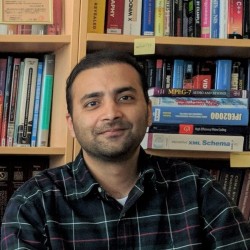
Dr Syed Sameed Husain
About
Biography
I am currently a Lecturer in PCAI at the Centre for Vision, Speech and Signal Processing (CVSSP), University of Surrey. I have a strong track record of high-impact fundamental advances in AI and its applications to real-world problems in healthcare, entertainment, and security, as evident from my google scholar (Nature, TPAMI, TNNLS, TIP, TCSVT, IJCV) and global technology awards (Gold Medals in Google Landmark Retrieval 2018 and 2019, Gold Medal in Google YouTube Video Understanding 2018 and top 2% position from 800 teams in HPA Single Cell Classification 2021). Since joining CVSSP in 2016 as a Research fellow, I have proposed novel commercial ideas and made significant contributions to research projects (InnovateUK RetinaScan, iTravel, CODAM, H2020 BRIDGET and MURI), generating high-impact research and technologies. I have led core AI R&D efforts and successfully delivered commercial People-Centric AI (PAI) systems to National Health Service, BBC and MET police.
Areas of specialism
My qualifications
ResearchResearch interests
I am an experienced researcher with a proven track record of world-class research in AI and generating a strong impact via successful projects with the industry. My research focuses on novel techniques in AI and their applications in healthcare, entertainment, and security. I have a particular interest in image and video recognition. Since joining CVSSP in 2016 as a Research fellow, I have made considerable contributions to research projects (InnovateUK RetinaScan, iTravel, CODAM, and H2020 BRIDGET), which, apart from generating novel research, have also involved taking the lead role in communication between partners and funding bodies at both national and international level. Several global awards have recognised my scientific contributions: Gold Medals in Google Landmark Retrieval Challenges 2018 and 2019, Gold Medal in Google YouTube Video Understanding Challenge 2018, and a top 2% position from 800 teams in Human Protein Atlas - Single Cell Classification Challenge 2021. I have also made important contributions to the ISO MPEG standard with technical submissions to ISO and BSI. In recognition of my outstanding scientific achievements and their strong international impact, I was awarded the Innovator of the year award in 2018 by CVSSP.
During my previous roles as a research fellow, I worked on European Commission-funded (FP7) project, Bridging the Gap for Enhanced broadcast (BRIDGET). I was responsible for developing large-scale visual search algorithms for the broadcast industry (Huawei, RAI, Fraunhofer, and Telecom Italia). My research led to the development of a novel AI system for deriving a compact and distinctive representation of image content called Robust Visual Descriptor (RVD). The University of Surrey filed a US patent based on RVD representation for visual search. My work was also published in IEEE Transactions on Pattern Analysis and Machine Intelligence. During the second year of research, I worked on Innovate UK project, Content-based Digital Asset Management (CODAM), to develop an advanced video classification system. Our team participated in the Google YouTube 8M Video Understanding Challenge 2018, where the task was to develop an AI system to accurately assign labels to videos. Our deep learning-based method won the Gold Medal from the 650 entries worldwide. The AI system we developed is being used by the BBC and the Metropolitan Police. The work on video classification was published in IEEE Transactions on Circuits and Systems for Video Technology. In the third year, I was part of the InnovateUK project iTravel, where the aim was to develop a smartphone-based intelligent "virtual journey assistant", providing end-to-end routing with proactive contextual information to the traveller. During the project, our team took part in the Google Landmark Retrieval Challenge 2018. The challenge was to develop the world's most accurate technology to identify landmarks and retrieve relevant photographs from a database automatically. Our deep learning-based system, "REMAP", won the prestigious competition, outperforming well-known international corporations and university groups, including Google, Facebook, Layer6 AI Canada, Naver Labs Europe, Stanford, and MIT USA. Our winning solution was published in IEEE Transactions on Image Processing. Moreover, my recent image recognition system "ACTNET", published in the International Journal of Computer Vision, won Gold Medal in Google Landmark Retrieval Challenge 2019.
From the year 2020 to 2022, I worked on the InnovateUK project "RetinaScan: AI-enabled automated image assessment system for diabetic retinopathy (DR) screening" in collaboration with National Health Service (NHS) and Seon diagnostics Ltd. Our deep learning system 'HydraNet' achieved high sensitivity and specificity for identifying DR using retinal images from multi-ethnic populations, significantly outperforming the current best, FDA USA and European Union approved, EyeArt system.
Recently, my team participated in the Human Protein Cell Classification challenge 2021, organised by the Human Protein Atlas team in Sweden and Kaggle. Here I developed a novel system, HCPL, to localise proteins at a subcellular level. Our system processes high-resolution confocal microscopy images to segment individual cells, assesses their visual integrity, and robustly predicts protein localisation patterns. HCPL makes large-scale single-cell data annotation feasible by helping to avoid the need to hand-label individual cells. HCPL currently defines state-of-the-art in the single-cell classification of protein localisation patterns and is published in Nature Communications Biology.
Currently, I am collaborating with Metropolitan Police on the Police STAR Knife Recognition AI project. The aim is to create the world's first Police AI-bladed weapon analysis system to effortlessly allow officers to identify the make, model and sellers of knives encountered by the police.
Research interests
I am an experienced researcher with a proven track record of world-class research in AI and generating a strong impact via successful projects with the industry. My research focuses on novel techniques in AI and their applications in healthcare, entertainment, and security. I have a particular interest in image and video recognition. Since joining CVSSP in 2016 as a Research fellow, I have made considerable contributions to research projects (InnovateUK RetinaScan, iTravel, CODAM, and H2020 BRIDGET), which, apart from generating novel research, have also involved taking the lead role in communication between partners and funding bodies at both national and international level. Several global awards have recognised my scientific contributions: Gold Medals in Google Landmark Retrieval Challenges 2018 and 2019, Gold Medal in Google YouTube Video Understanding Challenge 2018, and a top 2% position from 800 teams in Human Protein Atlas - Single Cell Classification Challenge 2021. I have also made important contributions to the ISO MPEG standard with technical submissions to ISO and BSI. In recognition of my outstanding scientific achievements and their strong international impact, I was awarded the Innovator of the year award in 2018 by CVSSP.
During my previous roles as a research fellow, I worked on European Commission-funded (FP7) project, Bridging the Gap for Enhanced broadcast (BRIDGET). I was responsible for developing large-scale visual search algorithms for the broadcast industry (Huawei, RAI, Fraunhofer, and Telecom Italia). My research led to the development of a novel AI system for deriving a compact and distinctive representation of image content called Robust Visual Descriptor (RVD). The University of Surrey filed a US patent based on RVD representation for visual search. My work was also published in IEEE Transactions on Pattern Analysis and Machine Intelligence. During the second year of research, I worked on Innovate UK project, Content-based Digital Asset Management (CODAM), to develop an advanced video classification system. Our team participated in the Google YouTube 8M Video Understanding Challenge 2018, where the task was to develop an AI system to accurately assign labels to videos. Our deep learning-based method won the Gold Medal from the 650 entries worldwide. The AI system we developed is being used by the BBC and the Metropolitan Police. The work on video classification was published in IEEE Transactions on Circuits and Systems for Video Technology. In the third year, I was part of the InnovateUK project iTravel, where the aim was to develop a smartphone-based intelligent "virtual journey assistant", providing end-to-end routing with proactive contextual information to the traveller. During the project, our team took part in the Google Landmark Retrieval Challenge 2018. The challenge was to develop the world's most accurate technology to identify landmarks and retrieve relevant photographs from a database automatically. Our deep learning-based system, "REMAP", won the prestigious competition, outperforming well-known international corporations and university groups, including Google, Facebook, Layer6 AI Canada, Naver Labs Europe, Stanford, and MIT USA. Our winning solution was published in IEEE Transactions on Image Processing. Moreover, my recent image recognition system "ACTNET", published in the International Journal of Computer Vision, won Gold Medal in Google Landmark Retrieval Challenge 2019.
From the year 2020 to 2022, I worked on the InnovateUK project "RetinaScan: AI-enabled automated image assessment system for diabetic retinopathy (DR) screening" in collaboration with National Health Service (NHS) and Seon diagnostics Ltd. Our deep learning system 'HydraNet' achieved high sensitivity and specificity for identifying DR using retinal images from multi-ethnic populations, significantly outperforming the current best, FDA USA and European Union approved, EyeArt system.
Recently, my team participated in the Human Protein Cell Classification challenge 2021, organised by the Human Protein Atlas team in Sweden and Kaggle. Here I developed a novel system, HCPL, to localise proteins at a subcellular level. Our system processes high-resolution confocal microscopy images to segment individual cells, assesses their visual integrity, and robustly predicts protein localisation patterns. HCPL makes large-scale single-cell data annotation feasible by helping to avoid the need to hand-label individual cells. HCPL currently defines state-of-the-art in the single-cell classification of protein localisation patterns and is published in Nature Communications Biology.
Currently, I am collaborating with Metropolitan Police on the Police STAR Knife Recognition AI project. The aim is to create the world's first Police AI-bladed weapon analysis system to effortlessly allow officers to identify the make, model and sellers of knives encountered by the police.
Teaching
Fundamentals of Machine Learning (EEEM066)