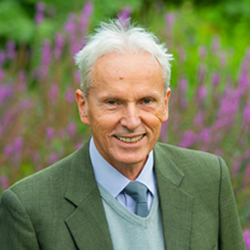
Professor Josef Kittler
Academic and research departments
Centre for Vision, Speech and Signal Processing (CVSSP), School of Computer Science and Electronic Engineering.About
Biography
I have been a Research Assistant in the Engineering Department of Cambridge University (1973--75), SERC Research Fellow at the University of Southampton (1975-77), Royal Society European Research Fellow, Ecole Nationale Superieure des Telecommuninations, Paris (1977--78), IBM Research Fellow, Balliol College, Oxford (1978--80), Principal Research Associate, SERC Rutherford Appleton Laboratory (1980--84) and Principal Scientific Officer, SERC Rutherford Appleton Laboratory (1985).
I also worked as the SERC Coordinator for Pattern Analysis (1982), and was Rutherford Research Fellow in Oxford University, Dept. Engineering Science (1985).
I joined the Department of Electrical Engineering of Surrey University in 1986 as a Reader in Information Technology, and became Professor of Machine Intelligence in 1991 and gained the title Distinguished Professor in 2004.
Affiliations and memberships
ResearchResearch interests
I have worked on various theoretical aspects of pattern recognition, image analysis and computer vision, and on many applications including:
- System identification
- Automatic inspection
- ECG diagnosis
- Mammographic image interpretation
- Remote sensing
- Robotics
- Speech recognition
- Character recognition and document processing
- Image coding
- Biometrics
- Image and video database retrieval
- Surveillance.
Contributions to statistical pattern recognition include k-nearest neighbour methods of pattern classification, feature selection, contextual classification, probabilistic relaxation and most recently to multiple expert fusion. In computer vision my major contributions include robust statistical methods for shape analysis and detection, motion estimation and segmentation, and image segmentation by thresholding and edge detection.
I have co-authored a book with the title `Pattern Recognition: a statistical approach' published by Prentice-Hall and published more than 500 papers.
Indicators of esteem
Received Best Paper awards from the Pattern Recognition Society, the British Machine Vision Association and IEE.
Received ``Honorary Medal'' from the Electrotechnical Faculty of the Czech Technical University in Prague in September 1995 for contributions to the field of pattern recognition and computer vision.
Elected Fellow of the International Association for Pattern Recognition in 1998.
Elected Fellow of Institution of Electrical Engineers in 1999.
Received Honorary Doctorate from the Lappeenranta University of Technology, Finland, for contributions to Pattern Recognition and Computer Vision in 1999.
Elected Fellow of the Royal Academy of Engineering, 2000.
Received Institution of Electrical Engineers Achievements Medal 2002 for outstanding contributions to Visual Information Engineering.
Elected BMVA Distinguished Fellow 2002.
Received, from the Czech Academy of Sciences, the 2003 Bernard Bolzano Honorary Medal for Merit in the Mathematical Sciences.
Awarded the title Distinguished Professor of the University of Surrey in 2004.
Appointed as Series Editor for Springer Lecture Notes in Computer Science 2004.
Awarded the KS Fu Prize 2006, by the International Association for Pattern Recognition, for outstanding contributions to Pattern Recognition (the prize awarded biennially).
Received Honorary Doctorate from the Czech Technical University in Prague in 2007, on the occasion of the 300th anniversary of its foundation.
Awarded the IET Faraday Medal 2008.
Research interests
I have worked on various theoretical aspects of pattern recognition, image analysis and computer vision, and on many applications including:
- System identification
- Automatic inspection
- ECG diagnosis
- Mammographic image interpretation
- Remote sensing
- Robotics
- Speech recognition
- Character recognition and document processing
- Image coding
- Biometrics
- Image and video database retrieval
- Surveillance.
Contributions to statistical pattern recognition include k-nearest neighbour methods of pattern classification, feature selection, contextual classification, probabilistic relaxation and most recently to multiple expert fusion. In computer vision my major contributions include robust statistical methods for shape analysis and detection, motion estimation and segmentation, and image segmentation by thresholding and edge detection.
I have co-authored a book with the title `Pattern Recognition: a statistical approach' published by Prentice-Hall and published more than 500 papers.
Indicators of esteem
Received Best Paper awards from the Pattern Recognition Society, the British Machine Vision Association and IEE.
Received ``Honorary Medal'' from the Electrotechnical Faculty of the Czech Technical University in Prague in September 1995 for contributions to the field of pattern recognition and computer vision.
Elected Fellow of the International Association for Pattern Recognition in 1998.
Elected Fellow of Institution of Electrical Engineers in 1999.
Received Honorary Doctorate from the Lappeenranta University of Technology, Finland, for contributions to Pattern Recognition and Computer Vision in 1999.
Elected Fellow of the Royal Academy of Engineering, 2000.
Received Institution of Electrical Engineers Achievements Medal 2002 for outstanding contributions to Visual Information Engineering.
Elected BMVA Distinguished Fellow 2002.
Received, from the Czech Academy of Sciences, the 2003 Bernard Bolzano Honorary Medal for Merit in the Mathematical Sciences.
Awarded the title Distinguished Professor of the University of Surrey in 2004.
Appointed as Series Editor for Springer Lecture Notes in Computer Science 2004.
Awarded the KS Fu Prize 2006, by the International Association for Pattern Recognition, for outstanding contributions to Pattern Recognition (the prize awarded biennially).
Received Honorary Doctorate from the Czech Technical University in Prague in 2007, on the occasion of the 300th anniversary of its foundation.
Awarded the IET Faraday Medal 2008.
Publications
In recent years, cross-media retrieval has drawn considerable attention due to the exponential growth of multimedia data. Many hashing approaches have been proposed for the cross-media search task. However, there are still open problems that warrant investigation. For example, most existing supervised hashing approaches employ a binary label matrix, which achieves small margins between wrong labels (0) and true labels (1). This may affect the retrieval performance by generating many false negatives and false positives. In addition, some methods adopt a relaxation scheme to solve the binary constraints, which may cause large quantization errors. There are also some discrete hashing methods that have been presented, but most of them are time-consuming. To conquer these problems, we present a label relaxation and discrete matrix factorization method (LRMF) for cross-modal retrieval. It offers a number of innovations. First of all, the proposed approach employs a novel label relaxation scheme to control the margins adaptively, which has the benefit of reducing the quantization error. Second, by virtue of the proposed discrete matrix factorization method designed to learn the binary codes, large quantization errors caused by relaxation can be avoided. The experimental results obtained on two widely-used databases demonstrate that LRMF outperforms state-of-the-art cross- media methods.
In recent years, Discriminative Correlation Filters (DCFs) have gained popularity due to their superior performance in visual object tracking. However, existing DCF trackers usually learn filters using fixed attention mechanisms that focus on the centre of an image and suppresses filter amplitudes in surroundings. In this paper, we propose an Adaptive Context-Aware Discriminative Correlation Filter (ACA-DCF) that is able to improve the existing DCF formulation with complementary attention mechanisms. Our ACA-DCF integrates foreground attention and background attention for complementary context-aware filter learning. More importantly, we ameliorate the design using an adaptive weighting strategy that takes complex appearance variations into account. The experimental results obtained on several well-known benchmarks demonstrate the effectiveness and superiority of the proposed method over the state-of-the-art approaches.
Any high-dimensional data arising from practical applications usually contains irrelevant features that may impact on the performance of existing subspace clustering methods. This paper proposes a novel subspace clustering method which reconstructs the feature matrix by the means of unsupervised feature selection (UFS) to achieve a better dictionary for subspace clustering (SC). Different from most existing clustering methods, the proposed approach uses the reconstructed feature matrix as the dictionary rather than the original data matrix. As the feature matrix reconstructed by representative features is more discriminative and closer to the ground-truth, it results in improved performance. The corresponding non-convex optimization problem is effectively solved using the half-quadratic and augmented Lagrange multiplier methods. Extensive experiments on four real datasets demonstrate the effectiveness of the proposed method.
The Softmax prediction function is widely used to train Deep Convolutional Neural Networks (DCNNs) for large-scale face recognition and other applications. The limitation of the softmax activation is that the resulting probability distribution always has a full support. This full support leads to larger intraclass variations. In this paper, we formulate a novel loss function, called Angular Sparsemax for face recognition. The proposed loss function promotes sparseness of the hypotheses prediction function similar to Sparsemax [1] with Fenchel-Young regularisation. By introducing an additive angular margin on the score vector, the discriminatory power of the face embedding is further improved. The proposed loss function is experimentally validated on several databases in terms of recognition accuracy. Its performance compares well with the state of the art Arcface loss.
The Visual Object Tracking challenge VOT2021 is the ninth annual tracker benchmarking activity organized by the VOT initiative. Results of 71 trackers are presented; many are state-of-the-art trackers published at major computer vision conferences or in journals in recent years. The VOT2021 challenge was composed of four sub-challenges focusing on different tracking domains: (i) VOT-ST2021 challenge focused on short-term tracking in RGB, (ii) VOT-RT2021 challenge focused on "real-time" short-term tracking in RGB, (iii) VOT-LT2021 focused on long-term tracking, namely coping with target disappearance and reappearance and (iv) VOT-RGBD2021 challenge focused on long-term tracking in RGB and depth imagery. The VOT-ST2021 dataset was refreshed, while VOT-RGBD2021 introduces a training dataset and sequestered dataset for winner identification. The source code for most of the trackers, the datasets, the evaluation kit and the results along with the source code for most trackers are publicly available at the challenge website 1 .
While the remarkable advances in face matching render face biometric technology more widely applicable, its successful deployment may be compromised by face spoofing. Recent studies have shown that anomaly-based face spoofing detectors offer an interesting alternative to the multiclass counterparts by generalising better to unseen types of attack. In this work, we investigate the merits of fusing multiple anomaly spoofing detectors in the unseen attack scenario via a Weighted Averaging (WA) and client-specific design. We propose to optimise the parameters of WA by a two-stage optimisation method consisting of Particle Swarm Optimisation (PSO) and the Pattern Search (PS) algorithms to avoid the local minimum problem. Besides, we propose a novel scoring normalisation method which could be effectively applied in extreme cases such as heavy-tailed distributions. We evaluate the capability of the proposed system on publicly available face anti-spoofing databases including Replay-Attack, Replay-Mobile and Rose-Youtu. The experimental results demonstrate that the proposed fusion system outperforms the majority of anomaly-based and state-of-the-art multiclass approaches.
Hashing methods help retrieve swiftly in large-scale dataset, which is important for real-world image retrieval. New data is produced continually in the real world which may cause concept drift and inaccurate retrieval results. To address this issue, hashing methods in non-stationary environments are proposed. However, most hashing methods in non-stationary data environments are supervised. In practice, it is hard to get exact labels of data especially in non-stationary data environments. Therefore, we propose the unsupervised multi-hashing (UMH) method for unsupervised image retrieval in non-stationary environments. Thus, in the UMH, a set of hash functions is trained and added to the kept list of hash functions sets when a new data chunk occurs. Then, multiple sets of hash functions are kept with different weights to guarantee that similarity information in old and new data are both adapted. Experiments on two real-world image datasets show that the UMH yields better retrieval performance in non-stationary environments than other comparative methods.
We present a review of current methods for 3D face modeling, 3D to 3D and 3D to 2D registration, 3D based recognition, and 3D assisted 2D based recognition. The emphasis is on the 3D registration which plays a crucial role in the recognition chain. An evaluation study of a mainstream state-of-the-art 3D face registration algorithm is carried out and the results discussed
Recently, impressively growing efforts have been devoted to the challenging task of facial age estimation. The improvements in performance achieved by new algorithms are measured on several benchmarking test databases with different characteristics to check on consistency. While this is a valuable methodology in itself, a significant issue in the most age estimation related studies is that the reported results lack an assessment of intrinsic system uncertainty. Hence, a more in-depth view is required to examine the robustness of age estimation systems in different scenarios. The purpose of this paper is to conduct an evaluative and comparative analysis of different age estimation systems to identify trends, as well as the points of their critical vulnerability. In particular, we investigate four age estimation systems, including the online Microsoft service, two best state-of-the-art approaches advocated in the literature, as well as a novel age estimation algorithm. We analyse the effect of different internal and external factors, including gender, ethnicity, expression, makeup, illumination conditions, quality and resolution of the face images, on the performance of these age estimation systems. The goal of this sensitivity analysis is to provide the biometrics community with the insight and understanding of the critical subject-, camera- and environmental-based factors that affect the overall performance of the age estimation system under study.
A big, diverse and balanced training data is the key to the success of deep neural network training. However, existing publicly available datasets used in facial landmark localization are usually much smaller than those for other computer vision tasks. To mitigate this issue, this paper presents a novel Separable Batch Normalization (SepBN) method. Different from the classical BN layer, the proposed SepBN module learns multiple sets of mapping parameters to adaptively scale and shift the normalized feature maps via a feed-forward attention mechanism. The channels of an input tensor are divided into several groups and the different mapping parameter combinations are calculated for each group according to the attention weights to improve the parameter utilization. The experimental results obtained on several well-known benchmarking datasets demonstrate the effectiveness and merits of the proposed method.
To counteract spoofing attacks, the majority of recent approaches to face spoofing attack detection formulate the problem as a binary classification task in which real data and attack-accesses are both used to train spoofing detectors. Although the classical training framework has been demonstrated to deliver satisfactory results, its robustness to unseen attacks is debatable. Inspired by the recent success of anomaly detection models in face spoofing detection, we propose an ensemble of one-class classifiers fused by a Stacking ensemble method to reduce the generalisation error in the more realistic unseen attack scenario. To be consistent with this scenario, anomalous samples are considered neither for training the component anomaly classifiers nor for the design of the Stacking ensemble. To achieve better face-anti spoofing results, we adopt client-specific information to build both constituent classifiers as well as the Stacking combiner. Besides, we propose a novel 2-stage Genetic Algorithm to further improve the generalisation performance of Stacking ensemble. We evaluate the effectiveness of the proposed systems on publicly available face anti-spoofing databases including Replay-Attack, Replay-Mobile and Rose-Youtu. The experimental results following the unseen attack evaluation protocol confirm the merits of the proposed model.
Cross-modal content generation has become very popular in recent years. To generate high-quality and realistic content, a variety of methods have been proposed. Among these approaches, visual content generation has attracted significant attention from academia and industry due to its vast potential in various applications. This survey provides an overview of recent advances in visual content generation conditioned on other modalities, such as text, audio, speech, and music, with a focus on their key contributions to the community. In addition, we summarize the existing publicly available datasets that can be used for training and benchmarking cross-modal visual content generation models. We provide an in-depth exploration of the datasets used for audio-to-visual content generation, filling a gap in the existing literature. Various evaluation metrics are also introduced along with the datasets. Furthermore, we discuss the challenges and limitations encountered in the area, such as modality alignment and semantic coherence. Last, we outline possible future directions for synthesizing visual content from other modalities including the exploration of new modalities, and the development of multi-task multi-modal networks. This survey serves as a resource for researchers interested in quickly gaining insights into this burgeoning field.
—Existing studies in facial age estimation have mostly focused on intra-dataset protocols that assume training and test images captured under similar conditions. However, this is rarely valid in practical applications, where training and test sets usually have different characteristics. In this paper, we advocate a cross-dataset protocol for age estimation benchmarking. In order to improve the cross-dataset age estimation performance, we mitigate the inherent bias caused by the learning algorithm itself. To this end, we propose a novel loss function that is more effective for neural network training. The relative smoothness of the proposed loss function is its advantage with regards to the optimisation process performed by stochastic gradient descent. Its lower gradient, compared with existing loss functions, facilitates the discovery of and convergence to a better optimum, and consequently a better generalisation. The crossdataset experimental results demonstrate the superiority of the proposed method over the state-of-the-art algorithms in terms of accuracy and generalisation capability.
Diagnosis of skin lesions is a challenging task due to the similarities between different lesion types, in terms of appearance, location, and size. We present a deep learning method for skin lesion classification by fine-tuning three pre-trained deep learning architectures (Xception, Inception-ResNet-V2, and NasNetLarge), using the training set provided by ISIC2019 organizers. We combine deep convolutional networks with the Error Correcting Output Codes (ECOC) framework to address the open set classification problem and to deal with the heavily imbalanced dataset of ISIC2019. Experimental results show that the proposed framework achieves promising performance that is comparable with the top results obtained in the ISIC2019 challenge leaderboard.
Self-supervised pretraining (SSP) has emerged as a popular technique in machine learning, enabling the extraction of meaningful feature representations without labelled data. In the realm of computer vision, pretrained vision transformers (ViTs) have played a pivotal role in advancing transfer learning. Nonetheless, the escalating cost of finetuning these large models has posed a challenge due to the explosion of model size. This study endeavours to evaluate the effectiveness of pure self-supervised learning (SSL) techniques in computer vision tasks, obviating the need for finetuning, with the intention of emulating human-like capabilities in generalisation and recognition of unseen objects. To this end, we propose an evaluation protocol for zero-shot segmentation based on a prompting patch. Given a point on the target object as a prompt, the algorithm calculates the similarity map between the selected patch and other patches, upon that, a simple thresholding is applied to segment the target. Another evaluation is intra-object and inter-object similarity to gauge discriminatory ability of SSP ViTs. Insights from zero-shot segmentation from prompting and discriminatory abilities of SSP led to the design of a simple SSP approach, termed MMC. This approaches combines Masked image modelling for encouraging similarity of local features, Momentum based self-distillation for transferring semantics from global to local features, and global Contrast for promoting semantics of global features, to enhance discriminative representations of SSP ViTs. Consequently, our proposed method significantly reduces the overlap of intra-object and inter-object similarities, thereby facilitating effective object segmentation within an image. Our experiments reveal that MMC delivers top-tier results in zero-shot semantic segmentation across various datasets.
Contrastive learning has achieved great success in skeleton-based action recognition. However, most existing approaches encode the skeleton sequences as entangled spatiotemporal representations and confine the contrasts to the same level of representation. Instead, this paper introduces a novel contrastive learning framework, namely Spatiotemporal Clues Disentanglement Network (SCD-Net). Specifically, we integrate the decoupling module with a feature extractor to derive explicit clues from spatial and temporal domains respectively. As for the training of SCD-Net, with a constructed global anchor, we encourage the interaction between the anchor and extracted clues. Further, we propose a new masking strategy with structural constraints to strengthen the contextual associations, leveraging the latest development from masked image modelling into the proposed SCD-Net. We conduct extensive evaluations on the NTU-RGB+D (60&120) and PKU-MMD (I&II) datasets, covering various downstream tasks such as action recognition, action retrieval, transfer learning, and semi-supervised learning. The experimental results demonstrate the effectiveness of our method, which outperforms the existing state-of-the-art (SOTA) approaches significantly.
As well as having the ability to formulate models of the world capable of experimental falsification, it is evident that human cognitive capability embraces some degree of representational plasticity, having the scope (at least in infancy) to modify the primitives in terms of which the world is delineated. We hence employ the term 'cognitive bootstrapping' to refer to the autonomous updating of an embodied agent's perceptual framework in response to the perceived requirements of the environment in such a way as to retain the ability to refine the environment model in a consistent fashion across perceptual changes.We will thus argue that the concept of cognitive bootstrapping is epistemically ill-founded unless there exists an a priori percept/motor interrelation capable of maintaining an empirical distinction between the various possibilities of perceptual categorization and the inherent uncertainties of environment modeling.As an instantiation of this idea, we shall specify a very general, logically-inductive model of perception-action learning capable of compact re-parameterization of the percept space. In consequence of the a priori percept/action coupling, the novel perceptual state transitions so generated always exist in bijective correlation with a set of novel action states, giving rise to the required empirical validation criterion for perceptual inferences. Environmental description is correspondingly accomplished in terms of progressively higher-level affordance conjectures which are likewise validated by exploratory action.Application of this mechanism within simulated perception-action environments indicates that, as well as significantly reducing the size and specificity of the a priori perceptual parameter-space, the method can significantly reduce the number of iterations required for accurate convergence of the world-model. It does so by virtue of the active learning characteristics implicit in the notion of cognitive bootstrapping.
Face recognition (FR) using deep convolutional neural networks (DCNNs) has seen remarkable success in recent years. One key ingredient of DCNN-based FR is the design of a loss function that ensures discrimination between various identities. The state-of-the-art (SOTA) solutions utilise normalised Softmax loss with additive and/or multiplicative margins. Despite being popular and effective, these losses are justified only intuitively with little theoretical explanations. In this work, we show that under the LogSumExp (LSE) approximation, the SOTA Softmax losses become equivalent to a proxy-triplet loss that focuses on nearest-neighbour negative proxies only. This motivates us to propose a variant of the proxy-triplet loss, entitled Nearest Proxies Triplet (NPT) loss, which unlike SOTA solutions, converges for a wider range of hyper-parameters and offers flexibility in proxy selection and thus outperforms SOTA techniques. We generalise many SOTA losses into a single framework and give theoretical justifications for the assertion that minimising the proposed loss ensures a minimum separability between all identities. We also show that the proposed loss has an implicit mechanism of hard-sample mining. We conduct extensive experiments using various DCNN architectures on a number of FR benchmarks to demonstrate the efficacy of the proposed scheme over SOTA methods.
Discriminative correlation filter (DCF) based tracking methods have achieved great success recently. However, the temporal learning scheme in the current paradigm is of a linear recursion form determined by a fixed learning rate which can not adaptively feedback appearance variations. In this paper, we propose a unified non-negative subspace representation constrained leaning scheme for DCF. The subspace is constructed by several templates with auxiliary memory mechanisms. Then the current template is projected onto the subspace to find the non-negative representation and to determine the corresponding template weights. Our learning scheme enables efficient combination of correlation filter and subspace structure. The experimental results on OTB50 demonstrate the effectiveness of our learning formulation.
Welcome to the 24th International Conference on Pattern Recognition (ICPR 2018) in Beijing, China! ICPR 2018 is sponsored by the International Association for Pattern Recognition (IAPR), hosted by the Institute of Automation of Chinese Academy of Sciences, and supported by the Chinese Association of Automation.
Modern facial age estimation systems can achieve high accuracy when training and test datasets are identically distributed and captured under similar conditions. However, domain shifts in data, encountered in practice, lead to a sharp drop in accuracy of most existing age estimation algorithms. In this work, we propose a novel method, namely RAgE, to improve the robustness and reduce the uncertainty of age estimates by leveraging unlabelled data through a subject anchoring strategy and a novel consistency regularisation term. First, we propose an similarity-preserving pseudo-labelling algorithm by which the model generates pseudo-labels for a cohort of unlabelled images belonging to the same subject, while taking into account the similarity among age labels. In order to improve the robustness of the system, a consistency regularisation term is then used to simultaneously encourage the model to produce invariant outputs for the images in the cohort with respect to an anchor image. We propose a novel consistency regularisation term the noise-tolerant property of which effectively mitigates the so-called confirmation bias caused by incorrect pseudo-labels. Experiments on multiple benchmark ageing datasets demonstrate substantial improvements over the state-of-the-art methods and robustness to confounding external factors, including subject's head pose, illumination variation and appearance of expression in the face image.
Visual object tracking has witnessed continuous improvements in performance, thanks to deep CNN learning that recently emerged. More complex CNN models invariably offer better accuracy. However, there is a conflict between the tracking efficiency and model complexity, which poses a challenge in balancing speed against accuracy. To optimize the trade-off between these two performance criteria, a distillation-ensemble-selection framework is proposed in this paper. Without any modification to the baseline network architecture, the proposed approach enables the construction of a Siamese-based tracker with improved capacity and efficiency. Specifically, multiple student trackers are designed by means of knowledge distillation from a given teacher tracking model. To manage the varying granularity of unknown targets, an ensemble module combines the outputs of the student trackers with the help of a learnable fine-grained attention module. Besides, in the online tracking stage, a selection module adaptively controls the complexity of the tracker by identifying an appropriate subset of the candidate tracker models. We verify the effectiveness of the proposed method in both anchor-based and anchor-free paradigms. The experimental results obtained on standard benchmarking datasets demonstrate the effectiveness of the proposed method, with an outstanding and balanced performance in both accuracy and speed.
The Visual Object Tracking challenge VOT2020 is the eighth annual tracker benchmarking activity organized by the VOT initiative. Results of 58 trackers are presented; many are state-of-the-art trackers published at major computer vision conferences or in journals in the recent years. The VOT2020 challenge was composed of five sub-challenges focusing on different tracking domains: (i) VOT-ST2020 challenge focused on short-term tracking in RGB, (ii) VOT-RT2020 challenge focused on “real-time” short-term tracking in RGB, (iii) VOT-LT2020 focused on long-term tracking namely coping with target disappearance and reappearance, (iv) VOT-RGBT2020 challenge focused on short-term tracking in RGB and thermal imagery and (v) VOT-RGBD2020 challenge focused on long-term tracking in RGB and depth imagery. Only the VOT-ST2020 datasets were refreshed. A significant novelty is introduction of a new VOT short-term tracking evaluation methodology, and introduction of segmentation ground truth in the VOT-ST2020 challenge – bounding boxes will no longer be used in the VOT-ST challenges. A new VOT Python toolkit that implements all these novelites was introduced. Performance of the tested trackers typically by far exceeds standard baselines. The source code for most of the trackers is publicly available from the VOT page. The dataset, the evaluation kit and the results are publicly available at the challenge website (http://votchallenge.net).
Classical person re-identification approaches assume that a person of interest has appeared across different cameras and can be queried by one of the existing images. However, in real-world surveillance scenarios, frequently no visual information will be available about the queried person. In such scenarios, a natural language description of the person by a witness will provide the only source of information for retrieval. In this work, person re-identification using both vision and language information is addressed under all possible gallery and query scenarios. A two stream deep convolutional neural network framework supervised by identity based cross entropy loss is presented. Canonical Correlation Analysis is performed to enhance the correlation between the two modalities in a joint latent embedding space. To investigate the benefits of the proposed approach, a new testing protocol under a multi modal ReID setting is proposed for the test split of the CUHK-PEDES and CUHK-SYSU benchmarks. The experimental results verify that the learnt visual representations are more robust and perform 20% better during retrieval as compared to a single modality system.
The Visual Object Tracking challenge VOT2022 is the tenth annual tracker benchmarking activity organized by the VOT initiative. Results of 93 entries are presented; many are state-of-the-art trackers published at major computer vision conferences or in journals in recent years. The VOT2022 challenge was composed of seven sub-challenges focusing on different tracking domains: (i) VOT-STs2022 challenge focused on short-term tracking in RGB by segmentation, (ii) VOT-STb2022 challenge focused on short-term tracking in RGB by bounding boxes, (iii) VOT-RTs2022 challenge focused on “real-time” short-term tracking in RGB by segmentation, (iv) VOT-RTb2022 challenge focused on “real-time” short-term tracking in RGB by bounding boxes, (v) VOT-LT2022 focused on long-term tracking, namely coping with target disappearance and reappearance, (vi) VOT-RGBD2022 challenge focused on short-term tracking in RGB and depth imagery, and (vii) VOT-D2022 challenge focused on short-term tracking in depth-only imagery. New datasets were introduced in VOT-LT2022 and VOT-RGBD2022, VOT-ST2022 dataset was refreshed, and a training dataset was introduced for VOT-LT2022. The source code for most of the trackers, the datasets, the evaluation kit and the results are publicly available at the challenge website (http://votchallenge.net).
Recently, impressively growing efforts have been devoted to the challenging task of facial age estimation. The improvements in performance achieved by new algorithms are measured on several benchmarking test databases with different characteristics to check on consistency. While this is a valuable methodology in itself, a significant issue in the most age estimation related studies is that the reported results lack an assessment of intrinsic system uncertainty. Hence, a more in-depth view is required to examine the robustness of age estimation systems in different scenarios. The purpose of this paper is to conduct an evaluative and comparative analysis of different age estimation systems to identify trends, as well as the points of their critical vulnerability. In particular, we investigate four age estimation systems, including the online Microsoft service, two best state-of-the-art approaches advocated in the literature, as well as a novel age estimation algorithm. We analyse the effect of different internal and external factors, including gender, ethnicity, expression, makeup, illumination conditions, quality and resolution of the face images, on the performance of these age estimation systems. The goal of this sensitivity analysis is to provide the biometrics community with the insight and understanding of the critical subject-, camera- and environmental-based factors that affect the overall performance of the age estimation system under study.
The methods of symmetric positive definite (SPD) matrix learning have attracted considerable attention in many pattern recognition tasks, as they are eligible to capture and learn appropriate statistical features while respecting the Riemannian geometry of SPD manifold where the data reside on. Accompanied with the advanced deep learning techniques, several Riemannian networks (RiemNets) for SPD matrix nonlinear processing have recently been studied. However, it is pertinent to ask, whether greater accuracy gains can be realized by simply increasing the depth of RiemNets. The answer appears to be negative, as deeper RiemNets may be difficult to train. To explore a possible solution to this issue, we propose a new architecture for SPD matrix learning. Specifically, to enrich the deep representations, we build a stacked Riemannian autoencoder (SRAE) on the tail of the backbone network, i.e., SPDNet [23]. With this design, the associated reconstruction error term can prompt the embedding functions of both SRAE and of each RAE to approach an identity mapping, which helps to prevent the degradation of statistical information. Then, we implant several residual-like blocks using shortcut connections to augment the representational capacity of SRAE, and to simplify the training of a deeper network. The experimental evidence demonstrates that our DreamNet can achieve improved accuracy with increased depth.
Thanks to the efficacy of Symmetric Positive Definite (SPD) manifold in characterizing video sequences (image sets), image set-based visual classification has made remarkable progress. However, the issue of large intra-class diversity and inter-class similarity is still an open challenge for the research community. Although several recent studies have alleviated the above issue by constructing Riemannian neural networks for SPD matrix nonlinear processing, the degradation of structural information during multi-stage feature transformation impedes them from going deeper. Besides, a single cross-entropy loss is insufficient for discriminative learning as it neglects the peculiarities of data distribution. To this end, this paper develops a novel framework for image set classification. Specifically, we first choose a mainstream neural network built on the SPD manifold (SPDNet) [25] as the backbone with a stacked SPD manifold autoencoder (SSMAE) built on the tail to enrich the structured representations. Due to the associated reconstruction error terms, the embedding mechanism of both SSMAE and each SPD manifold autoencoder (SMAE) forms an approximate identity mapping, simplifying the training of the suggested deeper network. Then, the ReCov layer is introduced with a nonlinear function for the constructed architecture to narrow the discrepancy of the intra-class distributions from the perspective of regularizing the local statistical information of the SPD data. Afterward, two progressive metric learning stages are coupled with the proposed SSMAE to explicitly capture, encode, and analyze the geometric distributions of the generated deep representations during training. In consequence, not only a more powerful Riemannian network embedding but also effective classifiers can be obtained. Finally, a simple maximum voting strategy is applied to the outputs of the learned multiple classifiers for classification. The proposed model is evaluated on three typical visual classification tasks using widely adopted benchmarking datasets. Extensive experiments show its superiority over the state of the arts.
The fields of computer vision and image processing are constantly evolving as new research and applications in these areas emerge. Staying abreast of the most up-to-date developments in this field is necessary in order to promote further research and apply these developments in real-world settings. Computer Vision: Concepts, Methodologies, Tools, and Applications is an innovative reference source for the latest academic material on development of computers for gaining understanding about videos and digital images. Highlighting a range of topics, such as computational models, machine learning, and image processing, this multi-volume book is ideally designed for academicians, technology professionals, students, and researchers interested in uncovering the latest innovations in the field. The fields of computer vision and image processing are constantly evolving as new research and applications in these areas emerge. Staying abreast of the most up-to-date developments in this field is necessary in order to promote further research and apply these developments in real-world settings. Computer Vision: Concepts, Methodologies, Tools, and Applications is an innovative reference source for the latest academic material on development of computers for gaining understanding about videos and digital images. Highlighting a range of topics, such as computational models, machine learning, and image processing, this multi-volume book is ideally designed for academicians, technology professionals, students, and researchers interested in uncovering the latest innovations in the field.
Tennis game annotation using broadcast video is a task with a wide range of applications. In particular, ball trajectories carry rich semantic information for the annotation. However, tracking a ball in broadcast tennis video is extremely challenging. In this chapter, we explicitly address the challenges, and propose a layered data association algorithm for tracking multiple tennis balls fully automatically. The effectiveness of the proposed algorithm is demonstrated on two data sets with more than 100 sequences from real-world tennis videos, where other data association methods perform poorly or fail completely.
Modern face recognition systems extract face representations using deep neural networks (DNNs) and give excellent identification and verification results, when tested on high resolution (HR) images. However, the performance of such an algorithm degrades significantly for low resolution (LR) images. A straight forward solution could be to train a DNN, using simultaneously, high and low resolution face images. This approach yields a definite improvement at lower resolutions but suffers a performance degradation for high resolution images. To overcome this shortcoming, we propose to train a network using both HR and LR images under the guidance of a fixed network, pretrained on HR face images. The guidance is provided by minimising the KL-divergence between the output Softmax probabilities of the pretrained (i.e., Teacher) and trainable (i.e., Student) network as well as by sharing the Softmax weights between the two networks. The resulting solution is tested on down-sampled images from FaceScrub and MegaFace datasets and shows a consistent performance improvement across various resolutions. We also tested our proposed solution on standard LR benchmarks such as TinyFace and SCFace. Our algorithm consistently outperforms the state-of-the-art methods on these datasets, confirming the effectiveness and merits of the proposed method.
In recent years, facial landmark detection – also known as face alignment or facial landmark localisation – has become a very active area, due to its importance to a variety of image and video-based face analysis systems, such as face recognition, emotion analysis, human-computer interaction and 3D face reconstruction. This article looks at the challenges and latest technology advances in facial landmarks.
We set out to address, in the form of a survey, the fundamental constraints upon self-updating representation in cognitive agents of natural and artificial origin. The foundational epistemic problem encountered by such agents is that of distinguishing errors of representation from inappropriateness of the representational framework. Resolving this conceptual difficulty involves ensuring the empirical falsifiability of both the representational hypotheses and the entities so represented, while at the same time retaining their epistemic distinguishability. We shall thus argue that perception-action frameworks provide an appropriate basis for the development of an empirically meaningful criterion for validating perceptual categories. In this scenario, hypotheses about the agent’s world are defined in terms of environmental affordances (characterised in terms of the agent’s active capabilities). Agents with the capability to hierarchically-abstract this framework to a level consonant with performing syntactic manipulations and making deductive conjectures are consequently able to form an implicitly symbolic representation of the environment within which new, higher-level, modes of environment manipulation are implied (e.g. tool-use). This abstraction process is inherently open-ended, admitting a wide-range of possible representational hypotheses — only the form of the lowest-level of the hierarchy need be constrained a priori (being the minimally sufficient condition necessary for retention of the ability to falsify high-level hypotheses). In biological agents capable of autonomous cognitive-updating, we argue that the grounding of such a priori ‘bootstrap’ representational hypotheses is ensured via the process of natural selection.
Sensory information acquired by pattern recognition systems is invariably subject to environmental and sensing conditions, which may change over time. This may have a significant negative impact on the performance of pattern recognition algorithms. In the past, these problems have been tackled by building in invariance to the various changes, by adaptation and by multiple expert systems. More recently, the possibility of enhancing the pattern classification system robustness by using auxiliary information has been explored. In particular, by measuring the extent of degradation, the resulting sensory data quality information can be used with advantage to combat the effect of the degradation phenomena. This can be achieved by using the auxiliary quality information as features in the fusion stage of a multiple classifier system which uses the discriminant function values from the first stage as inputs. Data quality can be measured directly from the sensory data. Different architectures have been suggested for decision making using quality information. Examples of these architectures are presented and their relative merits discussed. The problems and benefits associated with the use of auxiliary information in sensory data analysis are illustrated on the problem of personal identity verification in biometrics. © Springer-Verlag Berlin Heidelberg 2010.
The fusion of one-class classifiers (OCCs) has been shown to exhibit promising performance in a variety of machine learning applications. The ability to assess the similarity or correlation between the output of various OCCs is an important prerequisite for building of a meaningful OCCs ensemble. However, this aspect of the OCC fusion problem has been mostly ignored so far. In this paper, we propose a new method of constructing a fusion of OCCs with three contributions: (a) As a key contribution, enabling an OCC ensemble design using exclusively non anomalous samples, we propose a novel fitness function to evaluate the competency of OCCs without requiring samples from the anomalous class; (b) As a minor, but impactful contribution, we investigate alternative forms of score normalisation of OCCs, and identify a novel two-sided normalisation method as the best in coping with long tail non anomalous data distributions; (c) In the context of building our proposed OCC fusion system based on the weighted averaging approach, we find that the weights optimised using a particle swarm optimisation algorithm produce the most effective solution. We evaluate the merits of the proposed method on 15 benchmarking datasets from different application domains including medical, anti-spam and face spoofing detection. The comparison of the proposed approach with state-of-the-art methods alongside the statistical analysis confirm the effectiveness of the proposed model. (c) 2021 Elsevier Ltd. All rights reserved.
We consider a framework for taking into consideration the relative importance (ordinality) of object labels in the process of learning a label predictor function. The commonly used loss functions are not well matched to this problem, as they exhibit deficiencies in capturing natural correlations of the labels and the corresponding data. We propose to incorporate such correlations into our learning algorithm using an optimal transport formulation. Our approach is to learn the ground metric, which is partly involved in forming the optimal transport distance, by leveraging ordinality as a general form of side information in its formulation. Based on this idea, we then develop a novel loss function for training deep neural networks. A highly efficient alternating learning method is then devised to alternatively optimise the ground metric and the deep model in an end-to-end learning manner. This scheme allows us to adaptively adjust the shape of the ground metric, and consequently the shape of the loss function for each application. We back up our approach by theoretical analysis and verify the performance of our proposed scheme by applying it to two learning tasks, i.e. chronological age estimation from the face and image aesthetic assessment. The numerical results on several benchmark datasets demonstrate the superiority of the proposed algorithm.
Existing facial age estimation studies have mostly focused on intra-database protocols that assume training and test images are captured under similar conditions. This is rarely valid in practical applications, where we typically encounter training and test sets with different characteristics. In this article, we deal with such situations, namely subjective-exclusive cross-database age estimation. We formulate the age estimation problem as the distribution learning framework, where the age labels are encoded as a probability distribution. To improve the cross-database age estimation performance, we propose a new loss function which provides a more robust measure of the difference between ground-truth and predicted distributions. The desirable properties of the proposed loss function are theoretically analysed and compared with the state-of-the-art approaches. In addition, we compile a new balanced large-scale age estimation database. Last, we introduce a novel evaluation protocol, called subject-exclusive cross-database age estimation protocol, which provides meaningful information of a method in terms of the generalisation capability. The experimental results demonstrate that the proposed approach outperforms the state-of-the-art age estimation methods under both intra-database and subject-exclusive cross-database evaluation protocols. In addition, in this article, we provide a comparative sensitivity analysis of various algorithms to identify trends and issues inherent to their performance. This analysis introduces some open problems to the community which might be considered when designing a robust age estimation system.
The Symmetric Positive Definite (SPD) matrices have received wide attention for data representation in many scientific areas. Although there are many different attempts to develop effective deep architectures for data processing on the Riemannian manifold of SPD matrices, very few solutions explicitly mine the local geometrical information in deep SPD feature representations. Given the great success of local mechanisms in Euclidean methods, we argue that it is of utmost importance to ensure the preservation of local geometric information in the SPD networks. We first analyse the convolution operator commonly used for capturing local information in Euclidean deep networks from the perspective of a higher level of abstraction afforded by category theory. Based on this analysis, we define the local information in the SPD manifold and design a multi-scale submanifold block for mining local geometry. Experiments involving multiple visual tasks validate the effectiveness of our approach. The supplement and source code can be found in https://github.com/GitZH-Chen/MSNet.git.
Light Field Microscopy (LFM) is an imaging technique that offers the opportunity to study fast dynamics in biological systems due to its 3D imaging speed and is particularly attractive for functional neuroimaging. Traditional model-based approaches employed in microscopy for reconstructing 3D images from light-field data are affected by reconstruction artifacts and are computationally demanding. This work introduces a deep neural network for LFM to image neuronal activity under adverse conditions: limited training data, background noise, and scattering mammalian brain tissue. The architecture of the network is obtained by unfolding the ISTA algorithm and is based on the observation that neurons in the tissue are sparse. Our approach is also based on a novel modelling of the imaging system that uses a linear convolutional neural network to fit the physics of the acquisition process. We train the network in a semi-supervised manner based on an adversarial training framework. The small labelled dataset required for training is acquired from a single sample via two-photon microscopy, a point-scanning 3D imaging technique that achieves high spatial resolution and deep tissue penetration but at a lower speed than LFM. We introduce physics knowledge of the system in the design of the network architecture and during training to complete our semi-supervised approach. We experimentally show that in the proposed scenario, our method performs better than typical deep learning and model-based reconstruction strategies for imaging neuronal activity in mammalian brain tissue via LFM, considering reconstruction quality, generalization to functional imaging, and reconstruction speed.
Good generalization performance across a wide variety of domains caused by many external and internal factors is the fundamental goal of any machine learning algorithm. This paper theoretically proves that the choice of loss function matters for improving the generalization performance of deep learning-based systems. By deriving the generalization error bound for deep neural models trained by stochastic gradient descent, we pinpoint the characteristics of the loss function that is linked to the generalization error, and can therefore be used for guiding the loss function selection process. In summary, our main statement in this paper is: choose a stable loss function, generalize better. Focusing on human age estimation from the face which is a challenging topic in computer vision, we then propose a novel loss function for this learning problem. We theoretically prove that the proposed loss function achieves stronger stability, and consequently a tighter generalization error bound, compared to the other common loss functions for this problem. We have supported our findings theoretically, and demonstrated the merits of the guidance process experimentally, achieving significant improvements.
This paper investigates the evaluation of dense 3D face reconstruction from a single 2D image in the wild. To this end, we organise a competition that provides a new benchmark dataset that contains 2000 2D facial images of 135 subjects as well as their 3D ground truth face scans. In contrast to previous competitions or challenges, the aim of this new benchmark dataset is to evaluate the accuracy of a 3D dense face reconstruction algorithm using real, accurate and high-resolution 3D ground truth face scans. In addition to the dataset, we provide a standard protocol as well as a Python script for the evaluation. Last, we report the results obtained by three state-of-the-art 3D face reconstruction systems on the new benchmark dataset. The competition is organised along with the 2018 13th IEEE Conference on Automatic Face & Gesture Recognition.
We address the problem of anomaly detection in machine perception. The concept of domain anomaly is introduced as distinct from the conventional notion of anomaly used in the literature. We propose a unified framework for anomaly detection which exposes the multifaceted nature of anomalies and suggest effective mechanisms for identifying and distinguishing each facet as instruments for domain anomaly detection. The framework draws on the Bayesian probabilistic reasoning apparatus which clearly defines concepts such as outlier, noise, distribution drift, novelty detection (object, object primitive), rare events, and unexpected events. Based on these concepts we provide a taxonomy of domain anomaly events. One of the mechanisms helping to pinpoint the nature of anomaly is based on detecting incongruence between contextual and noncontextual sensor(y) data interpretation. The proposed methodology has wide applicability. It underpins in a unified way the anomaly detection applications found in the literature. To illustrate some of its distinguishing features, in here the domain anomaly detection methodology is applied to the problem of anomaly detection for a video annotation system.
Discriminative least squares regression (DLSR) has been shown to achieve promising performance in multi-class image classification tasks. Its key idea is to force the regression labels of different classes to move in opposite directions by means of the ε-dragging technique, yielding discriminative regression model exhibiting wider margins. However, the ε-dragging technique ignores an important problem: its relaxation matrix is dynamically updated in optimization, which means the dragging values can also cause the labels from the same class to be uncorrelated. In order to learn a more powerful projection, as well as regression labels, we propose a Fisher regularized ε-dragging framework (Fisher-ε) for image classification by constraining the relaxed labels using the Fisher criterion. On one hand, the Fisher criterion improves the intra-class compactness of the relaxed labels during relaxation learning. On the other hand, it is expected further to enhance the inter-class separability of ε-dragging. Fisher-ε for the first time ever attempts to integrate the Fisher criterion and ε-dragging technique into a unified model because they are complementary in learning discriminative projection. Extensive experiments on various datasets demonstrate that the proposed Fisher-ε method achieves performance that is superior to other state-of-the-art classification methods. The Matlab codes are available at https://github.com/chenzhe207/Fisher-epsilon.
Due to the explosive growth of multimedia data in recent years, cross-media hashing (CMH) approaches have recently received increasing attention. To learn the hash codes, most existing supervised CMH algorithms employ the strict binary label information, which has small margins between the incorrect labels (0) and the true labels (1), increasing the risk of classification error. Besides, most existing CMH approaches are one-stage algorithms, in which the hash functions and binary codes can be learned simultaneously, complicating the optimization. To avoid NP-hard optimization, many approaches utilize a relaxation strategy. However, this optimisation trick may cause large quantization errors. To address this, we present a novel tWo-stAge discreTe Cross-media Hashing method based on smooth matrix factorization and label relaxation, named WATCH. The proposed WATCH controls the margins adaptively by the novel label relaxation strategy. This innovation reduces the quantization error significantly. Besides, WATCH is a two-stage model. In stage 1, we employ a discrete smooth matrix factorization model. Then, the hash codes can be generated discretely, reducing the large quantization loss greatly. In stage 2, we adopt an effective hash function learning strategy, which produces more effective hash functions. Comprehensive experiments on several datasets demonstrate that WATCH outperforms some state-of-the-art methods.
Recently, deep learning has become the mainstream methodology for Compound-Protein Interaction (CPI) prediction. However, the existing compound-protein feature extraction methods have some issues that limit their performance. First, graph networks are widely used for structural compound feature extraction, but the chemical properties of a compound depend on functional groups rather than graphic structure. Besides, the existing methods lack capabilities in extracting rich and discriminative protein features. Last, the compound-protein features are usually simply combined for CPI prediction, without considering information redundancy and effective feature mining. To address the above issues, we propose a novel CPInformer method. Specifically, we extract heterogeneous compound features, including structural graph features and functional class fingerprints, to reduce prediction errors caused by similar structural compounds. Then, we combine local and global features using dense connections to obtain multi-scale protein features. Last, we apply ProbSparse self-attention to protein features, under the guidance of compound features, to eliminate information redundancy, and to improve the accuracy of CPInformer. More importantly, the proposed method identifies the activated local regions that link a CPI, providing a good visualisation for the CPI state. The results obtained on five benchmarks demonstrate the merits and superiority of CPInformer over the state-of-the-art approaches.
The proliferative activity of breast tumors, which is routinely estimated by counting of mitotic figures in hematoxylin and eosin stained histology sections, is considered to be one of the most important prognostic markers. However, mitosis counting is laborious, subjective and may suffer from low inter-observer agreement. With the wider acceptance of whole slide images in pathology labs, automatic image analysis has been proposed as a potential solution for these issues. In this paper, the results from the Assessment of Mitosis Detection Algorithms 2013 (AMIDA13) challenge are described. The challenge was based on a data set consisting of 12 training and 11 testing subjects, with more than one thousand annotated mitotic figures by multiple observers. Short descriptions and results from the evaluation of eleven methods are presented. The top performing method has an error rate that is comparable to the inter-observer agreement among pathologists.
With efficient appearance learning models, Discriminative Correlation Filter (DCF) has been proven to be very successful in recent video object tracking benchmarks and competitions. However, the existing DCF paradigm suffers from two major issues, i.e., spatial boundary effect and temporal filter degradation. To mitigate these challenges, we propose a new DCF-based tracking method. The key innovations of the proposed method include adaptive spatial feature selection and temporal consistent constraints, with which the new tracker enables joint spatial-temporal filter learning in a lower dimensional discriminative manifold. More specifically, we apply structured spatial sparsity constraints to multi-channel filers. Consequently, the process of learning spatial filters can be approximated by the lasso regularisation. To encourage temporal consistency, the filter model is restricted to lie around its historical value and updated locally to preserve the global structure in the manifold. Last, a unified optimisation framework is proposed to jointly select temporal consistency preserving spatial features and learn discriminative filters with the augmented Lagrangian method. Qualitative and quantitative evaluations have been conducted on a number of well-known benchmarking datasets such as OTB2013, OTB50, OTB100, Temple-Colour, UAV123 and VOT2018. The experimental results demonstrate the superiority of the proposed method over the state-of-the-art approaches.
In this letter, we present a random cascaded-regression copse (R-CR-C) for robust facial landmark detection. Its key innovations include a new parallel cascade structure design, and an adaptive scheme for scale-invariant shape update and local feature extraction. Evaluation on two challenging benchmarks shows the superiority of the proposed algorithm to state-of-the-art methods. © 1994-2012 IEEE.
Efficient and robust facial landmark localisation is crucial for the deployment of real-time face analysis systems. This paper presents a new loss function, namely Rectified Wing (RWing) loss, for regression-based facial landmark localisation with Convolutional Neural Networks (CNNs). We first systemically analyse different loss functions, including L2, L1 and smooth L1. The analysis suggests that the training of a network should pay more attention to small-medium errors. Motivated by this finding, we design a piece-wise loss that amplifies the impact of the samples with small-medium errors. Besides, we rectify the loss function for very small errors to mitigate the impact of inaccuracy of manual annotation. The use of our RWing loss boosts the performance significantly for regression-based CNNs in facial landmarking, especially for lightweight network architectures. To address the problem of under-representation of samples with large pose variations, we propose a simple but effective boosting strategy, referred to as pose-based data balancing. In particular, we deal with the data imbalance problem by duplicating the minority training samples and perturbing them by injecting random image rotation, bounding box translation and other data augmentation strategies. Last, the proposed approach is extended to create a coarse-to-fine framework for robust and efficient landmark localisation. Moreover, the proposed coarse-to-fine framework is able to deal with the small sample size problem effectively. The experimental results obtained on several well-known benchmarking datasets demonstrate the merits of our RWing loss and prove the superiority of the proposed method over the state-of-the-art approaches.
Visual semantic information comprises two important parts: the meaning of each visual semantic unit and the coherent visual semantic relation conveyed by these visual semantic units. Essentially, the former one is a visual perception task while the latter one corresponds to visual context reasoning. Remarkable advances in visual perception have been achieved due to the success of deep learning. In contrast, visual semantic information pursuit, a visual scene semantic interpretation task combining visual perception and visual context reasoning, is still in its early stage. It is the core task of many different computer vision applications, such as object detection, visual semantic segmentation, visual relationship detection or scene graph generation. Since it helps to enhance the accuracy and the consistency of the resulting interpretation, visual context reasoning is often incorporated with visual perception in current deep end-to-end visual semantic information pursuit methods. Surprisingly, a comprehensive review for this exciting area is still lacking. In this survey, we present a unified theoretical paradigm for all these methods, followed by an overview of the major developments and the future trends in each potential direction. The common benchmark datasets, the evaluation metrics and the comparisons of the corresponding methods are also introduced.
3D Morphable Face Models (3DMM) have been used in pattern recognition for some time now. They have been applied as a basis for 3D face recognition, as well as in an assistive role for 2D face recognition to perform geometric and photometric normalisation of the input image, or in 2D face recognition system training. The statistical distribution underlying 3DMM is Gaussian. However, the single-Gaussian model seems at odds with reality when we consider different cohorts of data, e.g. Black and Chinese faces. Their means are clearly different. This paper introduces the Gaussian Mixture 3DMM (GM-3DMM) which models the global population as a mixture of Gaussian subpopulations, each with its own mean. The proposed GM-3DMM extends the traditional 3DMM naturally, by adopting a shared covariance structure to mitigate small sample estimation problems associated with data in high dimensional spaces. We construct a GM-3DMM, the training of which involves a multiple cohort dataset, SURREY-JNU, comprising 942 3D face scans of people with mixed backgrounds. Experiments in fitting the GM-3DMM to 2D face images to facilitate their geometric and photometric normalisation for pose and illumination invariant face recognition demonstrate the merits of the proposed mixture of Gaussians 3D face model.
We present an algorithm that models the rate of change of biometric performance over time on a subject-dependent basis. It is called “homomorphic users grouping algorithm” or HUGA. Although the model is based on very simplistic assumptions that are inherent in linear regression, it has been applied successfully to estimate the performance of talking face and speech identity verification modalities, as well as their fusion, over a period of more than 600 days. Our experiments carried out on the MOBIO database show that subjects exhibit very different performance trends. While the performance of some users degrades over time, which is consistent with the literature, we also found that for a similar proportion of users, their performance actually improves with use. The latter finding has never been reported in the literature. Hence, our findings suggest that the problem of biometric performance degradation may be not as serious as previously thought, and so far, the community has ignored the possibility of improved biometric performance over time. The findings also suggest that adaptive biometric systems, that is, systems that attempt to update biometric templates, should be subject-dependent.
Strict ‘0-1’ block-diagonal structure has been widely used for learning structured representation in face recognition problems. However, it is questionable and unreasonable to assume the within-class representations are the same. To circumvent this problem, in this paper, we propose a slack block-diagonal (SBD) structure for representation where the target structure matrix is dynamically updated, yet its blockdiagonal nature is preserved. Furthermore, in order to depict the noise in face images more precisely, we propose a robust dictionary learning algorithm based on mixed-noise model by utilizing the above SBD structure (SBD2L). SBD2L considers that there exists two forms of noise in data which are drawn from Laplacian and Gaussion distribution, respectively. Moreover, SBD2L introduces a low-rank constraint on the representation matrix to enhance the dictionary’s robustness to noise. Extensive experiments on four benchmark databases show that the proposed SBD2L can achieve better classification results than several state-of-the-art dictionary learning methods.
Face recognition has been the focus of attention for the past couple of decades and, as a result, a significant progress has been made in this area. However, the problem of spoofing attacks can challenge face biometric systems in practical applications. In this paper, an effective countermeasure against face spoofing attacks based on a kernel discriminant analysis approach is presented. Its success derives from different innovations. First, it is shown that the recently proposed multiscale dynamic texture descriptor based on binarized statis- tical image features on three orthogonal planes (MBSIF-TOP) is effective in detecting spoofing attacks, showing promising perfor- mance compared with existing alternatives. Next, by combining MBSIF-TOP with a blur-tolerant descriptor, namely, the dynamic multiscale local phase quantization (MLPQ-TOP) representation, the robustness of the spoofing attack detector can be further improved. The fusion of the information provided by MBSIF-TOP and MLPQ-TOP is realized via a kernel fusion approach based on a fast kernel discriminant analysis (KDA) technique. It avoids the costly eigen-analysis computations by solving the KDA problem via spectral regression. The experimental evaluation of the proposed system on different databases demonstrates its advantages in detecting spoofing attacks in various imaging conditions, compared with the existing methods.
The paper presents a dictionary integration algorithm using 3D morphable face models (3DMM) for poseinvariant collaborative-representation-based face classification. To this end, we first fit a 3DMM to the 2D face images of a dictionary to reconstruct the 3D shape and texture of each image. The 3D faces are used to render a number of virtual 2D face images with arbitrary pose variations to augment the training data, by merging the original and rendered virtual samples to create an extended dictionary. Second, to reduce the information redundancy of the extended dictionary and improve the sparsity of reconstruction coefficient vectors using collaborative-representation-based classification (CRC), we exploit an on-line class elimination scheme to optimise the extended dictionary by identifying the training samples of the most representative classes for a given query. The final goal is to perform pose-invariant face classification using the proposed dictionary integration method and the on-line pruning strategy under the CRC framework. Experimental results obtained for a set of well-known face datasets demonstrate the merits of the proposed method, especially its robustness to pose variations.
To learn disentangled representations of facial images, we present a Dual Encoder-Decoder based Generative Adversarial Network (DED-GAN). In the proposed method, both the generator and discriminator are designed with deep encoder-decoder architectures as their backbones. To be more specific, the encoder-decoder structured generator is used to learn a pose disentangled face representation, and the encoder-decoder structured discriminator is tasked to perform real/fake classification, face reconstruction, determining identity and estimating face pose. We further improve the proposed network architecture by minimizing the additional pixel-wise loss defined by the Wasserstein distance at the output of the discriminator so that the adversarial framework can be better trained. Additionally, we consider face pose variation to be continuous, rather than discrete in existing literature, to inject richer pose information into our model. The pose estimation task is formulated as a regression problem, which helps to disentangle identity information from pose variations. The proposed network is evaluated on the tasks of pose-invariant face recognition (PIFR) and face synthesis across poses. An extensive quantitative and qualitative evaluation carried out on several controlled and in-the-wild benchmarking datasets demonstrates the superiority of the proposed DED-GAN method over the state-of-the-art approaches.
In this letter, we formulate sparse subspace clustering as a smoothed ℓp (0 ˂ p ˂ 1) minimization problem (SSC-SLp) and present a unified formulation for different practical clustering problems by introducing a new pseudo norm. Generally, the use of ℓp (0 ˂ p ˂ 1) norm approximating the ℓ0 one can lead to a more effective approximation than the ℓp norm, while the ℓp-regularization also causes the objective function to be non-convex and non-smooth. Besides, better adapting to the property of data representing real problems, the objective function is usually constrained by multiple factors (such as spatial distribution of data and errors). In view of this, we propose a computationally efficient method for solving the multi-constrained non-smooth ℓp minimization problem, which smooths the ℓp norm and minimizes the objective function by alternately updating a block (or a variable) and its weight. In addition, the convergence of the proposed algorithm is theoretically proven. Extensive experimental results on real datasets demonstrate the effectiveness of the proposed method.
Automation of HEp-2 cell pattern classification would drastically improve the accuracy and throughput of diagnostic services for many auto-immune diseases, but it has proven difficult to reach a sufficient level of precision. Correct diagnosis relies on a subtle assessment of texture type in microscopic images of indirect immunofluorescence (IIF), which has, so far, eluded reliable replication through automated measurements. Following the recent HEp-2 Cells Classification contest held at ICPR 2012, we extend the scope of research in this field to develop a method of feature comparison that goes beyond the analysis of individual cells and majority-vote decisions to consider the full distribution of cell parameters within a patient sample. We demonstrate that this richer analysis is better able to predict the results of majority vote decisions than the cell-level performance analysed in all previous works. © 2013.
In the domain of video-based image set classification, a considerable advance has been made by modeling each video sequence as a linear subspace, which typically resides on a Grassmann manifold. Due to the large intra-class variations, how to establish appropriate set models to encode these variations of set data and how to effectively measure the dissimilarity between any two image sets are two open challenges. To seek a possible way to tackle these issues, this paper presents a graph embedding multi-kernel metric learning (GEMKML) algorithm for image set classification. The proposed GEMKML implements set modeling, feature extraction, and classification in two steps. Firstly, the proposed framework constructs a novel cascaded feature learning architecture on Grassmann manifold for the sake of producing more effective Grassmann manifold-valued feature representations. To make a better use of these learned features, a graph embedding multi-kernel metric learning scheme is then devised to map them into a lower-dimensional Euclidean space, where the inter-class distances are maximized and the intra-class distances are minimized. We evaluate the proposed GEMKML on four different video-based image set classification tasks using widely adopted datasets. The extensive classification results confirm its superiority over the state-of-the-art methods.
This guest editorial introduces the twenty two papers accepted for this Special Issue on Articulated Motion and Deformable Objects (AMDO). They are grouped into four main categories within the field of AMDO: human motion analysis (action/gesture), human pose estimation, deformable shape segmentation, and face analysis. For each of the four topics, a survey of the recent developments in the field is presented. The accepted papers are briefly introduced in the context of this survey. They contribute novel methods, algorithms with improved performance as measured on benchmarking datasets, as well as two new datasets for hand action detection and human posture analysis. The special issue should be of high relevance to the reader interested in AMDO recognition and promote future research directions in the field.
The problem of tracking multiple moving speakers in indoor environments has received much attention. Earlier techniques were based purely on a single modality, e.g., vision. Recently, the fusion of multi-modal information has been shown to be instrumental in improving tracking performance, as well as robustness in the case of challenging situations like occlusions (by the limited field of view of cameras or by other speakers). However, data fusion algorithms often suffer from noise corrupting the sensor measurements which cause non-negligible detection errors. Here, a novel approach to combining audio and visual data is proposed. We employ the direction of arrival angles of the audio sources to reshape the typical Gaussian noise distribution of particles in the propagation step and to weight the observation model in the measurement step. This approach is further improved by solving a typical problem associated with the PF, whose efficiency and accuracy usually depend on the number of particles and noise variance used in state estimation and particle propagation. Both parameters are specified beforehand and kept fixed in the regular PF implementation which makes the tracker unstable in practice. To address these problems, we design an algorithm which adapts both the number of particles and noise variance based on tracking error and the area occupied by the particles in the image. Experiments on the AV16.3 dataset show the advantage of our proposed methods over the baseline PF method and an existing adaptive PF algorithm for tracking occluded speakers with a significantly reduced number of particles.
Effective data augmentation is crucial for facial landmark localisation with Convolutional Neural Networks (CNNs). In this letter, we investigate different data augmentation techniques that can be used to generate sufficient data for training CNN-based facial landmark localisation systems. To the best of our knowledge, this is the first study that provides a systematic analysis of different data augmentation techniques in the area. In addition, an online Hard Augmented Example Mining (HAEM) strategy is advocated for further performance boosting. We examine the effectiveness of those techniques using a regression-based CNN architecture. The experimental results obtained on the AFLW and COFW datasets demonstrate the importance of data augmentation and the effectiveness of HAEM. The performance achieved using these techniques is superior to the state-of-the-art algorithms.
Lip region deformation during speech contains biometric information and is termed visual speech. This biometric information can be interpreted as being genetic or behavioral depending on whether static or dynamic features are extracted. In this paper, we use a texture descriptor called local ordinal contrast pattern (LOCP) with a dynamic texture representation called three orthogonal planes to represent both the appearance and dynamics features observed in visual speech. This feature representation, when used in standard speaker verification engines, is shown to improve the performance of the lip-biometric trait compared to the state-of-the-art. The best baseline state-of-the-art performance was a half total error rate (HTER) of 13.35% for the XM2VTS database. We obtained HTER of less than 1%. The resilience of the LOCP texture descriptor to random image noise is also investigated. Finally, the effect of the amount of video information on speaker verification performance suggests that with the proposed approach, speaker identity can be verified with a much shorter biometric trait record than the length normally required for voice-based biometrics. In summary, the performance obtained is remarkable and suggests that there is enough discriminative information in the mouth-region to enable its use as a primary biometric trait.
Appearance variations result in many difficulties in face image analysis. To deal with this challenge, we present a Unified Tensor-based Active Appearance Model (UT-AAM) for jointly modelling the geometry and texture information of 2D faces. For each type of face information, namely shape and texture, we construct a unified tensor model capturing all relevant appearance variations. This contrasts with the variation-specific models of the classical tensor AAM. To achieve the unification across pose variations, a strategy for dealing with self-occluded faces is proposed to obtain consistent shape and texture representations of pose-varied faces. In addition, our UT-AAM is capable of constructing the model from an incomplete training dataset, using tensor completion methods. Last, we use an effective cascaded-regression-based method for UT-AAM fitting. With these advancements, the utility of UT-AAM in practice is considerably enhanced. As an example, we demonstrate the improvements in training facial landmark detectors through the use of UT-AAM to synthesise a large number of virtual samples. Experimental results obtained on a number of well-known face datasets demonstrate the merits of the proposed approach.
In decision making systems involving multiple classifiers there is the need to assess classifier (in)congruence, that is to gauge the degree of agreement between their outputs. A commonly used measure for this purpose is the Kullback–Leibler (KL) divergence. We propose a variant of the KL divergence, named decision cognizant Kullback–Leibler divergence (DC-KL), to reduce the contribution of the minority classes, which obscure the true degree of classifier incongruence. We investigate the properties of the novel divergence measure analytically and by simulation studies. The proposed measure is demonstrated to be more robust to minority class clutter. Its sensitivity to estimation noise is also shown to be considerably lower than that of the classical KL divergence. These properties render the DC-KL divergence a much better statistic for discriminating between classifier congruence and incongruence in pattern recognition systems.
The kernel null-space technique is known to be an effective one-class classification (OCC) technique. Nevertheless, the applicability of this method is limited due to its susceptibility to possible training data corruption and the inability to rank training observations according to their conformity with the model. This article addresses these shortcomings by regularizing the solution of the null-space kernel Fisher methodology in the context of its regression-based formulation. In this respect, first, the effect of the Tikhonov regularization in the Hilbert space is analyzed, where the one-class learning problem in the presence of contamination in the training set is posed as a sensitivity analysis problem. Next, the effect of the sparsity of the solution is studied. For both alternative regularization schemes, iterative algorithms are proposed which recursively update label confidences. Through extensive experiments, the proposed methodology is found to enhance robustness against contamination in the training set compared with the baseline kernel null-space method, as well as other existing approaches in the OCC paradigm, while providing the functionality to rank training samples effectively
Recently, the correlation filters have been successfully applied to visual tracking, but the boundary effect severely restrains their tracking performance. In this paper, to overcome this problem, we propose a correlation tracking framework with implicitly extending search region (TESR) without introducing background noise. The proposed tracking method is a two- stage detection framework. To implicitly extend the search region of the correlation tracking, firstly we add other four search centers except for the original search center in an elegant manner, which is given by the target location in previous frame, so our TESR will totally generate five potential object locations based on these five search centers. Then, an SVM classifier is used to determine the correct target position. We also apply the salient object detection score to regularize the output of the SVM classifier to improve its performance. The experimental results demonstrate that TESR exhibits superior performance in comparison with the state-of-the-art trackers.
In recent years, discriminative correlation filter (DCF) based algorithms have significantly advanced the state of the art in visual object tracking. The key to the success of DCF is an efficient discriminative regression model trained with powerful multi-cue features, including both hand-crafted and deep neural network features. However, the tracking performance is hindered by their inability to respond adequately to abrupt target appearance variations. This issue is posed by the limited representation capability of fixed image features. In this work, we set out to rectify this shortcoming by proposing a complementary representation of a visual content. Specifically, we propose the use of a collaborative representation between successive frames to extract the dynamic appearance information from a target with rapid appearance changes, which results in suppressing the undesirable impact of the background. The resulting collaborative representation coefficients are combined with the original feature maps using a spatially regularised DCF framework for performance boosting. The experimental results on several benchmarking datasets demonstrate the effectiveness and robustness of the proposed method, as compared with a number of state-of-the-art tracking algorithms.
In this paper, we present SALIC, an active learning method for selecting the most appropriate user tagged images to expand the training set of a binary classifier. The process of active learning can be fully automated in this social context by replacing the human oracle with the images' tags. However, their noisy nature adds further complexity to the sample selection process since, apart from the images' informativeness (i.e., how much they are expected to inform the classifier if we knew their label), our confidence about their actual label should also be maximized (i.e., how certain the oracle is on the images' true contents). The main contribution of this work is in proposing a probabilistic approach for jointly maximizing the two aforementioned quantities. In the examined noisy context, the oracle's confidence is necessary to provide a contextual-based indication of the images' true contents, while the samples' informativeness is required to reduce the computational complexity and minimize the mistakes of the unreliable oracle. To prove this, first, we show that SALIC allows us to select training data as effectively as typical active learning, without the cost of manual annotation. Finally, we argue that the speed-up achieved when learning actively in this social context (where labels can be obtained without the cost of human annotation) is necessary to cope with the continuously growing requirements of large-scale applications. In this respect, we demonstrate that SALIC requires ten times less training data in order to reach the same performance as a straightforward informativeness-agnostic learning approach.
The grounding of high-level semantic concepts is a key requirement of video annotation systems. Rule induction can thus constitute an invaluable intermediate step in characterizing protocol-governed domains, such as broadcast sports footage. The authors propose a clause grammar template approach to the problem of rule induction in video footage of court games that employs a second-order meta-grammar for Markov Logic Network construction. The aim is to build an adaptive system for sports video annotation capable, in principle, of both learning ab initio and adaptively transferring learning between distinct rule domains. The authors tested the method using a simulated game predicate generator as well as real data derived from tennis footage via computer-vision-based approaches including HOG3D-based player-action classification, Hough-transform-based court detection, and graph-theoretic ball tracking. Experiments demonstrate that the method exhibits both error resilience and learning transfer in the court domain context. Moreover, the clause template approach naturally generalizes to any suitably constrained, protocol-governed video domain characterized by feature noise or detector error.
The importance of wild video based image set recognition is becoming monotonically increasing. However, the contents of these collected videos are often complicated, and how to efficiently perform set modeling and feature extraction is a big challenge in CV community. Recently, some proposed image set classification methods have made a considerable advance by modeling the original image set with covariance matrix, linear subspace, or Gaussian distribution. Moreover, the distinctive geometry spanned by them are three types of Riemannian manifolds. As a matter of fact, most of them just adopt a single geometric model to describe each set data, which may lose some information for classification. To tackle this, we propose a novel algorithm to model each image set from a multi-geometric perspective. Specifically, the covariance matrix, linear subspace, and Gaussian distribution are applied for set representation simultaneously. In order to fuse these multiple heterogeneous features, the well-equipped Riemannian kernel functions are first utilized to map them into high dimensional Hilbert spaces. Then, a multi-kernel metric learning framework is devised to embed the learned hybrid kernels into a lower dimensional common subspace for classification. We conduct experiments on four widely used datasets. Extensive experimental results justify its superiority over the state-of-the-art.
In existing audio-visual blind source separation (AV-BSS) algorithms, the AV coherence is usually established through statistical modelling, using e.g. Gaussian mixture models (GMMs). These methods often operate in a lowdimensional feature space, rendering an effective global representation of the data. The local information, which is important in capturing the temporal structure of the data, however, has not been explicitly exploited. In this paper, we propose a new method for capturing such local information, based on audio-visual dictionary learning (AVDL). We address several challenges associated with AVDL, including cross-modality differences in size, dimension and sampling rate, as well as the issues of scalability and computational complexity. Following a commonly employed bootstrap coding-learning process, we have developed a new AVDL algorithm which features, a bimodality balanced and scalable matching criterion, a size and dimension adaptive dictionary, a fast search index for efficient coding, and cross-modality diverse sparsity. We also show how the proposed AVDL can be incorporated into a BSS algorithm. As an example, we consider binaural mixtures, mimicking aspects of human binaural hearing, and derive a new noise-robust AV-BSS algorithm by combining the proposed AVDL algorithm with Mandel’s BSS method, which is a state-of-the-art audio-domain method using time-frequency masking. We have systematically evaluated the proposed AVDL and AV-BSS algorithms, and show their advantages over the corresponding baseline methods, using both synthetic data and visual speech data from the multimodal LILiR Twotalk corpus.
Sparsity-inducing multiple kernel Fisher discriminant analysis (MK-FDA) has been studied in the literature. Building on recent advances in non-sparse multiple kernel learning (MKL), we propose a non-sparse version of MK-FDA, which imposes a general `p norm regularisation on the kernel weights. We formulate the associated optimisation problem as a semi-infinite program (SIP), and adapt an iterative wrapper algorithm to solve it. We then discuss, in light of latest advances inMKL optimisation techniques, several reformulations and optimisation strategies that can potentially lead to significant improvements in the efficiency and scalability of MK-FDA. We carry out extensive experiments on six datasets from various application areas, and compare closely the performance of `p MK-FDA, fixed norm MK-FDA, and several variants of SVM-based MKL (MK-SVM). Our results demonstrate that `p MK-FDA improves upon sparse MK-FDA in many practical situations. The results also show that on image categorisation problems, `p MK-FDA tends to outperform its SVM counterpart. Finally, we also discuss the connection between (MK-)FDA and (MK-)SVM, under the unified framework of regularised kernel machines.
Discriminative least squares regression (DLSR) aims to learn relaxed regression labels to replace strict zero-one labels. However, the distance of the labels from the same class can also be enlarged while using the ε-draggings technique to force the labels of different classes to move in the opposite directions, and roughly persuing relaxed labels may lead to the problem of overfitting. To solve above problems, we propose a low-rank discriminative least squares regression model (LRDLSR) for multi-class image classification. Specifically, LRDLSR class-wisely imposes low-rank constraint on the relaxed labels obtained by non-negative relaxation matrix to improve its within-class compactness and similarity. Moreover, LRDLSR introduces an additional regularization term on the learned labels to avoid the problem of overfitting. We show that these two improvements help to learn a more discriminative projection for regression, thus achieving better classification performance. The experimental results over a range of image datasets demonstrate the effectiveness of the proposed LRDLSR method. The Matlab code of the proposed method is available at https://github.com/chenzhe207/LRDLSR.
We present a fully automatic approach to real-time 3D face reconstruction from monocular in-the-wild videos. With the use of a cascaded-regressor based face tracking and a 3D Morphable Face Model shape fitting, we obtain a semi-dense 3D face shape. We further use the texture information from multiple frames to build a holistic 3D face representation from the video footage. Our system is able to capture facial expressions and does not require any person-specific training. We demonstrate the robustness of our approach on the challenging 300 Videos in the Wild (300-VW) dataset. Our real-time fitting framework is available as an open source library at http://4dface.org.
In this paper, a novel inverse random undersampling (IRUS) method is proposed for the class imbalance problem. The main idea is to severely under sample the majority class thus creating a large number of distinct training sets. For each training set we then find a decision boundary which separates the minority class from the majority class. By combining the multiple designs through fusion, we construct a composite boundary between the majority class and the minority class. The proposed methodology is applied on 22 UCI data sets and experimental results indicate a significant increase in performance when compared with many existing class-imbalance learning methods. We also present promising results for multi-label classification, a challenging research problem in many modern applications such as music, text and image categorization.
In pattern recognition, disagreement between two classifiers regarding the predicted class membership of an observation can be indicative of an anomaly and its nuance. As in general classifiers base their decisions on class aposteriori probabilities, the most natural approach to detecting classifier incongruence is to use divergence. However, existing divergences are not particularly suitable to gauge classifier incongruence. In this paper, we postulate the properties that a divergence measure should satisfy and propose a novel divergence measure, referred to as Delta divergence. In contrast to existing measures, it focuses on the dominant (most probable) hypotheses and thus reduces the effect of the probability mass distributed over the non dominant hypotheses (clutter). The proposed measure satisfies other important properties such as symmetry, and independence of classifier confidence. The relationship of the proposed divergence to some baseline measures, and its superiority, is shown experimentally.
A large amount of training data is usually crucial for successful supervised learning. However, the task of providing training samples is often time-consuming, involving a considerable amount of tedious manual work. Also the amount of training data available is often limited. As an alternative, in this paper, we discuss how best to augment the available data for the application of automatic facial landmark detection (FLD). We propose the use of a 3D morphable face model to generate synthesised faces for a regression-based detector training. Benefiting from the large synthetic training data, the learned detector is shown to exhibit a better capability to detect the landmarks of a face with pose variations. Furthermore, the synthesised training dataset provides accurate and consistent landmarks as compared to using manual landmarks, especially for occluded facial parts. The synthetic data and real data are from different domains; hence the detector trained using only synthesised faces does not generalise well to real faces. To deal with this problem, we propose a cascaded collaborative regression (CCR) algorithm, which generates a cascaded shape updater that has the ability to overcome the difficulties caused by pose variations, as well as achieving better accuracy when applied to real faces. The training is based on a mix of synthetic and real image data with the mixing controlled by a dynamic mixture weighting schedule. Initially the training uses heavily the synthetic data, as this can model the gross variations between the various poses. As the training proceeds, progressively more of the natural images are incorporated, as these can model finer detail. To improve the performance of the proposed algorithm further, we designed a dynamic multi-scale local feature extraction method, which captures more informative local features for detector training. An extensive evaluation on both controlled and uncontrolled face datasets demonstrates the merit of the proposed algorithm.
A pose-invariant face recognition system based on an image matching method formulated on MRFs s presented. The method uses the energy of the established match between a pair of images as a measure of goodness-of-match. The method can tolerate moderate global spatial transformations between the gallery and the test images and alleviates the need for geometric preprocessing of facial images by encapsulating a registration step as part of the system. It requires no training on non-frontal face images. A number of innovations, such as a dynamic block size and block shape adaptation, as well as label pruning and error prewhitening measures have been introduced to increase the effectiveness of the approach. The experimental evaluation of the method is performed on two publicly available databases. First, the method is tested on the rotation shots of the XM2VTS data set in a verification scenario. Next, the evaluation is conducted in an identification scenario on the CMU-PIE database. The method compares favorably with the existing 2D or 3D generative model based methods on both databases in both identification and verification scenarios.
Sparse-representation-based classification (SRC) has been widely studied and developed for various practical signal classification applications. However, the performance of a SRC-based method is degraded when both the training and test data are corrupted. To counteract this problem, we propose an approach that learns representation with block-diagonal structure (RBDS) for robust image recognition. To be more specific, we first introduce a regularization term that captures the block-diagonal structure of the target representation matrix of the training data. The resulting problem is then solved by an optimizer. Last, based on the learned representation, a simple yet effective linear classifier is used for the classification task. The experimental results obtained on several benchmarking datasets demonstrate the efficacy of the proposed RBDS method. The source code of our proposed RBDS is accessible at https://github.com/yinhefeng/RBDS.
Discriminative correlation filter (DCF) has achieved advanced performance in visual object tracking with remarkable efficiency guaranteed by its implementation in the frequency domain. However, the effect of the structural relationship of DCF and object features has not been adequately explored in the context of the filter design. To remedy this deficiency, this paper proposes a Low-rank and Sparse DCF (LSDCF) that improves the relevance of features used by discriminative filters. To be more specific, we extend the classical DCF paradigm from ridge regression to lasso regression, and constrain the estimate to be of low-rank across frames, thus identifying and retaining the informative filters distributed on a low-dimensional manifold. To this end, specific temporal-spatial-channel configurations are adaptively learned to achieve enhanced discrimination and interpretability. In addition, we analyse the complementary characteristics between hand-crafted features and deep features, and propose a coarse-to-fine heuristic tracking strategy to further improve the performance of our LSDCF. Last, the augmented Lagrange multiplier optimisation method is used to achieve efficient optimisation. The experimental results obtained on a number of well-known benchmarking datasets, including OTB2013, OTB50, OTB100, TC128, UAV123, VOT2016 and VOT2018, demonstrate the effectiveness and robustness of the proposed method, delivering outstanding performance compared to the state-of-the-art trackers.
Image decomposition is crucial for many image processing tasks, as it allows to extract salient features from source images. A good image decomposition method could lead to a better performance, especially in image fusion tasks. We propose a multi-level image decomposition method based on latent lowrank representation(LatLRR), which is called MDLatLRR. This decomposition method is applicable to many image processing fields. In this paper, we focus on the image fusion task. We build a novel image fusion framework based on MDLatLRR which is used to decompose source images into detail parts(salient features) and base parts. A nuclear-norm based fusion strategy is used to fuse the detail parts and the base parts are fused by an averaging strategy. Compared with other state-of-the-art fusion methods, the proposed algorithm exhibits better fusion performance in both subjective and objective evaluation.
3D face reconstruction of shape and skin texture from a single 2D image can be performed using a 3D Morphable Model (3DMM) in an analysis-by-synthesis approach. However, performing this reconstruction (fitting) efficiently and accurately in a general imaging scenario is a challenge. Such a scenario would involve a perspective camera to describe the geometric projection from 3D to 2D, and the Phong model to characterise illumination. Under these imaging assumptions the reconstruction problem is nonlinear and, consequently, computationally very demanding. In this work, we present an efficient stepwise 3DMM-to-2D image-fitting procedure, which sequentially optimises the pose, shape, light direction, light strength and skin texture parameters in separate steps. By linearising each step of the fitting process we derive closed-form solutions for the recovery of the respective parameters, leading to efficient fitting. The proposed optimisation process involves all the pixels of the input image, rather than randomly selected subsets, which enhances the accuracy of the fitting. It is referred to as Efficient Stepwise Optimisation (ESO). The proposed fitting strategy is evaluated using reconstruction error as a performance measure. In addition, we demonstrate its merits in the context of a 3D-assisted 2D face recognition system which detects landmarks automatically and extracts both holistic and local features using a 3DMM. This contrasts with most other methods which only report results that use manual face landmarking to initialise the fitting. Our method is tested on the public CMU-PIE and Multi-PIE face databases, as well as one internal database. The experimental results show that the face reconstruction using ESO is significantly faster, and its accuracy is at least as good as that achieved by the existing 3DMM fitting algorithms. A face recognition system integrating ESO to provide a pose and illumination invariant solution compares favourably with other state-of-the-art methods. In particular, it outperforms deep learning methods when tested on the Multi-PIE database.
The state of classfier incongruence in decision making systems incorporating multiple classifiers is often an indicator of anomaly caused by an unexpected observation or an unusual situation. Its assessment is important as one of the key mechanisms for domain anomaly detection. In this paper, we investigate the sensitivity of Delta divergence, a novel measure of classifier incongruence, to estimation errors. Statistical properties of Delta divergence are analysed both theoretically and experimentally. The results of the analysis provide guidelines on the selection of threshold for classifier incongruence detection based on this measure.
As biometric technology is rolled out on a larger scale, it will be a common scenario (known as cross-device matching) to have a template acquired by one biometric device used by another during testing. This requires a biometric system to work with different acquisition devices, an issue known as device interoperability. We further distinguish two subproblems, depending on whether the device identity is known or unknown. In the latter case, we show that the device information can be probabilistically inferred given quality measures (e.g., image resolution) derived from the raw biometric data. By keeping the template unchanged, cross-device matching can result in significant degradation in performance. We propose to minimize this degradation by using device-specific quality-dependent score normalization. In the context of fusion, after having normalized each device output independently, these outputs can be combined using the naive Bayes principal. We have compared and categorized several state-of-the-art quality-based score normalization procedures, depending on how the relationship between quality measures and score is modeled, as follows: 1) direct modeling; 2) modeling via the cluster index of quality measures; and 3) extending 2) to further include the device information (device-specific cluster index). Experimental results carried out on the Biosecure DS2 data set show that the last approach can reduce both false acceptance and false rejection rates simultaneously. Furthermore, the compounded effect of normalizing each system individually in multimodal fusion is a significant improvement in performance over the baseline fusion (without using any quality information) when the device information is given.
We seek to quantify both the classification performance and estimation error robustness of the authors' tomographic classifier fusion methodology by contrasting it in field tests and model scenarios with the sum and product classifier fusion methodologies. In particular, we seek to confirm that the tomographic methodology represents a generally optimal strategy across the entire range of problem dimensionalities, and at a sufficient margin to justify the general advocation of its use. Final results indicate, in particular, a near 25% improvement on the next nearest performing combination scheme at the extremity of the tested dimensional range.
To discover specific variants with relatively large effects on the human face, we have devised an approach to identifying facial features with high heritability. This is based on using twin data to estimate the additive genetic value of each point on a face, as provided by a 3D camera system. In addition, we have used the ethnic difference between East Asian and European faces as a further source of face genetic variation. We use principal components (PCs) analysis to provide a fine definition of the surface features of human faces around the eyes and of the profile, and chose upper and lower 10% extremes of the most heritable PCs for looking for genetic associations. Using this strategy for the analysis of 3D images of 1,832 unique volunteers from the wellcharacterized People of the British Isles study and 1,567 unique twin images from the TwinsUK cohort, together with genetic data for 500,000 SNPs, we have identified three specific genetic variants with notable effects on facial profiles and eyes.
A cell-free massive multiple-input multiple-output (MIMO) uplink is considered, where quantize-and-forward (QF) refers to the case where both the channel estimates and the received signals are quantized at the access points (APs) and forwarded to a central processing unit (CPU) whereas in combinequantize- and-forward (CQF), the APs send the quantized version of the combined signal to the CPU. To solve the non-convex sum rate maximization problem, a heuristic sub-optimal scheme is exploited to convert the power allocation problem into a standard geometric programme (GP). We exploit the knowledge of the channel statistics to design the power elements. Employing largescale-fading (LSF) with a deep convolutional neural network (DCNN) enables us to determine a mapping from the LSF coefficients and the optimal power through solving the sum rate maximization problem using the quantized channel. Four possible power control schemes are studied, which we refer to as i) small-scale fading (SSF)-based QF; ii) LSF-based CQF; iii) LSF use-and-then-forget (UatF)-based QF; and iv) LSF deep learning (DL)-based QF, according to where channel estimation is performed and exploited and how the optimization problem is solved. Numerical results show that for the same fronthaul rate, the throughput significantly increases thanks to the mapping obtained using DCNN.
Face recognition subject to uncontrolled illumination and blur is challenging. Interestingly, image degradation caused by blurring, often present in real-world imagery, has mostly been overlooked by the face recognition community. Such degradation corrupts face information and affects image alignment, which together negatively impact recognition accuracy. We propose a number of countermeasures designed to achieve system robustness to blurring. First, we propose a novel blur-robust face image descriptor based on Local Phase Quantization (LPQ) and extend it to a multiscale framework (MLPQ) to increase its effectiveness. To maximize the insensitivity to misalignment, the MLPQ descriptor is computed regionally by adopting a component-based framework. Second, the regional features are combined using kernel fusion. Third, the proposed MLPQ representation is combined with the Multiscale Local Binary Pattern (MLBP) descriptor using kernel fusion to increase insensitivity to illumination. Kernel Discriminant Analysis (KDA) of the combined features extracts discriminative information for face recognition. Last, two geometric normalizations are used to generate and combine multiple scores from different face image scales to further enhance the accuracy. The proposed approach has been comprehensively evaluated using the combined Yale and Extended Yale database B (degraded by artificially induced linear motion blur) as well as the FERET, FRGC 2.0, and LFW databases. The combined system is comparable to state-of-the-art approaches using similar system configurations. The reported work provides a new insight into the merits of various face representation and fusion methods, as well as their role in dealing with variable lighting and blur degradation.
Image set classification has recently received much attention due to its various applications in pattern recognition and computer vision. To compare and match image sets, the major challenges are to devise an effective and efficient representation and to define a measure of similarity between image sets. In this paper, we propose a method for representing image sets based on block-diagonal Covariance Descriptors (CovDs). In particular, the proposed image set representation is in the form of non-singular covariance matrices, also known as Symmetric Positive Definite (SPD) matrices, that lie on Riemannian manifold. By dividing each image of an image set into square blocks of the same size, we compute the corresponding block CovDs instead of the global one. Taking the relative discriminative power of these block CovDs into account, a block-diagonal SPD matrix can be constructed to achieve a better discriminative capability. We extend the proposed approach to work with bidirectional CovDs and achieve a further boost in performance. The resulting block-diagonal SPD matrices combined with Riemannian metrics are shown to provide a powerful basis for image set classification. We perform an extensive evaluation on four datasets for several image set classification tasks. The experimental results demonstrate the effectiveness and efficiency of the proposed method.
The one-class anomaly detection approach has previously been found to be effective in face presentation attack detection, especially in an ____textit{unseen} attack scenario, where the system is exposed to novel types of attacks. This work follows the same anomaly-based formulation of the problem and analyses the merits of deploying ____textit{client-specific} information for face spoofing detection. We propose training one-class client-specific classifiers (both generative and discriminative) using representations obtained from pre-trained deep convolutional neural networks. Next, based on subject-specific score distributions, a distinct threshold is set for each client, which is then used for decision making regarding a test query. Through extensive experiments using different one-class systems, it is shown that the use of client-specific information in a one-class anomaly detection formulation (both in model construction as well as decision threshold tuning) improves the performance significantly. In addition, it is demonstrated that the same set of deep convolutional features used for the recognition purposes is effective for face presentation attack detection in the class-specific one-class anomaly detection paradigm.
Fully automatic annotation of tennis game using broadcast video is a task with a great potential but with enormous challenges. In this paper we describe our approach to this task, which integrates computer vision, machine listening, and machine learning. At the low level processing, we improve upon our previously proposed state-of-the-art tennis ball tracking algorithm and employ audio signal processing techniques to detect key events and construct features for classifying the events. At high level analysis, we model event classification as a sequence labelling problem, and investigate four machine learning techniques using simulated event sequences. Finally, we evaluate our proposed approach on three real world tennis games, and discuss the interplay between audio, vision and learning. To the best of our knowledge, our system is the only one that can annotate tennis game at such a detailed level. © 2014 Elsevier B.V.
In recent years, attention mechanisms have been widely studied in Discriminative Correlation Filter (DCF) based visual object tracking. To realise spatial attention and discriminative feature mining, existing approaches usually apply regularisation terms to the spatial dimension of multi-channel features. However, these spatial regularisation approaches construct a shared spatial attention pattern for all multi-channel features, without considering the diversity across channels. As each feature map (channel) focuses on a specific visual attribute, a shared spatial attention pattern limits the capability for mining important information from different channels. To address this issue, we advocate channel-specific spatial attention for DCF-based trackers. The key ingredient of the proposed method is an Adaptive Attribute-Aware spatial attention mechanism for constructing a novel DCF-based tracker (A^3 DCF). To highlight the discriminative elements in each feature map, spatial sparsity is imposed in the filter learning stage, moderated by the prior knowledge regarding the expected concentration of signal energy. In addition, we perform a post processing of the identified spatial patterns to alleviate the impact of less significant channels. The net effect is that the irrelevant and inconsistent channels are removed by the proposed method. The results obtained on a number of well-known benchmarking datasets, including OTB2015, DTB70, UAV123, VOT2018, LaSOT, GOT-10 K and TrackingNet, demonstrate the merits of the proposed A^3 DCF tracker, with improved performance compared to the state-of-the-art methods.
We present a framework for robust face detection and landmark localisation of faces in the wild, which has been evaluated as part of 'the 2nd Facial Landmark Localisation Competition'. The framework has four stages: face detection, bounding box aggregation, pose estimation and landmark localisation. To achieve a high detection rate, we use two publicly available CNN-based face detectors and two proprietary detectors. We aggregate the detected face bounding boxes of each input image to reduce false positives and improve face detection accuracy. A cascaded shape regressor, trained using faces with a variety of pose variations, is then employed for pose estimation and image pre-processing. Last, we train the final cascaded shape regressor for fine-grained landmark localisation, using a large number of training samples with limited pose variations. The experimental results obtained on the 300W and Menpo benchmarks demonstrate the superiority of our framework over state-of-the-art methods.
This report presents results from the Video Person Recognition Evaluation held in conjunction with the 11th IEEE International Conference on Automatic Face and Gesture Recognition. Two experiments required algorithms to recognize people in videos from the Point-and-Shoot Face Recognition Challenge Problem (PaSC). The first consisted of videos from a tripod mounted high quality video camera. The second contained videos acquired from 5 different handheld video cameras. There were 1401 videos in each experiment of 265 subjects. The subjects, the scenes, and the actions carried out by the people are the same in both experiments. Five groups from around the world participated in the evaluation. The video handheld experiment was included in the International Joint Conference on Biometrics (IJCB) 2014 Handheld Video Face and Person Recognition Competition. The top verification rate from this evaluation is double that of the top performer in the IJCB competition. Analysis shows that the factor most effecting algorithm performance is the combination of location and action: where the video was acquired and what the person was doing.
We propose supervised spatial attention that employs a heatmap generator for instructive feature learning.•We formulate a rectified Gaussian scoring function to generate informative heatmaps.•We present scale-aware layer attention that eliminates redundant information from pyramid features.•A voting strategy is designed to produce more reliable classification results.•Our face detector achieves encouraging performance in accuracy and speed on several benchmarks. Modern anchor-based face detectors learn discriminative features using large-capacity networks and extensive anchor settings. In spite of their promising results, they are not without problems. First, most anchors extract redundant features from the background. As a consequence, the performance improvements are achieved at the expense of a disproportionate computational complexity. Second, the predicted face boxes are only distinguished by a classifier supervised by pre-defined positive, negative and ignored anchors. This strategy may ignore potential contributions from cohorts of anchors labelled negative/ignored during inference simply because of their inferior initialisation, although they can regress well to a target. In other words, true positives and representative features may get filtered out by unreliable confidence scores. To deal with the first concern and achieve more efficient face detection, we propose a Heatmap-assisted Spatial Attention (HSA) module and a Scale-aware Layer Attention (SLA) module to extract informative features using lower computational costs. To be specific, SLA incorporates the information from all the feature pyramid layers, weighted adaptively to remove redundant layers. HSA predicts a reshaped Gaussian heatmap and employs it to facilitate a spatial feature selection by better highlighting facial areas. For more reliable decision-making, we merge the predicted heatmap scores and classification results by voting. Since our heatmap scores are based on the distance to the face centres, they are able to retain all the well-regressed anchors. The experiments obtained on several well-known benchmarks demonstrate the merits of the proposed method.
The Visual Object Tracking challenge VOT2019 is the seventh annual tracker benchmarking activity organized by the VOT initiative. Results of 81 trackers are presented; many are state-of-the-art trackers published at major computer vision conferences or in journals in the recent years. The evaluation included the standard VOT and other popular methodologies for short-term tracking analysis as well as the standard VOT methodology for long-term tracking analysis. The VOT2019 challenge was composed of five challenges focusing on different tracking domains: (i) VOTST2019 challenge focused on short-term tracking in RGB, (ii) VOT-RT2019 challenge focused on "real-time" shortterm tracking in RGB, (iii) VOT-LT2019 focused on longterm tracking namely coping with target disappearance and reappearance. Two new challenges have been introduced: (iv) VOT-RGBT2019 challenge focused on short-term tracking in RGB and thermal imagery and (v) VOT-RGBD2019 challenge focused on long-term tracking in RGB and depth imagery. The VOT-ST2019, VOT-RT2019 and VOT-LT2019 datasets were refreshed while new datasets were introduced for VOT-RGBT2019 and VOT-RGBD2019. The VOT toolkit has been updated to support both standard shortterm, long-term tracking and tracking with multi-channel imagery. Performance of the tested trackers typically by far exceeds standard baselines. The source code for most of the trackers is publicly available from the VOT page. The dataset, the evaluation kit and the results are publicly available at the challenge website.
The Visual Object Tracking challenge VOT2018 is the sixth annual tracker benchmarking activity organized by the VOT initiative. Results of over eighty trackers are presented; many are state-of-the-art trackers published at major computer vision conferences or in journals in the recent years. The evaluation included the standard VOT and other popular methodologies for short-term tracking analysis and a "real-time" experiment simulating a situation where a tracker processes images as if provided by a continuously running sensor. A long-term tracking subchallenge has been introduced to the set of standard VOT sub-challenges. The new subchallenge focuses on long-term tracking properties, namely coping with target disappearance and reappearance. A new dataset has been compiled and a performance evaluation methodology that focuses on long-term tracking capabilities has been adopted. The VOT toolkit has been updated to support both standard short-term and the new long-term tracking subchallenges. Performance of the tested trackers typically by far exceeds standard baselines. The source code for most of the trackers is publicly available from the VOT page. The dataset, the evaluation kit and the results are publicly available at the challenge website (http://votchallenge.net).
We consider a family of structural descriptors for visual data, namely covariance descriptors (CovDs) that lie on a non-linear symmetric positive definite (SPD) manifold, a special type of Riemannian manifolds. We propose an improved version of CovDs for image set coding by extending the traditional CovDs from Euclidean space to the SPD manifold. Specifically, the manifold of SPD matrices is a complete inner product space with the operations of logarithmic multiplication and scalar logarithmic multiplication defined in the Log-Euclidean framework. In this framework, we characterise covariance structure in terms of the arc-cosine kernel which satisfies Mercer's condition and propose the operation of mean centralization on SPD matrices. Furthermore, we combine arc-cosine kernels of different orders using mixing parameters learnt by kernel alignment in a supervised manner. Our proposed framework provides a lower-dimensional and more discriminative data representation for the task of image set classification. The experimental results demonstrate its superior performance, measured in terms of recognition accuracy, as compared with the state-of-the-art methods.
With the development of neural networking techniques, several architectures for symmetric positive definite (SPD) matrix learning have recently been put forward in the computer vision and pattern recognition (CV&PR) community for mining fine-grained geometric features. However, the degradation of structural information during multi-stage feature transformation limits their capacity. To cope with this issue, this paper develops a U-shaped neural network on the SPD manifolds (U-SPDNet) for visual classification. The designed U-SPDNet contains two subsystems, one of which is a shrinking path (encoder) making up of a prevailing SPD manifold neural network (SPDNet (Huang and Van Gool, 2017)) for capturing compact representations from the input data. Another is a constructed symmetric expanding path (decoder) to upsample the encoded features, trained by a reconstruction error term. With this design, the degradation problem will be gradually alleviated during training. To enhance the representational capacity of U-SPDNet, we also append skip connections from encoder to decoder, realized by manifold-valued geometric operations, namely Riemannian barycenter and Riemannian optimization. On the MDSD, Virus, FPHA, and UAV-Human datasets, the accuracy achieved by our method is respectively 6.92%, 8.67%, 1.57%, and 1.08% higher than SPDNet, certifying its effectiveness. •This paper designs a U-shaped neural network in the context of SPD manifolds (USPDNet) to cope with the problem of statistical information degradation.•The geometric computation-based skip connections are added from the encoder to the decoder to improve the representational capacity of the proposed SPD network.•Extensive experiments show that our method can achieve improved accuracy, even with limited data.
In the domain of pattern recognition, using the CovDs (Covariance Descriptors) to represent data and taking the metrics of the resulting Riemannian manifold into account have been widely adopted for the task of image set classification. Recently, it has been proven that infinite-dimensional CovDs are more discriminative than their low-dimensional counterparts. However, the form of infinite-dimensional CovDs is implicit and the computational load is high. We propose a novel framework for representing image sets by approximating infinite-dimensional CovDs in the paradigm of the Nystrom method based on a Riemannian kernel. We start by modeling the images via CovDs, which lie on the Riemannian manifold spanned by SPD (Symmetric Positive Definite) matrices. We then extend the Nystrom method to the SPD manifold and obtain the approximations of CovDs in RKHS (Reproducing Kernel Hilbert Space). Finally, we approximate infinite-dimensional CovDs via these approximations. Empirically, we apply our framework to the task of image set classification. The experimental results obtained on three benchmark datasets show that our proposed approximate infinite-dimensional CovDs outperform the original CovDs.
Subspace clustering (SC) exploits the potential capacity of self-expressive modeling of unsupervised learning frameworks, representing each data point as a linear combination of the other related data points. Advanced self-expressive approaches construct an affinity matrix from the representation coefficients by imposing an additional regularization, reflecting the prior data distribution. An affine constraint is widely used for regularization in subspace clustering studies according on the grounds that, in real-world applications, data points usually lie in a union of multiple affine subspaces rather than linear subspaces. However, a strict affine constraint is not flexible enough to handle the real-world cases, as the observed data points are always corrupted by noise and outliers. To address this issue, we introduce the concept of scalable affine constraint to the SC formulation. Specifically, each coefficient vector is constrained to sum up to a soft scalar s rather than 1. The proposed method can estimate the most appropriate value of scalar s in the optimization stage, adaptively enhancing the clustering performance. Besides, as clustering benefits from multiple representations, we extend the scalable affine constraint to a multi-view clustering framework designed to achieve collaboration among the different representations adopted. An efficient optimization approach based on ADMM is developed to minimize the proposed objective functions. The experimental results on several datasets demonstrate the effectiveness of the proposed clustering approach constrained by scalable affine regularisation, with superior performance compared to the state-of-the-art.
With the continuously increasing amount of video data, image set classification has recently received widespread attention in the CV & PR community. However, the intra-class diversity and inter-class ambiguity of representations remain an open challenge. To tackle this issue, several methods have been put forward to perform multiple geometry-aware image set modelling and learning. Although the extracted complementary geometric information is beneficial for decision making, the sophisticated computational paradigm (e.g., scatter matrices computation and iterative optimisation) of such algorithms is counterproductive. As a countermeasure, we propose an effective hybrid Riemannian metric learning framework in this paper. Specifically, we design a multiple graph embedding-guided metric learning framework for the sake of fusing these complementary kernel features, obtained via the explicit RKHS embeddings of the Grassmannian manifold, SPD manifold, and Gaussian embedded Riemannian manifold, into a unified subspace for classification. Furthermore, the involved optimisation problem of the developed model can be solved in terms of a series of sub-problems, achieving improved efficiency theoretically and experimentally. Substantial experiments are carried out to evaluate the efficacy of our approach. The experimental results suggest the superiority of it over the state-of-the-art methods.
Face anti-spoofing (FAS) is crucial for safe and reliable biometric systems. In recent years, deep neural networks have been proven to be very effective for FAS as compared with classical approaches. However, deep learning-based FAS methods are data-driven and use learning-based features only. It is a legitimate question to ask whether hand-crafted features can provide any complementary information to a deep learning-based FAS method. To answer this question, we propose a two-stream network that consists of a convolutional network and a local difference network. To be specific, we first build a texture extraction convolutional block to calculate the gradient magnitude at each pixel of an input image. Our experiments demonstrate that additional liveness cues can be captured by the proposed method. Second, we design an attention fusion module to combine the features obtained from the RGB domain and gradient magnitude domain, aiming for discriminative information mining and information redundancy elimination. Finally, we advocate a simple binary facial mask supervision strategy for further performance boost. The proposed network has only 2.79M parameters and the inference speed is up to 118 frames per second, which makes it very convenient for real-time FAS systems. The experimental results obtained on several well-known benchmarking datasets demonstrate the merits and superiority of the proposed method over the state-of-the-art approaches.
In this paper we propose a new approach to person re-identification using images and natural language descriptions. We propose a joint vision and language model based on CNN and LSTM architectures to match across the two modalities as well as to enrich visual examples for which there are no language descriptions. We also introduce new annotations in the form of natural language descriptions for two standard Re-ID benchmarks, namely CUHK03 and VIPeR. We perform experiments on these two datasets with techniques based on CNN, hand-crafted features as well as LSTM for analysing visual and natural description data. We investigate and demonstrate the advantages of using natural language descriptions compared to attributes as well as CNN compared to LSTM in the context of Re-ID. We show that the joint use of language and vision can significantly improve the state-of-the-art performance on standard Re-ID benchmarks.
Efficient neural networks has received ever-increasing attention with the evolution of convolutional neural networks (CNNs), especially involving their deployment on embedded and mobile platforms. One of the biggest problems to obtaining such efficient neural networks is efficiency, even recent differentiable neural architecture search (DNAS) requires to sample a small number of candidate neural architectures for the selection of the optimal neural architecture. To address this computational efficiency issue, we introduce a novel architecture parameterization based on scaled sigmoid function , and propose a general Differentiable Neural Architecture Learning (DNAL) method to obtain efficient neural networks without the need to evaluate candidate neural networks. Specifically, for stochastic supernets as well as conventional CNNs, we build a new channel-wise module layer with the architecture components controlled by a scaled sigmoid function. We train these neural network models from scratch. The network optimization is decoupled into the weight optimization and the architecture optimization, which avoids the interaction between the two types of parameters and alleviates the vanishing gradient problem. We address the non-convex optimization problem of efficient neural networks by the continuous scaled sigmoid method instead of the common softmax method. Extensive experiments demonstrate our DNAL method delivers superior performance in terms of efficiency, and adapts to conventional CNNs (e.g., VGG16 and ResNet50), lightweight CNNs (e.g., MobileNetV2) and stochastic supernets (e.g., ProxylessNAS). The optimal neural networks learned by DNAL surpass those produced by the state-of-the-art methods on the benchmark CIFAR-10 and ImageNet-1K dataset in accuracy, model size and computational complexity. Our source code is available at https://github.com/QingbeiGuo/DNAL.git . (c) 2022 Elsevier Ltd. All rights reserved.
We propose a photorealistic style transfer network to emphasize the natural effect of photorealistic image stylization. In general, distortion of the image content and lacking of details are two typical issues in the style transfer field. To this end, we design a framework employing the U-Net structure to maintain the rich spatial clues, with a multi-layer feature aggregation (MFA) method to simultaneously provide the details obtained by the shallow layers in the stylization processing. In particular, an encoder based on the dense block and a decoder form a symmetrical structure of U-Net are jointly staked to realize an effective feature extraction and image reconstruction. In addition, a transfer module based on MFA and "adaptive instance normalization" is inserted in the skip connection positions to achieve the stylization. Accordingly, the stylized image possesses the texture of a real photo and preserves rich content details without introducing any mask or postprocessing steps. The experimental results on public datasets demonstrate that our method achieves a more faithful structural similarity with a lower style loss, reflecting the effectiveness and merit of our approach. (C) 2021 SPIE and IS&T
Spatiotemporal modeling is crucial for capturing motion information in videos for action recognition task. Despite of the promising progress in skeleton-based action recognition by graph convolutional networks (GCNs), the relative improvement of applying the classical attention mechanism has been limited. In this paper, we underline the importance of spatio-temporal interactions by proposing different categories of attention modules. Initially, we focus on providing an insight into different attention modules, the Spatial-wise Attention Module (SAM) and the Temporal-wise Attention Module (TAM), which model the contexts interdependencies in spatial and temporal dimensions respectively. Then, the Spatiotemporal Attention Module (STAM) explicitly leverages comprehensive dependency information by the feature fusion structure embedded in the framework, which is different from other action recognition models with additional information flow or complicated superposition of multiple existing attention modules. Given intermediate feature maps, STAM simultaneously infers the feature descriptors along the spatial and temporal dimensions. The fusion of the feature descriptors filters the input feature maps for adaptive feature refinement. Experimental results on NTU RGB+D and KineticsSkeleton datasets show consistent improvements in classification performance, demonstrating the merit and a wide applicability of STAM.
•A s parse non negative transition subspace learning (SN TSL) algorithm is proposed to solve the over fitting problem.•The l1 norm sparsity and non negativity constraints are imposed on the transition subspace to increase the explainability of model.•SN TSL is a general subspace learning model that can be combined with other methods designed to mitigate over fitting, such as label relaxation, graph embedding, etc. Recently many variations of least squares regression (LSR) have been developed to address the problem of over-fitting that widely exists in the task of image classification. Among these methods, the most prevalent two means, such as label relaxation and graph manifold embedding, have been demonstrated to be highly effective. In this paper, we present a new strategy named sparse non-negative transition subspace learning (SN-TSL) based least squares regression algorithm which aims to avoid over-fitting by learning a transition subspace between the multifarious high-dimensional inputs and low-dimensional binary labels. Moreover, considering the final regression targets are sparse binary positive matrices, we use the l1-norm and the non-negativity constraint to enforce the transition subspace to be sparse and non-negative. The resulting subspace features can be viewed as intermediate representations between the inputs and labels. Because SN-TSL can simultaneously learn two projection matrices in one regression model and the dimensionality of the transition subspace can be set to any integer, SN-TSL has the potential to obtain more distinct projections for classification. It is also suitable for classification problems involving a small number of classes. Extensive experiments on public datasets have shown the proposed SN-TSL outperforms other state-of-the-art LSR based image classification methods.
•MBy utilizing the NMR model, we can make full use of the low-rank structural information of contiguous error images.•Different to L2-norm based NMR, we simultaneously exploit low-rank error images and sparse regression representations.•Experiments show pursuing sparse representations is more helpful to remove low-rank contiguous noise. Nuclear norm based matrix regression (NMR) method has been proposed to alleviate the influence of contiguous occlusion on face recognition problems. NMR considers that the error image of a test sample has low-rank structure due to the contiguous nature of occlusion. Based on the observation that l1-norm can uncover more natural sparsity of representations than l2-norm, we propose a sparse regularized NMR (SR_NMR) algorithm by imposing the l1-norm constraint rather than l2-norm on the representations of NMR framework. SR_NMR seamlessly integrates the nuclear norm based error matrix regression and l1-norm based sparse representation into one joint framework. Finally, we use the training samples to learn a linear classifier to implement efficient classification. Extensive experiments on three face databases show the proposed SR_NMR can achieve better recognition performance compared with the traditional NMR and other regression methods which indicates that sparse representations are very helpful to recover low-rank error images in the presence of severe occlusion and illumination changes.
Network convergence as well as recognition accuracy are essential issues when applying Convolutional Neural Networks (CNN) to human action recognition. Most deep learning methods neglect model convergence when striving to improve the abstraction capability, thus degrading the performances sharply when computing resources are limited. To mitigate this problem, we propose a structure named 2D Progressive Fusion (2DPF) Module which is inserted after the 2D backbone CNN layers. 2DPF fuses features through a novel 2D convolution on the spatial and temporal dimensions called variation attenuating convolution and applies fusion techniques to improve the recognition accuracy and the convergency. Our experiments performed on several benchmarks (e.g., Something-Something V1&V2, Kinetics400 & 600, AViD, UCF101) demonstrate the effectiveness of the proposed method. ARTICLE INFO.
Face detection and recognition benchmarks have shifted toward more difficult environments. The challenge presented in this paper addresses the next step in the direction of automatic detection and identification of people from outdoor surveillance cameras. While face detection has shown remarkable success in images collected from the web, surveillance cameras include more diverse occlusions, poses, weather conditions and image blur. Although face verification or closed-set face identification have surpassed human capabilities on some datasets, open-set identification is much more complex as it needs to reject both unknown identities and false accepts from the face detector. We show that unconstrained face detection can approach high detection rates albeit with moderate false accept rates. By contrast, open-set face recognition is currently weak and requires much more attention.
3D assisted 2D face recognition involves the process of reconstructing 3D faces from 2D images and solving the problem of face recognition in 3D. To facilitate the use of deep neural networks, a 3D face, normally represented as a 3D mesh of vertices and its corresponding surface texture, is remapped to image-like square isomaps by a conformal mapping. Based on previous work, we assume that face recognition benefits more from texture. In this work, we focus on the surface texture and its discriminatory information content for recognition purposes. Our approach is to prepare a 3D mesh, the corresponding surface texture and the original 2D image as triple input for the recognition network, to show that 3D data is useful for face recognition. Texture enhancement methods to control the texture fusion process are introduced and we adapt data augmentation methods. Our results show that texture-map-based face recognition can not only compete with state-of-the-art systems under the same preconditions but also outperforms standard 2D methods from recent years.
•Characterizing the similarities between the regions that contain more comprehensive information than pixels.•Presenting two methods for computing Riemannian local difference vector on Gaussian manifold (RieLDV-G) and using RieLDV-G to define deviation.•Providing a novel framework for computing covariance on Gaussian manifold and generating the proposed Riemannian covariance descriptors (RieCovDs). Covariance descriptors (CovDs) for image set classification have been widely studied recently. Different from the conventional CovDs, which describe similarities between pixels at different locations, we focus more on similarities between regions that convey more comprehensive information. In this paper, we extract pixel-wise features of image regions and represent them by Gaussian models. We extend the conventional covariance computation onto a special type of Riemannian manifold, namely a Gaussian manifold, so that it is applicable to our image set data representation provided in terms of Gaussian models. We present two methods to calculate a Riemannian local difference vector on the Gaussian manifold (RieLDV-G) and generate our proposed Riemannian covariance descriptors (RieCovDs) using the resulting RieLDV-G. By measuring the recognition accuracy achieved on benchmarking datasets, we demonstrate experimentally the superior performance of our proposed RieCovDs descriptors, as compared with state-of-the-art methods. (The code is available at:https://github.com/Kai-Xuan/RiemannianCovDs)
Learning feature embeddings for pattern recognition is a relevant task for many applications. Deep learning methods such as convolutional neural networks can be employed for this assignment with different training strategies: leveraging pre-trained models as baselines; training from scratch with the target dataset; or fine-tuning from the pre-trained model. Although there are separate systems used for learning features from labelled and unlabelled data, there are few models combining all available information. Therefore, in this paper, we present a novel semi-supervised deep network training strategy that comprises a convolutional network and an autoencoder using a joint classification and reconstruction loss function. We show our network improves the learned feature embedding when including the unlabelled data in the training process. The results using the feature embedding obtained by our network achieve better classification accuracy when compared with competing methods, as well as offering good generalisation in the context of transfer learning. Furthermore, the proposed network ensemble and loss function is highly extensible and applicable in many recognition tasks.
Hashing techniques have been applied broadly in retrieval tasks due to their low storage requirements and high speed of processing. Many hashing methods based on a single view have been extensively studied for information retrieval. However, the representation capacity of a single view is insufficient and some discriminative information is not captured, which results in limited improvement. In this paper, we employ multiple views to represent images and texts for enriching the feature information. Our framework exploits the complementary information among multiple views to better learn the discriminative compact hash codes. A discrete hashing learning framework that jointly performs classifier learning and subspace learning is proposed to complete multiple search tasks simultaneously. Our framework includes two stages, namely a kernelization process and a quantization process. Kernelization aims to find a common subspace where multi-view features can be fused. The quantization stage is designed to learn discriminative unified hashing codes. Extensive experiments are performed on single-label datasets (WiKi and MMED) and multi-label datasets (MIRFlickr and NUS-WIDE), and the experimental results indicate the superiority of our method compared with the state-of-the-art methods.
Although group convolution operators are increasingly used in deep convolutional neural networks to improve the computational efficiency and to reduce the number of parameters, most existing methods construct their group convolution architectures by a predefined partitioning of the filters of each convolutional layer into multiple regular filter groups with an equal spatial group size and data-independence, which prevents a full exploitation of their potential. To tackle this issue, we propose a novel method of designing self-grouping convolutional neural networks, called SG-CNN, in which the filters of each convolutional layer group themselves based on the similarity of their importance vectors. Concretely, for each filter, we first evaluate the importance value of their input channels to identify the importance vectors, and then group these vectors by clustering. Using the resulting data-dependent centroids, we prune the less important connections, which implicitly minimizes the accuracy loss of the pruning, thus yielding a set of diverse group convolution filters. Subsequently, we develop two fine-tuning schemes, i.e. (1) both local and global fine-tuning and (2) global only fine-tuning, which experimentally deliver comparable results, to recover the recognition capacity of the pruned network. Comprehensive experiments carried out on the CIFAR-10/100 and ImageNet datasets demonstrate that our self-grouping convolution method adapts to various state-of-the-art CNN architectures, such as ResNet and DenseNet, and delivers superior performance in terms of compression ratio, speedup and recognition accuracy. We demonstrate the ability of SG-CNN to generalize by transfer learning, including domain adaption and object detection, showing competitive results. Our source code is available at https://github.com/QingbeiGuo/SG-CNN.git.
Skeleton representation has attracted a great deal of attention recently as an extremely robust feature for human action recognition. However, its non-Euclidean structural characteristics raise new challenges for conventional solutions. Recent studies have shown that there is a native superiority in modeling spatiotemporal skeleton information with a Graph Convolutional Network (GCN). Nevertheless, the skeleton graph modeling normally focuses on the physical adjacency of the elements of the human skeleton sequence, which contrasts with the requirement to provide a perceptually meaningful representation. To address this problem, in this paper, we propose a perceptually-enriched graph learning method by introducing innovative features to spatial and temporal skeleton graph modeling. For the spatial information modeling, we incorporate a Local-Global Graph Convolutional Network (LG-GCN) that builds a multifaceted spatial perceptual representation. This helps to overcome the limitations caused by over-reliance on the spatial adjacency relationships in the skeleton. For temporal modeling, we present a Region-Aware Graph Convolutional Network (RA-GCN), which directly embeds the regional relationships conveyed by a skeleton sequence into a temporal graph model. This innovation mitigates the deficiency of the original skeleton graph models. In addition, we strengthened the ability of the proposed channel modeling methods to extract multi-scale representations. These innovations result in a lightweight graph convolutional model, referred to as Graph2Net, that simultaneously extends the spatial and temporal perceptual fields, and thus enhances the capacity of the graph model to represent skeleton sequences. We conduct extensive experiments on NTU-RGB+D 60&120, Northwestern-UCLA, and Kinetics-400 datasets to show that our results surpass the performance of several mainstream methods while limiting the model complexity and computational overhead.
Cross-modal person re-identification (Re-ID) is critical for modern video surveillance systems. The key challenge is to align cross-modality representations induced by the semantic information present for a person and ignore background information. This work presents a novel convolutional neural network (CNN) based architecture designed to learn semantically aligned cross-modal visual and textual representations. The underlying building block, named AXM-Block, is a unified multi-layer network that dynamically exploits the multi-scale knowledge from both modalities and re-calibrates each modality according to shared semantics. To complement the convolutional design, contextual attention is applied in the text branch to manipulate long-term dependencies. Moreover, we propose a unique design to enhance visual part-based feature coherence and locality information. Our framework is novel in its ability to implicitly learn aligned semantics between modalities during the feature learning stage. The unified feature learning effectively utilizes textual data as a super-annotation signal for visual representation learning and automatically rejects irrelevant information. The entire AXM-Net is trained end-to-end on CUHK-PEDES data. We report results on two tasks, person search and cross-modal Re-ID. The AXM-Net outperforms the current state-of-the-art (SOTA) methods and achieves 64.44\% Rank@1 on the CUHK-PEDES test set. It also outperforms its competitors by \(>\)10\% in cross-viewpoint text-to-image Re-ID scenarios on CrossRe-ID and CUHK-SYSU datasets.
In image set classification, a considerable advance has been made by modeling the original image sets by second order statistics or linear subspace, which typically lie on the Riemannian manifold. Specifically, they are Symmetric Positive Definite (SPD) manifold and Grassmann manifold respectively, and some algorithms have been developed on them for classification tasks. Motivated by the inability of existing methods to extract discriminatory features for data on Riemannian manifolds, we propose a novel algorithm which combines multiple manifolds as the features of the original image sets. In order to fuse these manifolds, the well-studied Riemannian kernels have been utilized to map the original Riemannian spaces into high dimensional Hilbert spaces. A metric Learning method has been devised to embed these kernel spaces into a lower dimensional common subspace for classification. The state-of-the-art results achieved on three datasets corresponding to two different classification tasks, namely face recognition and object categorization, demonstrate the effectiveness of the proposed method.
The research in pedestrian detection has made remarkable progress in recent years. However, robust pedestrian detection in crowded scenes remains a considerable challenge. Many methods resort to additional annotations (visible body or head) of a dataset or develop attention mechanisms to alleviate the difficulties posed by occlusions. However, these methods rarely use contextual information to strengthen the features extracted by a backbone network. The main aim of this paper is to extract more effective and discriminative features of pedestrians for robust pedestrian detection with heavy occlusions. To this end, we propose a Global Context-Aware module to exploit contextual information for pedestrian detection. Fusing global context with the information derived from the visible part of occluded pedestrians enhances feature representations. The experimental results obtained on two challenging benchmarks, CrowdHuman and CityPersons, demonstrate the effectiveness and merits of the proposed method. Code and models are available at: https://github.com/FlyingZstar/crowded pedestrian detection.
We propose a novel structured analysis-synthesis dictionary pair learning method for efficient representation and image classification, referred to as relaxed block-diagonal dictionary pair learning with a locality constraint (RBD-DPL). RBD-DPL aims to learn relaxed block-diagonal representations of the input data to enhance the discriminability of both analysis and synthesis dictionaries by dynamically optimizing the block-diagonal components of representation, while the off-block-diagonal counterparts are set to zero. In this way, the learned synthesis subdictionary is allowed to be more flexible in reconstructing the samples from the same class, and the analysis dictionary effectively transforms the original samples into a relaxed coefficient subspace, which is closely associated with the label information. Besides, we incorporate a locality-constraint term as a complement of the relaxation learning to enhance the locality of the analytical encoding so that the learned representation exhibits high intraclass similarity. A linear classifier is trained in the learned relaxed representation space for consistent classification. RBD-DPL is computationally efficient because it avoids both the use of class-specific complementary data matrices to learn discriminative analysis dictionary, as well as the time-consuming l_{1}/l_{0} -norm sparse reconstruction process. The experimental results demonstrate that our RBD-DPL achieves at least comparable or better recognition performance than the state-of-the-art algorithms. Moreover, both the training and testing time are significantly reduced, which verifies the efficiency of our method. The MATLAB code of the proposed RBD-DPL is available at https://github.com/chenzhe207/RBD-DPL .
Face anti-spoofing problem can be quite challenging due to various factors including diversity of face spoofing attacks, any new means of spoofing, the problem of imaging sensor interoperability and other environmental factors in addition to the small sample size. Taking into account these observations, in this work, first, a new evaluation protocol called "innovative attack evaluation protocol" to study the effect of occurrence of unseen attack types is proposed which better reflects the realistic conditions in spoofing attacks. Second, a new formulation of the problem based on the anomaly detection concept is proposed where the training data comes from the positive class only. The test data, of course, may come from the positive or negative class. Finally, a thorough evaluation and comparison of 20 different one-class and two-class systems is performed and demonstrated that the anomaly-based formulation is not inferior as compared with the conventional two-class approach.
Recently, hashing-based multimodal learning systems have received increasing attention due to their query efficiency and parsimonious storage costs. However, impeded by the quantization loss caused by numerical optimization, the existing cross-media hashing approaches are unable to capture all the discriminative information present in the original multimodal data. Besides, most cross-modal methods belong to the one-step paradigm, which learn the binary codes and hash function simultaneously, increasing the complexity of optimization. To address these issues, we propose a novel two-stage approach, named the two-stage supervised discrete hashing (TSDH) method. In particular, in the first phase, TSDH generates a latent representation for each modality. These representations are then mapped to a common Hamming space to generate the binary codes. In addition, TSDH directly endows the hash codes with the semantic labels, enhancing the discriminatory power of the learned binary codes. A discrete hash optimization approach is developed to learn the binary codes without relaxation, avoiding the large quantization loss. The proposed hash function learning scheme reuses the semantic information contained by the embeddings, endowing the hash functions with enhanced discriminability. Extensive experiments on several databases demonstrate the effectiveness of the developed TSDH, outperforming several recent competitive cross-media algorithms.
Pruning methods to compress and accelerate deep convolutional neural networks (CNNs) have recently attracted growing attention, with the view of deploying pruned networks on resource-constrained hardware devices. However, most existing methods focus on small granularities, such as weight, kernel and filter, for the exploration of pruning. Thus, it will be bound to iteratively prune the whole neural networks based on those small granularities for high compression ratio with little performance loss. To address these issues, we theoretically analyze the relationship between the activation and gradient sparsity, and the channel saliency. Based on our findings, we propose a novel and effective method of weak sub-network pruning (WSP). Specifically, for a well-trained network model, we divide the whole compression process into two non-iterative stages. The first stage is to directly obtain a strong sub-network by pruning the weakest one. We first identify the less important channels from all the layers and determine the weakest sub-network, whereby each selected channel makes a minimal contribution to both the feed-forward and feed-backward processes. Then, a one-shot pruning strategy is executed to form a strong sub-network enabling fine tuning, while significantly reducing the impact of the network depth and width on the compression efficiency, especially for deep and wide network architectures. The second stage is to globally fine-tune the strong sub-network using several epochs to restore its original recognition accuracy. Furthermore, our proposed method impacts on the fully-connected layers as well as the convolutional layers for simultaneous compression and acceleration. Comprehensive experiments on VGG16 and ResNet-50 involving a variety of popular benchmarks, such as ImageNet-1K, CIFAR-10, CUB-200 and PASCAL VOC, demonstrate that our WSP method achieves superior performance on classification, domain adaption and object detection tasks with small model size. Our source code is available at https://github.com/QingbeiGuo/WSP.git.
In this paper, we address the problem of bird audio detection and propose a new convolutional neural network architecture together with a divergence based information channel weighing strategy in order to achieve improved state-of-the-art performance and faster convergence. The effectiveness of the methodology is shown on the Bird Audio Detection Challenge 2018 (Detection and Classification of Acoustic Scenes and Events Challenge, Task 3) development data set.
The problem of simultaneously predicting multiple real-valued outputs using a shared set of input variables is known as multi-target regression and has attracted considerable interest in the past couple of years. The dominant approach in the literature for multi-target regression is to capture the dependencies between the outputs through a linear model and express it as an output mixing matrix. This modelling formalism, however, is too simplistic in real-world problems where the output variables are related to one another in a more complex and non-linear fashion. To address this problem, in this study, we propose a structural modelling approach where the correlations between output variables are modelled using a non-linear approach. In particular, we pose the multi-target regression problem as one of vector-valued composition function learning in the reproducing kernel Hilbert space and propose a non-linear structure learning approach to capture the relationship between the outputs via an output kernel. By virtue of using a non-linear output kernel function, the proposed approach can better discover non-linear dependencies among targets for improved prediction performance. An extensive evaluation conducted on different databases reveals the benefits of the proposed multi-target regression technique against the baseline and the state-of-the-art methods.
We consider the problem of anomaly detection in an audio-visual analysis system designed to interpret sequences of actions from visual and audio cues. The scene activity recognition is based on a generative framework, with a high-level inference model for contextual recognition of sequences of actions. The system is endowed with anomaly detection mechanisms, which facilitate differentiation of various types of anomalies. This is accomplished using intelligence provided by a classifier incongruence detector, classifier confidence module and data quality assessment system, in addition to the classical outlier detection module. The paper focuses on one of the mechanisms, the classifier incongruence detector, the purpose of which is to flag situations when the video and audio modalities disagree in action interpretation. We demonstrate the merit of using the Delta divergence measure for this purpose. We show that this measure significantly enhances the incongruence detection rate in the Human Action Manipulation complex activity recognition data set.
Recently, hashing techniques have gained importance in large-scale retrieval tasks because of their retrieval speed. Most of the existing cross-view frameworks assume that data are well paired. However, the fully-paired multiview situation is not universal in real applications. The aim of the method proposed in this paper is to learn the hashing function for semi-paired cross-view retrieval tasks. To utilize the label information of partial data, we propose a semi-supervised hashing learning framework which jointly performs feature extraction and classifier learning. The experimental results on two datasets show that our method outperforms several state-of-the-art methods in terms of retrieval accuracy.
Some of the data collected from practical applications are usually heavily corrupted. In sub-space clustering, the common method is to use the specific regularization strategy to cor-rect these corrupted data by virtue of the prior knowledge, which could result in a suboptimal clustering solution. To alleviate the problem, we develop a novel formulation named subspace clustering via joint & POUND;1,2 and & POUND;2,1 (L12-21) norms (SCJL12-21). Specifically, we identify and exclude the heavily corrupted data points (unimportant data points) from participating in the linear representation of other points by imposing the & POUND;1,2 norm on the representation matrix, and improve the robustness to outliers by enforcing the & POUND;2,1 con-straint on the error matrix. The joint & POUND;1,2 and & POUND;2,1 minimization leads to a good representa-tion matrix which enhances the clustering performance. Related & POUND;1,2 and & POUND;2,1 norm constrained optimization problem is solved by utilizing the augmented Lagrange multiplier method. The effectiveness of the proposed method is demonstrated through experiments on the constructed datasets as well as the two practical problems of motion segmentation and face clustering.
Strict '0-1' block-diagonal low-rank representation is known to extract more structured information. However, it is often overlooked that a test sample from one class may be well represented by the dictionary atoms from other classes. To alleviate this problem, we propose a robust low-rank recovery algorithm (RLRR) with a distance-measure structure (DMS) for face recognition. When representing a test sample, DMS highlights the energy of the low-rank coefficients when the distance from the corresponding dictionary atoms is small. Moreover, RLRR introduces a structure-preserving regularization term to strengthen the similarity of within-class coefficients. Besides, RLRR builds a link between training and test samples to ensure the consistency of representation. The alternative direction multipliers method (ADMM) is used to optimize the proposed RLRR algorithm. Experiments on three benchmark face databases verify the superiorty of RLRR compared with state-of-the-art algorithms.
3D face alignment from monocular images is a crucial process in computer vision with applications to face recognition, animation and other areas. However, most algorithms are designed for faces in small to medium poses (below 45 degrees), lacking the ability to align faces in large poses up to 90 degrees. At the same time, many methods are not efficient. The main challenge is that it is time consuming to determine the parameters accurately. In order to address this issue, this paper proposes a novel and efficient end-to-end 3D face alignment framework. We build an efficient and stable network model through Depthwise Separable Convolution and Densely Connected Convolutional, named Mob-DenseNet. Simultaneously, different loss functions are used to constrain 3D parameters based on 3D Morphable Model (3DMM) and 3D vertices. Experiments on the challenging AFLW, AFLW2000-3D databases show that our algorithm significantly improves the accuracy of 3D face alignment. Model parameters and complexity of the proposed method are also reduced significantly.
We propose a deep learning network L1-2D(2)PCANet for face recognition, which is based on L1-norm-based two-dimensional principal component analysis (L1-2DPCA). In our network, the role of L1-2DPCA is to learn the filters of multiple convolution layers. After the convolution layers, we deploy binary hashing and blockwise histogram for pooling. We test our network on some benchmark facial datasets, including Yale, AR face database, extended Yale B, labeled faces in the wild-aligned, and Face Recognition Technology database with the convolution neural network, PCANet, 2DPCANet, and L1-PCANet as comparison. The results show that the recognition performance of L1-2D(2)PCANet in all tests is better than baseline networks, especially when there are outliers in the test data. Owing to the L1-norm, L1-2D(2)PCANet is robust to outliers and changes of the training images. (C) 2019 SPIE and IS&T
Three-dimensional (3-D) face reconstruction is an important task in the field of computer vision. Although 3-D face reconstruction has been developing rapidly in recent years, large pose face reconstruction is still a challenge. That is because much of the information about a face in a large pose will be unknowable. In order to address this issue, we propose a 3-D face reconstruction algorithm (PIFR) based on 3-D morphable model. A model alignment formulation is developed in which the original image and a normalized frontal image are combined to define a weighted loss in a landmark fitting process, with the intuition that the original image provides more expression and pose information, whereas the normalized image provides more identity information. Our method solves the problem of face reconstruction of a single image of a traditional method in a large pose, works on arbitrary pose and expressions, and greatly improves the accuracy of reconstruction. Experiments on the challenging AFW, LFPW, and AFLW database show that our algorithm significantly improves the accuracy of 3-D face reconstruction even under extreme poses (+/- 90 yaw angles). (C) 2019 SPIE and IS&T
Learning comprehensive spatiotemporal features is crucial for human action recognition. Existing methods tend to model the spatiotemporal feature blocks in an integrate-separate-integrate form, such as appearance-and-relation network (ARTNet) and spatiotemporal and motion network (STM). However, with blocks stacking up, the rear part of the network has poor interpretability. To avoid this problem, we propose a novel architecture called spatial temporal relation network (STRNet), which can learn explicit information of appearance, motion and especially the temporal relation information. Specifically, our STRNet is constructed by three branches, which separates the features into 1) appearance pathway, to obtain spatial semantics, 2) motion pathway, to reinforce the spatiotemporal feature representation, and 3) relation pathway, to focus on capturing temporal relation details of successive frames and to explore long-term representation dependency. In addition, our STRNet does not just simply merge the multi-branch information, but we apply a flexible and effective strategy to fuse the complementary information from multiple pathways. We evaluate our network on four major action recognition benchmarks: Kinetics-400, UCF-101, HMDB-51, and Something-Something v1, demonstrating that the performance of our STRNet achieves the state-of-the-art result on the UCF-101 and HMDB-51 datasets, as well as a comparable accuracy with the state-of-the-art method on Something-Something v1 and Kinetics-400.
Recent research has shown that modeling the dynamic joint features of the human body by a graph convolutional network (GCN) is a groundbreaking approach for skeleton-based action recognition, especially for the recognition of the body-motion, human-object and human-human interactions. Nevertheless, how to model and utilize coherent skeleton information comprehensively is still an open problem. In order to capture the rich spatiotemporal information and utilize features more effectively, we introduce a spatial residual layer and a dense connection block enhanced spatial temporal graph convolutional network. More specifically, our work introduces three aspects. Firstly, we extend spatial graph convolution to spatial temporal graph convolution of cross-domain residual to extract more precise and informative spatiotemporal feature, and reduce the training complexity by feature fusion in the, so-called, spatial residual layer. Secondly, instead of simply superimposing multiple similar layers, we use dense connection to take full advantage of the global information. Thirdly, we combine the above mentioned two components to create a spatial temporal graph convolutional network (ST-GCN), referred to as SDGCN. The proposed graph representation has a new structure. We perform extensive experiments on two large datasets: Kinetics and NTU-RGB+D. Our method achieves a great improvement in performance compared to the mainstream methods. We evaluate our method quantitatively and qualitatively, thus proving its effectiveness.
We propose an alternating deep-layer cascade (A-DLC) architecture for representation learning in the context of image classification. The merits of the proposed model are threefold. First, A-DLC is the first-ever method that alternatively cascades the sparse and collaborative representations using the class-discriminant softmax vector representation at the interface of each cascade section so that the sparsity and collaborativity can simultaneously be considered. Second, A-DLC inherits the hierarchy learning capability that effectively extends the traditional shallow sparse coding to a multi-layer learning model, thus enabling a full exploitation of the inherent latent discriminative information. Third, the simulation results show a significant amelioration in the classification accuracy, compared to earlier one-step single-layer classification algorithms. The Matlab code of this paper is available at https://github.com/chenzhe207/A-DLC .
We propose a novel end-to-end semi-supervised adversarial framework to generate photorealistic face images of new identities with a wide range of expressions, poses, and illuminations conditioned by synthetic images sampled from a 3D morphable model. Previous adversarial style-transfer methods either supervise their networks with a large volume of paired data or train highly under-constrained two-way generative networks in an unsupervised fashion. We propose a semi-supervised adversarial learning framework to constrain the two-way networks by a small number of paired real and synthetic images, along with a large volume of unpaired data. A set-based loss is also proposed to preserve identity coherence of generated images. Qualitative results show that generated face images of new identities contain pose, lighting and expression diversity. They are also highly constrained by the synthetic input images while adding photorealism and retaining identity information. We combine face images generated by the proposed method with a real data set to train face recognition algorithms and evaluate the model quantitatively on two challenging data sets: LFW and IJB-A. The generated images by our framework consistently improve the performance of deep face recognition networks trained with the Oxford VGG Face dataset, and achieve comparable results to the state-of-the-art.
In the image fusion field, the design of deep learning-based fusion methods is far from routine. It is invariably fusion-task specific and requires a careful consideration. The most difficult part of the design is to choose an appropriate strategy to generate the fused image for a specific task in hand. Thus, devising learnable fusion strategy is a very challenging problem in the community of image fusion. To address this problem, a novel end-to-end fusion network architecture (RFN-Nest) is developed for infrared and visible image fusion. We propose a residual fusion network (RFN) which is based on a residual architecture to replace the traditional fusion approach. A novel detail-preserving loss function, and a feature enhancing loss function are proposed to train RFN. The fusion model learning is accomplished by a novel two-stage training strategy. In the first stage, we train an auto-encoder based on an innovative nest connection (Nest) concept. Next, the RFN is trained using the proposed loss functions. The experimental results on public domain data sets show that, compared with the existing methods, our end-to-end fusion network delivers a better performance than the state-of-the-art methods in both subjective and objective evaluation. The code of our fusion method is available at https://github.com/hli1221/imagefusion-rfn-nest. •Residual fusion network (RFN) is proposed to supersede handcrafted fusion strategies.•Two-stage training strategy is developed to train RFN.•Detail preservation and feature enhancement loss functions are designed.•The proposed method achieves better performance compare with existing methods.
How to extract and integrate spatiotemporal information for video classification is a major challenge. Advanced approaches adopt 2D, and 3D convolution kernels, or their variants as a basis of a spatiotemporal modeling process. However, 2D convolution kernels perform poorly along the temporal dimension, while 3D convolution kernels tend to create confusion between the spatial and temporal sources of information, with an increased risk of explosion of the number of model parameters. In this paper, we develop a more explicit way to improve the spatiotemporal modeling capacity of a 2D convolution network, which integrates two components: (1) Using Motion Intensification Block (MIB) to mandate a specific subset of channels to explicitly encode temporal clues to complement the spatial patterns extracted by other channels, achieving controlled diversity in the convolution calculations. (2) Using Spatial-temporal Squeeze-and-excitation (ST-SE) block to intensify the fused features reflecting the importance of different channels. In this manner, we improve the spatiotemporal dynamic information within the 2D backbone network, without performing complex temporal convolutions. To verify the effectiveness of the proposed approach, we conduct extensive experiments on challenging benchmarks. Our model achieves a competitive result on Something-Something V1, Something-Something V2, and a state-of-the-art performance on the Diving48 dataset, providing supporting evidence for the merits of the proposed methodology of spatiotemporal information encoding and fusion for video classification. (c) 2021 Published by Elsevier B.V.
With the advantages of low storage cost and high retrieval efficiency, hashing techniques have recently been an emerging topic in cross-modal similarity search. As multiple modal data reflect similar semantic content, many works aim at learning unified binary codes. However, discriminative hashing features learned by these methods are not adequate. This results in lower accuracy and robustness. We propose a novel hashing learning framework which jointly performs classifier learning, subspace learning, and matrix factorization to preserve class-specific semantic content, termed Discriminative Supervised Hashing (DSH), to learn the discriminative unified binary codes for multi-modal data. Besides, reducing the loss of information and preserving the non-linear structure of data, DSH non-linearly projects different modalities into the common space in which the similarity among heterogeneous data points can be measured. Extensive experiments conducted on the three publicly available datasets demonstrate that the framework proposed in this paper outperforms several state-of-the-art methods.
We present a new loss function, namely Wing loss, for robust facial landmark localisation with Convolutional Neural Networks (CNNs). We first compare and analyse different loss functions including L2, L1 and smooth L1. The analysis of these loss functions suggests that, for the training of a CNN-based localisation model, more attention should be paid to small and medium range errors. To this end, we design a piece-wise loss function. The new loss amplifies the impact of errors from the interval (-w, w) by switching from L1 loss to a modified logarithm function. To address the problem of under-representation of samples with large out-of-plane head rotations in the training set, we propose a simple but effective boosting strategy, referred to as pose-based data balancing. In particular, we deal with the data imbalance problem by duplicating the minority training samples and perturbing them by injecting random image rotation, bounding box translation and other data augmentation approaches. Last, the proposed approach is extended to create a two-stage framework for robust facial landmark localisation. The experimental results obtained on AFLW and 300W demonstrate the merits of the Wing loss function, and prove the superiority of the proposed method over the state-of-the-art approaches.
3D face alignment of monocular images is a crucial process in the recognition of faces with disguise.3D face reconstruction facilitated by alignment can restore the face structure which is helpful in detcting disguise interference.This paper proposes a dual attention mechanism and an efficient end-to-end 3D face alignment framework. We build a stable network model through Depthwise Separable Convolution, Densely Connected Convolutional and Lightweight Channel Attention Mechanism. In order to enhance the ability of the network model to extract the spatial features of the face region, we adopt Spatial Group-wise Feature enhancement module to improve the representation ability of the network. Different loss functions are applied jointly to constrain the 3D parameters of a 3D Morphable Model (3DMM) and its 3D vertices. We use a variety of data enhancement methods and generate large virtual pose face data sets to solve the data imbalance problem. The experiments on the challenging AFLW,AFLW2000-3D datasets show that our algorithm significantly improves the accuracy of 3D face alignment. Our experiments using the field DFW dataset show that DAMDNet exhibits excellent performance in the 3D alignment and reconstruction of challenging disguised faces.The model parameters and the complexity of the proposed method are also reduced significantly.
The availability of large scale data with high quality ground truth labels is a challenge when developing supervised machine learning solutions for healthcare domain. Although, the amount of digital data in clinical workflows is increasing, most of this data is distributed on clinical sites and protected to ensure patient privacy. Radiological readings and dealing with large-scale clinical data puts a significant burden on the available resources, and this is where machine learning and artificial intelligence play a pivotal role. Magnetic Resonance Imaging (MRI) for musculoskeletal (MSK) diagnosis is one example where the scans have a wealth of information, but require a significant amount of time for reading and labeling. Self-supervised learning (SSL) can be a solution for handling the lack of availability of ground truth labels, but generally requires a large amount of training data during the pretraining stage. Herein, we propose a slice-based self-supervised deep learning framework (SB-SSL), a novel slice-based paradigm for classifying abnormality using knee MRI scans. We show that for a limited number of cases (
Recently, deep learning has become a rapidly developing tool in the field of image fusion. An innovative image fusion method for fusing infrared images and visible-light images is proposed. The backbone network is an autoencoder. Different from previous autoencoders, the information extraction capability of the encoder is enhanced, and the ability to select the most effective channels in the decoder is optimized. First, the features of the source image are extracted during the encoding process. Then, a new effective fusion strategy is designed to fuse these features. Finally, the fused image is reconstructed by the decoder. Compared with the existing fusion methods, the proposed algorithm achieves state-of-the-art performance in both objective evaluation and visual quality.
•We develop a solution for the face spoofing detection problem by fusing multiple anomaly experts using Weighted Averaging(WA).•We propose a novel three-stage optimisation approach to improve the generalisation capability and accuracy of the WA fusion.•We define a new score normalisation approach to support multiple anomaly detectors fusion.•We define an effective criterion to prune the WA to achieve better classification result and generalisation performance.•We experimentally demonstrate that the proposed anomaly-based WA achieves superior performance over state-of-theart methods. Despite the recent improvements in facial recognition, face spoofing attacks can still pose a serious security threat to biometric systems. As fraudsters are coming up with novel spoofing attacks, anomaly-based detectors, compared to the binary spoofing attack counterparts, have certain generalisation performance advantages. In this work, we investigate the merits of fusing multiple anomaly classifiers using weighted averaging (WA) fusion. The design of the entire system is based on genuine-access data only. To optimise the parameters of WA, we propose a novel three-stage optimisation method with the following contributions: (a) A new hybrid optimisation method using Genetic Algorithm (GA) and Pattern Search (PS) to explore the weight space more effectively (b) a novel two-sided score normalisation method to improve the anomaly detection performance (c) a new ensemble pruning method to improve the generalisation performance. To further boost the performance of the proposed anomaly detection ensemble, we incorporate client-specific information to train the proposed model. We evaluate the capability of the proposed model on publicly available face spoofing databases including Replay-Attack, Replay-Mobile and Rose-Youtu. The experimental results demonstrate that the proposed WA fusion outperforms the state-of-the-art anomaly-based and multiclass approaches.
Performing pattern analysis over the symmetric positive definite (SPD) manifold requires specific mathematical computations, characterizing the non-Euclidian property of the involved data points and learning tasks, such as the image set classification problem. Accompanied with the advanced neural networking techniques, several architectures for processing the SPD matrices have recently been studied to obtain fine-grained structured representations. However, existing approaches are challenged by the diversely changing appearance of the data points, begging the question of how to learn invariant representations for improved performance with supportive theories. Therefore, this paper designs two Riemannian operation modules for SPD manifold neural network. Specifically, a Riemannian batch regularization (RBR) layer is firstly proposed for the purpose of training a discriminative manifold-to-manifold transforming network with a novelly-designed metric learning regularization term. The second module realizes the Riemannian pooling operation with geometric computations on the Riemannian manifolds, notably the Riemannian barycenter, metric learning, and Riemannian optimization. Extensive experiments on five benchmarking datasets show the efficacy of the proposed approach.(C)& nbsp; 2022 Published by Elsevier Ltd.
As human action is a spatial–temporal process, modern action recognition research has focused on exploring more effective motion representations, rather than only taking human poses as input. To better model a motion pattern, in this paper, we exploit the rotation information to depict the spatial–temporal variation, thus enhancing the dynamic appearance, as well as forming a complementary component with the static coordinates of the joints. Specifically, we design to represent the movement of human body with joint units, consisting of performing regrouping human joints together with the adjacent two bones. Therefore, the rotation descriptors reduce the impact from the static values while focus on the dynamic movement. The proposed general features can be simply applied to existing CNN-based action recognition methods. The experimental results performed on NTU-RGB+D and ICL First Person Handpose datasets demonstrate the advantages of the proposed method.
In this paper, we propose a non-negative representation based discriminative dictionary learning algorithm (NRDL) for multi-category face classification. In contrast to traditional dictionary learning methods, NRDL investigates the use of non-negative representation (NR), which contributes to learning discriminative dictionary atoms. In order to make the learned dictionary more suitable for classification, NRDL seamlessly incorporates non-negative representation constraint, discriminative dictionary learning and linear classifier training into a unified model. Specifically, NRDL introduces a positive constraint on representation matrix to find distinct atoms from heterogeneous training samples, which results in sparse and discriminative representation. Moreover, a discriminative dictionary encouraging function is proposed to enhance the uniqueness of class-specific sub-dictionaries. Meanwhile, an inter-class incoherence constraint and a compact graph based regularization term are constructed to respectively improve the discriminability of learned classifier. Experimental results on several benchmark face data sets verify the advantages of our NRDL algorithm over the state-of-the-art dictionary learning methods.
Deep neural networks have enhanced the performance of decision making systems in many applications, including image understanding, and further gains can be achieved by constructing ensembles. However, designing an ensemble of deep networks is often not very beneficial since the time needed to train the networks is generally very high or the performance gain obtained is not very significant. In this paper, we analyse an error correcting output coding (ECOC) framework for constructing ensembles of deep networks and propose different design strategies to address the accuracy-complexity trade-off. We carry out an extensive comparative study between the introduced ECOC designs and the state-of-the-art ensemble techniques such as ensemble averaging and gradient boosting decision trees. Furthermore, we propose a fusion technique, that is shown to achieve the highest classification performance.
By representing each image set as a nonsingular covariance matrix on the symmetric positive definite (SPD) manifold, visual classification with image sets has attracted much attention. Despite the success made so far, the issue of large within-class variability of representations still remains a key challenge. Recently, several SPD matrix learning methods have been proposed to assuage this problem by directly constructing an embedding mapping from the original SPD manifold to a lower dimensional one. The advantage of this type of approach is that it cannot only implement discriminative feature selection but also preserve the Riemannian geometrical structure of the original data manifold. Inspired by this fact, we propose a simple SPD manifold deep learning network (SymNet) for image set classification in this article. Specifically, we first design SPD matrix mapping layers to map the input SPD matrices into new ones with lower dimensionality. Then, rectifying layers are devised to activate the input matrices for the purpose of forming a valid SPD manifold, chiefly to inject nonlinearity for SPD matrix learning with two nonlinear functions. Afterward, we introduce pooling layers to further compress the input SPD matrices, and the log-map layer is finally exploited to embed the resulting SPD matrices into the tangent space via log-Euclidean Riemannian computing, such that the Euclidean learning applies. For SymNet, the (2-D) 2 principal component analysis (PCA) technique is utilized to learn the multistage connection weights without requiring complicated computations, thus making it be built and trained easier. On the tail of SymNet, the kernel discriminant analysis (KDA) algorithm is coupled with the output vectorized feature representations to perform discriminative subspace learning. Extensive experiments and comparisons with state-of-the-art methods on six typical visual classification tasks demonstrate the feasibility and validity of the proposed SymNet.
Among existing clustering methods, sparse subspace clustering (SSC) obtains superior clustering performance in grouping data points from a union of subspaces by solving a relaxed 0-minimization problem by 1-norm. The use of 1-norm instead of the 0 one can make the object function convex, while it also causes large errors on large coefficients in some cases. In this work, we propose using the nonconvex approximation to replace 0-norm for SSC, termed as SSC via nonconvex approximation (SSCNA), and develop a novel clustering algorithm with the enhanced sparsity based on the Alternating Direction Method of Multipliers. We further prove that the proposed nonconvex approximation is closer to 0-norm than the 1 one and is bounded by 0-norm. Numerical studies show that the proposed nonconvex approximation helps to improve clustering performance. We also theoretically verify the convergence of the proposed algorithm with a three-variable objective function. Extensive experiments on four benchmark datasets demonstrate the effectiveness of the proposed method.
The improved generative adversarial network (improved GAN) is a successful method using a generative adversarial model to solve the problem of semi-supervised learning (SSL). The improved GAN learns a generator with the technique of mean feature matching which penalizes the discrepancy of the first-order moment of the latent features. To better describe common attributes of a distribution, this paper proposes a novel SSL method which incorporates the first-order and the secondorder moments of the features in an intermediate layer of the discriminator, called mean and variance feature matching GAN (MVFM-GAN). To capture more precisely the data manifold, not only the mean but also the variance is used in the latent feature learning. Compared with improved GAN and other traditional methods, MVFM-GAN achieves superior performance in semi-supervised classification tasks and a better stability of GAN training, particularly in the cases when the number of labeled samples is low. It shows a comparable performance with the state-of-the-art methods on several benchmark data sets. As a byproduct of the novel approach, MVFM-GAN generates realistic images of good visual quality.
In recent years, cross-media hashing technique has attracted increasing attention for its high computation efficiency and low storage cost. However, the existing approaches still have some limitations, which need to be explored. (1) A fixed hash length (e.g., 16bits or 32bits) is predefined before learning the binary codes. Therefore, these models need to be retrained when the hash length changes, that consumes additional computation power, reducing the scalability in practical applications. (2) Existing cross-modal approaches only explore the information in the original multimedia data to perform the hash learning, without exploiting the semantic information contained in the learned hash codes. To this end, we develop a novel Multiple hash cOdes jOint learNing method (MOON) for cross-media retrieval. Specifically, the developed MOON simultaneously learns the hash codes with multiple lengths in a unified framework. Besides, to enhance the underlying discrimination, we combine the clues from the multimodal data, semantic label and learned hash codes for hash learning. As far as we know, the proposed MOON is the first attempt to simultaneously learn different length hash codes without retraining in cross-media retrieval. Experiments on several databases show that our MOON can achieve promising performance, outperforming some recent competitive shallow and deep methods. (c) 2021 Published by Elsevier B.V.
In recent years, deep-learning-based face detectors have achieved promising results and been successfully used in a wide range of practical applications. However, extreme appearance variations are still the major obstacles for robust and accurate face detection in the wild. To address this issue, we propose an Improved Training Sample Selection (ITSS) strategy for mining effective positive and negative samples during network training. The proposed ITSS procedure collaborates with face sampling during data augmentation and selects suitable positive sample centres and IoU overlap for face detection. Moreover, we propose a Residual Feature Pyramid Fusion (RFPF) module that collects semantically robust features to improve the scale-invariance of deep features and better represent faces at different feature pyramid levels. The experimental results obtained on the FDDB and WiderFace datasets demonstrate the superiority of the proposed method over the state-of-the-art approaches. Specially, the proposed method achieves 96.9% and 96.2% in terms of AP on the easy and medium test sets of WiderFace.
In recent years, deep learning has become a very active research tool which is used in many image processing fields. In this paper, we propose an effective image fusion method using a deep learning framework to generate a single image which contains all the features from infrared and visible images. First, the source images are decomposed into base parts and detail content. Then the base parts are fused by weighted-averaging. For the detail content, we use a deep learning network to extract multi-layer features. Using these features, we use l(1)-norm and weighted-average strategy to generate several candidates of the fused detail content. Once we get these candidates, the max selection strategy is used to get the final fused detail content. Finally, the fused image will be reconstructed by combining the fused base part and the detail content. The experimental results demonstrate that our proposed method achieves state-of-the-art performance in both objective assessment and visual quality. The Code of our fusion method is available at https: //github.com/exceptionLi/imagefusiondeeplearning.
—Label distribution Learning (LDL) is the state-of-the-art approach to deal with a number of real-world applications , such as chronological age estimation from a face image, where there is an inherent similarity among adjacent age labels. LDL takes into account the semantic similarity by assigning a label distribution to each instance. The well-known Kullback–Leibler (KL) divergence is the widely used loss function for the LDL framework. However, the KL divergence does not fully and effectively capture the semantic similarity among age labels, thus leading to the sub-optimal performance. In this paper, we propose a novel loss function based on optimal transport theory for the LDL-based age estimation. A ground metric function plays an important role in the optimal transport formulation. It should be carefully determined based on underlying geometric structure of the label space of the application in-hand. The label space in the age estimation problem has a specific geometric structure, i.e. closer ages have more inherent semantic relationship. Inspired by this, we devise a novel ground metric function, which enables the loss function to increase the influence of highly correlated ages; thus exploiting the semantic similarity among ages more effectively than the existing loss functions. We then use the proposed loss function, namely γ–Wasserstein loss, for training a deep neural network (DNN). This leads to a notoriously computationally expensive and non-convex optimisa-tion problem. Following the standard methodology, we formulate the optimisation function as a convex problem and then use an efficient iterative algorithm to update the parameters of the DNN. Extensive experiments in age estimation on different benchmark datasets validate the effectiveness of the proposed method, which consistently outperforms state-of-the-art approaches.
3D assisted 2D face recognition involves the process of reconstructing 3D faces from 2D images and solving the problem of face recognition in 3D. To facilitate the use of deep neural networks, a 3D face, normally represented as a 3D mesh of vertices and its corresponding surface texture, is remapped to image-like square isomaps by a conformal mapping. Based on previous work, we assume that face recognition benefits more from texture. In this work, we focus on the surface texture and its discriminatory information content for recognition purposes. Our approach is to prepare a 3D mesh, the corresponding surface texture and the original 2D image as triple input for the recognition network, to show that 3D data is useful for face recognition. Texture enhancement methods to control the texture fusion process are introduced and we adapt data augmentation methods. Our results show that texture-map-based face recognition can not only compete with state-of-the-art systems under the same preconditions but also outperforms standard 2D methods from recent years.
We propose a new Group Feature Selection method for Discriminative Correlation Filters (GFS-DCF) based visual object tracking. The key innovation of the proposed method is to perform group feature selection across both channel and spatial dimensions, thus to pinpoint the structural relevance of multi-channel features to the filtering system. In contrast to the widely used spatial regularisation or feature selection methods, to the best of our knowledge, this is the first time that channel selection has been advocated for DCF-based tracking. We demonstrate that our GFS-DCF method is able to significantly improve the performance of a DCF tracker equipped with deep neural network features. In addition, our GFS-DCF enables joint feature selection and filter learning, achieving enhanced discrimination and interpretability of the learned filters. To further improve the performance, we adaptively integrate historical information by constraining filters to be smooth across temporal frames, using an efficient low-rank approximation. By design, specific temporal-spatial-channel configurations are dynamically learned in the tracking process, highlighting the relevant features, and alleviating the performance degrading impact of less discriminative representations and reducing information redundancy. The experimental results obtained on OTB2013, OTB2015, VOT2017, VOT2018 and TrackingNet demonstrate the merits of our GFS-DCF and its superiority over the state-of-the-art trackers. The code is publicly available at \url{https://github.com/XU-TIANYANG/GFS-DCF}.
Siamese trackers have become the mainstream framework for visual object tracking in recent years. However, the extraction of the template and search space features is disjoint for a Siamese tracker, resulting in a limited interaction between its classification and regression branches. This degrades the model capacity accurately to estimate the target, especially when it exhibits severe appearance variations. To address this problem, this paper presents a target-cognisant Siamese network for robust visual tracking. First, we introduce a new target-cognisant attention block that computes spatial cross-attention between the template and search branches to convey the relevant appearance information before correlation. Second, we advocate two mechanisms to promote the precision of obtained bounding boxes under complex tracking scenarios. Last, we propose a max filtering module to utilise the guidance of the regression branch to filter out potential interfering predictions in the classification map. The experimental results obtained on challenging benchmarks demonstrate the competitive performance of the proposed method.
•A formulation of the DCF design problem which focuses on informative feature channels and spatial structures by means of novel regularisation.•A proposed relaxed optimisation algorithm referred to as R_A-ADMM for optimising the regularised DCF. In contrast with the standard ADMM, the algorithm achieves a better convergence rate.•A temporal smoothness constraint, implemented by an adaptive initialisation mechanism, to achieve further speed up via transfer learning among video frames.•The proposed adoption of AlexNet to construct a light-weight deep representation with a tracking accuracy comparable to more complicated deep networks, such as VGG and ResNet.•An extensive evaluation of the proposed methodology on several well-known visual object tracking datasets, with the results confirming the acceleration gains for the regularised DCF paradigm.
Advanced Siamese visual object tracking architectures are jointly trained using pair-wise input images to perform target classification and bounding box regression. They have achieved promising results in recent benchmarks and competitions. However, the existing methods suffer from two limitations: First, though the Siamese structure can estimate the target state in an instance frame, provided the target appearance does not deviate too much from the template, the detection of the target in an image cannot be guaranteed in the presence of severe appearance variations. Second, despite the classification and regression tasks sharing the same output from the backbone network, their specific modules and loss functions are invariably designed independently, without promoting any interaction. Yet, in a general tracking task, the centre classification and bounding box regression tasks are collaboratively working to estimate the final target location. To address the above issues, it is essential to perform target-agnostic detection so as to promote cross-task interactions in a Siamese-based tracking framework. In this work, we endow a novel network with a target-agnostic object detection module to complement the direct target inference, and to avoid or minimise the misalignment of the key cues of potential template-instance matches. To unify the multi-task learning formulation, we develop a cross-task interaction module to ensure consistent supervision of the classification and regression branches, improving the synergy of different branches. To eliminate potential inconsistencies that may arise within a multi-task architecture, we assign adaptive labels, rather than fixed hard labels, to supervise the network training more effectively. The experimental results obtained on several benchmarks, i.e ., OTB100, UAV123, VOT2018, VOT2019, and LaSOT, demonstrate the effectiveness of the advanced target detection module, as well as the cross-task interaction, exhibiting superior tracking performance as compared with the state-of-the-art tracking methods.
Discriminative Correlation Filters (DCF) have been shown to achieve impressive performance in visual object tracking. However, existing DCF-based trackers rely heavily on learning regularised appearance models from invariant image feature representations. To further improve the performance of DCF in accuracy and provide a parsimonious model from the attribute perspective, we propose to gauge the relevance of multi-channel features for the purpose of channel selection. This is achieved by assessing the information conveyed by the features of each channel as a group, using an adaptive group elastic net inducing independent sparsity and temporal smoothness on the DCF solution. The robustness and stability of the learned appearance model are significantly enhanced by the proposed method as the process of channel selection performs implicit spatial regularisation. We use the augmented Lagrangian method to optimise the discriminative filters efficiently. The experimental results obtained on a number of well-known benchmarking datasets demonstrate the effectiveness and stability of the proposed method. A superior performance over the state-of-the-art trackers is achieved using less than $$10\%$$ 10 % deep feature channels.
We propose a new Group Feature Selection method for Discriminative Correlation Filters (GFS-DCF) based visual object tracking. The key innovation of the proposed method is to perform group feature selection across both channel and spatial dimensions, thus to pinpoint the structural relevance of multi-channel features to the filtering system. In contrast to the widely used spatial regularisation or feature selection methods, to the best of our knowledge, this is the first time that channel selection has been advocated for DCF-based tracking. We demonstrate that our GFS-DCF method is able to significantly improve the performance of a DCF tracker equipped with deep neural network features. In addition, our GFS-DCF enables joint feature selection and filter learning, achieving enhanced discrimination and interpretability of the learned filters. To further improve the performance, we adaptively integrate historical information by constraining filters to be smooth across temporal frames, using an efficient low-rank approximation. By design, specific temporal-spatial-channel configurations are dynamically learned in the tracking process, highlighting the relevant features, and alleviating the performance degrading impact of less discriminative representations and reducing information redundancy. The experimental results obtained on OTB2013, OTB2015, VOT2017, VOT2018 and TrackingNet demonstrate the merits of our GFS-DCF and its superiority over the state-of-the-art trackers. The code is publicly available at https://github.com/XU-TIANYANG/GFS-DCF.
Recently, the security of multimodal verification has become a grow-ing concern since many fusion systems have been known to be easily deceived by partial spoof attacks, i.e. only a subset of modalities is spoofed. In this paper, we verify such a vulnerability and propose to use two representation-based met-rics to close this gap. Firstly, we use the collaborative representation fidelity with non-target subjects to measure the affinity of a query sample to the claimed client. We further consider sparse coding as a competing comparison among the client and the non-target subjects, and hence explore two sparsity-based measures for recognition. Last, we select the representation-based measure, and assemble its score and the affinity score of each modality to train a support vector machine classifier. Our experimental results on a chimeric multimodal database with face and ear traits demonstrate that in both regular verification and partial spoof at-tacks, the proposed method significant
We present a new Cascaded Shape Regression (CSR) architecture, namely Dynamic Attention-Controlled CSR (DAC-CSR), for robust facial landmark detection on unconstrained faces. Our DAC-CSR divides facial landmark detection into three cascaded sub-tasks: face bounding box refinement, general CSR and attention-controlled CSR. The first two stages refine initial face bounding boxes and output intermediate facial landmarks. Then, an online dynamic model selection method is used to choose appropriate domain-specific CSRs for further landmark refinement. The key innovation of our DAC-CSR is the fault-tolerant mechanism, using fuzzy set sample weighting, for attentioncontrolled domain-specific model training. Moreover, we advocate data augmentation with a simple but effective 2D profile face generator, and context-aware feature extraction for better facial feature representation. Experimental results obtained on challenging datasets demonstrate the merits of our DAC-CSR over the state-of-the-art methods.
As mobile devices are becoming more ubiquitous, it is now possible to enhance the security of the phone, as well as remote services requiring identity verification, by means of biometric traits such as fingerprint and speech. We refer to this as mobile biometry. The objective of this study is to increase the usability of mobile biometry for visually impaired users, using face as biometrics. We illustrate a scenario of a person capturing his/her own face images which are as frontal as possible. This is a challenging task for the following reasons. Firstly, a greater variation in head pose and degradation in image quality (e.g., blur, de-focus) is expected due to the motion introduced by the hand manipulation and unsteadiness. Second, for the visually impaired users, there currently exists no mechanism to provide feedback on whether a frontal face image is detected. In this paper, an audio feedback mechanism is proposed to assist the visually impaired to acquire face images of better quality. A preliminary user study suggests that the proposed audio feedback can potentially (a) shorten the acquisition time and (b) improve the success rate of face detection, especially for the non-sighted users.
The probability hypothesis density (PHD) filter based on sequential Monte Carlo (SMC) approximation (also known as SMC-PHD filter) has proven to be a promising algorithm for multi-speaker tracking. However, it has a heavy computational cost as surviving, spawned and born particles need to be distributed in each frame to model the state of the speakers and to estimate jointly the variable number of speakers with their states. In particular, the computational cost is mostly caused by the born particles as they need to be propagated over the entire image in every frame to detect the new speaker presence in the view of the visual tracker. In this paper, we propose to use audio data to improve the visual SMC-PHD (VSMC- PHD) filter by using the direction of arrival (DOA) angles of the audio sources to determine when to propagate the born particles and re-allocate the surviving and spawned particles. The tracking accuracy of the AV-SMC-PHD algorithm is further improved by using a modified mean-shift algorithm to search and climb density gradients iteratively to find the peak of the probability distribution, and the extra computational complexity introduced by mean-shift is controlled with a sparse sampling technique. These improved algorithms, named as AVMS-SMCPHD and sparse-AVMS-SMC-PHD respectively, are compared systematically with AV-SMC-PHD and V-SMC-PHD based on the AV16.3, AMI and CLEAR datasets.
This paper proposes a new algorithm for fitting a 3D morphable face model on low-resolution (LR) facial images. We analyse the criterion commonly used by the main fitting algorithms and by comparing with an image formation model, show that this criterion is only valid if the resolution of the input image is high. We then derive an imaging model to describe the process of LR image formation given the 3D model. Finally, we use this imaging model to improve the fitting criterion. Experimental results show that our algorithm significantly improves fitting results on LR images and yields similar parameters to those that would have been obtained if the input image had a higher resolution. We also show that our algorithm can be used for face recognition in low-resolutions where the conventional fitting algorithms fail.
The application of biometric technology has so far been top-down, driven by governments and law enforcement agencies. The low demand of this technology from the public, despite its many advantages compared to the traditional means of authentication is probably due to the lack of human factor considerations in the design process. In this work, we propose a guideline to design an interactive quality-driven feedback mechanism. The mechanism aims to improve the quality of biométrie samples during the acquisition process by putting in place objective assessment of the quality and feeding this information back to the user instantaneously, thus eliminating subjective quality judgement by the user. We illustrate the feasibility of the design methodology using face recognition as a case study. Preliminary results show that the methodology can potentially increase efficiency, effectiveness and accessibility of a biométrie system.
Automation of HEp-2 cell pattern classification would drastically improve the accuracy and throughput of diagnostic services for many auto-immune diseases, but it has proven difficult to reach a sufficient level of precision. Correct diagnosis relies on a subtle assessment of texture type in microscopic images of indirect immunofluorescence (IIF), which so far has eluded reliable replication through automated measurements. We introduce a combination of spectral analysis and multiscale digital filtering to extract the most discriminative variables from the cell images. We also apply multistage classification techniques to make optimal use of the limited labelled data set. Overall error rate of 1.6% is achieved in recognition of 6 different cell patterns, which drops to 0.5% if only positive samples are considered.
Particle filtering has emerged as a useful tool for tracking problems. However, the efficiency and accuracy of the filter usually depend on the number of particles and noise variance used in the estimation and propagation functions for re-allocating these particles at each iteration. Both of these parameters are specified beforehand and are kept fixed in the regular implementation of the filter which makes the tracker unstable in practice. In this paper we are interested in the design of a particle filtering algorithm which is able to adapt the number of particles and noise variance. The new filter, which is based on audio-visual (AV) tracking, uses information from the tracking errors to modify the number of particles and noise variance used. Its performance is compared with a previously proposed audio-visual particle filtering algorithm with a fixed number of particles and an existing adaptive particle filtering algorithm, using the AV 16.3 dataset with single and multi-speaker sequences. Our proposed approach demonstrates good tracking performance with a significantly reduced number of particles. © 2013 EURASIP.
Multiple Kernel Learning (MKL) has become a preferred choice for information fusion in image recognition problem. Aim of MKL is to learn optimal combination of kernels formed from different features, thus, to learn importance of different feature spaces for classification. Augmented Kernel Matrix (AKM) has recently been proposed to accommodate for the fact that a single training example may have different importance in different feature spaces, in contrast to MKL that assigns same weight to all examples in one feature space. However, AKM approach is limited to small datasets due to its memory requirements. We propose a novel two stage technique to make AKM applicable to large data problems. In first stage various kernels are combined into different groups automatically using kernel alignment. Next, most influential training examples are identified within each group and used to construct an AKM of significantly reduced size. This reduced size AKM leads to same results as the original AKM. We demonstrate that proposed two stage approach is memory efficient and leads to better performance than original AKM and is robust to noise. Results are compared with other state-of-the art MKL techniques, and show improvement on challenging object recognition benchmarks.
In previous work, we developed a novel data association algorithm with graph-theoretic formulation, and used it to track a tennis ball in broadcast tennis video. However, the track initiation/termination was not automatic, and it could not deal with situations in which more than one ball appeared in the scene. In this paper, we extend our previous work to track multiple tennis balls fully automatically. The algorithm presented in this paper requires the set of all-pairs shortest paths in a directed and edge-weighted graph. We also propose an efficient All-Pairs Shortest Path algorithm by exploiting a special topological property of the graph. Comparative experiments show that the proposed data association algorithm performs well both in terms of efficiency and tracking accuracy.
Bags-of-visual-Words (BoW) and Spatio-Temporal Shapes (STS) are two very popular approaches for action recognition from video. The former (BoW) is an un-structured global representation of videos which is built using a large set of local features. The latter (STS) uses a single feature located on a region of interest (where the actor is) in the video. Despite the popularity of these methods, no comparison between them has been done. Also, given that BoW and STS differ intrinsically in terms of context inclusion and globality/locality of operation, an appropriate evaluation framework has to be designed carefully. This paper compares these two approaches using four different datasets with varied degree of space-time specificity of the actions and varied relevance of the contextual background. We use the same local feature extraction method and the same classifier for both approaches. Further to BoW and STS, we also evaluated novel variations of BoW constrained in time or space. We observe that the STS approach leads to better results in all datasets whose background is of little relevance to action classification.
Multiple Kernel Learning (MKL) has become a preferred choice for information fusion in image recognition problem. Aim of MKL is to learn optimal combination of kernels formed from different features, thus, to learn importance of different feature spaces for classification. Augmented Kernel Matrix (AKM) has recently been proposed to accommodate for the fact that a single training example may have different importance in different feature spaces, in contrast to MKL that assigns same weight to all examples in one feature space. However, AKM approach is limited to small datasets due to its memory requirements. We propose a novel two stage technique to make AKM applicable to large data problems. In first stage various kernels are combined into different groups automatically using kernel alignment. Next, most influential training examples are identified within each group and used to construct an AKM of significantly reduced size. This reduced size AKM leads to same results as the original AKM. We demonstrate that proposed two stage approach is memory efficient and leads to better performance than original AKM and is robust to noise. Results are compared with other state-of-the art MKL techniques, and show improvement on challenging object recognition benchmarks.
In practical applications of pattern recognition and computer vision, the performance of many approaches can be improved by using multiple models. In this paper, we develop a common theoretical framework for multiple model fusion at the feature level using multilinear subspace analysis (also known as tensor algebra). One disadvantage of the multilinear approach is that it is hard to obtain enough training observations for tensor decomposition algorithms. To overcome this difficulty, we adopted the M$^2$SA algorithm to reconstruct the missing entries of the incomplete training tensor. Furthermore, we apply the proposed framework to the problem of face image analysis using Active Appearance Model (AAM) to validate its performance. Evaluations of AAM using the proposed framework are conducted on Multi-PIE face database with promising results.
Significant improvements in face-recognition performance have recently been achieved by obtaining near infrared (NIR) probe images. We demonstrate that by taking into account the differential effects of sub-surface scattering, correlation between facial images in the visible (VIS) and NIR wavelengths can be significantly improved. Hence, by using Fourier analysis and Gaussian deconvolution with variable thresholds for the scattering deconvolution radius and frequency, sub-surface scattering effects are largely eliminated from perpendicular isomap transformations of the facial images. (Isomap images are obtained via scanning reconstruction, as in our case, or else, more generically, via model fitting). Thus, small-scale features visible in both the VIS and NIR, such as skin-pores and certain classes of skin-mottling, can be equally weighted within the correlation analysis. The method can consequently serves as the basis for more detailed forms of facial comparison
The use of non-negative matrix factorisation (NMF) on 2D face images has been shown to result in sparse feature vectors that encode for local patches on the face, and thus provides a statistically justified approach to learning parts from wholes. However successful on 2D images, the method has so far not been extended to 3D images. The main reason for this is that 3D space is a continuum and so it is not apparent how to represent 3D coordinates in a non-negative fashion. This work compares different non-negative representations for spatial coordinates, and demonstrates that not all non-negative representations are suitable. We analyse the representational properties that make NMF a successful method to learn sparse 3D facial features. Using our proposed representation, the factorisation results in sparse and interpretable facial features.
This report presents results from the Video Person Recognition Evaluation held in conjunction with the 11th IEEE International Conference on Automatic Face and Gesture Recognition. Two experiments required algorithms to recognize people in videos from the Point-and-Shoot Face Recognition Challenge Problem (PaSC). The first consisted of videos from a tripod mounted high quality video camera. The second contained videos acquired from 5 different handheld video cameras. There were 1401 videos in each experiment of 265 subjects. The subjects, the scenes, and the actions carried out by the people are the same in both experiments. Five groups from around the world participated in the evaluation. The video handheld experiment was included in the International Joint Conference on Biometrics (IJCB) 2014 Handheld Video Face and Person Recognition Competition. The top verification rate from this evaluation is double that of the top performer in the IJCB competition. Analysis shows that the factor most effecting algorithm performance is the combination of location and action: where the video was acquired and what the person was doing.
In this paper we formulate multiple kernel learning (MKL) as a distance metric learning (DML) problem. More specifically, we learn a linear combination of a set of base kernels by optimising two objective functions that are commonly used in distance metric learning. We first propose a global version of such an MKL via DML scheme, then a localised version. We argue that the localised version not only yields better performance than the global version, but also fits naturally into the framework of example based retrieval and relevance feedback. Finally the usefulness of the proposed schemes are verified through experiments on two image retrieval datasets.
In this paper we describe our TRECVID 2008 video retrieval experiments. The MediaMill team participated in three tasks: concept detection, automatic search, and interac- tive search. Rather than continuing to increase the number of concept detectors available for retrieval, our TRECVID 2008 experiments focus on increasing the robustness of a small set of detectors using a bag-of-words approach. To that end, our concept detection experiments emphasize in particular the role of visual sampling, the value of color in- variant features, the influence of codebook construction, and the effectiveness of kernel-based learning parameters. For retrieval, a robust but limited set of concept detectors ne- cessitates the need to rely on as many auxiliary information channels as possible. Therefore, our automatic search ex- periments focus on predicting which information channel to trust given a certain topic, leading to a novel framework for predictive video retrieval. To improve the video retrieval re- sults further, our interactive search experiments investigate the roles of visualizing preview results for a certain browse- dimension and active learning mechanisms that learn to solve complex search topics by analysis from user brows- ing behavior. The 2008 edition of the TRECVID bench- mark has been the most successful MediaMill participation to date, resulting in the top ranking for both concept de- tection and interactive search, and a runner-up ranking for automatic retrieval. Again a lot has been learned during this year’s TRECVID campaign; we highlight the most im- portant lessons at the end of this paper.
We consider the problem of learning a linear combination of pre-specified kernel matrices in the Fisher discriminant analysis setting. Existing methods for such a task impose an ¿1 norm regularisation on the kernel weights, which produces sparse solution but may lead to loss of information. In this paper, we propose to use ¿2 norm regularisation instead. The resulting learning problem is formulated as a semi-infinite program and can be solved efficiently. Through experiments on both synthetic data and a very challenging object recognition benchmark, the relative advantages of the proposed method and its ¿1 counterpart are demonstrated, and insights are gained as to how the choice of regularisation norm should be made.
Over the last few years, several approaches have been proposed for information fusion including different variants of classifier level fusion (ensemble methods), stacking and multiple kernel learning (MKL). MKL has become a preferred choice for information fusion in object recognition. However, in the case of highly discriminative and complementary feature channels, it does not significantly improve upon its trivial baseline which averages the kernels. Alternative ways are stacking and classifier level fusion (CLF) which rely on a two phase approach. There is a significant amount of work on linear programming formulations of ensemble methods particularly in the case of binary classification. In this paper we propose a multiclass extension of binary ν-LPBoost, which learns the contribution of each class in each feature channel. The existing approaches of classifier fusion promote sparse features combinations, due to regularization based on ℓ1-norm, and lead to a selection of a subset of feature channels, which is not good in the case of informative channels. Therefore, we generalize existing classifier fusion formulations to arbitrary ℓ p -norm for binary and multiclass problems which results in more effective use of complementary information. We also extended stacking for both binary and multiclass datasets. We present an extensive evaluation of the fusion methods on four datasets involving kernels that are all informative and achieve state-of-the-art results on all of them.
One of the key requirements for a cognitive vision system to support reasoning is the possession of an effective mechanism to exploit context both for scene interpretation and for action planning. Context can be used effectively provided the system is endowed with a conducive memory architecture that supports contextual reasoning at all levels of processing, as well as a contextual reasoning framework. In this paper we describe a unified apparatus for reasoning using context, cast in a Bayesian reasoning framework. We also describe a modular memory architecture developed as part of the VAMPIRE* vision system which allows the system to store raw video data at the lowest level and its semantic annotation of monotonically increasing abstraction at the higher levels. By way of illustration, we use as an application for the memory system the automatic annotation of a tennis match.
We review a multiple kernel learning (MKL) technique called ℓp regularised multiple kernel Fisher discriminant analysis (MK-FDA), and investigate the effect of feature space denoising on MKL. Experiments show that with both the original kernels or denoised kernels, ℓp MK-FDA outperforms its fixed-norm counterparts. Experiments also show that feature space denoising boosts the performance of both single kernel FDA and ℓp MK-FDA, and that there is a positive correlation between the learnt kernel weights and the amount of variance kept by feature space denoising. Based on these observations, we argue that in the case where the base feature spaces are noisy, linear combination of kernels cannot be optimal. An MKL objective function which can take care of feature space denoising automatically, and which can learn a truly optimal (non-linear) combination of the base kernels, is yet to be found.
We propose four variants of a novel hierarchical hidden Markov models strategy for rule induction in the context of automated sports video annotation including a multilevel Chinese takeaway process (MLCTP) based on the Chinese restaurant process and a novel Cartesian product label-based hierarchical bottom-up clustering (CLHBC) method that employs prior information contained within label structures. Our results show significant improvement by comparison against the flat Markov model: optimal performance is obtained using a hybrid method, which combines the MLCTP generated hierarchical topological structures with CLHBC generated event labels. We also show that the methods proposed are generalizable to other rule-based environments including human driving behavior and human actions.
3D face reconstruction from a single 2D image can be performed using a 3D Morphable Model (3DMM) in an analysis-by-synthesis approach. However, the reconstruction is an ill-posed problem. The recovery of the illumination characteristics of the 2D input image is particularly difficult because the proportion of the albedo and shading contributions in a pixel intensity is ambiguous. In this paper we propose the use of a facial symmetry constraint, which helps to identify the relative contributions of albedo and shading. The facial symmetry constraint is incorporated in a multi-feature optimisation framework, which realises the fitting process. By virtue of this constraint better illumination parameters can be recovered, and as a result the estimated 3D face shape and surface texture are more accurate. The proposed method is validated on the PIE face database. The experimental results show that the introduction of facial symmetry constraint improves the performance of both, face reconstruction and face recognition.
The 3D Morphable Model (3DMM) is currently receiving considerable attention for human face analysis. Most existing work focuses on fitting a 3DMM to high resolution images. However, in many applications, fitting a 3DMM to low-resolution images is also important. In this paper, we propose a Resolution-Aware 3DMM (RA- 3DMM), which consists of 3 different resolution 3DMMs: High-Resolution 3DMM (HR- 3DMM), Medium-Resolution 3DMM (MR-3DMM) and Low-Resolution 3DMM (LR-3DMM). RA-3DMM can automatically select the best model to fit the input images of different resolutions. The multi-resolution model was evaluated in experiments conducted on PIE and XM2VTS databases. The experimental results verified that HR- 3DMM achieves the best performance for input image of high resolution, and MR- 3DMM and LR-3DMM worked best for medium and low resolution input images, respectively. A model selection strategy incorporated in the RA-3DMM is proposed based on these results. The RA-3DMM model has been applied to pose correction of face images ranging from high to low resolution. The face verification results obtained with the pose-corrected images show considerable performance improvement over the result without pose correction in all resolutions
Performing facial recognition between Near Infrared (NIR) and visible-light (VIS) images has been established as a common method of countering illumination variation problems in face recognition. In this paper we present a new database to enable the evaluation of cross-spectral face recognition. A series of preprocessing algorithms, followed by Local Binary Pattern Histogram (LBPH) representation and combinations with Linear Discriminant Analysis (LDA) are used for recognition. These experiments are conducted on both NIR→VIS and the less common VIS→NIR protocols, with permutations of uni-modal training sets. 12 individual baseline algorithms are presented. In addition, the best performing fusion approaches involving a subset of 12 algorithms are also described. © 2011 IEEE.
Large pose and illumination variations are very challenging for face recognition. The 3D Morphable Model (3DMM) approach is one of the effective methods for pose and illumination invariant face recognition. However, it is very difficult for the 3DMM to recover the illumination of the 2D input image because the ratio of the albedo and illumination contributions in a pixel intensity is ambiguous. Unlike the traditional idea of separating the albedo and illumination contributions using a 3DMM, we propose a novel Albedo Based 3D Morphable Model (AB3DMM), which removes the illumination component from the images using illumination normalisation in a preprocessing step. A comparative study of different illumination normalisation methods for this step is conducted on PIE and Multi-PIE databases. The results show that overall performance of our method outperforms state-of-the-art methods.
By performing experiments on publicly available multi-class datasets we examine the effect of bootstrapping on the bias/variance behaviour of error-correcting output code ensembles. We present evidence to show that the general trend is for bootstrapping to reduce variance but to slightly increase bias error. This generally leads to an improvement in the lowest attainable ensemble error, however this is not always the case and bootstrapping appears to be most useful on datasets where the non-bootstrapped ensemble classifier is prone to overfitting.
Existing ensemble pruning algorithms in the literature have mainly been defined for unweighted or weighted voting ensembles, whose extensions to the Error Correcting Output Coding (ECOC) framework is not successful. This paper presents a novel pruning algorithm to be used in the pruning of ECOC, via using a new accuracy measure together with diversity and Hamming distance information. The results show that the novel method outperforms those existing in the state-of-the-art.
A method for applying weighted decoding to error-correcting output code ensembles of binary classifiers is presented. This method is sensitive to the target class in that a separate weight is computed for each base classifier and target class combination. Experiments on 11 UCI datasets show that the method tends to improve classification accuracy when using neural network or support vector machine base classifiers. It is further shown that weighted decoding combines well with the technique of bootstrapping to improve classification accuracy still further.
There are a variety of methods for inducing predictive systems from observed data. Many of these methods fall into the field of study of machine learning. Some of the most effective algorithms in this domain succeed by combining a number of distinct predictive elements to form what can be described as a type of committee. Well known examples of such algorithms are AdaBoost, bagging and random forests. Stochastic discrimination is a committee-forming algorithm that attempts to combine a large number of relatively simple predictive elements in an effort to achieve a high degree of accuracy. A key element of the success of this technique is that its coverage of the observed feature space should be uniform in nature. We introduce a new uniformity enforcement method, which on benchmark datasets, leads to greater predictive efficiency than the currently published method.
This paper proposes a methodology for the automatic detec- tion of anomalous shipping tracks traced by ferries. The ap- proach comprises a set of models as a basis for outlier detec- tion: A Gaussian process (GP) model regresses displacement information collected over time, and a Markov chain based detector makes use of the direction (heading) information. GP regression is performed together with Median Absolute Devi- ation to account for contaminated training data. The method- ology utilizes the coordinates of a given ferry recorded on a second by second basis via Automatic Identification System. Its effectiveness is demonstrated on a dataset collected in the Solent area.
We present a framework for robust face detection and landmark localisation of faces in the wild, which has been evaluated as part of ‘the 2nd Facial Landmark Localisation Competition’. The framework has four stages: face detection, bounding box aggregation, pose estimation and landmark localisation. To achieve a high detection rate, we use two publicly available CNN-based face detectors and two proprietary detectors. We aggregate the detected face bounding boxes of each input image to reduce false positives and improve face detection accuracy. A cascaded shape regressor, trained using faces with a variety of pose variations, is then employed for pose estimation and image pre-processing. Last, we train the final cascaded shape regressor for fine-grained landmark localisation, using a large number of training samples with limited pose variations. The experimental results obtained on the 300W and Menpo benchmarks demonstrate the superiority of our framework over state-of-the-art methods.
One of the most promising ways to improve biometric person recognition is indisputably via information fusion, that is, to combine different sources of information. This paper proposes a novel fusion paradigm that combines heterogeneous sources of information such as user-specific, cohort and quality information. Two formulations of this problem are proposed, differing in the assumption on the independence of the information sources. Unlike the more common multimodal/multi-algorithmic fusion, the novel paradigm has to deal with information that is not necessarily discriminative but still it is relevant. The methodology can be applied to any biometric system. Furthermore, extensive experiments based on 30 face and fingerprint experiments indicate that the performance gain with respect to the baseline system is about 30%. In contrast, solving this problem using conventional fusion paradigm leads to degraded results. © 2011 IEEE.
While using more biometric traits in multimodal biometric fusion can effectively increase the system robustness, often, the cost associated to adding additional systems is not considered. In this paper, we propose an algorithm that can efficiently bound the biometric system error. This helps not only to speed up the search for the optimal system configuration by an order of magnitude but also unexpectedly to enhance the robustness to population mismatch. This suggests that bounding the error of biometric system from above can possibly be better than directly estimating it from the data. The latter strategy can be susceptible to spurious biometric samples and the particular choice of users. The efficiency of the proposal is achieved thanks to the use of Chernoff bound in estimating the authentication error. Unfortunately, such a bound assumes that the match scores are normally distributed, which is not necessarily the correct distribution model. We propose to transform simultaneously the class conditional match scores (genuine user or impostor scores) into ones that are more conforming to normal distributions using a modified criterion of the Box-Cox transform.
Cohort models are non-match models available in a biometric system. They could be other enrolled models in the gallery of the system. Cohort models have been widely used in biometric systems. A well-established scheme such as T-norm exploits cohort models to predict the statistical parameters of non-match scores for biometric authentication. They have also been used to predict failure or recognition performance of biometric system. In this paper we show that cohort models that are sorted by their similarity to the claimed target model, can produce a discriminative score pattern. We also show that polynomial regression can be used to extract discriminative parameters from these patterns. These parameters can be combined with the raw score to improve the recognition performance of an authentication system. The experimental results obtained for the face and fingerprint modalities of the Biosecure database validate this claim.
Automatically recognizing humans using their biometric traits such as face and fingerprint will have very important implications in our daily lives. This problem is challenging because biometric traits can be affected by the acquisition process which is sensitive to the environmental conditions (e.g., lighting) and the user interaction. It has been shown that post-processing the classifier output, so called score normalization, is an important mechanism to counteract the above problem. In the literature, two dominant research directions have been explored: cohort normalization and quality-based normalization. The first approach relies on a set of competing cohort models, essentially making use of the resultant cohort scores. A well-established example is the T-norm. In the second approach, the normalization is based on deriving the quality information from the raw biometric signal. We propose to combine both the cohort score- and signal-derived information via logistic regression. Based on 12 independent fingerprint experiments, our proposal is found to be significantly better than the T-norm and two recently proposed cohort-based normalization methods. © EURASIP, 2009.
Most existing cognitive architectures integrate computer vision and symbolic reasoning. However, there is still a gap between low-level scene representations (signals) and abstract symbols. Manually attaching, i.e. grounding, the symbols on the physical context makes it impossible to expand system capabilities by learning new concepts. This paper presents a visual bootstrapping approach for the unsupervised symbol grounding. The method is based on a recursive clustering of a perceptual category domain controlled by goal acquisition from the visual environment. The novelty of the method consists in division of goals into the classes of parameter goal, invariant goal and context goal. The proposed system exhibits incremental learning in such a manner as to allow effective transferable representation of high-level concepts.
One-class spoofing detection approaches have been an effective alternative to the two-class learners in the face presentation attack detection particularly in unseen attack scenarios. We propose an ensemble based anomaly detection approach applicable to one-class classifiers. A new score normalisation method is proposed to normalise the output of individual outlier detectors before fusion. To comply with the accuracy and diversity objectives for the component classifiers, three different strategies are utilised to build a pool of anomaly experts. To boost the performance, we also make use of the client-specific information both in the design of individual experts as well as in setting a distinct threshold for each client. We carry out extensive experiments on three face anti-spoofing datasets and show that the proposed ensemble approaches are comparable superior to the techniques based on the two-class formulation or class-independent settings.
Error Correcting Output Coding (ECOC) is a multi- class classification technique in which multiple binary classifiers are trained according to a preset code matrix such that each one learns a separate dichotomy of the classes. While ECOC is one of the best solutions for multi-class problems, one issue which makes it suboptimal is that the training of the base classifiers is done independently of the generation of the code matrix. In this paper, we propose to modify a given ECOC matrix to improve its performance by reducing this decoupling. The proposed algorithm uses beam search to iteratively modify the original matrix, using validation accuracy as a guide. It does not involve further training of the classifiers and can be applied to any ECOC matrix. We evaluate the accuracy of the proposed algorithm (BeamE- COC) using 10-fold cross-validation experiments on 6 UCI datasets, using random code matrices of different sizes, and base classifiers of different strengths. Compared to the random ECOC approach, BeamECOC increases the average cross-validation accuracy in 83 : 3% of the experimental settings involving all datasets, and gives better results than the state-of-the-art in 75% of the scenarios. By employing BeamECOC, it is also possible to reduce the number of columns of a random matrix down to 13% and still obtain comparable or even better results at times.
Deep learning, in particular Convolutional Neural Network (CNN), has achieved promising results in face recognition recently. However, it remains an open question: why CNNs work well and how to design a ‘good’ architecture. The existing works tend to focus on reporting CNN architectures that work well for face recognition rather than investigate the reason. In this work, we conduct an extensive evaluation of CNN-based face recognition systems (CNN-FRS) on a common ground to make our work easily reproducible. Specifically, we use public database LFW (Labeled Faces in the Wild) to train CNNs, unlike most existing CNNs trained on private databases. We propose three CNN architectures which are the first reported architectures trained using LFW data. This paper quantitatively compares the architectures of CNNs and evaluates the effect of different implementation choices. We identify several useful properties of CNN-FRS. For instance, the dimensionality of the learned features can be significantly reduced without adverse effect on face recognition accuracy. In addition, a traditional metric learning method exploiting CNN-learned features is evaluated. Experiments show two crucial factors to good CNN-FRS performance are the fusion of multiple CNNs and metric learning. To make our work reproducible, source code and models will be made publicly available.
This paper proposes a unified framework for quality-based fusion of multimodal biometrics. Quality- dependent fusion algorithms aim to dynamically combine several classifier (biometric expert) outputs as a function of automatically derived (biometric) sample quality. Quality measures used for this purpose quantify the degree of conformance of biometric samples to some predefined criteria known to influence the system performance. Designing a fusion classifier to take quality into consideration is difficult because quality measures cannot be used to distinguish genuine users from impostors, i.e., they are non- discriminative; yet, still useful for classification. We propose a general Bayesian framework that can utilize the quality infor- mation effectively. We show that this framework encompasses several recently proposed quality-based fusion algorithms in the literature -- Nandakumar et al., 2006; Poh et al., 2007; Kryszczuk and Drygajo, 2007; Kittler et al., 2007; Alonso- Fernandez, 2008; Maurer and Baker, 2007; Poh et al., 2010. Furthermore, thanks to the systematic study concluded herein, we also develop two alternative formulations of the problem, leading to more efficient implementation (with fewer parameters) and achieving performance comparable to, or better than the state of the art. Last but not least, the framework also improves the understanding of the role of quality in multiple classifier combination.
© Springer-Verlag Berlin Heidelberg 1996.The paper presents a novel approach to the Robust Analysis of Complex Motion. It employs a low-level robust motion estimator, conceptually based on the Hough Transform, and uses Multiresolution Markov Random Fields for the global interpretation of the local, low-level estimates. Motion segmentation is performed in the front-end estimator, in parallel with the motion parameter estimation process. This significantly improves the accuracy of estimates, particularly in the vicinity of motion boundaries, facilitates the detection of such boundaries, and allows the use of larger regions, thus improving robustness. The measurements extracted from the sequence in the front-end estimator include displacement, the spatial derivatives of the displacement, confidence measures, and the location of motion boundaries. The measurements are then combined within the MRF framework, employing the supercoupling approach for fast convergence. The excellent performance, in terms of estimate accuracy, boundary detection and robustness is demonstrated on synthetic and real-word sequences.
We describe a novel framework to detect ball hits in a tennis game by combining audio and visual information. Ball hit detection is a key step in understanding a game such as tennis, but single-mode approaches are not very successful: audio detection suffers from interfering noise and acoustic mismatch, video detection is made difficult by the small size of the ball and the complex background of the surrounding environment. Our goal in this paper is to improve detection performance by focusing on high-level information (rather than low-level features), including the detected audio events, the ball’s trajectory, and inter-event timing information. Visual information supplies coarse detection of the ball-hits events. This information is used as a constraint for audio detection. In addition, useful gains in detection performance can be obtained by using and inter-ballhit timing information, which aids prediction of the next ball hit. This method seems to be very effective in reducing the interference present in low-level features. After applying this method to a women’s doubles tennis game, we obtained improvements in the F-score of about 30% (absolute) for audio detection and about 10% for video detection.
We present a new loss function, namely Wing loss, for robust facial landmark localisation with Convolutional Neural Networks (CNNs). We first compare and analyse different loss functions including L2, L1 and smooth L1. The analysis of these loss functions suggests that, for the training of a CNN-based localisation model, more attention should be paid to small and medium range errors. To this end, we design a piece-wise loss function. The new loss amplifies the impact of errors from the interval (-w, w) by switching from L1 loss to a modified logarithm function. To address the problem of under-representation of samples with large out-of-plane head rotations in the training set, we propose a simple but effective boosting strategy, referred to as pose-based data balancing. In particular, we deal with the data imbalance problem by duplicating the minority training samples and perturbing them by injecting random image rotation, bounding box translation and other data augmentation approaches. Last, the proposed approach is extended to create a two-stage framework for robust facial landmark localisation. The experimental results obtained on AFLW and 300W demonstrate the merits of the Wing loss function, and prove the superiority of the proposed method over the state-of-the-art approaches.
Several tennis ball tracking algorithms have been reported in the literature. However, most of them use high quality video and multiple cameras, and the emphasis has been on coordinating the cameras, or visualising the tracking results. In this paper, we propose a tennis ball tracking algorithm for low quality off-air video recorded with a single camera. Multiple visual cues are exploited for tennis candidate detection. A particle filter with improved sampling efficiency is used to track the tennis candidates. Experimental results show that our algorithm is robust and has a tracking accuracy that is sufficiently high for automatic annotation of tennis matches.
This paper addresses issues of analysis of DAPI-stained microscopy images of cell samples, particularly classification of objects as single nuclei, nuclei clusters or nonnuclear material. First, segmentation is significantly improved compared to Otsu’s method[5] by choosing a more appropriate threshold, using a cost-function that explicitly relates to the quality of resulting boundary, rather than image histogram. This method applies ideas from active contour models to threshold-based segmentation, combining the local image sensitivity of the former with the simplicity and lower computational complexity of the latter. Secondly, we evaluate some novel measurements that are useful in classification of resulting shapes. Particularly, analysis of central distance profiles provides a method for improved detection of notches in nuclei clusters. Error rates are reduced to less than half compared to those of the base system, which used Fourier shape descriptors alone.
Human gesture recognition plays an important role in automating the analysis of video material at a high level. Especially in sports videos, the determination of the player’s gestures is a key task. In many sports views, the camera covers a large part of the sports arena, resulting in low resolution of the player’s region. Moreover, the camera is not static, but moves dynamically around its optical center, i.e. pan/tilt/zoom camera. These factors make the determination of the player’s gestures a challenging task. To overcome these problems, we propose a posture descriptor that is robust to shape corruption of the player’s silhouette, and a gesture spotting method that is robust to noisy sequences of data and needs only a small amount of training data. The proposed posture descriptor extracts the feature points of a shape, based on the curvature scale space (CSS) method. The use of CSS makes this method robust to local noise, and our method is also robust to significant shape corruption of the player’s silhouette. The proposed spotting method provides probabilistic similarity and is robust to noisy sequences of data. It needs only a small number of training data sets, which is a very useful characteristic when it is difficult to obtain enough data for model training. In this paper, we conducted experiments spotting serve gestures using broadcast tennis play video. From our experiments, for 63 shots of playing tennis, some of which include a serve gesture and while some do not, it achieved 97.5% precision rate and 86.7% recall rate.
Video-based biometric systems are becoming feasible thanks to advancement in both algorithms and computation platforms. Such systems have many advantages: improved robustness to spoof attack, performance gain thanks to variance reduction, and increased data quality/resolution, among others. We investigate a discriminative video-based score-level fusion mechanism, which enables an existing biometric system to further harness the riches of temporarily sampled biometric data using a set of distribution descriptors. Our approach shows that higher order moments of the video scores contain discriminative information. To our best knowledge, this is the first time this higher order moment is reported to be effective in the score-level fusion literature. Experimental results based on face and speech unimodal systems, as well as multimodal fusion, show that our proposal can improve the performance over that of the standard fixed rule fusion strategies by as much as 50%. © 2012 ICPR Org Committee.
In this paper we describe our TRECVID 2009 video re- trieval experiments. The MediaMill team participated in three tasks: concept detection, automatic search, and in- teractive search. The starting point for the MediaMill con- cept detection approach is our top-performing bag-of-words system of last year, which uses multiple color descriptors, codebooks with soft-assignment, and kernel-based supervised learning. We improve upon this baseline system by explor- ing two novel research directions. Firstly, we study a multi- modal extension by including 20 audio concepts and fusion using two novel multi-kernel supervised learning methods. Secondly, with the help of recently proposed algorithmic re- nements of bag-of-word representations, a GPU implemen- tation, and compute clusters, we scale-up the amount of vi- sual information analyzed by an order of magnitude, to a total of 1,000,000 i-frames. Our experiments evaluate the merit of these new components, ultimately leading to 64 ro- bust concept detectors for video retrieval. For retrieval, a robust but limited set of concept detectors justi es the need to rely on as many auxiliary information channels as pos- sible. For automatic search we therefore explore how we can learn to rank various information channels simultane- ously to maximize video search results for a given topic. To further improve the video retrieval results, our interactive search experiments investigate the roles of visualizing pre- view results for a certain browse-dimension and relevance feedback mechanisms that learn to solve complex search top- ics by analysis from user browsing behavior. The 2009 edi- tion of the TRECVID benchmark has again been a fruitful participation for the MediaMill team, resulting in the top ranking for both concept detection and interactive search. Again a lot has been learned during this year's TRECVID campaign; we highlight the most important lessons at the end of this paper.
Automation of HEp-2 cell pattern classification would drastically improve the accuracy and throughput of diagnostic services for many auto-immune diseases, but it has proven difficult to reach a sufficient level of precision. Correct diagnosis relies on a subtle assessment of texture type in microscopic images of indirect immunofluorescence (IIF), which so far has eluded reliable replication through automated measurements. We introduce a combination of spectral analysis and multiscale digital filtering to extract the most discriminative variables from the cell images. We also apply multistage classification techniques to make optimal use of the limited labelled data set. Overall error rate of 1.6% is achieved in recognition of 6 different cell patterns, which drops to 0.5% if only positive samples are considered.
Fitting 3D Morphable Face Models (3DMM) to a 2D face image allows the separation of face shape from skin texture, as well as correction for face expression. However, the recovered 3D face representation is not readily amenable to processing by convolutional neural networks (CNN). We propose a conformal mapping from a 3D mesh to a 2D image, which makes these machine learning tools accessible by 3D face data. Experiments with a CNN based face recognition system designed using the proposed representation have been carried out to validate the advocated approach. The results obtained on standard benchmarking data sets show its promise.
In what way is information processing influenced by the rules underlying a dynamic scene? In two studies we consider this question by examining the relationship between attention allocation in a dynamic visual scene (ie a singles tennis match) and the absence/presence of rule application (ie point allocation task). During training participants observed short clips of a tennis match, and for each they indicated the order of the items (eg players, ball, court lines, umpire, and crowd) from most to least attended. Participants performed a similar task in the test phase, but were also presented with a specific goal which was to indicate which of the two players won the point. In the second experiment, the effects of goal-directed vs non-goal directed observation were compared based on behavioural measures (self-reported ranks and point allocation) and eye-tracking data. Critical differences were revealed between observers regarding their attention allocation for items related to the specific goal (eg court lines). Overall, by varying the levels of goal specificity, observers showed different sensitivity to rule-based items in a dynamic visual scene according to the allocation of attention.
Head pose is an important cue in many applications such as, speech recognition and face recognition. Most approaches to head pose estimation to date have used visual information to model and recognise a subject's head in different configurations. These approaches have a number of limitations such as, inability to cope with occlusions, changes in the appearance of the head, and low resolution images. We present here a novel method for determining coarse head pose orientation purely from audio information, exploiting the direct to reverberant speech energy ratio (DRR) within a highly reverberant meeting room environment. Our hypothesis is that a speaker facing towards a microphone will have a higher DRR and a speaker facing away from the microphone will have a lower DRR. This hypothesis is confirmed by experiments conducted on the publicly available AV16.3 database. © 2013 IEEE.
We consider a multiple classifier system which combines the hard decisions of experts by voting. We argue that the individual experts should not set their own decision thresholds. The respective thresholds should be selected jointly as this will allow compensation of the weaknesses of some experts by the relative strengths of the others. We perform the joint optimization of decision thresholds for a multiple expert system by a systematic sampling of the multidimensional decision threshold space. We show the effectiveness of this approach on the important practical application of video shot cut detection.
We present a method to estimate, based on the horizontal symmetry, an intrinsic coordinate system of faces scanned in 3D. We show that this coordinate system provides an excellent basis for subsequent landmark positioning and model-based refinement such as Active Shape Models, outperforming other -explicit- landmark localisation methods including the commonly-used ICP+ASM approach. © 2012 ICPR Org Committee.
The frequency response of the filter consists of two independent parts. The first is a prolate spheroidal sequence that is dependent on the polar radius. The second is a cosine function of the polar angle. The product of these two parts constitutes a 2-D filtering function. The frequency characteristics of the new filter are similar to that of the 2-D Cartesian separable filter which is defined in terms of two prolate spheroidal sequences. However, in contrast to the 2-D Cartesian separable filter, the position and direction of the new filter in the frequency domain is easy to control. Some applications of the new filter in texture processing, such as generation of synthetic texture, estimation of texture orientation, feature extraction, and texture segmentation, are discussed.
3D Morphable Face Models are a powerful tool in computer vision. They consist of a PCA model of face shape and colour information and allow to reconstruct a 3D face from a single 2D image. 3D Morphable Face Models are used for 3D head pose estimation, face analysis, face recognition, and, more recently, facial landmark detection and tracking. However, they are not as widely used as 2D methods - the process of building and using a 3D model is much more involved. In this paper, we present the Surrey Face Model, a multi-resolution 3D Morphable Model that we make available to the public for non-commercial purposes. The model contains different mesh resolution levels and landmark point annotations as well as metadata for texture remapping. Accompanying the model is a lightweight open-source C++ library designed with simplicity and ease of integration as its foremost goals. In addition to basic functionality, it contains pose estimation and face frontalisation algorithms. With the tools presented in this paper, we aim to close two gaps. First, by offering different model resolution levels and fast fitting functionality, we enable the use of a 3D Morphable Model in time-critical applications like tracking. Second, the software library makes it easy for the community to adopt the 3D Morphable Face Model in their research, and it offers a public place for collaboration.
© 2000 EUSIPCO. We propose a novel motion compensation technique for the precise reconstruction of regions over several frames within a region-based coding scheme. This is achieved by using a more accurate internal representation of arbitrarily shaped regions than the standard grid structure, thus avoiding repeated approximations for a region at each frame.
We apply domain adaptation to the problem of recognizing common actions between differing court-game sport videos (in particular tennis and badminton games). Actions are characterized in terms of HOG3D features extracted at the bounding box of each detected player, and thus have large intrinsic dimensionality. The techniques evaluated here for domain adaptation are based on estimating linear transformations to adapt the source domain features in order to maximize the similarity between posterior PDFs for each class in the source domain and the expected posterior PDF for each class in the target domain. As such, the problem scales linearly with feature dimensionality, making the video-environment domain adaptation problem tractable on reasonable time scales and resilient to over-fitting. We thus demonstrate that significant performance improvement can be achieved by applying domain adaptation in this context.
We present a robust and efficient audio-visual (AV) approach to speaker tracking in a room environment. A challenging problem with visual tracking is to deal with occlusions (caused by the limited field of view of cameras or by other speakers). Another challenge is associated with the particle filtering (PF) algorithm, commonly used for visual tracking, which requires a large number of particles to ensure the distribution is well modelled. In this paper, we propose a new method of fusing audio into the PF based visual tracking. We use the direction of arrival angles (DOAs) of the audio sources to reshape the typical Gaussian noise distribution of particles in the propagation step and to weight the observation model in the measurement step. Experiments on AV16.3 datasets show the advantage of our proposed method over the baseline PF method for tracking occluded speakers with a significantly reduced number of particles. © 2013 IEEE.
Spoofing attacks on biometric systems can seriously compromise their practical utility. In this paper we focus on face spoofing detection. The majority of papers on spoofing attack detection formulate the problem as a two or multiclass learning task, attempting to separate normal accesses from samples of different types of spoofing attacks. In this paper we adopt the anomaly detection approach proposed in [1], where the detector is trained on genuine accesses only using one-class classifiers and investigate the merit of subject specific solutions. We show experimentally that subject specific models are superior to the commonly used client independent method. We also demonstrate that the proposed approach is more robust than multiclass formulations to unseen attacks.
We address the problem of score level fusion of intramodal and multimodal experts in the context of biometric identity verification. We investigate the merits of confidence based weighting of component experts. In contrast to the conventional approach where confidence values are derived from scores, we use instead raw measures of biometric data quality to control the influence of each expert on the final fused score. We show that quality based fusion gives better performance than quality free fusion. The use of quality weighted scores as features in the definition of the fusion functions leads to further improvements. We demonstrate that the achievable performance gain is also affected by the choice of fusion architecture. The evaluation of the proposed methodology involves 6 face and one speech verification experts. It is carried out on the XM2VTS data, base.
This paper proposes a novel method for segmenting lips from face images or video sequences. A non-linear learning method in the form of an SVM classifier is trained to recognise lip colour over a variety of faces. The pixel-level information that the trained classifier outputs is integrated effectively by minimising an energy functional using level set methods, which yields the lip contour(s). The method works over a wide variety of face types, and can elegantly deal with both the case where the subjects' mouths are open and the mouth contour is prominent, and with the closed mouth case where the mouth contour is not visible. © Springer-Verlag Berlin Heidelberg 2007.
In this paper, we propose a multi-layered data association scheme with graph-theoretic formulation for tracking multiple objects that undergo switching dynamics in clutter. The proposed scheme takes as input object candidates detected in each frame. At the object candidate level, "tracklets" are "grown" from sets of candidates that have high probabilities of containing only true positives. At the tracklet level, a directed and weighted graph is constructed, where each node is a tracklet, and the edge weight between two nodes is defined according to the "compatibility” of the two tracklets. The association problem is then formulated as an all-pairs shortest path (APSP) problem in this graph. Finally, at the path level, by analyzing the all-pairs shortest paths, all object trajectories are identified, and track initiation and track termination are automatically dealt with. By exploiting a special topological property of the graph, we have also developed a more efficient APSP algorithm than the general-purpose ones. The proposed data association scheme is applied to tennis sequences to track tennis balls. Experiments show that it works well on sequences where other data association methods perform poorly or fail completely.
Novelty detection is a crucial task in the development of autonomous vision systems. It aims at detecting if samples do not conform with the learnt models. In this paper, we consider the problem of detecting novelty in object recognition problems in which the set of object classes are grouped to form a semantic hierarchy. We follow the idea that, within a semantic hierarchy, novel samples can be defined as samples whose categorization at a specific level contrasts with the categorization at a more general level. This measure indicates if a sample is novel and, in that case, if it is likely to belong to a novel broad category or to a novel sub-category. We present an evaluation of this approach on two hierarchical subsets of the Caltech256 objects dataset and on the SUN scenes dataset, with different classification schemes. We obtain an improvement over Weinshall et al. and show that it is possible to bypass their normalisation heuristic. We demonstrate that this approach achieves good novelty detection rates as far as the conceptual taxonomy is congruent with the visual hierarchy, but tends to fail if this assumption is not satisfied. Copyright 2014 ACM.
The determination of the player's gestures and actions in sports video is a key task in automating the analysis of the video material at a high level. In many sports views, the camera covers a large part of the sports arena, so that the resolution of player's region is low. This makes the determination of the player's gestures and actions a challenging task, especially if there is large camera motion. To overcome these problems, we propose a method based on curvature scale space templates of the player's silhouette. The use of curvature scale space makes the method robust to noise and our method is robust to significant shape corruption of a part of player's silhouette. We also propose a new recognition method which is robust to noisy sequences of data and needs only a small amount of training data. © Springer-Verlag Berlin Heidelberg 2006.
The problem of re-identification of people in a crowd com- monly arises in real application scenarios, yet it has received less atten- tion than it deserves. To facilitate research focusing on this problem, we have embarked on constructing a new person re-identification dataset with many instances of crowded indoor and outdoor scenes. This paper proposes a two-stage robust method for pedestrian detection in a complex crowded background to provide bounding box annotations. The first stage is to generate pedestrian proposals using Faster R-CNN and locate each pedestrian using Non-maximum Suppression (NMS). Candidates in dense proposal regions are merged to identify crowd patches. We then apply a bottom-up human pose estimation method to detect individual pedestrians in the crowd patches. The locations of all subjects are achieved based on the bounding boxes from the two stages. The identity of the detected subjects throughout each video is then automatically annotated using multiple features and spatial-temporal clues. The experimental results on a crowded pedestrians dataset demonstrate the effectiveness and efficiency of the proposed method.
The lip-region can be interpreted as either a genetic or behavioural biometric trait depending on whether static or dynamic information is used. In this paper, we use a texture descriptor called Local Ordinal Contrast Pattern (LOCP) in conjunction with a novel spatiotemporal sampling method called Windowed Three Orthogonal Planes (WTOP) to represent both appearance and dynamics features observed in visual speech. This representation, with standard speaker verification engines, is shown to improve the performance of the lipbiometric trait compared to the state-of-the-art. The improvement obtained suggests that there is enough discriminative information in the mouth-region to enable its use as a primary biometric as opposed to a "soft" biometric trait.
A key question in machine perception is how to adaptively build upon existing capabilities so as to permit novel functionalities. Implicit in this are the notions of anomaly detection and learning transfer. A perceptual system must firstly determine at what point the existing learned model ceases to apply, and secondly, what aspects of the existing model can be brought to bear on the newlydefined learning domain. Anomalies must thus be distinguished from mere outliers, i.e. cases in which the learned model has failed to produce a clear response; it is also necessary to distinguish novel (but meaningful) input from misclassification error within the existing models.We thus apply a methodology of anomaly detection based on comparing the outputs of strong and weak classifiers [8] to the problem of detecting the rule-incongruence involved in the transition from singles to doubles tennis videos. We then demonstrate how the detected anomalies can be used to transfer learning from one (initially known) rule-governed structure to another. Our ultimate aim, building on existing annotation technology, is to construct an adaptive system for court-based sport video annotation.
An important aspect of any scientific discipline is the objective and independent comparison of algorithms which perform common tasks. In image analysis this problem has been neglected. In this paper we present the results and conclusions of a comparison of four Hough Transform, HT, based line finding algorithms on a range of realistic images from the industrial domain. We introduce the line detection problem and show the role of the Hough Transform in it. The basic idea underlying the Hough Transform is presented and is followed by a brief description of each of the four HT based methods considered in our work. The experimental evaluation and comparison of the four methods is given and a section offers our conclusions on the merits and deficiencies of each of the four methods.
A new, combined human activity detection method is proposed. Our method is based on Efros et al.'s motion descriptors[2] and Ke et al.'s event detectors[3]. Since both methods use optical flow, it is easy to combine them. However, the computational cost of the training increases considerably because of the increased number of weak classifiers. We reduce this computational cost by extend Ke et al.'s weak classifiers to incorporate multi-dimensional features. The proposed method is applied to off-air tennis video data, and its performance is evaluated by comparison with the original two methods. Experimental results show that the performance of the proposed method is a good compromise in terms of detection rate and of computation time of testing and training. © 2006 IEEE.
This paper presents a novel fully automatic bi-modal, face and speaker, recognition system which runs in real-time on a mobile phone. The implemented system runs in real-time on a Nokia N900 and demonstrates the feasibility of performing both automatic face and speaker recognition on a mobile phone. We evaluate this recognition system on a novel publicly-available mobile phone database and provide a well defined evaluation protocol. This database was captured almost exclusively using mobile phones and aims to improve research into deploying biometric techniques to mobile devices. We show, on this mobile phone database, that face and speaker recognition can be performed in a mobile environment and using score fusion can improve the performance by more than 25% in terms of error rates. © 2012 IEEE.
In this paper, we propose a novel fitting method that uses local image features to fit a 3D Morphable Face Model to 2D images. To overcome the obstacle of optimising a cost function that contains a non-differentiable feature extraction operator, we use a learning-based cascaded regression method that learns the gradient direction from data. The method allows to simultaneously solve for shape and pose parameters. Our method is thoroughly evaluated on Morphable Model generated data and first results on real data are presented. Compared to traditional fitting methods, which use simple raw features like pixel colour or edge maps, local features have been shown to be much more robust against variations in imaging conditions. Our approach is unique in that we are the first to use local features to fit a 3D Morphable Model. Because of the speed of our method, it is applicable for real-time applications. Our cascaded regression framework is available as an open source library at github.com/patrikhuber/superviseddescent.
Visual concept detection is one of the most important tasks in image and video indexing. This paper describes our system in the ImageCLEF@ICPR Visual Concept Detection Task which ranked first for large-scale visual concept detection tasks in terms of Equal Error Rate (EER) and Area under Curve (AUC) and ranked third in terms of hierarchical measure. The presented approach involves state-of-the-art local descriptor computation, vector quantisation via clustering, structured scene or object representation via localised histograms of vector codes, similarity measure for kernel construction and classifier learning. The main novelty is the classifier-level and kernel-level fusion using Kernel Discriminant Analysis with RBF/Power Chi-Squared kernels obtained from various image descriptors. For 32 out of 53 individual concepts, we obtain the best performance of all 12 submissions to this task.
Region-based coding schemes for video sequences have recently received much attention owing to their potential to enable several interesting multimedia applications. In this paper, we focus on the optimisation of the coding of uncovered regions. We find that the widely-used tools for coding the texture within arbitrarily shaped regions of the intra image are not appropriate for failure regions. We propose a new method for doing so which consists in applying a predictive coding step to the newly visible portions based on already transmitted spatial data.
Player's gesture and action spotting in sports video is a key task in automatic analysis of the video material at a high level. In many sports views, the camera covers a large part of the sports arena, so that the area of player's region is small, and has large motion. These make the determination of the player's gestures and actions a challenging task. To overcome these problems, we propose a method based on curvature scale space templates of the player's silhouette. The use of curvature scale space makes the method robust to noise and our method is robust to significant shape corruption of a part of player's silhouette. We also propose a new recognition method which is robust to noisy sequence of posture and needs only a small amount of training data, which is essential characteristic for many practical applications. © 2006 IEEE.
Object recognition using graph-matching techniques can be viewed as a two-stage process: extracting suitable object primitives from an image and corresponding models, and matching graphs constructed from these two sets of object primitives. In this paper we concentrate mainly on the latter issue of graph matching, for which we derive a technique based on probabilistic relaxation graph labelling. The new method was evaluated on two standard data sets, SOIL47 and COIL100, in both of which objects must be recognised from a variety of different views. The results indicated that our method is comparable with the best of other current object recognition techniques. The potential of the method was also demonstrated on challenging examples of object recognition in cluttered scenes.
The past decade has seen a considerable increase in interest in the field of facial feature extraction. The primary reason for this is the variety of uses, in particular of the mouth region, in communicating important information about an individual which can in turn be used in a wide array of applications. The shape and dynamics of the mouth region convey the content of a communicated message, useful in applications involving speech processing as well as man-machine user interfaces. The mouth region can also be used as a parameter in a biometric verification system. Extraction of the mouth region from a face often uses lip contour processing to achieve these objectives. Thus, solving the problem of reliably segmenting the lip region given a talking face image is critical. This paper compares the use of statistical estimators, both robust and non-robust, when applied to the problem of automatic lip region segmentation. It then compares the results of the two systems with a state-of-the art method for lip segmentation.
A new, combined human activity detection method is proposed. Our method is based on Efros et al.’s motion descriptors and Ke et al.’s event detectors. Since both methods use optical flow, it is easy to combine them. However, the computational cost of the training increases considerably because of the increased number of weak classifiers. We reduce this computational cost by extending Ke et al.’s weak classifiers to incorporate multi-dimensional features. We also introduce a Look Up Table for further high-speed computation. The proposed method is applied to off-air tennis video data, and its performance is evaluated by comparison with the original two methods. Experimental results show that the performance of the proposed method is a good compromise in terms of detection rate and computation time of testing and training.
A novel method of automatic threshold selection based on a simple image statistic is proposed. The method avoids the analysis of complicated image histograms. The properties of the algorithm are presented and experimentally verified on computer generated and real world images.
3D Morphable Face Models (3DMM) have been used in face recognition for some time now. They can be applied in their own right as a basis for 3D face recognition and analysis involving 3D face data. However their prevalent use over the last decade has been as a versatile tool in 2D face recognition to normalise pose, illumination and expression of 2D face images. A 3DMM has the generative capacity to augment the training and test databases for various 2D face processing related tasks. It can be used to expand the gallery set for pose-invariant face matching. For any 2D face image it can furnish complementary information, in terms of its 3D face shape and texture. It can also aid multiple frame fusion by providing the means of registering a set of 2D images. A key enabling technology for this versatility is 3D face model to 2D face image fitting. In this paper recent developments in 3D face modelling and model fitting will be overviewed, and their merits in the context of diverse applications illustrated on several examples, including pose and illumination invariant face recognition, and 3D face reconstruction from video.