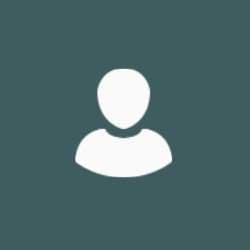
Dr Yin Cao
Academic and research departments
Centre for Vision, Speech and Signal Processing (CVSSP), Faculty of Engineering and Physical Sciences.Publications
Polyphonic sound event localization and detection (SELD), which jointly performs sound event detection (SED) and direction-of-arrival (DoA) estimation, detects the type and occurrence time of sound events as well as their corresponding DoA angles simultaneously. We study the SELD task from a multi-task learning perspective. Two open problems are addressed in this paper. Firstly, to detect overlapping sound events of the same type but with different DoAs, we propose to use a trackwise output format and solve the accompanying track permutation problem with permutation-invariant training. Multi-head self-attention is further used to separate tracks. Secondly, a previous finding is that, by using hard parameter-sharing, SELD suffers from a performance loss compared with learning the subtasks separately. This is solved by a soft parameter-sharing scheme. We term the proposed method as Event Independent Network V2 (EINV2), which is an improved version of our previously-proposed method and an end-to-end network for SELD. We show that our proposed EINV2 for joint SED and DoA estimation outperforms previous methods by a large margin, and has comparable performance to state-of-the-art ensemble models. Index Terms— Sound event localization and detection, direction of arrival, event-independent, permutation-invariant training, multi-task learning.
Polyphonic sound event localization and detection is not only detecting what sound events are happening but localizing corresponding sound sources. This series of tasks was first introduced in DCASE 2019 Task 3. In 2020, the sound event localization and detection task introduces additional challenges in moving sound sources and overlapping-event cases, which include two events of the same type with two different direction-of-arrival (DoA) angles. In this paper, a novel event-independent network for polyphonic sound event lo-calization and detection is proposed. Unlike the two-stage method we proposed in DCASE 2019 Task 3, this new network is fully end-to-end. Inputs to the network are first-order Ambisonics (FOA) time-domain signals, which are then fed into a 1-D convolutional layer to extract acoustic features. The network is then split into two parallel branches. The first branch is for sound event detection (SED), and the second branch is for DoA estimation. There are three types of predictions from the network, SED predictions, DoA predictions , and event activity detection (EAD) predictions that are used to combine the SED and DoA features for onset and offset estimation. All of these predictions have the format of two tracks indicating that there are at most two overlapping events. Within each track, there could be at most one event happening. This architecture introduces a problem of track permutation. To address this problem, a frame-level permutation invariant training method is used. Experimental results show that the proposed method can detect polyphonic sound events and their corresponding DoAs. Its performance on the Task 3 dataset is greatly increased as compared with that of the baseline method. Index Terms— Sound event localization and detection, direction of arrival, event-independent, permutation invariant training.
Large Language Models (LLMs) have shown great promise in integrating diverse expert models to tackle intricate language and vision tasks. Despite their significance in advancing the field of Artificial Intelligence Generated Content (AIGC), their potential in intelligent audio content creation remains unexplored. In this work, we tackle the problem of creating audio content with storylines encompassing speech, music, and sound effects, guided by text instructions. We present WavJourney, a system that leverages LLMs to connect various audio models for audio content generation. Given a text description of an auditory scene, WavJourney first prompts LLMs to generate a structured script dedicated to audio storytelling. The audio script incorporates diverse audio elements, organized based on their spatio-temporal relationships. As a conceptual representation of audio, the audio script provides an interactive and interpretable rationale for human engagement. Afterward, the audio script is fed into a script compiler, converting it into a computer program. Each line of the program calls a task-specific audio generation model or computational operation function (e.g., concatenate, mix). The computer program is then executed to obtain an explainable solution for audio generation. We demonstrate the practicality of WavJourney across diverse real-world scenarios, including science fiction, education, and radio play. The explainable and interactive design of WavJourney fosters human-machine co-creation in multi-round dialogues, enhancing creative control and adaptability in audio production. WavJourney audiolizes the human imagination, opening up new avenues for creativity in multimedia content creation.
For learning-based sound event localization and detection (SELD) methods, different acoustic environments in the training and test sets may result in large performance differences in the validation and evaluation stages. Different environments, such as different sizes of rooms, different reverberation times, and different background noise, may be reasons for a learning-based system to fail. On the other hand, acquiring annotated spatial sound event samples, which include onset and offset time stamps, class types of sound events, and direction-of-arrival (DOA) of sound sources is very expensive. In addition, deploying a SELD system in a new environment often poses challenges due to time-consuming training and fine-tuning processes. To address these issues, we propose Meta-SELD, which applies meta-learning methods to achieve fast adaptation to new environments. More specifically, based on Model Agnostic Meta-Learning (MAML), the proposed Meta-SELD aims to find good meta-initialized parameters to adapt to new environments with only a small number of samples and parameter updating iterations. We can then quickly adapt the meta-trained SELD model to unseen environments. Our experiments compare fine-tuning methods from pre-trained SELD models with our Meta-SELD on the Sony-TAU Realistic Spatial Soundscapes 2023 (STARSSS23) dataset. The evaluation results demonstrate the effectiveness of Meta-SELD when adapting to new environments.
Humans are able to identify a large number of environmental sounds and categorise them according to high-level semantic categories, e.g. urban sounds or music. They are also capable of generalising from past experience to new sounds when applying these categories. In this paper we report on the creation of a data set that is structured according to the top-level of a taxonomy derived from human judgements and the design of an associated machine learning challenge, in which strong generalisation abilities are required to be successful. We introduce a baseline classification system, a deep convolutional network, which showed strong performance with an average accuracy on the evaluation data of 80.8%. The result is discussed in the light of two alternative explanations: An unlikely accidental category bias in the sound recordings or a more plausible true acoustic grounding of the high-level categories.
Sound event localization and detection (SELD) is a joint task of sound event detection and direction-of-arrival estimation. In DCASE 2022 Task 3, types of data transform from computationally generated spatial recordings to recordings of real-sound scenes. Our system submitted to the DCASE 2022 Task 3 is based on our previous proposed Event-Independent Network V2 (EINV2) with a novel data augmentation method. Our method employs EINV2 with a track-wise output format, permutation-invariant training, and a soft parameter-sharing strategy, to detect different sound events of the same class but in different locations. The Conformer structure is used for extending EINV2 to learn local and global features. A data augmentation method, which contains several data augmentation chains composed of stochastic combinations of several different data augmentation operations, is utilized to generalize the model. To mitigate the lack of real-scene recordings in the development dataset and the presence of sound events being unbalanced, we exploit FSD50K, AudioSet, and TAU Spatial Room Impulse Response Database (TAU-SRIR DB) to generate simulated datasets for training. We present results on the validation set of Sony-TAu Realistic Spatial Soundscapes 2022 (STARSS22) in detail. Experimental results indicate that the ability to generalize to different environments and unbalanced performance among different classes are two main challenges. We evaluate our proposed method in Task 3 of the DCASE 2022 challenge and obtain the second rank in the teams ranking.
Previous geographic routing schemes in Delay/Disruption Tolerant Networks (DTNs) only consider the homogeneous scenario where nodal mobility is identical. Motivated by this gap, we turn to design a DTN based geographic routing scheme in heterogeneous scenario. Systematically, our target is achieved via two steps: 1) We first propose “The-BestGeographic-Relay (TBGR)” routing scheme to relay messages via a limited number of copies, under the homogeneous scenario. We further overcome the local maximum problem of TBGR given a sparse network density, different from those efforts in dense networks like clustered Wireless Sensor Networks (WSNs). 2) We next extend TBGR for heterogeneous scenario, and propose “TheBest-Heterogeneity-Geographic-Relay (TBHGR)” routing scheme considering individual nodal visiting preference (referred to nonidentical nodal mobility). Extensive results under a realistic heterogeneous scenario show the advantage of TBHGR over literature works in terms of reliable message delivery, while with low routing overhead.
This paper addresses delay/disruption tolerant networking routing under a highly dynamic scenario, envisioned for communication in vehicular sensor networks (VSNs) suffering from intermittent connection. Here, we focus on the design of a high-level routing framework, rather than the dedicated encounter prediction. Based on an analyzed utility metric to predict nodal encounter, our proposed routing framework considers the following three cases. First, messages are efficiently replicated to a better qualified candidate node, based on the analyzed utility metric related to destination. Second, messages are conditionally replicated if the node with a better utility metric has not been met. Third, messages are probabilistically replicated if the information in relation to destination is unavailable in the worst case. With this framework in mind, we propose two routing schemes covering two major technique branches in literature, namely: 1) encounter-based replication routing and 2) encounter-based spraying routing. Results under the scenario applicable to VSNs show that, in addition to achieving high delivery ratio for reliability, our schemes are more efficient in terms of a lower overhead ratio. Our core investigation indicates that apart from what information to use for encounter prediction, how to deliver messages based on the given utility metric is also important.
Although geographic routing is an alternative approach to topology routing in delay/disruption tolerant networks (DTNs), sparse network density and high mobility result in challenges to obtain the real time geographic information of destination if taking its mobility into account. Furthermore, sparse network density is also in contrast with high-network density, for handling the local maximum problem that the message carrier cannot find a better candidate node to relay a message. In this article, the authors investigate geographic routing in DTNs from another perspective, assuming the real time geographic information of mobile destination is always unavailable. The key insight is to estimate the movement range of the destination using its historical geographic information, to promote message replication reaching the edge of this range using a Reach Phase and spreading within this range using a Spread Phase. Then, these two phases are combined to promote message delivery within the limited message lifetime. The evaluation of results under the Helsinki city scenario show the advantage of our proposed Reach-and-Spread in terms of delivery ratio and average delivery latency as well as overhead ratio.
The design of an efficient charging management system for on-the-move Electric Vehicles (EVs) has become an emerging research problem, in future connected vehicle applications given their mobility uncertainties. Major technical challenges here involve decision-making intelligence for the selection of Charging Stations (CSs), as well as the corresponding communication infrastructure for necessary information dissemination between the power grid and mobile EVs. In this article, we propose a holistic solution that aims to create high impact on the improvement of end users’ driving experiences (e.g., to minimize EVs’ charging waiting time during their journeys) and charging efficiency at the power grid side. Particularly, the CS-selection decision on where to charge is made by individual EVs for privacy and scalability benefits. The communication framework is based on a mobile Publish/Subscribe (P/S) paradigm to efficiently disseminate CSs condition information to EVs on-the-move. In order to circumvent the rigidity of having stationary Road Side Units (RSUs) for information dissemination, we promote the concept of Mobility as a Service (MaaS) by exploiting the mobility of public transportation vehicles (e.g. buses) to bridge the information flow to EVs, given their opportunistic encounters. We analyze various factors affecting the possibility for EVs to access CSs information via opportunistic Vehicle-to-Vehicle (V2V) communications, and also demonstrate the advantage of introducing buses as mobile intermediaries for information dissemination, based on a common EV charging management system under the Helsinki city scenario. We further study the feasibility and benefit of enabling EVs to send their charging reservations involved for CS-selection logic, via opportunistically encountered buses as well. Results show this advanced management system improves both performances at CS and EV sides.
The research in this letter focuses on geographic routing in Delay/Disruption Tolerant Networks (DTNs), by considering sparse network density. We explore the Delegation Forwarding (DF) approach to overcome the limitation of the geometric metric which requires mobile node moving towards destination, with the Delegation Geographic Routing (DGR) proposed. Besides, we handle the local maximum problem of DGR, by considering nodal mobility and message lifetime. Analysis and evaluation results show that DGR overcomes the limitation of the algorithm based on the given geometric metric. By overcoming the limited routing decision and handling the local maximum problem, DGR is reliable for delivering messages before expiration lifetime. Meanwhile, the efficiency of DGR regarding low overhead ratio is contributed by utilizing DF. © 2013 IEEE.
Data collection is a fundamental yet challenging task of Wireless Sensor Networks (WSN) to support a variety of applications, due to the inherent distinguish characteristics for sensor networks, such as limited energy supply, self-organizing deployment and QoS requirements for different applications. Mobile sink and virtual MIMO (vMIMO) techniques can be jointly considered to achieve both time efficient and energy efficient for data collection. In this paper, we aim to minimize the overall data collection latency including both sink moving time and sensor data uploading time. We formulate the problem and propose a multihop weighted revenue (MWR) algorithm to approximate the optimal solution. To achieve the trade-off between full utilization of concurrent uploading of vMIMO and the shortest moving tour of mobile sink, the proposed algorithm combines the amount of concurrent uploaded data, the number of neighbours, and the moving tour length of sink in one metric for polling point selection. The simulation results show that the proposed MWR effectively reduces total data collection latency in different network scenarios with less overall network energy consumption.
Humans are able to identify a large number of environmental sounds and categorise them according to high-level semantic categories, e.g. urban sounds or music. They are also capable of generalising from past experience to new sounds when applying these categories. In this paper we report on the creation of a data set that is structured according to the top-level of a taxonomy derived from human judgements and the design of an associated machine learning challenge, in which strong generalisation abilities are required to be successful. We introduce a baseline classification system, a deep convolutional network, which showed strong performance with an average accuracy on the evaluation data of 80.8%. The result is discussed in the light of two alternative explanations: An unlikely accidental category bias in the sound recordings or a more plausible true acoustic grounding of the high-level categories.
Acoustic scene generation (ASG) is a task to generate waveforms for acoustic scenes. ASG can be used to generate audio scenes for movies and computer games. Recently, neural networks such as SampleRNN have been used for speech and music generation. However, ASG is more challenging due to its wide variety. In addition, evaluating a generative model is also difficult. In this paper, we propose to use a conditional SampleRNN model to generate acoustic scenes conditioned on the input classes. We also propose objective criteria to evaluate the quality and diversity of the generated samples based on classification accuracy. The experiments on the DCASE 2016 Task 1 acoustic scene data show that with the generated audio samples, a classification accuracy of 65:5% can be achieved compared to samples generated by a random model of 6:7% and samples from real recording of 83:1%. The performance of a classifier trained only on generated samples achieves an accuracy of 51:3%, as opposed to an accuracy of 6:7% with samples generated by a random model.
The availability of audio data on sound sharing platforms such as Freesound gives users access to large amounts of annotated audio. Utilising such data for training is becoming increasingly popular, but the problem of label noise that is often prevalent in such datasets requires further investigation. This paper introduces ARCA23K, an Automatically Retrieved and Curated Audio dataset comprised of over 23 000 labelled Freesound clips. Unlike past datasets such as FSDKaggle2018 and FSDnoisy18K, ARCA23K facilitates the study of label noise in a more controlled manner. We describe the entire process of creating the dataset such that it is fully reproducible, meaning researchers can extend our work with little effort. We show that the majority of labelling errors in ARCA23K are due to out-of-vocabulary audio clips, and we refer to this type of label noise as open-set label noise. Experiments are carried out in which we study the impact of label noise in terms of classification performance and representation learning.
Source separation is the task of separating an audio recording into individual sound sources. Source separation is fundamental for computational auditory scene analysis. Previous work on source separation has focused on separating particular sound classes such as speech and music. Much previous work requires mixtures and clean source pairs for training. In this work, we propose a source separation framework trained with weakly labelled data. Weakly labelled data only contains the tags of an audio clip, without the occurrence time of sound events. We first train a sound event detection system with AudioSet. The trained sound event detection system is used to detect segments that are most likely to contain a target sound event. Then a regression is learnt from a mixture of two randomly selected segments to a target segment conditioned on the audio tagging prediction of the target segment. Our proposed system can separate 527 kinds of sound classes from AudioSet within a single system. A U-Net is adopted for the separation system and achieves an average SDR of 5.67 dB over 527 sound classes in AudioSet.
In supervised machine learning, the assumption that training data is labelled correctly is not always satisfied. In this paper, we investigate an instance of labelling error for classification tasks in which the dataset is corrupted with out-of-distribution (OOD) instances: data that does not belong to any of the target classes, but is labelled as such. We show that detecting and relabelling certain OOD instances, rather than discarding them, can have a positive effect on learning. The proposed method uses an auxiliary classifier, trained on data that is known to be in-distribution, for detection and relabelling. The amount of data required for this is shown to be small. Experiments are carried out on the FSDnoisy18k audio dataset, where OOD instances are very prevalent. The proposed method is shown to improve the performance of convolutional neural networks by a significant margin. Comparisons with other noise-robust techniques are similarly encouraging.
—Audio pattern recognition is an important research topic in the machine learning area, and includes several tasks such as audio tagging, acoustic scene classification, music classification , speech emotion classification and sound event detection. Recently, neural networks have been applied to tackle audio pattern recognition problems. However, previous systems are built on specific datasets with limited durations. Recently, in computer vision and natural language processing, systems pretrained on large-scale datasets have generalized well to several tasks. However, there is limited research on pretraining systems on large-scale datasets for audio pattern recognition. In this paper, we propose pretrained audio neural networks (PANNs) trained on the large-scale AudioSet dataset. These PANNs are transferred to other audio related tasks. We investigate the performance and computational complexity of PANNs modeled by a variety of convolutional neural networks. We propose an architecture called Wavegram-Logmel-CNN using both log-mel spectrogram and waveform as input feature. Our best PANN system achieves a state-of-the-art mean average precision (mAP) of 0.439 on AudioSet tagging, outperforming the best previous system of 0.392. We transfer PANNs to six audio pattern recognition tasks, and demonstrate state-of-the-art performance in several of those tasks. We have released the source code and pretrained models of PANNs: https://github.com/qiuqiangkong/audioset_tagging_cnn.
Polyphonic sound event localization and detection (SELD) aims at detecting types of sound events with corresponding temporal activities and spatial locations. In this paper, a track-wise ensemble event independent network with a novel data augmentation method is proposed. The proposed model is based on our previous proposed Event-Independent Network V2 and is extended by conformer blocks and dense blocks. The track-wise ensemble model with track-wise output format is proposed to solve an ensemble model problem for track-wise output format that track permutation may occur among different models. The data augmentation approach contains several data augmentation chains, which are composed of random combinations of several data augmentation operations. The method also utilizes log-mel spectrograms, intensity vectors, and Spatial Cues-Augmented Log-Spectrogram (SALSA) for different models. We evaluate our proposed method in the Task of the L3DAS22 challenge and obtain the top ranking solution with a location-dependent F-score to be 0.699. Source code is released 1 .
Vehicular Delay Tolerant Networking (VDTN) is a special instance of Vehicular Ad hoc Networking (VANET) and in particular Delay Tolerant Networking (DTN) that utilizes infrastructure to enhance connectivity in challenged environments. While VANETs assume end-to-end connectivity, DTNs and VDTNs do not. Such networks are characterized by dynamic topology, partitioning due to lack of end-to-end connectivity, and opportunistic encounters between nodes. Notably, VDTNs enhances the capabilities DTNs to provide support for delay and intermittent connectivity. Hence, they can easily find applicability in the early stages of the deployment of vehicular networks characterized by low infrastructure deployment as is obtainable in rural areas and in military communications. Privacy implementation and evaluation is a major challenge in VDTNs. Group communication has become one of the well discussed means for achieving effective privacy and packet routing in ad hoc networks including VDTNs. However, most existing privacy schemes lack flexibility in terms of the dynamics of group formation and the level of privacy achievable. Again, it is difficult to evaluate privacy for sparse VDTNs for rural area and early stages of deployment. This paper reports on an improved privacy scheme based on group communication scheme in VDTNs. We analyze the performance of our model in terms of trade-off between privacy and performance based on delivery overhead and message delivery ratio using simulations. While this is a work in progress, we report that our scheme has considerable improvement compared to other similar schemes described in literature.
The concept of Delay Tolerant Networks (DTNs) has been utilized for wireless sensor networks, mobile ad hoc networks, interplanetary networks, pocket switched networks and suburb networks for developing region. Because of these application prospects, DTNs have received attention from academic community. Whereas only a few state of the art routing algorithms in DTNs address the problem of aborted messages due to the insufficient encounter duration. In order to reduce these aborted messages, we propose a routing framework which consists of two optional routing functions. Specifically, only one of them is activated according to the encounter angle between pairwise nodes. Besides, the copies of the undelivered message carried by most of the nodes in the network are more likely to be cleared out after successful transfer, which reduces the number of unnecessary transmissions for message delivery. By means of the priority for message transmission and deletion in case of the limited network resource, the proposed algorithm achieves the high delivery ratio with low overhead as well as less number of aborted messages due to the insufficient encounter duration, thus is more energy efficient.
The framework of Delay Tolerant Networks (DTNs) has received an extensive attention from academic community because of its application ranging from Wireless Sensor Networks (WSNs) to interplanetary networks. It has a promising future in military affairs, scientific research and exploration. Due to the characteristic of long delay, intermittent connectivity and limited network resource, the traditional routing algorithms do not perform well in DTNs. In this paper, our proposed algorithm is based on an asymmetric spray mechanism combining with the concept of message classes. For each message class, a corresponding forwarding queue is designed and these queues are scheduled according to their priorities. Together with other designed assistant functions, our proposed algorithm outperforms other state of the art algorithms in terms of delivery ratio, overhead ratio, average latency as well as energy consumption.
In this paper, we present a Mobile Edge Computing (MEC) scheme for enabling network edge-assisted video adaptation based on MPEG-DASH (Dynamic Adaptive Streaming over HTTP). In contrast to the traditional over-the-top (OTT) adaptation performed by DASH clients, the MEC server at the mobile network edge can capture radio access network (RAN) conditions through its intrinsic Radio Network Information Service (RNIS) function, and use the knowledge to provide guidance to clients so that they can perform more intelligent video adaptation. In order to support such MECassisted DASH video adaptation, the MEC server needs to locally cache the most popular content segments at the qualities that can be supported by the current network throughput. Towards this end, we introduce a two-dimensional user Quality-of-Experience (QoE)-driven algorithm for making caching / replacement decisions based on both content context (e.g., segment popularity) and network context (e.g., RAN downlink throughput). We conducted experiments by deploying a prototype MEC server at a real LTE-A based network testbed. The results show that our QoE-driven algorithm is able to achieve significant improvement on user QoE over 2 benchmark schemes
Sound event detection (SED) and localization refer to recognizing sound events and estimating their spatial and temporal locations. Using neural networks has become the prevailing method for SED. In the area of sound localization, which is usually performed by estimating the direction of arrival (DOA), learning-based methods have recently been developed. In this paper, it is experimentally shown that the trained SED model is able to contribute to the direction of arrival estimation (DOAE). However, joint training of SED and DOAE degrades the performance of both. Based on these results, a two-stage polyphonic sound event detection and localization method is proposed. The method learns SED first, after which the learned feature layers are transferred for DOAE. It then uses the SED ground truth as a mask to train DOAE. The proposed method is evaluated on the DCASE 2019 Task 3 dataset, which contains different overlapping sound events in different environments. Experimental results show that the proposed method is able to improve the performance of both SED and DOAE, and also performs significantly better than the baseline method.