiety symptoms using a probabilistic learning task. Event-related potential (ERP) measures were used to assess how the bias for either positive or negative feedback learning is reflected by the feedback-related negativity component (FRN), an ERP extracted from the electroencephalogram. Anxious individuals, identified by means of the Penn State Worry Questionnaire, showed a diminished FRN and increased accuracy after negative compared to positive feedback. Non-anxious individuals exhibited the reversed pattern with better learning from positive feedback, highlighting their preference for positive feedback. Our ERP results imply that impairments with feedback-based learning in anxious individuals are due to alterations in the mesolimbic dopaminergic system. Our finding that anxious individuals seem to favor negative as opposed to positive feedback has important implications for teacher–student feedback communication.
We investigated 4th-grade children’s search strategies on sequential search tasks in which the goal is to identify an unknown target object by asking yes–no questions about its features. We used exhaustive search to identify the most efficient question strategies and evaluated the usefulness of children’s questions accordingly. Results show that children have good intuitions regarding questions’ usefulness and search adaptively, relative to the statistical structure of the task environment. Search was especially efficient in a task environment that was representative of real-world experiences. This suggests that children may use their knowledge of real-world environmental statistics to guide their search behavior. We also compared different related search tasks. We found positive transfer effects from first doing a number search task on a later person search task.
Deciding which piece of information to acquire or attend to is fundamental to perception, categorization, medical diagnosis, and scientific inference. Four statistical theories of the value of information—information gain, Kullback-Liebler distance, probability gain (error minimization), and impact—are equally consistent with extant data on human information acquisition. Three experiments, designed via computer optimization to be maximally informative, tested which of these theories best describes human information search. Experiment 1, which used natural sampling and experience-based learning to convey environmental probabilities, found that probability gain explained subjects’ information search better than the other statistical theories or the probability-of-certainty heuristic. Experiments 1 and 2 found that subjects behaved differently when the standard method of verbally presented summary statistics (rather than experience-based learning) was used to convey environmental probabilities. Experiment 3 found that subjects’ preference for probability gain is robust, suggesting that the other models contribute little to subjects’ search behavior.
Research in psychology and artificial intelligence has sought to ground information-seeking behavior in rational terms, typically assuming that people or agents prefer more informative data over less informative data. While this seems reasonable on its surface, it assumes that informativeness is only a property of the data, rather than a joint property of the data and a (potentially bounded) learner. That is, to the extent that it is hard to draw the right inferences from data that are theoretically "high information," the data will not actually be highly informative to the learner. Here, we investigate active learning in humans using the code-breaking game Mastermind, which requires deductive reasoning from evidence. We find that people make queries that are less informative than random guesses, challenging standard rational or resource-rational accounts of information-seeking. We then show that people make queries are informative to them assuming they have a bounded capacity to draw inferences. We also find that participants prefer queries that provide easily-interpretable information over queries that provide more information but are less interpretable. Our results suggest that people are aware of their own cognitive limitations and seek information that they can use.
The goal of obtaining information to improve classification accuracy can strongly conflict with the goal of obtaining information for improving payoffs. Two environments with such a conflict were identified through computer optimization. Three subsequent experiments investigated people’s search behavior in these environments. Experiments 1 and 2 used a multiple-cue probabilistic category-learning task to convey environmental probabilities. In a subsequent search task subjects could query only a single feature before making a classification decision. The crucial manipulation concerned the search-task reward structure. The payoffs corresponded either to accuracy, with equal rewards associated with the two categories, or to an asymmetric payoff function, with different rewards associated with each category. In Experiment 1, in which learning-task feedback corresponded to the true category, people later preferentially searched the accuracy-maximizing feature, whether or not this would improve monetary rewards. In Experiment 2, an asymmetric reward structure was used during learning. Subjects searched the reward-maximizing feature when asymmetric payoffs were preserved in the search task. However, if search-task payoffs corresponded to accuracy, subjects preferentially searched a feature that was suboptimal for reward and accuracy alike. Importantly, this feature would have been most useful, under the learning-task payoff structure. Experiment 3 found that, if words and numbers are used to convey environmental probabilities, neither reward nor accuracy consistently predicts search. These findings emphasize the necessity of taking into account people’s goals and search-and-decision processes during learning, thereby challenging current models of information search.
The extent to which different cognitive processes are “embodied” is widely debated. Previous studies have implicated sensorimotor regions such as lateral intraparietal (LIP) area in perceptual decision making. This has led to the view that perceptual decisions are embodied in the same sensorimotor networks that guide body movements. We use event-related fMRI and effective connectivity analysis to investigate whether the human sensorimotor system implements perceptual decisions. We show that when eye and hand motor preparation is disentangled from perceptual decisions, sensorimotor areas are not involved in accumulating sensory evidence toward a perceptual decision. Instead, inferior frontal cortex increases its effective connectivity with sensory regions representing the evidence, is modulated by the amount of evidence, and shows greater task-positive BOLD responses during the perceptual decision stage. Once eye movement planning can begin, however, an intraparietal sulcus (IPS) area, putative LIP, participates in motor decisions. Moreover, sensory evidence levels modulate decision and motor preparation stages differently in different IPS regions, suggesting functional heterogeneity of the IPS. This suggests that different systems implement perceptual versus motor decisions, using different neural signatures.
Additional publications
Wu, CM; Schulz, E; Speekenbrink, M; Nelson, JD; Meder, B (in press). Mapping the unknown: The spatially correlated multi-armed bandit. Proceedings of the 2017 Conference of the Cognitive Science Society.
Wong, THJ; Nelson, JD; Schooler, LJ (in press). Sequential search behavior changes according to distribution shape despite having a rank-based goal. Proceedings of the International Conference on Cognitive Modeling.
Moussaïd, M; Nelson, JD (2014). Simple heuristics and the modeling of crowd behaviours. Pedestrian and Evacuation Dynamics 2012, 75-90.
Meder, BM*; Nelson, JD* (2012). Information search under asymmetric reward conditions. Judgment and Decision Making, 7(2), 119-148. http://journal.sjdm.org/12/12314/jdm12314.pdf *joint first authors
Filimon, F; Nelson, JD; Huang, R.-S.; Sereno, M. I. (2009). Multiple parietal reach regions in humans: cortical representations for visual and proprioceptive feedback during online reaching. Journal of Neuroscience, 29(9), 2961-2971
Nelson, JD & McKenzie, CRM (2009). Confirmation bias. In M. Kattan (Ed.), The Encyclopedia of Medical Decision Making, pp. 167-171. London, UK: Sage.
Nelson, JD (2008). Towards a rational theory of human information acquisition. In Oaksford, M & Chater, N (Eds.), The Probabilistic Mind: Prospects for Bayesian Cognitive Science (pp. 143-163). Oxford, UK: Oxford University Press.
Nelson, JD (2008). Conversations on cognitivism and the study of language. Cognitive Science Online, 6, 45-60.
Filimon, F; Nelson, JD; Hagler, DJ; Sereno, MI (2007). Human cortical representations for reaching: mirror neurons for execution, observation, and imagery. NeuroImage, 37(4), 1315-1328.
Nelson, JD; Cottrell, GW (2007). A probabilistic model of eye movements in concept formation. Neurocomputing, 70, 2256-2272.
Nelson, JD (2005). Finding useful questions: on Bayesian diagnosticity, probability, impact and information gain. Psychological Review, 112(4), 979-999.
McKenzie, CRM; Nelson, JD (2003). What a speaker's choice of frame reveals: Reference points, frame selection, and framing effects. Psychonomic Bulletin and Review, 10(3), 596-602.
Nelson, JD; Movellan, JR (2001) Active inference in concept learning. Advances in Neural Information Processing Systems, 13, 45-51.
Movellan, JR; Nelson, JD (2001). Probabilistic functionalism: a unifying paradigm for cognitive science. Behavioral and Brain Sciences, 24(4), 690-692.
Nelson, JD; Tenenbaum, JB; Movellan, JR (2001). Active inference in concept learning. In J. D. Moore & K. Stenning (Eds.), Proceedings of the 23rd Conference of the Cognitive Science Society, 692-697. Mahwah, NJ: Erlbaum.