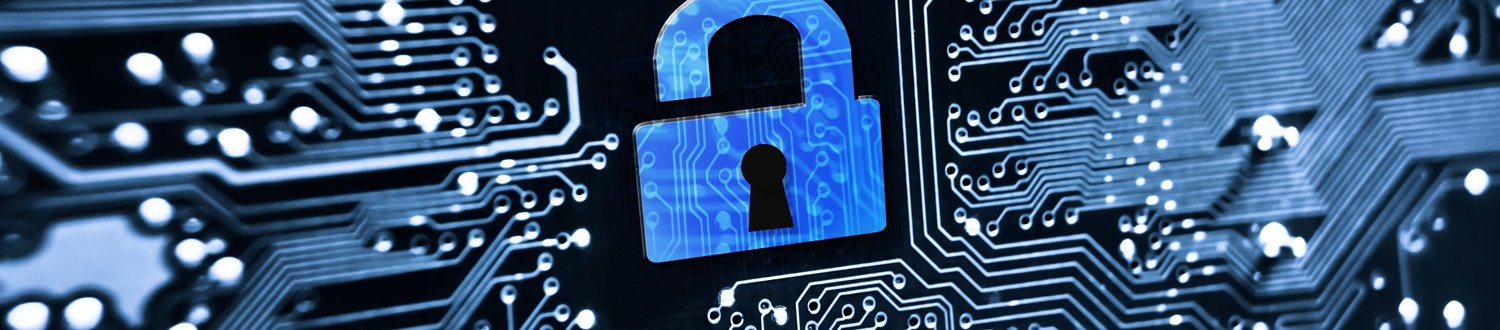
People
We are a friendly group of academic staff, research staff, tutors and administrators, with additional dedicated staff within the Faculty and the University to support us.
We are also proud to have strong links with people in industry who provide support for our activities through our Industrial Advisory Board or through guest lectures in our undergraduate or postgraduate modules.
Our staff and students
Find out more about our staff and students and get in contact.