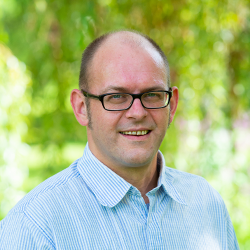
Dr Michael Danner
Academic and research departments
Faculty of Engineering and Physical Sciences, Centre for Vision, Speech and Signal Processing (CVSSP).Publications
In recent years, 3D facial reconstructions from single images have garnered significant interest. Most of the approaches are based on 3D Morphable Model (3DMM) fitting to reconstruct the 3D face shape. Concurrently, the adoption of Generative Adversarial Networks (GAN) has been gaining momentum to improve the texture of reconstructed faces. In this paper, we propose a fundamentally different approach to reconstructing the 3D head shape from a single image by harnessing the power of GAN. Our method predicts three maps of normal vectors of the head’s frontal, left, and right poses. We are thus presenting a model-free method that does not require any prior knowledge of the object’s geometry to be reconstructed. The key advantage of our proposed approach is the substantial improvement in reconstruction quality compared to existing methods, particularly in the case of facial regions that are self-occluded in the input image. Our method is not limited to 3d face reconstruction. It is generic and applicable to multiple kinds of 3D objects. To illustrate the versatility of our method, we demonstrate its efficacy in reconstructing the entire human body. By delivering a model-free method capable of generating high-quality 3D reconstructions, this paper not only advances the field of 3D facial reconstruction but also provides a foundation for future research and applications spanning multiple object types. The implications of this work have the potential to extend far beyond facial reconstruction, paving the way for innovative solutions and discoveries in various domains.
3D assisted 2D face recognition involves the process of reconstructing 3D faces from 2D images and solving the problem of face recognition in 3D. To facilitate the use of deep neural networks, a 3D face, normally represented as a 3D mesh of vertices and its corresponding surface texture, is remapped to image-like square isomaps by a conformal mapping. Based on previous work, we assume that face recognition benefits more from texture. In this work, we focus on the surface texture and its discriminatory information content for recognition purposes. Our approach is to prepare a 3D mesh, the corresponding surface texture and the original 2D image as triple input for the recognition network, to show that 3D data is useful for face recognition. Texture enhancement methods to control the texture fusion process are introduced and we adapt data augmentation methods. Our results show that texture-map-based face recognition can not only compete with state-of-the-art systems under the same preconditions but also outperforms standard 2D methods from recent years.
3D assisted 2D face recognition involves the process of reconstructing 3D faces from 2D images and solving the problem of face recognition in 3D. To facilitate the use of deep neural networks, a 3D face, normally represented as a 3D mesh of vertices and its corresponding surface texture, is remapped to image-like square isomaps by a conformal mapping. Based on previous work, we assume that face recognition benefits more from texture. In this work, we focus on the surface texture and its discriminatory information content for recognition purposes. Our approach is to prepare a 3D mesh, the corresponding surface texture and the original 2D image as triple input for the recognition network, to show that 3D data is useful for face recognition. Texture enhancement methods to control the texture fusion process are introduced and we adapt data augmentation methods. Our results show that texture-map-based face recognition can not only compete with state-of-the-art systems under the same preconditions but also outperforms standard 2D methods from recent years.