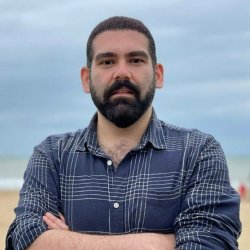
Dr Mahdi Boloursaz Mashhadi
Academic and research departments
Institute for Communication Systems, School of Computer Science and Electronic Engineering.About
Biography
Dr Mahdi Boloursaz Mashhadi (Senior Member, IEEE) is a Lecturer at the 5G/6G Innovation Centre (5G/6GIC) at the Institute for Communication Systems (ICS), School of Computer Science and Electronic Engineering (CSEE), University of Surrey, UK. Prior to joining ICS, he was a postdoctoral research associate at the Intelligent Systems and Networks (ISN) Research Group, Imperial College London, 2019-2021. He received B.S., M.S., and Ph.D. degrees in mobile telecommunications from the Sharif University of Technology (SUT), Tehran, Iran, in 2011, 2013, and 2018, respectively. He was a visiting research associate with the University of Central Florida, Orlando, USA, in 2018, and Queen’s University, Ontario, Canada, in 2017. He has more than 40 peer reviewed publications and patents in the areas of wireless communications, machine learning, and signal processing. He received the Best Paper Award from the IEEE EWDTS 2012 conference, and the Exemplary Reviewer Award from the IEEE ComSoc in 2021 and 2022. He has served as a panel judge for the International Telecommunication Union (ITU) to evaluate innovative submissions on applications of AI/ML in 5G and beyond wireless networks since 2021. He is an associate editor for the Springer Nature Wireless Personal Communications Journal.
Affiliations and memberships
Fellow of the Higher Education Academy (FHEA)
Editor, Springer Nature Wireless Personal Communications Journal
News
ResearchResearch interests
My current research is focused at the intersection of AI and machine learning and wireless communications. I'm interested in the specific role of AI and machine learning in future generation wireless networks. I am working on generative AI for telecommunications, AIoT systems, and the joint design of smart machine learning agents and the underlying wireless network to achieve goal oriented and semantic communications. I am looking at the interactions between AI and wireless communications which can be either AI for wireless communications or wireless communications for collaborative/distributed/federated machine learning.
Research projects
TOWARDS UBIQUITOUS 3D OPEN RESILIENT NETWORK (TUDOR) (Co-PI)
The TUDOR Project is a £12M UK flagship research project funded by the Department of Science, Innovation and Technology (DSIT). It targets low technology readiness level (TRL) research, aiming to tackle strategic technical challenges oriented to the design of future 6G paradigm.
Start date: February 2023 - End date: January 2025.
Research interests
My current research is focused at the intersection of AI and machine learning and wireless communications. I'm interested in the specific role of AI and machine learning in future generation wireless networks. I am working on generative AI for telecommunications, AIoT systems, and the joint design of smart machine learning agents and the underlying wireless network to achieve goal oriented and semantic communications. I am looking at the interactions between AI and wireless communications which can be either AI for wireless communications or wireless communications for collaborative/distributed/federated machine learning.
Research projects
TOWARDS UBIQUITOUS 3D OPEN RESILIENT NETWORK (TUDOR) (Co-PI)
The TUDOR Project is a £12M UK flagship research project funded by the Department of Science, Innovation and Technology (DSIT). It targets low technology readiness level (TRL) research, aiming to tackle strategic technical challenges oriented to the design of future 6G paradigm.
Start date: February 2023 - End date: January 2025.
Supervision
Postgraduate research supervision
Post Doctoral Researchers:
-Dr. Chunmei Xu, Researcher in Semantic Communications
-Dr. Daesung Yu, Researcher in AI for Communications
Current PhD Students:
-Xinkai Liu (PG/R - Comp Sci & Elec Eng, ICS)
-Sotiris Chatzimiltis (PG/R - Comp Sci & Elec Eng, ICS)
-Li Qiao (PG/R - Comp Sci & Elec Eng, ICS)
Alumni:
-Tatsuya Kikuzuki, Visiting Researcher from Fujitsu Japan
-Mahnoosh Mahdavimoghadam (PG/R - Comp Sci & Elec Eng, ICS)
I am recruiting PhD students in Advanced Wireless and Distributed Data Processing to work on cutting-edge distributed learning technologies over 6G. Interested applicants send CV's to: m.boloursazmashhadi@surrey.ac.uk
Publications
Massive multiple-input multiple-output (MIMO) systems require downlink channel state information (CSI) at the base station (BS) to better utilize the available spatial diversity and multiplexing gains. However, in a frequency division duplex (FDD) massive MIMO system, CSI feedback overhead degrades the overall spectral efficiency. Deep Learning (DL)based CSI feedback compression schemes have received a lot of attention recently as they provide significant improvements in compression efficiency; however, they still require reliable feedback links to convey the compressed CSI information to the BS. Instead, we propose here a Convolutional neural network (CNN)-based analog feedback scheme, called AnalogDeepCMC, which directly maps the downlink CSI to uplink channel input. Corresponding noisy channel outputs are used by another CNN to reconstruct the downlink channel estimate. The proposed analog scheme not only outperforms existing digital CSI feedback schemes in terms of the achievable downlink rate, but also simplifies the feedback transmission as it does not require explicit quantization, coding, and modulation, and provides a low-latency alternative particularly in rapidly changing MIMO channels, where the CSI needs to be estimated and fed back periodically.
Wireless communications is often subject to channel fading. Various statistical models have been proposed to capture the inherent randomness in fading, and conventional model-based receiver designs rely on accurate knowledge of this underlying distribution, which, in practice, may be complex and intractable. In this work, we propose a neural network-based symbol detection technique for down-link fading channels, which is based on the maximum a-posteriori probability (MAP) detector. To enable training on a diverse ensemble of fading realizations, we propose a federated training scheme, in which multiple users collaborate to jointly learn a universal data-driven detector, hence the name FedRec. The performance of the resulting receiver is shown to approach the MAP performance in diverse channel conditions without requiring knowledge of the fading statistics, while inducing a substantially reduced communication overhead in its training procedure compared to centralized training.
—Generative foundation AI models have recently shown great success in synthesizing natural signals with high perceptual quality using only textual prompts and conditioning signals to guide the generation process. This enables semantic communications at extremely low data rates in future wireless networks. In this paper, we develop a latency-aware semantic communications framework with pre-trained generative models. The transmitter performs multi-modal semantic decomposition on the input signal and transmits each semantic stream with the appropriate coding and communication schemes based on the intent. For the prompt, we adopt a re-transmission-based scheme to ensure reliable transmission, and for the other semantic modalities we use an adaptive modulation/coding scheme to achieve robustness to the changing wireless channel. Furthermore , we design a semantic and latency-aware scheme to allocate transmission power to different semantic modalities based on their importance subjected to semantic quality constraints. At the receiver, a pre-trained generative model synthesizes a high fidelity signal using the received multi-stream semantics. Simulation results demonstrate ultra-low-rate, low-latency, and channel-adaptive semantic communications.
Over-the-air computation (AirComp) is a promising technology converging communication and computation over wireless networks, which can be particularly effective in model training, inference, and more emerging edge intelligence applications. AirComp relies on uncoded transmission of individual signals, which are added naturally over the multiple access channel thanks to the superposition property of the wireless medium. Despite significantly improved communication efficiency, how to accommodate AirComp in the existing and future digital communication networks, that are based on discrete modulation schemes, remains a challenge. This paper proposes a massive digital AirComp (MD-AirComp) scheme, that leverages an unsourced massive access protocol, to enhance compatibility with both current and next-generation wireless networks. MD-AirComp utilizes vector quantization to reduce the uplink communication overhead, and employs shared quantization and modulation codebooks. At the receiver, we propose a near-optimal approximate message passing-based algorithm to compute the model aggregation results from the superposed sequences, which relies on estimating the number of devices transmitting each code sequence, rather than trying to decode the messages of individual transmitters. We apply MD-AirComp to the federated edge learning (FEEL), and show that it significantly accelerates FEEL convergence compared to state-of-the-art while using the same amount of communication resources.
—With the huge number of broadband users, automated network management becomes of huge interest to service providers. A major challenge is automated monitoring of user Quality of Experience (QoE), where Artificial Intelligence (AI) and Machine Learning (ML) models provide powerful tools to predict user QoE from basic protocol indicators such as Round Trip Time (RTT), retransmission rate, etc. In this paper, we introduce an effective feature selection method along with the corresponding classification algorithms to address this challenge. The simulation results show a prediction accuracy of 78% on the benchmark ITU ML5G-PS-012 dataset, improving 11% over the state-of-the-art result whilst reducing the model complexity at the same time. Moreover, we show that the local area network round trip time (LAN RTT) value during daytime and midweek plays the most prominent factor affecting the user QoE.
In this paper, we consider an uplink transmission of a multiuser single-input multiple-output (SIMO) system assisted with multiple reconfigurable intelligent surfaces (RISs). We investigate the energy efficiency (EE) maximization problem with an electromagnetic field (EMF) exposure constraint. In order to solve the problem, we present a lower bound for the EE and adopt an alternate optimization problem. Then, we propose the Energy Efficient Multi-RIS (EEMR) algorithm to obtain the optimal transmit power of the users and phase shifts of the RISs. Moreover, we study this problem for a system with a central RIS and compare the results. The simulation results show that for a sufficient total number of RIS elements, the system with distributed RISs is more energy efficient compared to the system with a central RIS. In addition, for both the systems the EMF exposure constraints enforce a trade-off between the EE and EMF-awareness of the system.
In this paper, the problem of drone-assisted collaborative learning is considered. In this scenario, swarm of intelligent wireless devices train a shared neural network (NN) model with the help of a drone. Using its sensors, each device records samples from its environment to gather a local dataset for training. The training data is severely heterogeneous as various devices have different amount of data and sensor noise level. The intelligent devices iteratively train the NN on their local datasets and exchange the model parameters with the drone for aggregation. For this system, the convergence rate of collaborative learning is derived while considering data heterogeneity, sensor noise levels, and communication errors, then, the drone trajectory that maximizes the final accuracy of the trained NN is obtained. The proposed trajectory optimization approach is aware of both the devices data characteristics (i.e., local dataset size and noise level) and their wireless channel conditions, and significantly improves the convergence rate and final accuracy in comparison with baselines that only consider data characteristics or channel conditions. Compared to state-of-the-art baselines, the proposed approach achieves an average 3.85 improvement in the final accuracy of the trained NN on benchmark datasets for image recognition and semantic segmentation tasks, respectively. Moreover, the proposed framework achieves a significant speedup in training, leading to an average 24% and 87% saving in the drone's hovering time, communication overhead, and battery usage, respectively for these tasks.
Deep neural networks (DNNs) in the wireless communication domain have been shown to be hardly generalizable to scenarios where the train and test datasets follow a different distribution. This lack of generalization poses a significant hurdle to the practical utilization of DNNs in wireless communication. In this paper, we propose a generalizable deep learning approach for millimeter wave (mmWave) beam selection using sub-6 GHz channel state information (CSI) measurements, referred to as PARAMOUNT. First, we provide a detailed discussion on physical aspects of the electromagnetic wave scattering in the mmWave and sub-6 GHz bands. Based on this discussion, we develop the augmented discrete angle delay profile (ADADP) which is a novel linear transformation for the sub-6 GHz CSI that extracts the angle-delay attributes and provides a semantic visual representation of the multi-path clusters. Next, we introduce a convolutional neural network (CNN) structure that can learn the signatures of the path clusters in the sub-6 GHz ADADP representation and transform it to mmWave band beam indices. We demonstrate by extensive simulations on several different datasets that PARAMOUNT can generalize beyond the training dataset which is mainly due to transfer learning principles that allow transferring information from previously learned tasks to the learning of new unseen tasks.
Current technological advancements in Software Defined Networks (SDN) can provide efficient solutions for smart grids (SGs). An SDN-based SG promises to enhance the efficiency, reliability and sustainability of the communication network. However, new security breaches can be introduced with this adaptation. A layer of defence against insider attacks can be established using machine learning based intrusion detection system (IDS) located on the SDN application layer. Conventional centralised practises, violate the user data privacy aspect, thus distributed or collaborative approaches can be adapted so that attacks can be detected and actions can be taken. This paper proposes a new SDN-based SG architecture, highlighting the existence of IDSs in the SDN application layer. We implemented a new smart meter (SM) collaborative intrusion detection system (SM-IDS), by adapting the split learning methodology. Finally, a comparison of a federated learning and split learning neighbourhood area network (NAN) IDS was made. Numerical results showed, a five class classification accuracy of over 80.3% and F1-score 78.9 for a SM-IDS adapting the split learning technique. Also, the split learning NAN-IDS exhibit an accuracy of over 81.1% and F1-score 79.9.
We present a new Deep Neural Network (DNN)-based error correction code for fading channels with output feedback, called the Deep SNR-Robust Feedback (DRF) code. At the encoder, parity symbols are generated by a Long Short Term Memory (LSTM) network based on the message, as well as the past forward channel outputs observed by the transmitter in a noisy fashion. The decoder uses a bidirectional LSTM architecture along with a Signal to Noise Ratio (SNR)-aware attention NN to decode the message. The proposed code overcomes two major shortcomings of DNN-based codes over channels with passive output feedback: (i) the SNR-aware attention mechanism at the decoder enables reliable application of the same trained NN over a wide range of SNR values; (ii) curriculum training with batch size scheduling is used to speed up and stabilize training while improving the SNR-robustness of the resulting code. We show that the DRF codes outperform the existing DNN-based codes in terms of both the SNR-robustness and the error rate in an Additive White Gaussian Noise (AWGN) channel with noisy output feedback. In fading channels with perfect phase compensation at the receiver, DRF codes learn to efficiently exploit knowledge of the instantaneous fading amplitude (which is available to the encoder through feedback) to reduce the overhead and complexity associated with channel estimation at the decoder. Finally, we show the effectiveness of DRF codes in multicast channels with feedback, where linear feedback codes are known to be strictly suboptimal. These results show the feasibility of automatic design of new channel codes using DNN-based language models.
The communication bottleneck severely constrains the scalability of distributed deep learning, and efficient communication scheduling accelerates distributed DNN training by overlapping computation and communication tasks. However, existing approaches based on tensor partitioning are not efficient and suffer from two challenges: (1) the fixed number of tensor blocks transferred in parallel can not necessarily minimize the communication overheads; (2) although the scheduling order that preferentially transmits tensor blocks close to the input layer can start forward propagation in the next iteration earlier, the shortest per-iteration time is not obtained. In this paper, we propose an efficient communication framework called US-Byte. It can schedule unequal-sized tensor blocks in a near-optimal order to minimize the training time. We build the mathematical model of US-Byte by two phases: (1) the overlap of gradient communication and backward propagation, and (2) the overlap of gradient communication and forward propagation. We theoretically derive the optimal solution for the second phase and efficiently solve the first phase with a low-complexity algorithm. We implement the US-Byte architecture on PyTorch framework. Extensive experiments on two different 8-node GPU clusters demonstrate that US-Byte can achieve up to 1.26x and 1.56x speedup compared to ByteScheduler and WFBP, respectively. We further exploit simulations of 128 GPUs to verify the potential scaling performance of US-Byte. Simulation results show that US-Byte can achieve up to 1.69x speedup compared to the state-of-the-art communication framework.
Massive multiple-input multiple-output (MIMO) systems are a main enabler of the excessive throughput requirements in 5G and future generation wireless networks as they can serve many users simultaneously with high spectral and energy efficiency. To achieve this massive MIMO systems require accurate and timely channel state information (CSI), which is acquired by a training process that involves pilot transmission, CSI estimation, and feedback. This training process incurs a training overhead, which scales with the number of antennas, users, and subcarriers. Reducing the training overhead in massive MIMO systems has been a major topic of research since the emergence of the concept. Recently, deep learning (DL)-based approaches have been proposed and shown to provide significant reduction in the CSI acquisition and feedback overhead in massive MIMO systems compared to traditional techniques. In this paper, we present an overview of the state-of-the-art DL architectures and algorithms used for CSI acquisition and feedback, and provide further research directions.
In this letter, we investigate the signal-to-interference-plus-noise-ratio (SINR) maximization problem in a multi-user massive multiple-input-multiple-output (massive MIMO) system enabled with multiple reconfigurable intelligent surfaces (RISs). We examine two zero-forcing (ZF) beamforming approaches for interference management namely BS-UE-ZF and BS-RIS-ZF that enforce the interference to zero at the users (UEs) and the RISs, respectively. Then, for each case, we resolve the SINR maximization problem to find the optimal phase shifts of the elements of the RISs. Also, we evaluate the asymptotic expressions for the optimal phase shifts and the maximum SINRs when the number of the base station (BS) antennas tends to infinity. We show that if the channels of the RIS elements are independent and the number of the BS antennas tends to infinity, random phase shifts achieve the maximum SINR using the BS-UE-ZF beamforming approach. The simulation results illustrate that by employing the BS-RIS-ZF beamforming approach, the asymptotic expressions of the phase shifts and maximum SINRs achieve the rate obtained by the optimal phase shifts even for a small number of the BS antennas.
Efficient link configuration in millimeter wave (mmWave) communication systems is a crucial yet challenging task due to the overhead imposed by beam selection. For vehicle-to-infrastructure (V2I) networks, side information from LIDAR sensors mounted on the vehicles has been leveraged to reduce the beam search overhead. In this letter, we propose a federated LIDAR aided beam selection method for V2I mmWave communication systems. In the proposed scheme, connected vehicles collaborate to train a shared neural network (NN) on their locally available LIDAR data during normal operation of the system. We also propose a reduced-complexity convolutional NN (CNN) classifier architecture and LIDAR preprocessing, which significantly outperforms previous works in terms of both the performance and the complexity.
With the large number of antennas and subcarriers the overhead due to pilot transmission for channel estimation can be prohibitive in wideband massive multiple-input multiple-output (MIMO) systems. This can degrade the overall spectral efficiency significantly, and as a result, curtail the potential benefits of massive MIMO. In this paper, we propose a neural network (NN)-based joint pilot design and downlink channel estimation scheme for frequency division duplex (FDD) MIMO orthogonal frequency division multiplex (OFDM) systems. The proposed NN architecture uses fully connected layers for frequency-aware pilot design, and outperforms linear minimum mean square error (LMMSE) estimation by exploiting inherent correlations in MIMO channel matrices utilizing convolutional NN layers. Our proposed NN architecture uses a non-local attention module to learn longer range correlations in the channel matrix to further improve the channel estimation performance.We also propose an effective pilot reduction technique by gradually pruning less significant neurons from the dense NN layers during training. This constitutes a novel application of NN pruning to reduce the pilot transmission overhead. Our pruning-based pilot reduction technique reduces the overhead by allocating pilots across subcarriers non-uniformly and exploiting the inter-frequency and inter-antenna correlations in the channel matrix efficiently through convolutional layers and attention module.
Massive multiple-input multiple-output (MIMO) systems require downlink channel state information (CSI) at the base station (BS) to achieve spatial diversity and multiplexing gains. In a frequency division duplex (FDD) multiuser massive MIMO network, each user needs to compress and feedback its downlink CSI to the BS. The CSI overhead scales with the number of antennas, users and subcarriers, and becomes a major bottleneck for the overall spectral efficiency. In this paper, we propose a deep learning (DL)-based CSI compression scheme, called DeepCMC , composed of convolutional layers followed by quantization and entropy coding blocks. In comparison with previous DL-based CSI reduction structures, DeepCMC proposes a novel fully-convolutional neural network (NN) architecture, with residual layers at the decoder, and incorporates quantization and entropy coding blocks into its design. DeepCMC is trained to minimize a weighted rate-distortion cost, which enables a trade-off between the CSI quality and its feedback overhead. Simulation results demonstrate that DeepCMC outperforms the state of the art CSI compression schemes in terms of the reconstruction quality of CSI for the same compression rate. We also propose a distributed version of DeepCMC for a multi-user MIMO scenario to encode and reconstruct the CSI from multiple users in a distributed manner. Distributed DeepCMC not only utilizes the inherent CSI structures of a single MIMO user for compression, but also benefits from the correlations among the channel matrices of nearby users to further improve the performance in comparison with DeepCMC. We also propose a reduced-complexity training method for distributed DeepCMC, allowing to scale it to multiple users, and suggest a cluster-based distributed DeepCMC approach for practical implementation.
Efficient millimeter wave (mmWave) beam selection in vehicle-to-infrastructure (V2I) communication is a crucial yet challenging task due to the narrow mmWave beamwidth and high user mobility. To reduce the search overhead of iterative beam discovery procedures, contextual information from light detection and ranging (LIDAR) sensors mounted on vehicles has been leveraged by data-driven methods to produce useful side information. In this paper, we propose a lightweight neural network (NN) architecture along with the corresponding LIDAR preprocessing, which significantly outperforms previous works. Our solution comprises multiple novelties that improve both the convergence speed and the final accuracy of the model. In particular, we define a novel loss function inspired by the knowledge distillation idea, introduce a curriculum training approach exploiting line-of-sight (LOS)/non-line-of-sight (NLOS) information, and we propose a non-local attention module to improve the performance for the more challenging NLOS cases. Simulation results on benchmark datasets show that utilizing solely LIDAR data and the receiver position, our NN-based beam selection scheme can achieve 79.9% throughput of an exhaustive beam sweeping approach without any beam search overhead and 95% by searching among as few as 6 beams. In a typical mmWave V2I scenario, our proposed method considerably reduces the beam search time required to achieve a desired throughput, in comparison with the inverse fingerprinting and hierarchical beam selection schemes.
Additional publications
For a comprehensive list of my publications please refer to my Google Scholar.