MultiSphere: Consistently parallelizing high-dimensional sphere decoders
Start date
01 October 2015End date
15 December 2016Overview
Targeting a theoretical and practical framework for efficiently parallelizing sphere decoders used to optimally reconstruct a large number of mutually interfering information streams.
Sphere decoding is a well-known technique that dramatically reduces the related complexity. However, while sphere decoding is simpler, compared to other solutions that are able to deliver optimal performance, its complexity still increases exponentially with the number of interfering streams, preventing the practical throughput gains from being scaled by increasing the number of mutually interfering streams as predicted in theory.
This research targets practical sphere decoders able to support a large number of interfering streams with processing latency or power consumption requirements which are orders of magnitude smaller than those of single processor systems.
Paradigm shifts
This project is about targeting pragmatic future wireless systems able to deliver the capacity scaling predicted in theory, the proposed research focuses on two paradigm shifts that have a strong potential to transform the way we design wireless communications systems.
First paradigm shift
The one from orthogonal to non-orthogonal signal transmissions according to which, instead of trying to prevent transmitting signals from interfering, we now intentionally allow mutually interfering information streams.
Second paradigm shift
The one from sequential to parallel (receiver) processing according to which instead of using one processing element to perform the calculations of a functionality, we now split the corresponding processing load onto several processing units.
While digital processing systems with tens or even hundreds of processing elements have been predicted, it is still not obvious how we can efficiently exploit this processing power to develop high-throughput and power efficient wireless communication systems, and specifically how we can cope with the exponentially computationally intensive case of optimally recovering a large number of (intentionally) interfering information streams.
Acknowledgements
The research leading to these results has been supported from the UK’s Engineering and Physical Sciences Research Council (EPSRC Grant EP/M029441/1) and the 5G/6G Innovation Centre within the Institute for Communication Systems, University of Surrey.
Funding
The research leading to these results has received funding from the European Research Council under the EU’s Seventh Framework Programme (FP/2007-2013), ERC Grant Agreement number 279976.
Funding amount
£98,465
Team
Principal investigator
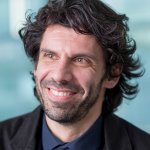
Dr Konstantinos Nikitopoulos
Reader in Signal Processing for Wireless Communications
Biography
Dr Nikitopoulos is currently a reader, within the Institute for Communication Systems, University of Surrey, UK, and the Director of its newly established “Wireless Systems Lab”. He is an active academic member of the 5G Innovation Centre (5GIC) where he leads the “Theory and Practice of Advanced Concepts in Wireless Communications” work area.
Collaborators
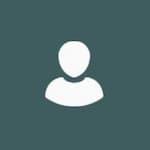
Professor Kyle Jamieson
External collaborator
Biography
Dr Jamieson received BSc and MEng degrees in computer science from the Massachusetts Institute of Technology, USA, and a PhD in computer science, from the same university, in 2008.
He is currently an Associate Professor with the Department of Computer Science, Princeton University, USA, and an Honorary Reader with University College London, UK. His research focuses on building mobile and wireless systems for sensing, localisation, and communication that cut across the boundaries of digital communications and networking.
He received the Starting Investigator Fellowship from the European Research Council in 2011, the Best Paper awards at USENIX 2013 and CoNEXT 2014, and the Google Faculty Research Award in 2015.
Graduate students and engineers
- Georgios Georgis - Senior Researcher
- Christopher Husmann - Researcher
- Chathura Jayawardena - Researcher
- Nauman Iqbal - Researcher
- Daniel Chatzipanagiotis - Alumnus.