Dr Muhammad Awais
Academic and research departments
Surrey Institute for People-Centred Artificial Intelligence (PAI), Centre for Vision, Speech and Signal Processing (CVSSP).About
Biography
I was lucky to be part of the research (together with colleagues Sara Atito and Josef Kittler) which resulted in the first state-of-the-art (SOTA) masked image modelling (MIM) approach for vision transformers using the simple principles of heavy masking and recovery of information without using human annotated labels. Proposed MIM outperformed all existing self-supervised learning (SSL) SOTA methods including joint embedding-based architectures. It marked a milestone in computer vision to become the first method which used self-supervised pretraining (SSP) to outperform supervised pretraining (SP). According to leading Artificial Intelligence (AI) researcher and chief AI scientist of Meta, Yann LeCun the MIM has revolutionised the SSL. To put it in context I will introduce the challenges of AI and SSL first and then the breakthrough.
The Challenge: AI has seen a phenomenal growth over last decade mainly thanks to Supervised Learning (SL) and supervised pretraining (SP) of deep neural networks (DNNs). The growth of AI was somewhat stagnated after the first few years due to lack of available labelled data for training DNNs. Tech giants' solution to the problem was to collect millions or even billions of weakly labelled samples to pretrain DNNs. Despite that there was an anticipation that Un/Self-Supervised Learning (SSL), i.e., learning without human annotated labels is the way forward in AI due to closeness of SSL to human-like learning. In the words Yann LeCun, “The revolution will not be supervised”. However, the problem with self-supervised pretraining (SSP) of DNNs was that it did not outperform supervised pretraining (SP) of DNNs for downstream tasks particularly in computer vision despite significant efforts from tech giants and top researchers in AI. In 2018 SSL has seen huge success in natural language processing (NLP) by models like BERT and GPT which are trained using masked language modelling (MLM) and auto regressive generative pretraining. However, this principle of MLM was not easy to adopt to computer vision as indicated by Yann LeCun in the March 2021 blog (The dark matter of intelligence), “But we cannot use this trick (MLM) for images because we cannot enumerate all possible images. Is there a solution to this problem? The short answer is no. There are interesting ideas in this direction, but they have not yet led to results that are as good as joint embedding architectures.”.
The breakthrough: At the beginning of 2021, we conducted the research on first working version of masked image modelling (MIM) which we dubbed as GMML (Group Masked Model Learning) in our seminal work SiT (Self-Supervised vIsion Transformers; released on 8th of April 2021 along with code). GMML marked a milestone in computer vision by being the first SSP method which outperformed SP across multiple tasks and also outperformed all existing SSL methods including joint embedding architectures. In this sense, GMML is the first working foundation model for vision (image only) modality. Prior to GMML, the SSL methods in computer vision applications were unsustainable and mainly limited to large groups and AI tech giants due to complexities of SSL algorithms, like requirements of huge resources because of large batch size, DNNs model size, dataset size etc. GMML democratized and made SSL sustainable by enabling SSP using a single GPU and on small datasets. In fact, the principles of GMML, i.e., heavy masking and recovery of information is shown to be the best way to utilise information for both small/medium amount of data by our research group as well as later for large datasets with large models by tech giants like Microsoft, Meta, Nvidia etc. The remarkable strength of GMML over SOTA SSL including joint-embedding based methods can be seen when combined with self-supervised clustering methods, the GMML show remarkable improvements over joint-embedding based SOTA methods which were proposed by tech giants. The tweet of Yann LeCun in March 2023 acknowledges that SSL for computer vision is revolutionised by MIM, the principles which we laid down at the beginning of 2021.
I am a senior lecturer (associate professor) jointly at Centre for Vision Speech & Signal Processing (CVSSP) and Surrey Institute for People Centred AI (SI-PAI) where I lead the research on foundation models and self-supervised learning. I am also responsible for technical aspects of trustworthy and explainable AI.
Building next generation AI algorithms is futile unless it benefits society. Therefore, I pivot the fundamental AI development with applications for the benefit of society. This is evident from the applicability of my research to a range of applications areas from healthcare to security. An example is manifested in terms of a startup Sensus Futuris which I co-founded with Prof. Kittler. The focus of Sensus Futuris is to use innovative AI algorithms to make society safer and more efficient. Another aspect of people-centred AI is evident from industrial fundings (e.g., innovateUK, ignite etc.) I have co-designed and co-led with a particular focus on the people-centred nature of the AI products. Some of the AI algorithms we worked on (at Imperial College London’s startup) were deployed on huge scales at eBay, Macy’s, Zalando etc. for recommending visually related items to their users. My experience of working in both industry and academia places me well to develop innovative AI algorithms and advance their theoretical underpinning as well as do technology transfer and have bigger impact on society.
ResearchResearch interests
The focus of my research is on core AI/ML/DL algorithms and their applicability to a wide range of application areas. My research interests include foundation models, un/self-supervised learning, cross/multi-modal learning, theoretical insights and understanding of deep learning, computer vision, NLP, medical image analysis, audio, retrieval, biometrics, security.
You can find some of interesting research work in my google scholar profile (Note that it is usually not up to date because if I enable auto-update I start to receive dozens of papers not belonging to me. I very occasionally find the time to update and add a few interesting papers).
Research interests
The focus of my research is on core AI/ML/DL algorithms and their applicability to a wide range of application areas. My research interests include foundation models, un/self-supervised learning, cross/multi-modal learning, theoretical insights and understanding of deep learning, computer vision, NLP, medical image analysis, audio, retrieval, biometrics, security.
You can find some of interesting research work in my google scholar profile (Note that it is usually not up to date because if I enable auto-update I start to receive dozens of papers not belonging to me. I very occasionally find the time to update and add a few interesting papers).
Sustainable development goals
My research interests are related to the following:
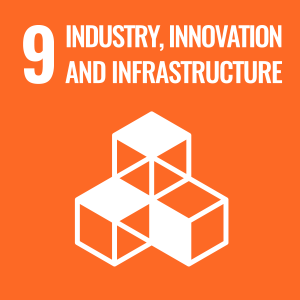
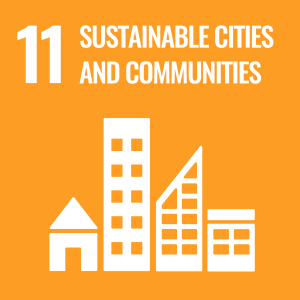
Publications
—Label distribution Learning (LDL) is the state-of-the-art approach to deal with a number of real-world applications , such as chronological age estimation from a face image, where there is an inherent similarity among adjacent age labels. LDL takes into account the semantic similarity by assigning a label distribution to each instance. The well-known Kullback–Leibler (KL) divergence is the widely used loss function for the LDL framework. However, the KL divergence does not fully and effectively capture the semantic similarity among age labels, thus leading to the sub-optimal performance. In this paper, we propose a novel loss function based on optimal transport theory for the LDL-based age estimation. A ground metric function plays an important role in the optimal transport formulation. It should be carefully determined based on underlying geometric structure of the label space of the application in-hand. The label space in the age estimation problem has a specific geometric structure, i.e. closer ages have more inherent semantic relationship. Inspired by this, we devise a novel ground metric function, which enables the loss function to increase the influence of highly correlated ages; thus exploiting the semantic similarity among ages more effectively than the existing loss functions. We then use the proposed loss function, namely γ–Wasserstein loss, for training a deep neural network (DNN). This leads to a notoriously computationally expensive and non-convex optimisa-tion problem. Following the standard methodology, we formulate the optimisation function as a convex problem and then use an efficient iterative algorithm to update the parameters of the DNN. Extensive experiments in age estimation on different benchmark datasets validate the effectiveness of the proposed method, which consistently outperforms state-of-the-art approaches.
Machine learning, including deep learning, reinforcement learning, and generative artificial intelligence are revolutionising every area of our lives when data are made available. With the help of these methods, we can decipher information from larger datasets while addressing the complex nature of biological systems in a more efficient way. Although machine learning methods have been introduced to human genetic epidemiological research as early as 2004, those were never used to their full capacity. In this review, we outline some of the main applications of machine learning to assigning human genetic loci to health outcomes. We summarise widely used methods and discuss their advantages and challenges. We also identify several tools, such as Combi, GenNet, and GMSTool, specifically designed to integrate these methods for hypothesis-free analysis of genetic variation data. We elaborate on the additional value and limitations of these tools from a geneticist’s perspective. Finally, we discuss the fast-moving field of foundation models and large multi-modal omics biobank initiatives.
In recent years, 3D facial reconstructions from single images have garnered significant interest. Most of the approaches are based on 3D Morphable Model (3DMM) fitting to reconstruct the 3D face shape. Concurrently, the adoption of Generative Adversarial Networks (GAN) has been gaining momentum to improve the texture of reconstructed faces. In this paper, we propose a fundamentally different approach to reconstructing the 3D head shape from a single image by harnessing the power of GAN. Our method predicts three maps of normal vectors of the head’s frontal, left, and right poses. We are thus presenting a model-free method that does not require any prior knowledge of the object’s geometry to be reconstructed. The key advantage of our proposed approach is the substantial improvement in reconstruction quality compared to existing methods, particularly in the case of facial regions that are self-occluded in the input image. Our method is not limited to 3d face reconstruction. It is generic and applicable to multiple kinds of 3D objects. To illustrate the versatility of our method, we demonstrate its efficacy in reconstructing the entire human body. By delivering a model-free method capable of generating high-quality 3D reconstructions, this paper not only advances the field of 3D facial reconstruction but also provides a foundation for future research and applications spanning multiple object types. The implications of this work have the potential to extend far beyond facial reconstruction, paving the way for innovative solutions and discoveries in various domains.
Cross-modal content generation has become very popular in recent years. To generate high-quality and realistic content, a variety of methods have been proposed. Among these approaches, visual content generation has attracted significant attention from academia and industry due to its vast potential in various applications. This survey provides an overview of recent advances in visual content generation conditioned on other modalities, such as text, audio, speech, and music, with a focus on their key contributions to the community. In addition, we summarize the existing publicly available datasets that can be used for training and benchmarking cross-modal visual content generation models. We provide an in-depth exploration of the datasets used for audio-to-visual content generation, filling a gap in the existing literature. Various evaluation metrics are also introduced along with the datasets. Furthermore, we discuss the challenges and limitations encountered in the area, such as modality alignment and semantic coherence. Last, we outline possible future directions for synthesizing visual content from other modalities including the exploration of new modalities, and the development of multi-task multi-modal networks. This survey serves as a resource for researchers interested in quickly gaining insights into this burgeoning field.
Recently, impressively growing efforts have been devoted to the challenging task of facial age estimation. The improvements in performance achieved by new algorithms are measured on several benchmarking test databases with different characteristics to check on consistency. While this is a valuable methodology in itself, a significant issue in the most age estimation related studies is that the reported results lack an assessment of intrinsic system uncertainty. Hence, a more in-depth view is required to examine the robustness of age estimation systems in different scenarios. The purpose of this paper is to conduct an evaluative and comparative analysis of different age estimation systems to identify trends, as well as the points of their critical vulnerability. In particular, we investigate four age estimation systems, including the online Microsoft service, two best state-of-the-art approaches advocated in the literature, as well as a novel age estimation algorithm. We analyse the effect of different internal and external factors, including gender, ethnicity, expression, makeup, illumination conditions, quality and resolution of the face images, on the performance of these age estimation systems. The goal of this sensitivity analysis is to provide the biometrics community with the insight and understanding of the critical subject-, camera- and environmental-based factors that affect the overall performance of the age estimation system under study.
Contrastive learning has achieved great success in skeleton-based action recognition. However, most existing approaches encode the skeleton sequences as entangled spatiotemporal representations and confine the contrasts to the same level of representation. Instead, this paper introduces a novel contrastive learning framework, namely Spatiotemporal Clues Disentanglement Network (SCD-Net). Specifically, we integrate the decoupling module with a feature extractor to derive explicit clues from spatial and temporal domains respectively. As for the training of SCD-Net, with a constructed global anchor, we encourage the interaction between the anchor and extracted clues. Further, we propose a new masking strategy with structural constraints to strengthen the contextual associations, leveraging the latest development from masked image modelling into the proposed SCD-Net. We conduct extensive evaluations on the NTU-RGB+D (60&120) and PKU-MMD (I&II) datasets, covering various downstream tasks such as action recognition, action retrieval, transfer learning, and semi-supervised learning. The experimental results demonstrate the effectiveness of our method, which outperforms the existing state-of-the-art (SOTA) approaches significantly.
Self-supervised pretraining (SSP) has emerged as a popular technique in machine learning, enabling the extraction of meaningful feature representations without labelled data. In the realm of computer vision, pretrained vision transformers (ViTs) have played a pivotal role in advancing transfer learning. Nonetheless, the escalating cost of finetuning these large models has posed a challenge due to the explosion of model size. This study endeavours to evaluate the effectiveness of pure self-supervised learning (SSL) techniques in computer vision tasks, obviating the need for finetuning, with the intention of emulating human-like capabilities in generalisation and recognition of unseen objects. To this end, we propose an evaluation protocol for zero-shot segmentation based on a prompting patch. Given a point on the target object as a prompt, the algorithm calculates the similarity map between the selected patch and other patches, upon that, a simple thresholding is applied to segment the target. Another evaluation is intra-object and inter-object similarity to gauge discriminatory ability of SSP ViTs. Insights from zero-shot segmentation from prompting and discriminatory abilities of SSP led to the design of a simple SSP approach, termed MMC. This approaches combines Masked image modelling for encouraging similarity of local features, Momentum based self-distillation for transferring semantics from global to local features, and global Contrast for promoting semantics of global features, to enhance discriminative representations of SSP ViTs. Consequently, our proposed method significantly reduces the overlap of intra-object and inter-object similarities, thereby facilitating effective object segmentation within an image. Our experiments reveal that MMC delivers top-tier results in zero-shot semantic segmentation across various datasets.
Transformers, which were originally developed for natural language processing, have recently generated significant interest in the computer vision and audio communities due to their flexibility in learning long-range relationships. Constrained by the data hungry nature of transformers and the limited amount of labelled data, most transformer-based models for audio tasks are finetuned from ImageNet pretrained models, despite the huge gap between the domain of natural images and audio. This has motivated the research in self-supervised pretraining of audio transformers, which reduces the dependency on large amounts of labeled data and focuses on extracting concise representations of audio spectrograms. In this paper, we propose L ocal- G lobal A udio S pectrogram v I sion T ransformer, namely ASiT, a novel self-supervised learning framework that captures local and global contextual information by employing group masked model learning and self-distillation. We evaluate our pretrained models on both audio and speech classification tasks, including audio event classification, keyword spotting, and speaker identification. We further conduct comprehensive ablation studies, including evaluations of different pretraining strategies. The proposed ASiT framework significantly boosts the performance on all tasks and sets a new state-of-the-art performance in five audio and speech classification tasks, outperforming recent methods, including the approaches that use additional datasets for pretraining.
Modern facial age estimation systems can achieve high accuracy when training and test datasets are identically distributed and captured under similar conditions. However, domain shifts in data, encountered in practice, lead to a sharp drop in accuracy of most existing age estimation algorithms. In this work, we propose a novel method, namely RAgE, to improve the robustness and reduce the uncertainty of age estimates by leveraging unlabelled data through a subject anchoring strategy and a novel consistency regularisation term. First, we propose an similarity-preserving pseudo-labelling algorithm by which the model generates pseudo-labels for a cohort of unlabelled images belonging to the same subject, while taking into account the similarity among age labels. In order to improve the robustness of the system, a consistency regularisation term is then used to simultaneously encourage the model to produce invariant outputs for the images in the cohort with respect to an anchor image. We propose a novel consistency regularisation term the noise-tolerant property of which effectively mitigates the so-called confirmation bias caused by incorrect pseudo-labels. Experiments on multiple benchmark ageing datasets demonstrate substantial improvements over the state-of-the-art methods and robustness to confounding external factors, including subject's head pose, illumination variation and appearance of expression in the face image.
We propose supervised spatial attention that employs a heatmap generator for instructive feature learning.•We formulate a rectified Gaussian scoring function to generate informative heatmaps.•We present scale-aware layer attention that eliminates redundant information from pyramid features.•A voting strategy is designed to produce more reliable classification results.•Our face detector achieves encouraging performance in accuracy and speed on several benchmarks. Modern anchor-based face detectors learn discriminative features using large-capacity networks and extensive anchor settings. In spite of their promising results, they are not without problems. First, most anchors extract redundant features from the background. As a consequence, the performance improvements are achieved at the expense of a disproportionate computational complexity. Second, the predicted face boxes are only distinguished by a classifier supervised by pre-defined positive, negative and ignored anchors. This strategy may ignore potential contributions from cohorts of anchors labelled negative/ignored during inference simply because of their inferior initialisation, although they can regress well to a target. In other words, true positives and representative features may get filtered out by unreliable confidence scores. To deal with the first concern and achieve more efficient face detection, we propose a Heatmap-assisted Spatial Attention (HSA) module and a Scale-aware Layer Attention (SLA) module to extract informative features using lower computational costs. To be specific, SLA incorporates the information from all the feature pyramid layers, weighted adaptively to remove redundant layers. HSA predicts a reshaped Gaussian heatmap and employs it to facilitate a spatial feature selection by better highlighting facial areas. For more reliable decision-making, we merge the predicted heatmap scores and classification results by voting. Since our heatmap scores are based on the distance to the face centres, they are able to retain all the well-regressed anchors. The experiments obtained on several well-known benchmarks demonstrate the merits of the proposed method.
In this paper, we address the problem of bird audio detection and propose a new convolutional neural network architecture together with a divergence based information channel weighing strategy in order to achieve improved state-of-the-art performance and faster convergence. The effectiveness of the methodology is shown on the Bird Audio Detection Challenge 2018 (Detection and Classification of Acoustic Scenes and Events Challenge, Task 3) development data set.
We consider a framework for taking into consideration the relative importance (ordinality) of object labels in the process of learning a label predictor function. The commonly used loss functions are not well matched to this problem, as they exhibit deficiencies in capturing natural correlations of the labels and the corresponding data. We propose to incorporate such correlations into our learning algorithm using an optimal transport formulation. Our approach is to learn the ground metric, which is partly involved in forming the optimal transport distance, by leveraging ordinality as a general form of side information in its formulation. Based on this idea, we then develop a novel loss function for training deep neural networks. A highly efficient alternating learning method is then devised to alternatively optimise the ground metric and the deep model in an end-to-end learning manner. This scheme allows us to adaptively adjust the shape of the ground metric, and consequently the shape of the loss function for each application. We back up our approach by theoretical analysis and verify the performance of our proposed scheme by applying it to two learning tasks, i.e. chronological age estimation from the face and image aesthetic assessment. The numerical results on several benchmark datasets demonstrate the superiority of the proposed algorithm.
Recently, masked image modeling (MIM), an important self-supervised learning (SSL) method, has drawn attention for its effectiveness in learning data representation from unlabeled data. Numerous studies underscore the advantages of MIM, highlighting how models pretrained on extensive datasets can enhance the performance of downstream tasks. However, the high computational demands of pretraining pose significant challenges, particularly within academic environments, thereby impeding the SSL research progress. In this study, we propose efficient training recipes for MIM based SSL that focuses on mitigating data loading bottlenecks and employing progressive training techniques and other tricks to closely maintain pretraining performance. Our library enables the training of a MAE-Base/16 model on the ImageNet 1K dataset for 800 epochs within just 18 hours, using a single machine equipped with 8 A100 GPUs. By achieving speed gains of up to 5.8 times, this work not only demonstrates the feasibility of conducting high-efficiency SSL training but also paves the way for broader accessibility and promotes advancement in SSL research particularly for prototyping and initial testing of SSL ideas.
To counteract spoofing attacks, the majority of recent approaches to face spoofing attack detection formulate the problem as a binary classification task in which real data and attack-accesses are both used to train spoofing detectors. Although the classical training framework has been demonstrated to deliver satisfactory results, its robustness to unseen attacks is debatable. Inspired by the recent success of anomaly detection models in face spoofing detection, we propose an ensemble of one-class classifiers fused by a Stacking ensemble method to reduce the generalisation error in the more realistic unseen attack scenario. To be consistent with this scenario, anomalous samples are considered neither for training the component anomaly classifiers nor for the design of the Stacking ensemble. To achieve better face-anti spoofing results, we adopt client-specific information to build both constituent classifiers as well as the Stacking combiner. Besides, we propose a novel 2-stage Genetic Algorithm to further improve the generalisation performance of Stacking ensemble. We evaluate the effectiveness of the proposed systems on publicly available face anti-spoofing databases including Replay-Attack, Replay-Mobile and Rose-Youtu. The experimental results following the unseen attack evaluation protocol confirm the merits of the proposed model.
Deep neural networks have enhanced the performance of decision making systems in many applications, including image understanding, and further gains can be achieved by constructing ensembles. However, designing an ensemble of deep networks is often not very beneficial since the time needed to train the networks is generally very high or the performance gain obtained is not very significant. In this paper, we analyse an error correcting output coding (ECOC) framework for constructing ensembles of deep networks and propose different design strategies to address the accuracy-complexity trade-off. We carry out an extensive comparative study between the introduced ECOC designs and the state-of-the-art ensemble techniques such as ensemble averaging and gradient boosting decision trees. Furthermore, we propose a fusion technique, that is shown to achieve the highest classification performance.
The fusion of one-class classifiers (OCCs) has been shown to exhibit promising performance in a variety of machine learning applications. The ability to assess the similarity or correlation between the output of various OCCs is an important prerequisite for building of a meaningful OCCs ensemble. However, this aspect of the OCC fusion problem has been mostly ignored so far. In this paper, we propose a new method of constructing a fusion of OCCs with three contributions: (a) As a key contribution, enabling an OCC ensemble design using exclusively non anomalous samples, we propose a novel fitness function to evaluate the competency of OCCs without requiring samples from the anomalous class; (b) As a minor, but impactful contribution, we investigate alternative forms of score normalisation of OCCs, and identify a novel two-sided normalisation method as the best in coping with long tail non anomalous data distributions; (c) In the context of building our proposed OCC fusion system based on the weighted averaging approach, we find that the weights optimised using a particle swarm optimisation algorithm produce the most effective solution. We evaluate the merits of the proposed method on 15 benchmarking datasets from different application domains including medical, anti-spam and face spoofing detection. The comparison of the proposed approach with state-of-the-art methods alongside the statistical analysis confirm the effectiveness of the proposed model. (c) 2021 Elsevier Ltd. All rights reserved.
Face recognition (FR) using deep convolutional neural networks (DCNNs) has seen remarkable success in recent years. One key ingredient of DCNN-based FR is the design of a loss function that ensures discrimination between various identities. The state-of-the-art (SOTA) solutions utilise normalised Softmax loss with additive and/or multiplicative margins. Despite being popular and effective, these losses are justified only intuitively with little theoretical explanations. In this work, we show that under the LogSumExp (LSE) approximation, the SOTA Softmax losses become equivalent to a proxy-triplet loss that focuses on nearest-neighbour negative proxies only. This motivates us to propose a variant of the proxy-triplet loss, entitled Nearest Proxies Triplet (NPT) loss, which unlike SOTA solutions, converges for a wider range of hyper-parameters and offers flexibility in proxy selection and thus outperforms SOTA techniques. We generalise many SOTA losses into a single framework and give theoretical justifications for the assertion that minimising the proposed loss ensures a minimum separability between all identities. We also show that the proposed loss has an implicit mechanism of hard-sample mining. We conduct extensive experiments using various DCNN architectures on a number of FR benchmarks to demonstrate the efficacy of the proposed scheme over SOTA methods.