11am - 12 noon
Friday 3 May 2024
Attribute-Aware Face Recognition
PhD Open Viva Presentation by Lei Ju
All Welcome!
Free
University of Surrey
Guildford
Surrey
GU2 7XH
This event has passed
Attribute-Aware Face Recognition
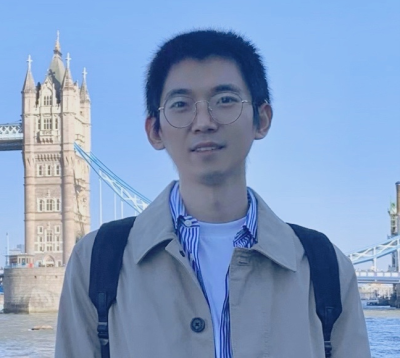
Abstract:
Our research presents novel contributions to the field of attribute-aware face recognition, addressing fundamental challenges in efficiency, bias, and integration of attributes with identity features. Our work spans three main areas, each contributing to the overarching goal of enhancing the robustness and fairness or a face recognition system while managing computational complexity.
First, we tackle the inefficiencies of modern anchor-based face detectors, which, despite their effectiveness, suffer from computational complexity due to redundant feature extraction and unreliable decision-making processes. We introduce a heatmap-assisted spatial attention module and a scale-aware layer attention module, which significantly reduces computational costs by adaptively focusing on informative features and employing a spatial feature selection that highlights facial areas. Our approach, which combines heatmap scores with classification results for decision-making, demonstrates notable improvements in efficiency and reliability on well-known benchmarks.
Second, recognising the importance of attribute awareness in face recognition, we address the challenge of feature disentanglement to mitigate potential biases and privacy concerns. By leveraging the Nearest neighbours Proxy Triple (NPT) loss and introducing an innovative Adaptive-rank NPT loss, we achieve a natural separation of identity and attribute features, enhancing both accuracy and fairness. Our method, namely Ada2NPT loss, outperforms the state-of-the-art losses by promoting inter-class separability and intra-class compactness, as evidenced by our experiments on several benchmarking datasets.
Last, we propose a novel approach to overcome the limitations of traditional representation learning, which struggles with integrating multiple attribute features with identity features due to discretised labels and attribute prediction fallibility. Utilising a pre-trained vision-language model, we transfer facial attributes into prompts for extracting embeddings, thereby achieving a dynamic integration of attribute information into identity embeddings. This prompt-driven method not only reduces false positives across diverse attributes but also establishes a new state-of-the-art for attribute-aware face recognition as validated on several benchmarks.
In conclusion, our research advances the state-of-the-art in attribute-aware face recognition by introducing efficient, fair, and dynamic methods for feature extraction, decision-making, and attribute integration. Our contributions promise significant improvements in the robustness and fairness of a face recognition system, setting a new standard for future developments in the field.