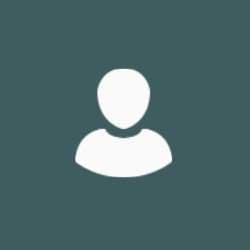
Severin Skillman
Academic and research departments
Centre for Vision, Speech and Signal Processing (CVSSP), Faculty of Engineering and Physical Sciences.Publications
In this paper we discuss a technical design and an ongoing trial that is being conducted in the UK, called Technology Integrated Health Management (TIHM). TIHM uses Internet of Things (IoT) enabled solutions provided by various companies in a collaborative project. The IoT devices and solutions are integrated in a common platform that supports interoperable and open standards. A set of machine learning and data analytics algorithms generate notifications regarding the well-being of the patients. The information is monitored around the clock by a group of healthcare practitioners who take appropriate decisions according to the collected data and generated notifications. In this paper we discuss the design principles and the lessons that we have learned by co-designing this system with patients, their carers, clinicians, and also our industry partners. We discuss the technical design of TIHM and explain why user-centred and human-experience should be an integral part of the technological design.
We describe a digital platform developed in collaboration with clinicians and user groups to provide remote healthcare monitoring and support in a dementia care application. The platform uses data from sensory devices that are deployed in participants' homes and utilises a set machine learning and analytical algorithms to identify risks of adverse health conditions such as Urinary Tract Infections (UTIs) and hypertension in people with dementia. The platform includes a clinical interface that is used by a monitoring team to view alerts and notifications that are generated by the algorithms and to also browse the in-home activity and physiological data in a secure and privacy-aware system. The platform complies to the information governance requirements of the UK National Healthcare Service (NHS) and is registered as a class 1 medical device. The platform has been deployed and tested in over 150 homes.
In this paper, we present a Mobile Edge Computing (MEC) scheme for enabling network edge-assisted video adaptation based on MPEG-DASH (Dynamic Adaptive Streaming over HTTP). In contrast to the traditional over-the-top (OTT) adaptation performed by DASH clients, the MEC server at the mobile network edge can capture radio access network (RAN) conditions through its intrinsic Radio Network Information Service (RNIS) function, and use the knowledge to provide guidance to clients so that they can perform more intelligent video adaptation. In order to support such MECassisted DASH video adaptation, the MEC server needs to locally cache the most popular content segments at the qualities that can be supported by the current network throughput. Towards this end, we introduce a two-dimensional user Quality-of-Experience (QoE)-driven algorithm for making caching / replacement decisions based on both content context (e.g., segment popularity) and network context (e.g., RAN downlink throughput). We conducted experiments by deploying a prototype MEC server at a real LTE-A based network testbed. The results show that our QoE-driven algorithm is able to achieve significant improvement on user QoE over 2 benchmark schemes
Dementia is a neurological and cognitive condition that affects millions of people around the world. At any given time in the United Kingdom, 1 in 4 hospital beds are occupied by a person with dementia, while about 22% of these hospital admissions are due to preventable causes. In this paper we discuss using Internet of Things (IoT) technologies and in-home sensory devices in combination with machine learning techniques to monitor health and well-being of people with dementia. This will allow us to provide more effective and preventative care and reduce preventable hospital admissions. One of the unique aspects of this work is combining environmental data with physiological data collected via low cost in-home sensory devices to extract actionable information regarding the health and well-being of people with dementia in their own home environment. We have worked with clinicians to design our machine learning algorithms where we focused on developing solutions for real-world settings. In our solutions, we avoid generating too many alerts/alarms to prevent increasing the monitoring and support workload. We have designed an algorithm to detect Urinary Tract Infections (UTI) which is one of the top five reasons of hospital admissions for people with dementia (around 9% of hospital admissions for people with dementia in the UK). To develop the UTI detection algorithm, we have used a Non-negative Matrix Factorisation (NMF) technique to extract latent factors from raw observation and use them for clustering and identifying the possible UTI cases. In addition, we have designed an algorithm for detecting changes in activity patterns to identify early symptoms of cognitive decline or health decline in order to provide personalised and preventative care services. For this purpose, we have used an Isolation Forest (iForest) technique to create a holistic view of the daily activity patterns. This paper describes the algorithms and discusses the evaluation of the work using a large set of real-world data collected from a trial with people with dementia and their caregivers.