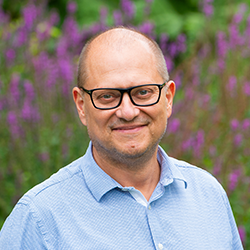
Professor Miroslaw Bober
Academic and research departments
Centre for Vision, Speech and Signal Processing (CVSSP), School of Computer Science and Electronic Engineering.About
Biography
Miroslaw Bober joined Surrey in 2011 as Professor of Video Processing. He is leading the Visual Media Analysis team within the Centre for Vision, Speech and Signal Processing (CVSSP).
Prior to his appointment at Surrey Prof Bober was the General Manager of the Mitsubishi Electric R&D Centre Europe (MERCE-UK), and the Head of Research for its Visual & Sensing Division. He was leading this European Corporate R&D centre for 15 years. His technical achievements were recognized in numerous awards, including the Presidential Award for strengthening the TV business in Japan via an innovative “Visual Navigation” content access technology (2010) and the prestigious Mitsubishi Best Invention Award for his Image Signature Technology (2008-one winner selected globally).
Prof Bober received a BSc and an MSc degree with distinction in electrical engineering from the AGH University of Science and Technology (Krakow, Poland) (1990), an MSc degree in Machine Intelligence (with distinction) from Surrey University (1991), and a PhD degree in computer vision from Surrey University (1995).
Miroslaw has published over 60 peer-reviewed publications and is the named inventor on over 80 unique patent applications. He has held over 30 research and industrial grants, with the value exceeding £16M. He is a member of the British Standards Institution (BSI) committee IST/37, responsible for UK contributions into MPEG and JPEG and represents UK in the area of image and video analysis and associated metadata. Prof Bober is chairing MPEG technical work on Compact Descriptors for Visual Search (CDVS - standard ISO/IEC FDIS 15938-13) and Compact Descriptors for Video Analysis (CDVA - work in progress).
University roles and responsibilities
- Programme Director for MSc in Multimedia Signal Processing and Communications
- Industrial tutor for undergraduate industrial placement year
- Personal tutor for undergraduate students (L1, L2, L3, L4)
- MSc-level tutor
- Member of Faculty Research Degrees Committee (FRDC)
- Member of the Departmental Industrial Advisory Board (IAB)
Affiliations
I have extensive collaboration links with universities and research institutions in Europe (UK, Switzerland, Germany, Poland, France, Spain), US, Japan and China.
I have also worked with the following companies: the BBC (UK), Bang and Olufsen (DE), CEDEO (IT), Casio (JP), Ericsson (SE), Huawei (DE), Mitsubishi Electric (JP), RAI television (IT), Renesas Electronics (JP), Telecom Italia (IT), and Visual Atoms (UK).
ResearchResearch interests
My research focuses on novel techniques in signal processing, computer vision and machine learning and their applications in industry, healthcare, big-data and security.
I have a particular interest in image and video analysis and retrieval (visual search, object recognition, analysis of motion, shape and texture). The broad research objective is to develop unique methods and technology solutions for visual content understanding that can dramatically improve on existing state-of-the art leading to new applications.
My algorithms for shape analysis and image/video fingerprinting as well as visual search are considered world-leading and were selected for ISO International standards within MPEG and used by, e.g. Metropolitan Police.
Research projects
I am the project coordinator and PI for the BRIDGET FP-7 project [5.28 M€], where my team is responsible for the development of ultra large-scale visual search and media analysis algorithms for the broadcast industry. The project aims to open new dimensions for multimedia content creation and consumption by bridging the gap between the Broadcast and Internet. Project partners include RAI television, Huawei, Telecom Italia and more.
CODAM is my latest project (PI) and is funded by the TSB creative media call [£1.05 M]. My team is working with the BBC and Visual Atoms to develop an advanced video asset management system with unique visual fingerprinting and visual search capabilities. It will aid content creation and deployment by enabling visual content tracking, identification and searching across multiple devices and platforms, and across diverse digital media ecosystems and markets. Where is the original version of the low-quality clip? Which video clip has been used most often in BBC programmes? Is it a stock shot of a red double decker bus, or an excerpt from a royal wedding? Is there other footage in the archive that shows the same event but can provide a fresh viewpoint? The CODAM system will answer these questions, track the origins of video clips across multi-platform productions and search for related material. It will take the form of a modular software system that can identify individual video clips in edited programmes, and perform object or scene recognition to find similar footage in an archive without relying on manually entered and often incomplete metadata.
Research interests
My research focuses on novel techniques in signal processing, computer vision and machine learning and their applications in industry, healthcare, big-data and security.
I have a particular interest in image and video analysis and retrieval (visual search, object recognition, analysis of motion, shape and texture). The broad research objective is to develop unique methods and technology solutions for visual content understanding that can dramatically improve on existing state-of-the art leading to new applications.
My algorithms for shape analysis and image/video fingerprinting as well as visual search are considered world-leading and were selected for ISO International standards within MPEG and used by, e.g. Metropolitan Police.
Research projects
I am the project coordinator and PI for the BRIDGET FP-7 project [5.28 M€], where my team is responsible for the development of ultra large-scale visual search and media analysis algorithms for the broadcast industry. The project aims to open new dimensions for multimedia content creation and consumption by bridging the gap between the Broadcast and Internet. Project partners include RAI television, Huawei, Telecom Italia and more.
CODAM is my latest project (PI) and is funded by the TSB creative media call [£1.05 M]. My team is working with the BBC and Visual Atoms to develop an advanced video asset management system with unique visual fingerprinting and visual search capabilities. It will aid content creation and deployment by enabling visual content tracking, identification and searching across multiple devices and platforms, and across diverse digital media ecosystems and markets. Where is the original version of the low-quality clip? Which video clip has been used most often in BBC programmes? Is it a stock shot of a red double decker bus, or an excerpt from a royal wedding? Is there other footage in the archive that shows the same event but can provide a fresh viewpoint? The CODAM system will answer these questions, track the origins of video clips across multi-platform productions and search for related material. It will take the form of a modular software system that can identify individual video clips in edited programmes, and perform object or scene recognition to find similar footage in an archive without relying on manually entered and often incomplete metadata.
Teaching
- EEE3034 - Media Casting (Module Coordinator)
- EEE3029 - Multimedia Systems and Component Technology
- EEEM001 - Image and Video Compression
- EEE3035 - Engineering Professional Studies.
Publications
Hybrid networks combine fixed and learnable filters to address the limitations of fully trained CNNs such as poor interpretability, high computational complexity and a need for large training sets. Many hybrid designs were proposed, utilising different filter types, backbone CNNs and different approaches to learning. They were evaluated on different (and often simplistic) datasets, making it difficult to understand their relative performance, their strengths and weaknesses, also there are no design guides on building a hybrid application for the problem at hand. We present and benchmark a collection of 27 networks, some new learnable extensions to existing designs, all within a framework that allows an assessment of a wide range of scattering types and their effects on the system performance. Also, we outline application scenarios most suitable for hybrid networks, identify previously unnoticed trends and provide guidance in building hybrids.
Recent work showed that hybrid networks, which combine predefined and learnt filters within a single architecture, are more amenable to theoretical analysis and less prone to overfitting in data-limited scenarios. However, their performance has yet to prove competitive against the conventional counterparts when sufficient amounts of training data are available. In an attempt to address this core limitation of current hybrid networks, we introduce an Efficient Hybrid Network (E-HybridNet). We show that it is the first scattering based approach that consistently outperforms its conventional counterparts on a diverse range of datasets. It is achieved with a novel inductive architecture that embeds scattering features into the network flow using Hybrid Fusion Blocks. We also demonstrate that the proposed design inherits the key property of prior hybrid networks - an effective generalisation in data-limited scenarios. Our approach successfully combines the best of the two worlds: flexibility and power of learnt features and stability and predictability of scattering representations.
Many studies show the possibilities and benefits of combining physical and digital information through augmented paper. Furthermore, the rise of Augmented Reality hardware and software for annotating the physical world with information is becoming more commonplace as a new computing paradigm. But so far, this has not been commercially applied to paper in a way that publishers can control. In fact, there is currently no standard way for book publishers to augment their printed products with digital media, short of using QR codes or creating custom AR apps. In this paper we outline a new publishing ecosystem for the creation and consumption of augmented books, and report the lab and field evaluation of a first commercial travel guide to use this. This is based simply on the use of the standard EPUB3 format for interactive e-books that forms the basis of a new 'a-book' file format and app.
A method of representing an object appearing in a still or video image, by processing signals corresponding to the image, comprises deriving a plurality of sets of co-ordinate values representing the shape of the object and quantising the co-ordinate values to derive a coded representation of the shape, and further comprises quantising a first co-ordinate value over a first quantisation range and quantising a smaller co-ordinate value over a smaller range.
A method of representing a data distribution derived from an object or image by processing signals corresponding to the object or image comprising deriving an approximate representation of the data distribution and analysing the errors of the data elements when expressed in terms of the approximate representation.
A method representing an object appearing in still or video image for use in searching, wherein the object appears in the image with a first two-dimensional outline, by processing signals corresponding to the image, comprises deriving a view descriptor of the first outline of the object and deriving at least one additional view descriptor of the outline of the object in a different view, and associating the two or more view descriptors to form an object descriptor.
A Hough transform based method of estimating N parameters a=(a.sub.1, . . . , a.sub.N) of motion of a region Y in a first image to a following image, the first and following images represented, in a first spatial resolution, by intensities at pixels having coordinates in a coordinate system, the method including: determining the total support H(Y,a) as a sum of the values of an error function for the intensities at pixels in the region Y; determining the motion parameters a that give the total support a minimum value; the determining being made in steps of an iterative process moving along a series of parameter estimates a.sub.1, a.sub.2, . . . by calculating partial derivatives dH.sub.i =MH(Y,a.sub.n)/Ma.sub.n,i of the total support for a parameter estimate a.sub.n with respect to each of the parameters a.sub.i and evaluating the calculated partial derivatives for taking a new a.sub.n+1 ; and wherein, in the evaluating of the partial derivatives, the partial derivatives dH.sub.i are first scaled by multiplying by scaling factors dependent on the spatial extension of the region to produce scaled partial derivatives dHN.sub.i.
Method and apparatus for motion vector field encoding Abstract A method and apparatus for representing motion in a sequence of digitized images derives a dense motion vector field and vector quantizes the motion vector field.
A method of deriving a representation of a video sequence comprises deriving metadata expressing at least one temporal characteristic of a frame or group of frames, and one or both of metadata expressing at least one content-based characteristic of a frame or group of frames and relational metadata expressing relationships between at least one content-based characteristic of a frame or group of frames and at least one other frame or group of frames, and associating said metadata and/or relational metadata with the respective frame or group of frames.
A method of analysing an image comprises performing a Hough transform on points in an image space to an n-dimensional Hough space, selecting points in the Hough space representing features in the image space, and analysing m of the n variables for the selected points, where m is less than n, for information about the features in the image space.
A method of representing an object appearing in a still or video image, by processing signals corresponding to the image, comprises deriving the peak values in CSS space for the object outline and applying a non-linear transformation to said peak values to arrive at a representation of the outline.
A method of estimating a transformation between a pair of images, comprises estimating local transformations for a plurality of regions of the images to derive a set of estimated transformations, and selecting a subset of said estimated local transformations as estimated global transformations for the image.
Drawing Description Claims Patent number: 7236629 Filing date: 8 Apr 2003 Issue date: 26 Jun 2007 Application number: 10/408,316 A method of detecting a region having predetermined colour characteristics in an image comprises transforming colour values of pixels in the image from a first colour space to a second colour space, using the colour values in the second colour space to determine probability values expressing a match between pixels and the predetermined colour characteristics, where the probability values range over a multiplicity of values, using said probability values to identify pixels at least approximating to said predetermined colour characteristics, grouping pixels which at least approximate to said predetermined colour characteristics, and extracting information about each group, wherein pixels are weighted according to the respective multiplicity of probability values, and the weightings are used when grouping the pixels and/or when extracting information about a group.
A method for deriving an image identifier comprises deriving a scale-space representation of an image, and processing the scale-space representation to detect a plurality of feature points having values that are maxima or minima. A representation is derived for a scale-dependent image region associated with one or more of the detected plurality of feature points. In an embodiment, the size of the image region is dependent on the scale associated with the corresponding feature point. An image identifier is derived using the representations derived for the scale-dependent image regions. The image identifiers may be used in a method for comparing images.
A method of representing an image or sequence of images using a depth map comprises transforming an n-bit depth map representation into an m-bit depth map representation, where m
A method of representing an object appearing in a still or video image, by processing signals corresponding to the image, comprises deriving the peak values in CSS space for the object outline and applying a non-linear transformation to said peak values to arrive at a representation of the outline.
A method of detecting lines in an image comprises using one or more masks for detecting lines in one or more directions out of horizontal, vertical, left diagonal and right diagonal, and further comprises one or more additional masks for detecting lines in one or more additional directions.
A method of identifying or tracking a line in an image comprises determining a start point that belongs to a line, and identifying a plurality of possible end points belonging to the line using a search window, and calculating values for a plurality of paths connecting the start point and the end points to determine an optimum end point and path, characterised in that the search window is non-rectangular.
A method of representing an image comprises deriving at least one 1-dimensional representation of the image by projecting the image onto an axis, wherein the projection involves summing values of selected pixels in a respective line of the image perpendicular to said axis, characterised in that the number of selected pixels is less than the number of pixels in the line.
An entity is subjected to an interrogating signal, and the reflection from the entity is repeatedly sampled to obtain a first set of values each dependent on the intensity of the reflected signal. A logarithmic transformation is applied to the sample values to obtain a second set of values. A set of descriptor values is derived, the set comprising at least a first descriptor value (L) representing the difference between the mean and the median of the second set of values, and a second descriptor value (D) representing the mean of the absolute value of the deviation between each second set value and an average of the second set of values.
A method of representing an object appearing in a still or video image for use in searching, wherein the object appears in the image with a first two-dimensional outline, by processing signals corresponding to the image, comprises deriving a view descriptor of the first outline of the object and deriving at least one additional view descriptor of the outline of the object in a different view, and associating the two or more view descriptors to form an object descriptor.
A method of representing at least one image comprises deriving at least one descriptor based on color information and color interrelation information for at least one region of the image, the descriptor having at least one descriptor element, derived using values of pixels in said region, wherein at least one descriptor element for a region is derived using a non-wavelet transform. The representations may be used for image comparisons.
A method of representing an object appearing in a still or video image, by processing signals corresponding to the image, comprises deriving a curvature scale space (CSS) representation of the object outline by smoothing the object outline, deriving at least one additional parameter reflecting the shape or mass distribution of a smoothed version of the original curve, and associating the CSS representation and the additional parameter as a shape descriptor of the object.
A method of representing a 2-dimensional image comprises deriving at least one 1-dimensional representation of the image by projecting the image onto at least one axis, and applying a Fourier transform to said 1-dimensional representation. The representation can be used for estimation of dominant motion between images.
A method and apparatus for processing a first sequence of images and a second sequence of images to compare the first and second sequences is disclosed. Each of a plurality of the images in the first sequence and each of a plurality of the images in the second sequence is processed by (i) processing the image data for each of a plurality of pixel neighbourhoods in the image to generate at least one respective descriptor element for each of the pixel neighbourhoods, each descriptor element comprising one or more bits; and (ii) forming a plurality of words from the descriptor elements of the image such that each word comprises a unique combination of descriptor element bits. The words for the second sequence are generated from the same respective combinations of descriptor element bits as the words for the first sequence. Processing is performed to compare the first and second sequences by comparing the words generated for the plurality of images in the first sequences with the words...
A one-dimensional representation of an image is obtained using a mapping function defining a closed scanning curve. The function is decomposed into component signals which represent different parts of the bandwidth of the representation using bi-directional filters to achieve zero group delay.
A method and apparatus for deriving a representation of an image is described. The method involves processing signals corresponding to the image. A two-dimensional function of the image, such as a Trace transform (T (d, θ)), of the image using at least one functional T, is derived and processed using a mask function (β) to derive an intermediate representation of the image, corresponding to a one-dimensional function. In one embodiment, the mask function defines pairs of image bands of the Trace transform in the Trace domain. The representation of the image may be derived by applying existing techniques to the derived one-dimensional function.
This paper presents an advanced process for designing “a-books”; augmented printed books with multimedia links presented on a nearby device. Although augmented paper is not new, our solution facilitates mass market use through industry standard publishing software that generates the a-book, and regular smartphones that play related digital media by optically recognising its ordinary paper pages through the phone’s built-in camera. This augmented paper strategy informs new classifications of digital content within publication design, enabling new immersive reading possibilities. Complementary affordances of print and digital, and how these are combined and harnessed by a-books in comparison to previous augmented paper concepts are first discussed. Subsequently, an explanation of the workflow for designing a-books is described. The final discussion includes implications for content creators of paper-based publishing, and future research plans.
Necrosis seen in histopathology Whole Slide Images is a major criterion that contributes towards scoring tumour grade which then determines treatment options. However conventional manual assessment suffers from inter-operator reproducibility impacting grading precision. To address this, automatic necrosis detection using AI may be used to assess necrosis for final scoring that contributes towards the final clinical grade. Using deep learning AI, we describe a novel approach for automating necrosis detection in Whole Slide Images, tested on a canine Soft Tissue Sarcoma (cSTS) data set consisting of canine Perivascular Wall Tumours (cPWTs). A patch-based deep learning approach was developed where different variations of training a DenseNet-161 Convolutional Neural Network architecture were investigated as well as a stacking ensemble. An optimised DenseNet-161 with post-processing produced a hold-out test F1-score of 0.708 demonstrating state-of-the-art performance. This represents a novel first-time automated necrosis detection method in the cSTS domain as well specifically in detecting necrosis in cPWTs demonstrating a significant step forward in reproducible and reliable necrosis assessment for improving the precision of tumour grading.
Unravelling protein distributions within individual cells is vital to understanding their function and state and indispensable to developing new treatments. Here we present the Hybrid subCellular Protein Localiser (HCPL), which learns from weakly labelled data to robustly localise single-cell subcellular protein patterns. It comprises innovative DNN architectures exploiting wavelet filters and learnt parametric activations that successfully tackle drastic cell variability. HCPL features correlation-based ensembling of novel architectures that boosts performance and aids generalisation. Large-scale data annotation is made feasible by our AI-trains-AI approach, which determines the visual integrity of cells and emphasises reliable labels for efficient training. In the Human Protein Atlas context, we demonstrate that HCPL is best performing in the single-cell classification of protein localisation patterns. To better understand the inner workings of HCPL and assess its biological relevance, we analyse the contributions of each system component and dissect the emergent features from which the localisation predictions are derived. The Hybrid subCellular Protein Localiser (HCPL) improves single-cell classification for protein localization, allowing for large-scale data annotation through deep-learning architecture.
© 2012 Springer Science+Business Media, LLC. All rights reserved. MPEG-7, formally called ISO/IEC 15938 Multimedia Content Description Interface, is an international standard aimed at providing an interoperable solution to the description of various types of multimedia content, irrespective of their representation format. It is quite different from standards such as MPEG-1, MPEG-2 and MPEG-4, which aim to represent the content itself, since MPEG-7 aims to represent information about the content, known as metadata, so as to allow users to search, identify, navigate and browse audio-visual content more effectively. The MPEG-7 standard provides not only elementary visual and audio descriptors, but also multi-media description schemes, which combine the elementary audio and visual descriptors, a description definition language (DDL), and a binary compression scheme for the efficient compression and transportation of MPEG-7 metadata. In addition, reference software and conformance testing information are also part of the MPEG-7 standard and provide valuable tools to the development of standard-compliant systems.
Electronic publishing usually presents readers with book or e-book options for reading on paper or screen. In this paper, we introduce a third method of reading on paper-and-screen through the use of an augmented book (‘a-book’) with printed hotlinks than can be viewed on a nearby smartphone or other device. Two experimental versions of an augmented guide to Cornwall are shown using either optically recognised pages or embedded electronics making the book sensitive to light and touch. We refer to these as second generation (2G) and third generation (3G) paper respectively. A common architectural framework, authoring workflow and interaction model is used for both technologies, enabling the creation of two future generations of augmented books with interactive features and content. In the travel domain we use these features creatively to illustrate the printed book with local multimedia and updatable web media, to point to the printed pages from the digital content, and to record personal and web media into the book.
We compare experimentally the performance of three approaches to ensemble-based classification on general multi-class datasets. These are the methods of random forest, error-correcting output codes (ECOC) and ECOC enhanced by the use of bootstrapping and class-separability weighting (ECOC-BW). These experiments suggest that ECOC-BW yields better generalisation performance than either random forest or unmodified ECOC. A bias-variance analysis indicates that ECOC benefits from reduced bias, when compared to random forest, and that ECOC-BW benefits additionally from reduced variance. One disadvantage of ECOC-based algorithms, however, when compared with random forest, is that they impose a greater computational demand leading to longer training times.
We propose a novel CNN architecture called ACTNET for robust instance image retrieval from large-scale datasets. Our key innovation is a learnable activation layer designed to improve the signal-to-noise ratio of deep convolutional feature maps. Further, we introduce a controlled multi-stream aggregation, where complementary deep features from different convolutional layers are optimally transformed and balanced using our novel activation layers, before aggregation into a global descriptor. Importantly, the learnable parameters of our activation blocks are explicitly trained, together with the CNN parameters, in an end-to-end manner minimising triplet loss. This means that our network jointly learns the CNN filters and their optimal activation and aggregation for retrieval tasks. To our knowledge, this is the first time parametric functions have been used to control and learn optimal multi-stream aggregation. We conduct an in-depth experimental study on three non-linear activation functions: Sine-Hyperbolic, Exponential and modified Weibull, showing that while all bring significant gains the Weibull function performs best thanks to its ability to equalise strong activations. The results clearly demonstrate that our ACTNET architecture significantly enhances the discriminative power of deep features, improving significantly over the state-of-the-art retrieval results on all datasets.
This paper presents an approach for generating class-specific image segmentation. We introduce two novel features that use the quantized data of the Discrete Cosine Transform (DCT) in a Semantic Texton Forest based framework (STF), by combining together colour and texture information for semantic segmentation purpose. The combination of multiple features in a segmentation system is not a straightforward process. The proposed system is designed to exploit complementary features in a computationally efficient manner. Our DCT based features describe complex textures represented in the frequency domain and not just simple textures obtained using differences between intensity of pixels as in the classic STF approach. Differently than existing methods (e.g., filter bank) just a limited amount of resources is required. The proposed method has been tested on two popular databases: CamVid and MSRC-v2. Comparison with respect to recent state-of-the-art methods shows improvement in terms of semantic segmentation accuracy.
Visual search and image retrieval underpin numerous applications, however the task is still challenging predominantly due to the variability of object appearance and ever increasing size of the databases, often exceeding billions of images. Prior art methods rely on aggregation of local scale-invariant descriptors, such as SIFT, via mechanisms including Bag of Visual Words (BoW), Vector of Locally Aggregated Descriptors (VLAD) and Fisher Vectors (FV). However, their performance is still short of what is required. This paper presents a novel method for deriving a compact and distinctive representation of image content called Robust Visual Descriptor with Whitening (RVD-W). It significantly advances the state of the art and delivers world-class performance. In our approach local descriptors are rank-assigned to multiple clusters. Residual vectors are then computed in each cluster, normalized using a direction-preserving normalization function and aggregated based on the neighborhood rank. Importantly, the residual vectors are de-correlated and whitened in each cluster before aggregation, leading to a balanced energy distribution in each dimension and significantly improved performance. We also propose a new post-PCA normalization approach which improves separability between the matching and non-matching global descriptors. This new normalization benefits not only our RVD-W descriptor but also improves existing approaches based on FV and VLAD aggregation. Furthermore, we show that the aggregation framework developed using hand-crafted SIFT features also performs exceptionally well with Convolutional Neural Network (CNN) based features. The RVD-W pipeline outperforms state-of-the-art global descriptors on both the Holidays and Oxford datasets. On the large scale datasets, Holidays1M and Oxford1M, SIFT-based RVD-W representation obtains a mAP of 45.1% and 35.1%, while CNN-based RVD-W achieve a mAP of 63.5% and 44.8%, all yielding superior performance to the state-of-the-art.
This work addresses the problem of accurate semantic labelling of short videos. To this end, a multitude of different deep nets, ranging from traditional recurrent neural networks (LSTM, GRU), temporal agnostic networks (FV,VLAD,BoW), fully connected neural networks mid-stage AV fusion and others. Additionally, we also propose a residual architecture-based DNN for video classification, with state-of-the art classification performance at significantly reduced complexity. Furthermore, we propose four new approaches to diversity-driven multi-net ensembling, one based on fast correlation measure and three incorporating a DNN-based combiner. We show that significant performance gains can be achieved by ensembling diverse nets and we investigate factors contributing to high diversity. Based on the extensive YouTube8M dataset, we provide an in-depth evaluation and analysis of their behaviour. We show that the performance of the ensemble is state-of-the-art achieving the highest accuracy on the YouTube8M Kaggle test data. The performance of the ensemble of classifiers was also evaluated on the HMDB51 and UCF101 datasets, and show that the resulting method achieves comparable accuracy with state-ofthe- art methods using similar input features.
This paper presents the core technologies of the Video Signature Tools recently standardized by ISO/IEC MPEG as an amendment to the MPEG-7 Standard (ISO/IEC 15938). The Video Signature is a high-performance content fingerprint which is suitable for desktop-scale to web-scale deployment and provides high levels of robustness to common video editing operations and high temporal localization accuracy at extremely low false alarm rates, achieving a detection rate in the order of 96% at a false alarm rate in the order of five false matches per million comparisons. The applications of the Video Signature are numerous and include rights management and monetization, distribution management, usage monitoring, metadata association, and corporate or personal database management. In this paper we review the prior work in the field, we explain the standardization process and status, and we provide the details and evaluation results of the Video Signature Tools.
Visual semantic information comprises two important parts: the meaning of each visual semantic unit and the coherent visual semantic relation conveyed by these visual semantic units. Essentially, the former one is a visual perception task while the latter one corresponds to visual context reasoning. Remarkable advances in visual perception have been achieved due to the success of deep learning. In contrast, visual semantic information pursuit, a visual scene semantic interpretation task combining visual perception and visual context reasoning, is still in its early stage. It is the core task of many different computer vision applications, such as object detection, visual semantic segmentation, visual relationship detection or scene graph generation. Since it helps to enhance the accuracy and the consistency of the resulting interpretation, visual context reasoning is often incorporated with visual perception in current deep end-to-end visual semantic information pursuit methods. Surprisingly, a comprehensive review for this exciting area is still lacking. In this survey, we present a unified theoretical paradigm for all these methods, followed by an overview of the major developments and the future trends in each potential direction. The common benchmark datasets, the evaluation metrics and the comparisons of the corresponding methods are also introduced.
Local feature descriptors underpin many diverse applications, supporting object recognition, image registration, database search, 3D reconstruction and more. The recent phenomenal growth in mobile devices and mobile computing in general has created demand for descriptors that are not only discriminative, but also compact in size and fast to extract and match. In response, a large number of binary descriptors have been proposed, each claiming to overcome some limitations of the predecessors. This paper provides a comprehensive evaluation of several promising binary designs. We show that existing evaluation methodologies are not sufficient to fully characterize descriptors’ performance and propose a new evaluation protocol and a challenging dataset. In contrast to the previous reviews, we investigate the effects of the matching criteria, operating points and compaction methods, showing that they all have a major impact on the systems’ design and performance. Finally, we provide descriptor extraction times for both general-purpose systems and mobile devices, in order to better understand the real complexity of the extraction task. The objective is to provide a comprehensive reference and a guide that will help in selection and design of the future descriptors.
This paper addresses the problem of very large-scale image retrieval, focusing on improving its accuracy and robustness. We target enhanced robustness of search to factors such as variations in illumination, object appearance and scale, partial occlusions, and cluttered backgrounds -particularly important when search is performed across very large datasets with significant variability. We propose a novel CNN-based global descriptor, called REMAP, which learns and aggregates a hierarchy of deep features from multiple CNN layers, and is trained end-to-end with a triplet loss. REMAP explicitly learns discriminative features which are mutually-supportive and complementary at various semantic levels of visual abstraction. These dense local features are max-pooled spatially at each layer, within multi-scale overlapping regions, before aggregation into a single image-level descriptor. To identify the semantically useful regions and layers for retrieval, we propose to measure the information gain of each region and layer using KL-divergence. Our system effectively learns during training how useful various regions and layers are and weights them accordingly. We show that such relative entropy-guided aggregation outperforms classical CNN-based aggregation controlled by SGD. The entire framework is trained in an end-to-end fashion, outperforming the latest state-of-the-art results. On image retrieval datasets Holidays, Oxford and MPEG, the REMAP descriptor achieves mAP of 95.5%, 91.5% and 80.1% respectively, outperforming any results published to date. REMAP also formed the core of the winning submission to the Google Landmark Retrieval Challenge on Kaggle.
Images of the kidneys using dynamic contrast-enhanced magnetic resonance renography (DCE-MRR) contains unwanted complex organ motion due to respiration. This gives rise to motion artefacts that hinder the clinical assessment of kidney function. However, due to the rapid change in contrast agent within the DCE-MR image sequence, commonly used intensity-based image registration techniques are likely to fail. While semi-automated approaches involving human experts are a possible alternative, they pose significant drawbacks including inter-observer variability, and the bottleneck introduced through manual inspection of the multiplicity of images produced during a DCE-MRR study. To address this issue, we present a novel automated, registration-free movement correction approach based on windowed and reconstruction variants of dynamic mode decomposition (WR-DMD). Our proposed method is validated on ten different healthy volunteers’ kidney DCE-MRI data sets. The results, using block-matching-block evaluation on the image sequence produced by WR-DMD, show the elimination of 99%99% of mean motion magnitude when compared to the original data sets, thereby demonstrating the viability of automatic movement correction using WR-DMD.
A Machine Learning approach to the problem of calculating the proton paths inside a scanned object in proton Computed Tomography is presented. The method is developed in order to mitigate the loss in both spatial resolution and quantitative integrity of the reconstructed images caused by multiple Coulomb scattering of protons traversing the matter. Two Machine Learning models were used: a forward neural network and the XGBoost method. A heuristic approach, based on track averaging was also implemented in order to evaluate the accuracy limits on track calculation, imposed by the statistical nature of the scattering. Synthetic data from anthropomorphic voxelized phantoms, generated by the Monte Carlo Geant4 code, were utilised to train the models and evaluate their accuracy, in comparison to a widely used analytical method that is based on likelihood maximization and Fermi-Eyges scattering model. Both neural network and XGBoost model were found to perform very close or at the accuracy limit, further improving the accuracy of the analytical method (by 12% in the typical case of 200MeV protons on 20 cm of water object), especially for protons scattered at large angles. Inclusion of the material information along the path in terms of radiation length did not show improvement in accuracy, for the phantoms simulated in the study. A neural network was also constructed to predict the error in path calculation, thus enabling a criterion to filter out proton events that may have a negative effect on the quality of the reconstructed image. By parametrizing a large set of synthetic data, the Machine Learning models were proved capable to bring - in an indirect and time efficient way - the accuracy of the Monte Carlo method into the problem of proton tracking.
This paper is concerned with design of a compact, binary and scalable image representation that is easy to compute, fast to match and delivers beyond state-of-the-art performance in visual recognition of objects, buildings and scenes. A novel descriptor is proposed which combines rank-based multi-assignment with robust aggregation framework and cluster/bit selection mechanisms for size scalability. Extensive performance evaluation is presented, including experiments within the state-of-the art pipeline developed by the MPEG group standardising Compact Descriptors for Visual Search (CVDS).
This paper addresses the problem of ultra-large-scale search in Hamming spaces. There has been considerable research on generating compact binary codes in vision, for example for visual search tasks. However the issue of efficient searching through huge sets of binary codes remains largely unsolved. To this end, we propose a novel, unsupervised approach to thresholded search in Hamming space, supporting long codes (e.g. 512-bits) with a wide-range of Hamming distance radii. Our method is capable of working efficiently with billions of codes delivering between one to three orders of magnitude acceleration, as compared to prior art. This is achieved by relaxing the equal-size constraint in the Multi-Index Hashing approach, leading to multiple hash-tables with variable length hash-keys. Based on the theoretical analysis of the retrieval probabilities of multiple hash-tables we propose a novel search algorithm for obtaining a suitable set of hash-key lengths. The resulting retrieval mechanism is shown empirically to improve the efficiency over the state-of-the-art, across a range of datasets, bit-depths and retrieval thresholds.
Recent studies have shown that it is possible to attack a finger vein (FV) based biometric system using printed materials. In this study, we propose a novel method to detect spoofing of static finger vein images using Windowed Dynamic mode decomposition (W-DMD). This is an atemporal variant of the recently proposed Dynamic Mode Decomposition for image sequences. The proposed method achieves better results when compared to established methods such as local binary patterns (LBP), discrete wavelet transforms (DWT), histogram of gradients (HoG), and filter methods such as range-filters, standard deviation filters (STD) and entropy filters, when using SVM with a minimum intersection kernel. The overall pipeline which consists ofW-DMD and SVM, proves to be efficient, and convenient to use, given the absence of additional parameter tuning requirements. The effectiveness of our methodology is demonstrated using FV-Spoofing-Attack database which is publicly available. Our test results show that W-DMD can successfully detect printed finger vein images because they contain micro-level artefacts that not only differ in quality but also in light reflection properties compared to valid/live finger vein images.
Multiple cues play a crucial role in image interpretation. A vision system that combines shape, colour, motion, prior scene knowledge and object motion behaviour is described. We show that the use of interpretation strategies which depend on the image data, temporal context and visual goals significantly simplifies the complexity of the image interpretation problem and makes it computationally feasible.
A seat occupant monitoring apparatus which includes a sensor reactive to a force distribution applied to the seat by the occupant, means for making a plurality of measurements of the force distribution and means for monitoring the occupant based on the measurements. The measurements are used to classify the occupant and on a basis of the classification, parameters of the occupant's environment such as a seat orientation, the rate of deployment of an air bag or control of a seat belt pre-tensioner are altered. A neural network or other learning based technique is used for the classification.
© Springer-Verlag Berlin Heidelberg 1997.Phase correlation techniques have been used in image registration to estimate image displacements. These techniques have been also used to estimate optical flow by applying it locally. In this work a different phase correlation-based method is proposed to deal with a deformation/translation motion model, instead of the pure translations that the basic phase correlation technique can estimate. Some experimentals results are also presented to show the accuracy of the motion paramenters estimated and the use of the phase correlation to estimate optical flow.
The complete theory for Fisher and dual discriminant analysis is presented as the background of the novel algorithms. LDA is found as composition of projection onto the singular subspace for within-class normalised data with the projection onto the singular subspace for between-class normalised data. The dual LDA consists of those projections applied in reverse order. The experiments show that using suitable composition of dual LDA transformations gives as least as good results as recent state-of-the-art solutions.
MPEG-7 is the first international standard which contains a number of key techniques from Computer Vision and Image Processing. The Curvature Scale Space technique was selected as a contour shape descriptor for MPEG-7 after substantial and comprehensive testing, which demonstrated the superior performance of the CSS-based descriptor. Curvature Scale Space Representation: Theory, Applications, and MPEG-7 Standardization is based on key publications on the CSS technique, as well as its multiple applications and generalizations. The goal was to ensure that the reader will have access to the most fundamental results concerning the CSS method in one volume. These results have been categorized into a number of chapters to reflect their focus as well as content. The book also includes a chapter on the development of the CSS technique within MPEG standardization, including details of the MPEG-7 testing and evaluation processes which led to the selection of the CSS shape descriptor for the standard. The book can be used as a supplementary textbook by any university or institution offering courses in computer and information science.
© Springer-Verlag Berlin Heidelberg 1996.The paper presents a novel approach to the Robust Analysis of Complex Motion. It employs a low-level robust motion estimator, conceptually based on the Hough Transform, and uses Multiresolution Markov Random Fields for the global interpretation of the local, low-level estimates. Motion segmentation is performed in the front-end estimator, in parallel with the motion parameter estimation process. This significantly improves the accuracy of estimates, particularly in the vicinity of motion boundaries, facilitates the detection of such boundaries, and allows the use of larger regions, thus improving robustness. The measurements extracted from the sequence in the front-end estimator include displacement, the spatial derivatives of the displacement, confidence measures, and the location of motion boundaries. The measurements are then combined within the MRF framework, employing the supercoupling approach for fast convergence. The excellent performance, in terms of estimate accuracy, boundary detection and robustness is demonstrated on synthetic and real-word sequences.
The use of a robust, low-level motion estimator based on a Robust Hough Transform (RHT) in a range of tasks, such as optical flow estimation, and motion estimation for video coding and retrieval from video sequences was discussed. RHT derived not only pixels displacements, but also provided direct motion segmentation and other motion-related clues. The RHT algorithm employed an affine region-to-region transformation model and was invariant to illumination changes, in addition to being statistically robust. It was found that RHT did not base the correspondence analyses on any specific type of feature, but used textured regions in the image as non-localized features.
Linear Discriminant Analysis (LDA) is a popular feature extraction technique that aims at creating a feature set of enhanced discriminatory power. The authors introduced a novel approach Dual LDA (DLDA) and proposed an efficient SVD-based implementation. This paper focuses on feature space reduction aspect of DLDA achieved in course of proper choice of the parameters controlling the DLDA algorithm. The comparative experiments conducted on a collection of five facial databases consisting in total of more than 10000 photos show that DLDA outperforms by a great margin the methods reducing the feature space by means of feature subset selection. © 2005 IEEE.
A method of representing a group of data items comprises, for each of a plurality of data items in the group, determining the similarity between said data item and each of a plurality of other data items in the group, assigning a rank to each pair on the basis of similarity, wherein the ranked similarity values for each of said plurality of data items are associated to reflect the overall relative similarities of data items in the group.
The incorporation of intelligent video processing algorithms into digital surveillance systems has been examined in this work. In particular, the use of the latest standard in multi-media feature extraction and matching is discussed. The use of such technology makes a system very different to current surveillance systems which store text-based meta-data. In our system, descriptions based upon shape and colour are extracted in real-time from two sequences of video recorded from a real-life scenario. The stored database of descriptions can then be searched using a query description constructed by the operator; this query is then compared with every description stored for the video sequence. We show examples of the fast and accurate search made possible with this latest technology for multimedia content description applied to a video surveillance database.
We present an FPGA face detection and tracking system for audiovisual communications, with a particular focus on mobile videoconferencing. The advantages of deploying such a technology in a mobile handset are many, including face stabilisation, reduced bitrate, and higher quality video on practical display sizes. Most face detection methods, however, assume at least modest general purpose processing capabilities, making them inappropriate for real-time applications, especially for power-limited devices,as well as modestcustom hardware implementations. We present a method which achieves a very high detection and tracking performance and, at the same time, entails a significantly reduced computational complexity, allowing real-time implementations on custom hardware or simple microprocessors. We then propose an FPGA implementation which entails very low logic and memory costs and achieves extremely high processing rates at very low clock speeds.
© Springer-Verlag Berlin Heidelberg 1997.This paper is concerned with an efficient estimation and segmentation of 2-D motion from image sequences, with the focus on traffic monitoring applications. In order to reduce the computational load and facilitate real-time implementation, the proposed approach makes use of simplifying assumptions that the camera is stationary and that the projection of vehicles motion on the image plane can be approximated by translation. We show that a good performance can be achieved even under such apparently restrictive assumptions. To further reduce processing time, we perform gray-level based segmentation that extracts regions of uniform intensity. Subsequently, we estimate motion for the regions. Regions moving with the coherent motion are allowed to merge. The use of 2D motion analysis and the pre-segmentation stage significantly reduces the computational load, and the region-based estimator gives robustness to noise and changes of illumination.
This paper addresses a problem of robust, accurate and fast object detection in complex environments, such as cluttered backgrounds and low-quality images. To overcome the problems with existing methods, we propose a new object detection approach, called Statistical Template Matching. It is based on generalized description of the object by a set of template regions and statistical testing of object/non-object hypotheses. A similarity measure between the image and a template is derived from the Fisher criterion. We show how to apply our method to face and facial feature detection tasks, and demonstrate its performance in some difficult cases, such as moderate variation of scale factor of the object, local image warping and distortions caused by image compression. The method is very fast; its speed is independent of the template size and depends only on the template complexity.
Transmission of compressed video over error prone channels such as mobile networks is a challenging issue. Maintaining an acceptable quality of service in such an environment demands additional post-processing tools to limit the impact of uncorrected transmission errors. Significant visual degradation of a video stream occurs when the motion vector component is corrupted. In this paper, an effective and computationally efficient method for the recovery of lost motion vectors (MVs) is proposed. The novel idea selects a neighbouring block MV that has the minimum distance from an estimated MV. Simulation results are presented, including comparison with existing methods. Our method follows the performance of the best existing method by approximately 0.1-0.5 dB. However, it has a significant advantage in that it is 50% computationally simpler. This makes our method ideal for use in mobile handsets and other applications with limited processing power.
This paper presents work in progress of the European Commission FP7 project BRIDGET "BRIDging the Gap for Enhanced broadcasT". The project is developing innovative technology and the underlying architecture for efficient production of second screen applications for broadcasters and media companies. The project advancements include novel front-end authoring tools as well as back-end enabling technologies such as visual search, media structure analysis and 3D A/V reconstruction to support new editorial workflows.
This paper addresses the problem of aggregating local binary descriptors for large scale image retrieval in mobile scenarios. Binary descriptors are becoming increasingly popular, especially in mobile applications, as they deliver high matching speed, have a small memory footprint and are fast to extract. However, little research has been done on how to efficiently aggregate binary descriptors. Direct application of methods developed for conventional descriptors, such as SIFT, results in unsatisfactory performance. In this paper we introduce and evaluate several algorithms to compress high-dimensional binary local descriptors, for efficient retrieval in large databases. In addition, we propose a robust global image representation; Binary Robust Visual Descriptor (B-RVD), with rank-based multi-assignment of local descriptors and direction-based aggregation, achieved by the use of L1-norm on residual vectors. The performance of the B-RVD is further improved by balancing the variances of residual vector directions in order to maximize the discriminatory power of the aggregated vectors. Standard datasets and measures have been used for evaluation showing significant improvement of around 4% mean Average Precision as compared to the state-of-the-art.
Fisher linear discriminant analysis (FLDA) based on variance ratio is compared with scatter linear discriminant (SLDA) analysis based on determinant ratio. It is shown that each optimal FLDA data model is optimal SLDA data model but not opposite. The novel algorithm 2SS4LDA (two singular subspaces for LDA) is presented using two singular value decompositions applied directly to normalized multiclass input data matrix and normalized class means data matrix. It is controlled by two singular subspace dimension parameters q and r, respectively. It appears in face recognition experiments on the union of MPEG-7, Altkom, and Feret facial databases that 2SS4LDA reaches about 94% person identification rate and about 0.21 average normalized mean retrieval rank. The best face recognition performance measures are achieved for those combinations of q, r values for which the variance ratio is close to its maximum, too. None such correlation is observed for SLDA separation measure. © Springer-Verlag Berlin Heidelberg 2003.
Introduction to MPEG-7 takes a systematic approach to the standard and provides a unique overview of the principles and concepts behind audio-visual indexing, ...
© Springer-Verlag Berlin Heidelberg 2001.The ISO MPEG-7 Standard, also known as a Multimedia Content Description Interface, will be soon finalized. After several years of intensive work on technology development, implementation and testing by almost all major players in the digital multimedia arena, the results of this international project will be assessed by the most cruel and demanding judge: the market. Will it meet all the high expectations of the developers and, above all, future users? Will it result in a revolution, evolution or will it just simply pass unnoticed? In this invited lecture, I will review the components of the MPEG-7 Standard in the context of some novel applications. I will go beyond the classical image/ video retrieval scenarios, and look into more generic image/object recognition framework relying on the MPEG-7 technology. Such a framework is applicable to a wide range of new applications. The benefits of using standardized technology, over other state-of-the art techniques from computer vision, image processing, and database retrieval, will be investigated. Demonstrations of the generic object recognition system will be presented, followed by some other examples of emerging applications made possible by the Standard. In conclusion, I will assess the potential impact of this new standard on emerging services, products and future technology developments.
Despite the rise of digital photography, physical photos remain significant. They support social practices for maintaining social bonds, particularly in family contexts as their handling can trigger emotions associated with the individuals and themes depicted. Also, digital media can be used to strengthen the meaning of physical objects and environments represented in the material world through augmented reality, where such are overlaid with additional digital information that provide supplementary sensory context to topics conveyed. This poster therefore presents initial findings from the development of augmented photobooks to create ‘a-photobooks’, printed photobooks that are augmented by travellers with additional multimedia of their trip using a smartphone-based authoring tool. Results suggest a-photobooks could support more immersive engagement and reminiscing of holidays encounters, increasing cognitive, and emotional effects of associated experiences.
A method of detecting an object in an image comprises comparing a template with a region of an image and determining a similarity measure, wherein the similarity measure is determined using a statistical measure. The template comprises a number of regions corresponding to parts of the object and their spatial relations. The variance of the pixels within the total template is set in relation to the variances of the pixels in all individual regions, to provide a similarity measure.