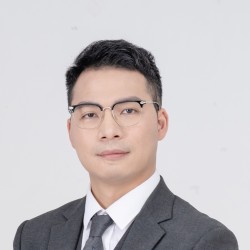
Dr Yinglong (Ian) He
Academic and research departments
School of Mechanical Engineering Sciences, Faculty of Engineering and Physical Sciences, Centre for Automotive Engineering.About
Biography
Dr Yinglong He is a Lecturer in Autonomous Electric Transport (AcT) Systems at the School of Mechanical Engineering Sciences (MES), University of Surrey, UK. Additionally, he holds the title of Honorary Assistant Professor at the School of Engineering, University of Birmingham. In 2022, Dr He worked as a Postdoctoral Research Associate (PDRA) at the Institute of Astronomy (IoA) and the Department of Architecture, University of Cambridge. Between 2019 and 2021 he held an expert position in the Directorate for Energy, Transport, and Climate at the Joint Research Centre (JRC) of the European Commission. Please visit his personal website for more details.
University roles and responsibilities
- Member of MES EDI committee
Affiliations and memberships
- Energy and Environment (EaE)
- AI and Sustainability
News
In the media
ResearchResearch interests
Intelligent transportation systems (ITS)
Energy management & decarbonisation
Vehicle & powertrain dynamics
Multi-agent systems & distributed control
Driving behaviour prediction & state classification
Reinforcement learning & optimal control
Data-driven modelling & AI-driven optimisation
Research interests
Intelligent transportation systems (ITS)
Energy management & decarbonisation
Vehicle & powertrain dynamics
Multi-agent systems & distributed control
Driving behaviour prediction & state classification
Reinforcement learning & optimal control
Data-driven modelling & AI-driven optimisation
Sustainable development goals
My research interests are related to the following:
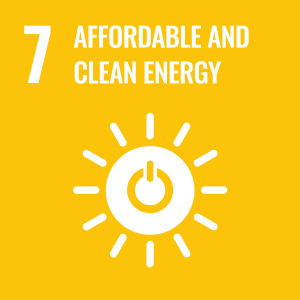
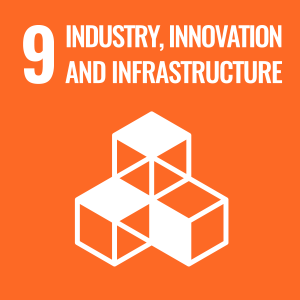
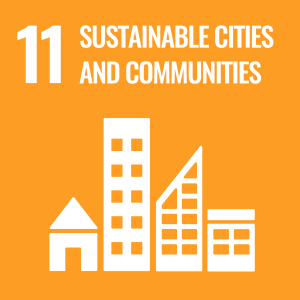
Publications
Hybrid electric vehicles (HEVs) have reached the market share required to meaningfully affect many aspects of the road transport system, including traffic behaviour, energy consumption, and emissions. However, traffic models for hybrids remain insufficiently addressed in microscopic simulation because traditional models ignore vehicle dynamics, and therefore, cannot capture driving differences that exist among the hybrid, conventional, and electric vehicles. This study extends the lightweight microsimulation free-flow acceleration (MFC) model and fills the above gap in the literature by introducing hybrid vehicle dynamics into traffic simulation. First, the methodology underlying the MFC model to reproduce hybrid vehicle dynamics is described, for both charge depleting (CD) and charge sustaining (CS) modes. Then, the experimental setup for model validation and implementation was introduced. The simulations suggest the proposed MFC model can ensure smooth speed and acceleration profiles while converging to the steady state. The results show the MFC model can accurately capture the dynamics of the hybrid vehicle tested on the chassis dynamometer. The MFC model is compared with the Gipps' model and the intelligent driver model (IDM) regarding their abilities to reproduce driving trajectories of the hybrid vehicle. It was found, in CD mode, the MFC model leads to reductions in both speed and acceleration root mean square errors (RMSEs). In CS mode, the MFC model yields even greater accuracy gains. When predicting the 0-100 km/h acceleration specifications, the MFC model also outperforms the Gipps' and the IDM, reducing RMSE by 45.8 % and 51.9 %, respectively.
Hybrid electric vehicles (HEVs) have reached the market share required to meaningfully affect many aspects of the road transport system, including traffic behaviour, energy consumption, and emissions. However, traffic models for hybrids remain insufficiently addressed in microscopic simulation because traditional models ignore vehicle dynamics, and therefore, cannot capture driving differences that exist among the hybrid, conventional, and electric vehicles. This study extends the lightweight microsimulation free-flow acceleration (MFC) model and fills the above gap in the literature by introducing hybrid vehicle dynamics into traffic simulation. First, the methodology underlying the MFC model to reproduce hybrid vehicle dynamics is described, for both charge depleting (CD) and charge sustaining (CS) modes. Then, the experimental setup for model validation and implementation was introduced. The simulations suggest the proposed MFC model can ensure smooth speed and acceleration profiles while converging to the steady state. The results show the MFC model can accurately capture the dynamics of the hybrid vehicle tested on the chassis dynamometer. The MFC model is compared with the Gipps’ model and the intelligent driver model (IDM) regarding their abilities to reproduce driving trajectories of the hybrid vehicle. It was found, in CD mode, the MFC model leads to reductions in both speed and acceleration root mean square errors (RMSEs). In CS mode, the MFC model yields even greater accuracy gains. When predicting the 0–100 km/h acceleration specifications, the MFC model also outperforms the Gipps’ and the IDM, reducing RMSE by 45.8 % and 51.9 %, respectively.
Non-aqueous Li–O2 battery (NALiO2B) is a promising alternative to lithium-ion batteries, offering high theoretical energy density. However, its practical applications are hampered by limited understanding of the underlying mechanisms. In this study, a three-dimensional electrochemical lattice Boltzmann method is proposed to simulate the physical and electrochemical processes during NALiO2B discharge at the pore scale. The discharge performance of NALiO2B is evaluated for various electrode and electrolyte designs. It is found that the limited O2 diffusion within homogeneous electrodes is the primary cause of the declined reactive electrode surface area, the intensified electrochemical reaction (or overpotential), and finally the premature battery death. This issue can be mitigated by employing the hierarchical electrode BP2 with a bi-porous structure. The large pores in BP2 improve O2 transport to sustain the continuous electrochemical reaction process, thus enhancing the discharge capacity of NALiO2B. To further boost the rate capability of NALiO2B, BP2 is partially infiltrated with electrolyte to form the multiphase (MP) electrode, where air bubbles exist and serve as O2 reservoirs. These bubbles effectively provide adequate O2 to support the extensive O2 consumption during the fast electrochemical reaction at high current densities. Consequently, NALiO2B with MP demonstrates the satisfactory discharge capacity and rate capability. This study provides valuable insights into the complex physics and reaction kinetics behind NALiO2B discharge, which facilitates the optimization and development of NALiO2B.
Both energy performance certificates (EPCs) and thermal infrared (TIR) images play key roles in mapping the energy performance of the urban building stock. In this paper, we developed parametric building archetypes using an EPC database and conducted temperature clustering on TIR images acquired from drones and satellite datasets. We evaluated 1,725 EPCs of existing building stock in Cambridge, UK, to generate energy consumption profiles. Drone-based TIR images of individual buildings in two Cambridge University colleges were processed using a machine learning pipeline for thermal anomaly detection and investigated the influence of two specific factors that affect the reliability of TIR for energy management applications: ground sample distance (GSD) and angle of view (AOV). The EPC results suggest that the construction year of the buildings influences their energy consumption. For example, modern buildings were over 30% more energy-efficient than older ones. In parallel, older buildings were found to show almost double the energy savings potential through retrofitting compared to newly constructed buildings. TIR imaging results showed that thermal anomalies can only be properly identified in images with a GSD of 1 m/pixel or less. A GSD of 1-6 m/pixel can detect hot areas of building surfaces. We found that a GSD > 6 m/pixel cannot characterize individual buildings but does help identify urban heat island effects. Additional sensitivity analysis showed that building thermal anomaly detection is more sensitive to AOV than to GSD. Our study informs newer approaches to building energy diagnostics using thermography and supports decision-making for large-scale retrofitting.
Fusion In-vessel components, assembled and maintained using laser welding, one of the most promising techniques, exhibit complex distributions of residual stress, microstructures, and material properties. These residual stresses can compromise structural integrity and lifespan of critical components. Although using advanced experimental measurements can evaluate the residual stress for individual case, extending the measurements to massive number of components are costly and time-consuming. Traditional machine learning (ML) models struggle to account for the heterogeneity and anisotropy of these stress distributions. Here, we develop a novel ML framework based on the Eurofer97 steel, the structural material for in-vessel components. The ML framework is trained on high-resolution residual stress data derived from recently-developed evaluation techniques. Combining with microstructures, the model enables prediction of heterogenous and anisotropic residual stress distribution. It successfully predicts the compressive residual stress in fusion zone (∼−200 MPa) balanced by tensile residual stress in heat affected zone (∼300 MPa), aligning closely with experimental results with the R-squared value of 0.989 and the mean square error of 10−4. Unlike experiments that take hours, the ML model provides predictions within seconds. It offers valuable insights into residual stress prediction for various joints, enhancing the reliability and lifetime prediction of in-vessel components.
The study of driving behaviour has become increasingly important in the development of transport and vehicle technologies. Microscopic traffic models simulate individual driver behaviour to understand and predict traffic flow. One of the key components in microscopic simulation is the car-following (CF) model, which describes the behaviour of vehicles in terms of how they follow the vehicle in front of them. Some excellent reviews of CF models are available, however, to the best of the authors’ knowledge, none of them provides a comprehensive analysis that covers and compares different model categories including kinematics-based, dynamics-based, psychological-based, and learning-based. This paper, therefore, provides an overview of the developments and future directions of CF models, encompassing all the previously mentioned categories. It first introduces the fundamental concepts of traffic models, in particular CF models. It then reviews the progress of CF models, which are classified into the above four categories. The advantages and limitations of existing CF models are discussed. The paper further identifies several research directions for future work, including the integration of emerging vehicle technologies, the incorporation of real-world traffic data, and the calibration and validation of model parameters. It concludes by emphasizing the importance of interdisciplinary collaboration and the need for further research to improve the accuracy and practicality of CF models.
On-ramp merging zones have long been accident-prone spots as well as major triggers of traffic congestion in freeway networks. This paper proposes a collision-free merging control for connected and autonomous vehicles based on trajectory optimization to facilitate traffic safety and efficiency. The optimization is embedded in a model predictive control algorithm with the aim of maximizing the average travel speeds of all vehicles in the planning horizon. To guarantee collision-free merging, the merging control allows only one vehicle to pass the merging zone at a time. Safety constraints, including minimum time gaps and minimum distances between vehicles, are also considered. A rolling horizon control framework is established to enable dynamic traffic control; specifically, generating optimal acceleration decisions for each time step. The optimization problem is formulated as mixed-integer linear programming to enable efficient computation. Numerical studies and sensitivity analysis were conducted to validate the effectiveness and applicability of the proposed method. The results show that the introduced safety constraints can guarantee collision-free merging. Compared to the traditional method, Ih is built on the intelligent driver model and the minimizing overall braking induced by lane-change model, the proposed optimization-based approach results in 5.0%–22.2% improvements with respect to average travel speeds during the merging process. The safety and efficiency improvements are more significant when dealing with higher demand levels. Notably, compared to the difference in initial speeds between the mainline and on-ramp vehicles, the length of the acceleration lane has a greater impact on the control performance.
With the expansion of large-scale wind farms, wind power penetration has surged. However, high turbine operations correlate with rising maintenance costs and failures, often due to blade icing. Blade icing in wind turbines often leads to expensive mechanical and operational emergencies. While many detection methods identify icing presence, they fall short in assessing its impact severity. By observing power changes before and after icing, we can gauge its effect. Hence, we suggest an icing risk assessment method incorporating wind power predictions (WPPs). Single prediction methods, whether physical or data-driven, have distinct strengths and limitations. Physical models are clear but may struggle with complex nonlinearities, while data-driven ones handle such complexities but lack interpretability and rely heavily on data quality. A hybrid approach merges the clarity of physical models with the flexibility of data-driven ones. Therefore, a two-stage hybrid forecasting strategy is developed to improve the accuracy of WPPs. Specifically, a four-parameter model based on power curves is constructed to find the preliminary WPPs, and an error correction model based on a multilayer perceptron is constructed to correct the WPP results. Previous research illustrates the benefits that additional feature data (beyond wind power) brings to the identification of icing failures. To fully utilize more feature information and improve icing risk assessment reliability, we propose a hybrid sand cat swarm optimization and improved fuzzy C-means clustering algorithm to determine power deviation and other feature data related to icing detection. Real-world sensor data from supervisory control and data acquisition systems are used to validate the proposed icing risk assessment method (that considers WPPs). The results indicate that the proposed method can achieve effective risk classification and provide a basis for O&M decisions affecting wind turbine equipment.
•There is a clear relationship between string stability and hysteresis.•String unstable platoons are hysteretic both for simulations and observed trajectories.•A new mechanism that causes hysteresis is investigated.•A new criterion for hysteresis partly explaining the connection to string stability.•The most string stable platoons produced poor flows during the acceleration phase. The existence, source, and characteristics of hysteresis loops in traffic oscillations have been extensively investigated in the past decades. At the same time, the string stability of vehicle platoons has also attracted significant attention and has been considered the main cause of the spontaneously emerging oscillations. However, the relationship between these two seemingly related properties remains underexplored, leading to deficits in knowledge about the effect of microscopic controllers’ operations on macroscopic traffic characteristics. Filling this gap becomes important with the advent of driving automation and the possibility to make future road transport not only safer but also more efficient. This would however require setting proper requirements to their operations, able to ensure that they would generate an efficient traffic flow without limiting too much the freedom of the system developers. By analyzing both simulation and real-world data, the present work has confirmed the existence of a strong correlation between hysteresis and string instability. In particular, string unstable platoons are found to be also hysteretic, while only a limited number of string stable platoons were hysteretic. Further, for a simple linear car following model, hysteresis loops are shown to emerge in the absence of inter-driver and/or intra-driver heterogeneity, which have been suggested as the main sources of hysteresis. This implies a new mechanism that can lead to hysteresis, emerging as a characteristic of the driving behavior. An analytical investigation of this mechanism shows a link to string instability, at least for the simple linear model used. Although further research will be needed in order to generalize the findings of this paper, the present results strongly suggest that introducing a requirement for string stability may lead to a significant reduction in the occurrence and the negative effects of traffic oscillations.
Road geometry (e.g. slope and curvature) has significant impacts on driving behaviours of low-level automated vehicles (AVs), but it has been largely ignored in microscopic traffic models. To capture these effects, this study proposes a generic approach to extend any (free-flow or car-following) microscopic models characterized by acceleration functions. To this end, three model extensions are developed, each of which can use different submodels for comparison. Their effectiveness is demonstrated with a microscopic free-flow model that represents the AVs' control logic. Finally, all possible combinations of the microscopic model and the model extensions are calibrated and cross-validated against data sets, which contain empirical trajectories of commercial adaptive cruise control (ACC) systems on different test tracks. Results suggest that two submodels, i.e., the nonlinear vehicle dynamics (NVD) and the radius difference method (RDM), can extend the microscopic model to effectively capture the effects of road slope and road curvature, respectively, on automated driving. Specifically, the NVD is the dominant factor contributing to increasing (by 34.9% on average) model accuracy. In addition, when simulating reckless turning behaviours (i.e. the vehicle turns at high speeds), the inclusion of the developed RDM is significant for model performance, and the models extended with both the NVD and the RDM can achieve the largest accuracy gains (39.6%).
The growing adoption of hybrid electric vehicles (HEVs) presents a transformative opportunity for revolutionizing transportation energy systems. The shift towards electrifying transportation aims to curb environmental concerns related to fossil fuel consumption. This necessitates efficient energy management systems (EMS) to optimize energy efficiency. The evolution of EMS from HEVs to connected hybrid electric vehicles (CHEVs) represent a pivotal shift. For HEVs, EMS now confronts the intricate energy cooperation requirements of CHEVs, necessitating advanced algorithms for route optimization, charging coordination, and load distribution. Challenges persist in both domains, including optimal energy utilization for HEVs, and cooperative eco-driving control (CED) for CHEVs across diverse vehicle types. Reinforcement learning (RL) stands out as a promising tool for addressing these challenges. Specifically, within the realm of CHEVs, the application of multi-agent reinforcement learning (MARL) emerges as a powerful approach for effectively tackling the intricacies of CED control. Despite extensive research, few reviews span from individual vehicles to multi-vehicle scenarios. This review bridges the gap, highlighting challenges, advancements, and potential contributions of RL-based solutions for future sustainable transportation systems.
This article develops a distributed cooperative energy management system (EMS) with two distributed control layers for speed-coupling plug-in hybrid electric vehicles (PHEVs). By introducing personalized non-stationary inference, this system can fuse driving behavior and vehicle state information to adaptively adjust power-split control parameters for the improvement of vehicle energy economy. In the on-board control layer, five sets of personalized control parameters are optimized offline using chaos-enhanced accelerated particle swarm optimization (CAPSO). In the distributed control layer, interval type 2 (IT2) fuzzy sets are applied to develop a real-time driving style recognition function. The driving behavior is detected remotely, via the vehicle-to-everything (V2X) network, and downloaded to adaptively adjust the power-split control parameters in the on-board vehicle controller. Hardware-in-the-loop testing is carried out based on the four laboratory driving cycles and four personal driving cycles. The proposed system has been demonstrated with strong robustness that saves energy by up to 5.25% over the equivalent consumption minimization strategy (ECMS), especially for gentle drivers. Even under harsh communication conditions (with signal loss 80+%), it still performs better than the ECMS (by 0.57%) and the series–parallel (SP) control strategy (by 2.66%).
•Accuracy of ninety CF models and ACC algorithms is compared.•New controllers with spacing policies from the IDM and Gipps’ models are studied.•The impact on accuracy of increasingly complex lower-level dynamics is evaluated.•The linear controller with Gipps’ spacing policy is the most accurate model.•The IDM is the most robust model, also showing zero crashes in cross-validation. This paper investigates the accuracy and robustness of car-following (CF) and adaptive cruise control (ACC) models in reproducing measured trajectories of commercial ACCs. To this aim, a general modelling framework is proposed, in which ACC and CF models have been incrementally augmented with physics-based extensions: namely, perception delay, linear or nonlinear vehicle dynamics, and acceleration constraints. This framework has been applied to the Intelligent Driver Model (IDM), Gipps’ model, and to three basic ACC algorithms. These are linear controllers which are coupled with a constant time-headway spacing policy, and with two other policies derived from the traffic flow theory: the IDM desired distance function, and Gipps’ equilibrium distance-speed function. The ninety models resulting from the combination of the five base models with the aforementioned extensions, have been assessed and compared through a vast calibration and validation experiment against measured trajectory data of vehicles driven by ACC systems. Overall, the study has shown that physics-based extensions provide limited improvements to the accuracy of existing models. In addition, if an investigation against measured data is not carried out, it is not possible to argue which extension is the most suited for a specific model. The linear controller with Gipps’ spacing policy has resulted the most accurate model, while the IDM the most robust to different input trajectories. Eventually, all models have failed to capture the behaviour of some car brands – just as models fail with some human drivers. Therefore, the choice of the “best” model is independent of the car brand to simulate.
This article proposes a new modular design method for hybrid powertrains using a modified accelerated particle swarm optimization (MAPSO) algorithm. The method determines the optimal combination of component specifications and control parameters, where the component specifications include integer variables (e.g., the number of battery modules). A unified chaotic attraction strategy for MAPSO is developed based on a logistic map to improve the probability of achieving the global optimal result. The Pareto analysis is carried out to identify the weighting value for the tradeoff in modular design. The comprehensive reputation score (CRS), considering both Monte Carlo results and the probability of achieving global optima, is employed to evaluate the advantages of the MAPSO compared with conventional PSO and four other PSO variants. The MAPSO is verified as the best because it has the highest CRS. Both two-level and simultaneous methods for modular design are developed with the MAPSO, where the former first operates component sizing at the level 1 and then conducts control optimization at the level 2, and the later optimizes the size and control simultaneously. Compared with the two-level method, the simultaneous method achieves a 7% higher cost function value and saves 50% time.
This article introduces the concept of the driver-identified supervisory control system, which forms a novel architecture of adaptive energy management for hybrid electric vehicles (HEVs). As a man-machine system, the proposed system can accurately identify the human driver from natural operating signals and provides driver-identified globally optimal control policies as opposed to mere control actions. To help improve the identifiability and efficiency of this control system, the method of spectrum-guided fuzzy feature extraction (SFFE) is developed. First, the configuration of the HEV model and its control system are analyzed. Second, design procedures of the SFFE algorithm are set out to extract 15 groups of features from primitive operating signals. Third, long-term and short-term memory networks are developed as a driver recognizer and tested by the features. The driver identity maps to corresponding control policies optimized by dynamic programming. Finally, the comparative study includes involved extraction methods and their identification system performance as well as their application to HEV systems. The results demonstrate that with help of the SFFE, the driver recognizer improves identifiability by at least 10% compared to that obtained using other involved extraction methods. The improved HEV system is a significant advance over the 5.53% reduction on fuel consumption obtained by the fuzzy-logic-based system.
This paper investigates a model-free supervisory control methodology with double Q-learning for the hybrid vehicle in charge-sustaining scenarios. It aims to improve the vehicle's energy efficiency continuously while maintaining the battery's state-of-charge in real-world driving. Two new heuristic action execution policies, the max-value-based policy and the random policy, are proposed for the double Q-learning method to reduce overestimation of the merit-function values for each action in power-split control of the vehicle. Experimental studies based on software-in-the-loop (offline learning) and hardware-in-the-loop (online learning) platforms are carried out to explore the potential of energy-saving in four driving cycles defined with real-world vehicle operations. The results from 35 rounds of offline undisturbed learning show that the heuristic action execution policies can improve the learning performance of conventional double Q-learning by achieving at least 1.09% higher energy efficiency. The proposed methods achieve similar results obtained by dynamic programming, but they have the capability of real-time online application. Double Q-learnings are shown more robust to turbulence during the disturbed learning: they realise at least three times improvement in energy efficiency compared to the standard Q-learning. Random execution policy achieves 1.18% higher energy efficiency than the max-valuebased policy for the same driving condition. Significant tests show that deciding factor in the random execution policy has little impact on learning performance. By implementing the control strategies for online learning, the proposed model-free control method can save energy by more than 4.55% in the predefined real-world driving conditions compared to the method using standard Q-learning.
•An ethanol-gasoline blend model is incorporated into the PHEV powertrain simulation.•The proposed method can provide market-oriented PHEV design solutions.•Relationships among economic, energy and environment goals in PHEV design are studied.•E25 PHEV can achieve energy saving (5.9%) and GHG emissions reduction (12.3%).•Ethanol in E25 counts 13.8% of the total energy but only 4.8% of the GHG emissions. Concerns over energy efficiency and greenhouse gas (GHG) emissions are driving research investments into advanced propulsion technologies. Plug-in hybrid electric vehicles (PHEVs) can provide a bridge that connects transport electrification to renewable bioenergy sources such as ethanol. However, it remains unclear how this pathway can simultaneously address economic, energy and environmental goals. To tackle this challenge, the present study explores, for the first time, the multiobjective optimal sizing of PHEVs powered by low-carbon sources of electricity and ethanol-gasoline blend. The empirical ethanol-gasoline blend model is incorporated into the PHEV simulation whose relevant parameters are validated using laboratory data from the European Commission – Joint Research Centre. We develop a full picture of the use-phase well-to-wheel (WTW) GHG emissions from ethanol, gasoline and grid electricity and their energy consumptions. Consequently, market-oriented PHEV sizing solutions are provided as per the power utility generation portfolio and automobile fuel properties of the target region. The results indicate that better performances of the PHEV, regarding GHG emissions and energy consumption, are associated with larger battery size and smaller engine displacement but result in a higher cost-to-power ratio. Specifically, for E25-fuelled PHEVs in markets with world average electricity carbon intensity, every 1.0 USD/kW increase in cost-to-power ratio leads to savings of 1.6 MJ energy consumption and 1.7 g CO2-eq/km WTW GHG emissions. Moreover, a clear benefit of using E25 in the hybrid propulsion system is identified, where the energy consumption and GHG emissions can be reduced by 5.9% and 12.3%, respectively.
It has been over two decades since the first generation of adaptive cruise control (ACC) equipped vehicles were launched onto the market. However, control strategies adopted by commercially available ACC systems are the closely-guarded intellectual property of their industrial developers, so these are not publicly available and there is no uniform standard for ACC dynamic response. However, the impact of ACC dynamics on transport networks needs to be studied. In the open literature, there are many ACC systems published and embedded in simulation studies, nevertheless, inappropriate model design can easily point to misleading conclusions. Only a few projects dealing with automated systems are founded on real experimental data, which is important for developing precise ACC models and furthermore, evaluating their realistic effects on highway capacity and traffic flow dynamics This review firstly summarizes the available literature on control strategies for ACC systems implemented on experimental vehicles, then propose a five-layer framework for the development and evaluation of ACC or CACC control strategies based on future trends. (C) 2019, IFAC (International Federation of Automatic Control) Hosting by Elsevier Ltd. All rights reserved.
This study reports occurrence forms of key ash-forming elements in a defatted microalga, and for comparison, its corresponding raw microalga. Freeze-dried powders of a marine microalga (Nannochloropsis oceanica) were sieved to a size fraction of > P > Mg > Na > Ca. Chemical fractionation results suggest that virtually all of the Na, K, and Cl in the raw and defatted microalgae are water-soluble. While majority of P in the two fuels are water-soluble and acid-soluble, most of Mg and Ca are leached in water and NH4Ac solution. As determined via chemical fractionation analysis, lipid extraction leads to the content of water-soluble Ca in the defatted microalga being similar to 69.6% higher than that in the raw microalga counterpart, which is accompanied by a reduction in the amount of Ca leached in NH4Ac solution. Similar trend is also observed for Mg, but to a lesser extent. (C) 2017 Elsevier Ltd. All rights reserved.
Connected and automated vehicles (CAVs) promise to significantly improve road traffic. To a certain extent, this situation is similar to the expectations at the end of the last century about the positive effects that the introduction of Adaptive Cruise Control (ACC) systems would have had on motorway traffic. The parallelism is interesting because ACC equipped vehicles represent the first level of vehicle automation and are now widely available on the market. In this light, studying ACC impacts can help to anticipate potential problems related to its widespread application and to avoid that AVs and CAVs will lead to the same problems. Only a few test-campaigns had been carried out studying the ACC impacts under real-world driving conditions in quantitative terms. To bridge this gap, the Joint Research Centre of the European Commission has organized a number of experimental campaigns involving several ACC-equipped vehicles to study different implications of their widespread. In this context, the present paper summarizes the outcomes of a test campaign involving 10 commercially available ACC-equipped vehicles. The test campaign has been executed in two different test-tracks of the ZalaZONE proving ground, in Hungary. The tests have been carried out at low-speeds (30–60 km/h) and have involved platoons of vehicles of different brands and different powertrains, which were tested in a variety of vehicle orders and with different settings of their ACC systems. Test results have been used to derive information about the properties of the different ACC systems, to study their string stability, to study the effect of ACC systems on traffic flow, and to draw inference about the possible implications on energy consumption and traffic safety. Results confirm the previous findings in terms of string instability of the ACC and highlight that in the present form, ACC systems will possibly lead to higher energy consumption and introduce new safety risks when their penetration in the fleet increases. However, they also highlight that the materialization of the above findings for AVs depends on the operational logic that manufacturers will adopt during the implementation phase. Therefore, results suggest that functional requirements to guarantee string stability and in general to not disrupt the normal flow of traffic should be introduced both for ACC and for any automated system that will be placed on the market in the future.
•Reinforcement Learning is researched for energy saving in a hybrid vehicle.•Energy efficiency can be continuously improved by multi-step learning.•The ‘Recurrent-to-Terminal’ strategy is shown the most effective learning strategy.•The method can save energy by more than 7.8% in the selected real-time operations. The energy management system of an electrified vehicle is one of the most important supervisory control systems which manages the use of on-board energy resources. This paper researches a ‘model-free’ predictive energy management system for a connected electrified off-highway vehicle. A new reinforcement learning algorithm with the capability of ‘multi-step’ learning is proposed to enable the all-life-long online optimisation of the energy management control policy. Three multi-step learning strategies (Sum-to-Terminal, Average-to-Neighbour Recurrent-to-Terminal) are researched for the first time. Hardware-in-the-loop tests are carried out to examine the control functionality for real application of the proposed ‘model-free’ method. The results show that the proposed method can continuously improve the vehicle’s energy efficiency during the real-time hardware-in-the-loop test, which increased from the initial level of 34% to 44% after 5 h’ 35-step learning. Compared with a well-designed model-based predictive energy management control policy, the model-free predictive energy management method can increase the prediction horizon length by 71% (from 35 to 65 steps with 1 s interval in real-time computation) and can save energy by at least 7.8% for the same driving conditions.
This paper investigates an online predictive control strategy for series-parallel plug-in hybrid electric vehicles (PHEVs), resulting in a novel online optimization methodology named the dual-loop online intelligent programming (DOIP) that is proposed for velocity prediction and energy-flow control. By reconsidering the change of driving behaviours at each look-ahead step, this methodology guarantees the effectiveness of optimal control sequence in the energy-saving efficiency of online predictive energy management. The design procedure starts with the simulation of a series-parallel PHEV using a systematic control-oriented model and the definition of a cost function. Inspired by fuzzy granulation technology, a deep fuzzy predictor is created to achieve driver-oriented velocity prediction, and a finite-state Markov chain is exploited to learn transition probabilities between vehicle speed and acceleration. To determine the optimal control behaviours and power distribution between two energy sources, chaos-enhanced accelerated swarm optimization is developed for the DOIP algorithm. The prediction capability of the deep fuzzy predictor is evaluated by comparing with two existing predictors over the WLTP-based driving cycle. The proposed control strategy is contrasted with short-sighted and dynamic programming based counterparts, and validated by a driver-in-the-loop test. The results demonstrate that the deep fuzzy predictor can effectively recognize driving behaviour and reduce at least 19% errors compared to involved Markov chain based predictors. Online predictive control strategy using the DOIP algorithm is able to significantly reduce 9.37% fuel consumption from the baseline and shorten computational time.
Background Surging acceptance of adaptive cruise control (ACC) across the globe is further escalating concerns over its energy impact. Two questions have directed much of this project: how to distinguish ACC driving behaviour from that of the human driver and how to identify the ACC energy impact. As opposed to simulations or test-track experiments as described in previous studies, this work is unique because it was performed in real-world car-following scenarios with a variety of vehicle specifications, propulsion systems, drivers, and road and traffic conditions. Methods Tractive energy consumption serves as the energy impact indicator, ruling out the effect of the propulsion system. To further isolate the driving behaviour as the only possible contributor to tractive energy differences, two techniques are offered to normalize heterogeneous vehicle specifications and road and traffic conditions. Finally, ACC driving behaviour is compared with that of the human driver from transient and statistical perspectives. Its impact on tractive energy consumption is then evaluated from individual and platoon perspectives. Results Our data suggest that unlike human drivers, ACC followers lead to string instability. Their inability to absorb the speed overshoots may partly be explained by their high responsiveness from a control theory perspective. Statistical results might imply the followers in the automated or mixed traffic flow generally perform worse in reproducing the driving style of the preceding vehicle. On the individual level, ACC followers have tractive energy consumption 2.7-20.5% higher than those of human counterparts. On the platoon level, the tractive energy values of ACC followers tend to consecutively increase (11.2-17.3%). Conclusions In general, therefore, ACC impacts negatively on tractive energy efficiency. This research provides a feasible path for evaluating the energy impact of ACC in real-world applications. Moreover, the findings have significant implications for ACC safety design when handling the stability-responsiveness trade-off.
Electrification, automation, and connectivity in the automotive and transport industries are gathering momentum, but there are escalating concerns over their need for co-optimization to improve energy efficiency, traffic safety, and ride comfort. Previous approaches to these multiobjective co-optimization problems often overlook tradeoffs and scale differences between the objectives, resulting in misleading optimizations. To overcome these limitations, this article proposes a Pareto-based framework that demonstrably optimizes the system parameters of the cooperative adaptive cruise control (CACC) and the energy management strategy (EMS) for plug-in hybrid electric vehicles (PHEVs). The high-level Pareto knowledge assists in finding a best compromise solution. The results of this article suggest that the energy and the comfort targets are harmonious, but both conflict with the safety target. Validation using real-world driving data shows that the Pareto optimum for CACC and EMS systems, relative to the baseline, can reduce energy consumption (by 7.57%) and tracking error (by 68.94%) while simultaneously satisfying ride comfort needs. In contrast to the weighted-sum method, the proposed Pareto method can optimally balance and scale the multiple-objective functions. In addition, sensitivity analysis proves that the vehicle reaction time impacts significantly on tracking safety, but its effect on energy saving is trivial.
Low-carbon transitions require joint efforts from electricity grid and transport network, where electric vehicles (EVs) play a key role. Particularly, EVs can reduce the carbon emissions of transport networks through eco-routing while providing the carbon intensity service for power networks via vehicle-to-grid technique. Distinguishing from previous research that focused on EV routing and scheduling problems separately, this paper studies their coordinated effect with the objective of carbon emission reduction on both sides. To solve this problem, we propose a multi-agent reinforcement learning method that does not rely on prior knowledge of the system and can adapt to various uncertainties and dynamics. The proposed method learns a hierarchical structure for the mutually exclusive discrete routing and continuous scheduling decisions via a hybrid policy. Extensive case studies based on a virtual 7-node 10-edge transport and 15-bus power network as well as a coupled real-world central London transport and 33-bus power network are developed to demonstrate the effectiveness of the proposed MARL method on reducing carbon emissions in transport network and providing carbon intensity service in power network.
IntroductionThe successful use of machine learning (ML) for medical diagnostic purposes has prompted myriad applications in cancer image analysis. Particularly for hepatocellular carcinoma (HCC) grading, there has been a surge of interest in ML-based selection of the discriminative features from high-dimensional magnetic resonance imaging (MRI) radiomics data. As one of the most commonly used ML-based selection methods, the least absolute shrinkage and selection operator (LASSO) has high discriminative power of the essential feature based on linear representation between input features and output labels. However, most LASSO methods directly explore the original training data rather than effectively exploiting the most informative features of radiomics data for HCC grading. To overcome this limitation, this study marks the first attempt to propose a feature selection method based on LASSO with dictionary learning, where a dictionary is learned from the training features, using the Fisher ratio to maximize the discriminative information in the feature. MethodsThis study proposes a LASSO method with dictionary learning to ensure the accuracy and discrimination of feature selection. Specifically, based on the Fisher ratio score, each radiomic feature is classified into two groups: the high-information and the low-information group. Then, a dictionary is learned through an optimal mapping matrix to enhance the high-information part and suppress the low discriminative information for the task of HCC grading. Finally, we select the most discrimination features according to the LASSO coefficients based on the learned dictionary. Results and discussionThe experimental results based on two classifiers (KNN and SVM) showed that the proposed method yielded accuracy gains, compared favorably with another 5 state-of-the-practice feature selection methods.
Plug-in hybrid vehicles will become a major part of urban transportation before 2030. The hybrid powertrain is a micro energy system that needs to be managed to achieve low carbon emission. Dynamic programming is widely adopted to optimize the energy efficiency, but it cannot be directly used for real-time control. This paper proposes a new Global K-fold Fuzzy Learning (GKFL) scheme to implement the offline optimization results in real-time control with adaptive neuro-fuzzy inference system (ANFIS). It aims to obtain an ANFIS network that can robustly achieve the optimum control utility which is defined as a function of the vehicle’s energy efficiency and the battery state-of-charge (SoC). The performances of the 2 ANFIS network systems developed by both standard method and the GKFL method respectively are evaluated through experimental studies. GKFL is shown effective in knowledge implementation. Compared to the default solver in the MATLAB ANFIS toolbox, GKFL can increase the control utility of the studied vehicle by 8% in the Worldwide-harmonized Light-duty Testing Cycle.
•Commercial ACC systems have a string-unstable behavior.•String-stable ACCs rely on the use of communication which is not currently available.•ACC can still be string stable by taking advantage of RADAR sensing characteristics.•Monitoring two vehicles downstream is sufficient to ensure string-stability in a wide range of scenarios.•RADAR based multianticipation can provide a plug-and-play solution to achieve efficient traffic. Adaptive Cruise Control (ACC) systems have been expected to solve many problems of motorway traffic. Now that they are widespread, it is observed that the majority of existing systems are string unstable. Therefore, small perturbations in the speed profile of a vehicle are amplified for the vehicles following upstream, with negative impacts on traffic flow, fuel consumption, and safety. Increased headway settings provide more stable flow but at the same time it deteriorates the capacity. Substantial research has been carried out in the past decade on utilizing connectivity to overcome this trade-off. However, such connectivity solutions have to overcome several obstacles before deployment and there is the concrete risk that motorway traffic flow will considerably deteriorate in the meanwhile. As an alternative solution, the paper explores multianticipation without inter-vehicle communication, taking advantage of the recent advancements in the field of RADAR sensing. An analytical study is carried out, based on the most widely used model and parameter settings used to simulate currently available commercial ACC systems, comparing the transfer functions and step responses for the nominal and the multianticipative formulations. Then, a microsimulation framework is employed to validate our claim on different speed profiles. Analytical results demonstrate that multianticipation enhances stability without impacting traffic flow. On the contrary, the simulation study indicates that the multianticipative-ACC can produce higher road capacity even in the presence of external disturbances and for a wide range of calibrated parameters. Finally, optimality conditions for the tuning of the headway policy are derived from a Pareto optimization.
Many studies have highlighted the added value of incorporating vehicle dynamics into microsimulation. Such models usually focus on simulation of conventional vehicles, failing to account for the acceleration dynamics of electrified vehicles that have different power characteristics from those of internal combustion engine vehicles (ICEV). In addition, none of them have explicitly dealt with the vehicle's deceleration characteristics. Although it is not commonly considered critical how a vehicle decelerates, unrealistic behaviors in simulations can distort both traffic flow and emissions results. The present work builds on the lightweight microsimulation free-flow acceleration (MFC) model and proposes an extension, marking the first attempt to address these research gaps. First, a comprehensive review of dynamics-based car-following (including free-flow) models is conducted. Second, the methodology of the MFC model to capture the dynamics of electrified vehicles is described. Then, the experimental setup in different dimensions is introduced for the model validation and implementation. Finally, the results of this study indicate that: (1) the acceleration and deceleration potential curves underlying the MFC model can accurately represent real dynamics of electrified vehicles tested on the chassis dynamometer; (2) smooth transitions can be guaranteed after implementing the MFC model in microsimulation; (3) when reproducing the on-road driving trajectories, the MFC model can deliver significant reductions in root mean square error (RMSE) of speed (by similar to 69%) and acceleration (by similar to 50%) compared with benchmarks; (4) the MFC model can accurately predict the vehicle 0-100 km/h acceleration specifications, with RMSE 49.4% and 56.8% lower than those of the Gipps model and the intelligent driver model (IDM), respectively.
Additional publications
- Quan Zhou, Ji Li, YINGLONG HE, Bin Shuai, Huw Williams, Hongming Xu, Yanfei Li, Fuwu Yan (2020) K-fold fuzzy learning for implementation of dynamic programming results in real-time energy management of the plug-in hybrid vehicle, In: Applied Energy Symposium 2020: Low Carbon Cities and Urban Energy Systems. Tokyo, Japan.
- YINGLONG HE, Dunxi Yu, Timan Lei, Weizhi Lv, Minghou Xu (2016) Chemical looping CO2/CH4 reforming using Fe-based oxygen carrier for syngas production, In: CIESC Journal/Huagong Xuebao 67(12) pp. 5222-5228.
- Dunxi Yu, YINGLONG HE, Minghou Xu, Weizhi Lv, Bin Fan (2016) Method and apparatus for synthesizing dimethyl ether using CO2, In: China Patent (ZL 2014 1 0853700.5).