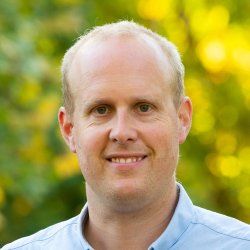
Dr Andrew Gilbert
About
Biography
Dr Andrew Gilbert is an Associate Professor in Machine Learning at the University of Surrey, where he co-leads the interdisciplinary Centre for Creative Arts and Technologies (C-CATS). His research lies at the intersection of computer vision, generative modelling, and multimodal learning, with a particular focus on building interpretable and human-centred AI systems. His work aims to develop machines that not only see and recognise the world, but also understand and creatively respond to it.
Dr Gilbert has made significant contributions to the fields of video understanding, , long-form video captioning, visual style modelling, and AI-driven story understanding. A distinctive feature of his research is its integration into the creative industries, applying technical advances to domains such as media production, performance capture), and digital arts. From training models to classify genre from movie trailers to designing systems that can generate synthetic images and narrative content, his work consistently pushes the boundaries of how AI can support and enhance human creativity.
He leads a vibrant and diverse team of PhD students, collaborating on cutting-edge projects in areas such as self-supervised learning from video, video diffusion models, and multimodal scene understanding. Many of these projects are conducted in close partnership with creative practitioners, industry partners, and other academic disciplines, reflecting Dr Gilbert’s commitment to interdisciplinary and impact-driven research.
In addition to his research leadership, Dr Gilbert is an active contributor to the UK computer vision community. He serves on the British Machine Vision Association (BMVA) Executive Committee, where he organises national technical meetings to foster collaboration between academia and industry. Through this work, he helps shape the research agenda for future AI systems that are explainable, responsible, and aligned with human values.
Research opportunities
There exist many options for securing PhD funding for outstanding candidates that can always be explored. I am always looking for dedicated and ambitious individuals to join my team. Please first send an email to discuss, specifying your interest and availability to join. Include a description of achievements that you are particularly proud of, and write a short paragraph on your ideal research project at Surrey.
Please drop me an email, if interested in any of the areas I am researching.
Current PhD Students
- Oberon Buckingham-West - Adaptive Game Engines for Personalised Learning 2024
- Adrienne Deganutti - Long Term Video Captioning 2023
- Xu Dong - Group Activity Recognition in Video 2023
- Sadegh Rahmani - Human Inspire Video Understanding 2023
- Mona Ahmadian - Self-Supervised Audio Visual Video Understanding 2022
- Soon Yau - Text to Storyboard generation - 2021
- Kar Balan - Decentralized virtual content Understanding for Blockchain- 2021
- Gemma Canet Tarrés - Levering Multimodality for Controllable Image Generation 2021
- Katharina Botel-Azzinnaro - Real stories through faked media: Non-fiction in AI-generated environments 2021
- Tony Orme - Temporal Prediction of IP packets in network switches - 2019
Alumi
- Ed Fish - Film Trailer Genre Understanding and Exploration - 2019
- Dan Ruta - Exploring and understanding fine-grained style - 2019
- Violeta Menendez Gonzalez - Novel viewpoint and stereo inpainting - 2019
- Kary Ho 2019
- Mat Trumble - 2015
- Phil Krejov - 2012
- Segun Oshin - 2008
Areas of specialism
University roles and responsibilities
- Associate Lecturer of Film Production and Broadcast Engineering
- Undergraduate final year project supervision
- Undergraduate placement year supervisor
- Postgraduate (PhD) Supervisor
- Head of Departmental Athena Swan Self Assessment Team
My qualifications
Previous roles
Affiliations and memberships
Academic networks
Recent Publications
2025
Human vs. Machine Minds: Ego-Centric Action Recognition Compared
Sadegh Rahmani, Filip Rybansky, Quoc Vuong, Frank Guerin, Andrew Gilbert, IEEE/CVF Conference on Computer Vision and Pattern Recognition - Workshop on Multimodal Algorithmic Reasoning (MAR’25) , 2025
DANTE-AD: Dual-Vision Attention Network for Long-Term Audio Description
Adrienne Deganutti, Simon Hadfield, Andrew Gilbert, Arxiv Preprint 2503.24096, 2025
Multitwine: Multi-Object Compositing with Text and Layout Control
Gemma C Tarrés, Zhe Lin, Zhifei Zhang, He Zhang, Andrew Gilbert, John Collomosse, Soo Ye Kim, IEEE/CVF Conference on Computer Vision and Pattern Recognition (CVPR’25) 2025
MultiNeRF: Multiple Watermark Embedding for Neural Radiance Fields
Yash Kulthe, Andrew Gilbert, John Collomosse, International Conference on Learning Representations (ICLR’25) - The 1st Workshop on GenAI Watermarking, 2025
2024
Boosting Camera Motion Control for Video Diffusion Transformers
Soon Yau Cheong, Duygu Ceylan, Armin Mustafa, Andrew Gilbert, Chun-Hao Paul Huang, Arxiv Preprint 2410.10802, 2024
FILS: Self-Supervised Video Feature Prediction In Semantic Language Space
Mona Ahmadian, Frank Guerin, Andrew Gilbert, The 35th British Machine Vision Conference (BMVC’24) 2024
Interpretable Long-term Action Quality Assessment
Xu Dong, Xinran Liu, Wanqing Li, Anthony Adeyemi-Ejeye,Andrew Gilbert, The 35th British Machine Vision Conference (BMVC’24) (Oral) 2024
PDFed: Privacy-Preserving and Decentralized Asynchronous Federated Learning for Diffusion Models
Kar Balan, Andrew Gilbert, John Collomosse, Conference on Visual Media Production (CVMP’24) 2024
Detection and Re-Identification in the case of Horse Racing
Will Binning, Sadegh Rahmani, Xu Dong, Andrew Gilbert, Conference on Visual Media Production (CVMP’24) 2024
Thinking Outside the BBox: Unconstrained Generative Object Compositing
Gemma C Tarrés, Zhe Lin, Zhifei Zhang, Jianming Zhang, Yizhi Song, Dan Ruta, Andrew Gilbert, John Collomosse, Soo Ye Kim, European Conference on Computer Vision ECCV’24 2024
ViscoNet: Bridging and Harmonizing Visual and Textual Conditioning for ControlNet
Soon Cheong, Armin Mustafa, Andrew Gilbert, European Conference of Computer Vision 2024, FashionAI: Exploring the intersection of Fashion and Artificial Intelligence for reshaping the Industry, 2024
Diff-nst: Diffusion interleaving for deformable neural style transfer
Dan Ruta, Gemma C Tarrés, Andrew Gilbert, Eli Shechtman, Nick Kolkin, John Collomosse, European Conference of Computer Vision 2024, Vision for Art (VISART VII) Workshop, 2024
Aladin-nst: Self-supervised disentangled representation learning of artistic style through neural style transfer
Dan Ruta, Gemma Canet Tarres, Alexander Black, Andrew Gilbert, John Collomosse, European Conference of Computer Vision 2024, Vision for Art (VISART VII) Workshop, 2024
NeAT: Neural Artistic Tracing for Beautiful Style Transfer
Dan Ruta, Andrew Gilbert, John Collomosse, Eli Shechtman, Nicholas Kolkin, European Conference of Computer Vision 2024, Vision for Art (VISART VII) Workshop, 2024
Interpretable Action Recognition on Hard to Classify Actions
Anastasia Anichenko, Frank Guerin, and Andrew Gilbert, European Conference of Computer Vision 2024, Human-inspired Computer Vision Workshop, 2024
DEAR: Depth-Estimated Action Recognition
Sadegh Rahmani, Filip Rybansky, Quoc Vuong, Frank Guerin, Andrew Gilbert, European Conference of Computer Vision 2024, Human-inspired Computer Vision Workshop, 2024
Towards Rapid Elephant Flow Detection Using Time Series Prediction for OTT Streaming
Anthony Orme, Anthony Adeyemi-Ejeye and Gilbert, Andrew, 19th IEEE International Symposium on Broadband Multimedia Systems and Broadcasting BMSB 2024
PLOT-TAL–Prompt Learning with Optimal Transport for Few-Shot Temporal Action Localization
Ed Fish, Jon Weinbren, Andrew Gilbert, ArXiv abs/2403.18915, 2024
Supervision
Postgraduate research supervision
Research opportunities
There exist many options for securing PhD funding for outstanding candidates that can always be explored. I am always looking for dedicated and ambitious individuals to join my team. Please first send an email to discuss, specifying your interest and availability to join. Include a description of achievements that you are particularly proud of, and write a short paragraph on your ideal research project at Surrey.
Please drop me an email, if interested in any of the areas I am researching.
Current PhD Students
I currently supervise a couple of PhD students
- Mr Ed Fish(2019-) (Exploring Bias in Machine Learning through film)
- Miss Kary Ho(2017-) (Image Inpainting)
Completed postgraduate research projects I have supervised
Alumi
- Ed Fish - Film Trailer Genre Understanding and Exploration - 2019
- Dan Ruta - Exploring and understanding fine-grained style - 2019
- Violeta Menendez Gonzalez - Novel viewpoint and stereo inpainting - 2019
- Kary Ho 2019
- Mat Trumble - 2015
- Phil Krejov - 2012
- Segun Oshin - 2008
Teaching
Ad-hoc tutorials
I am available for tutorials throughout the week, please email me to arrange a convenient time to meet. include in your email an outline of the topics for discussion.
Film and Video Production Technology
I teaching on the Film and Video Production Technology, an undergraduate Broadcast Engineering programme. an exciting course that combines broadcast engineering, artistic storytelling and professional film making. The students study the fundamentals of signals, computing and broadcast technologies, video and audio engineering, wireless links and video over IP. On the practical side, they study camera skills, cinematography for cinema, film sound post-production, TV production, titles and graphics. Examples of the students’ work can be found here.
Teaching modules
Publications
Broadcast television traditionally employs a unidi-rectional transmission path to deliver low latency, high-quality media to viewers. To expand their viewing choices, audiences now demand internet OTT (Over The Top) streamed media with the same quality of experience they have become accustomed to with traditional broadcasting. Media streaming over the internet employs elephant flow characteristics and suffers long delays due to the inherent and variable latency of TCP/IP. This paper proposes to perform rapid elephant flow detection on IP networks within 200ms using a data-driven temporal sequence prediction model, reducing the existing detection time by half. Early detection of media streams (elephant flows) as they enter the network allows the controller in a software-defined network to reroute the elephant flows so that the probability of congestion is reduced and the latency-sensitive mice flows can be given priority. We propose a two-stage machine learning method that encodes the inherent and non-linear temporal data and volume characteristics of the sequential network packets using an ensemble of Long Short-Term Memory (LSTM) layers, followed by a Mixture Density Network (MDN) to model uncertainty, thus determining when an elephant flow (media stream) is being sent within 200ms of the flow starting. We demonstrate that on two standard datasets, we can rapidly identify elephant flows and signal them to the controller within 200ms, improving the current count-min-sketch method that requires more than 450ms of data to achieve comparable results.
We introduce the first generative model capable of simultaneous multi-object compositing, guided by both text and layout. Our model allows for the addition of multiple objects within a scene, capturing a range of interactions from simple positional relations (e.g., next to, in front of) to complex actions requiring reposing (e.g., hugging, playing guitar). When an interaction implies additional props, like `taking a selfie', our model autonomously generates these supporting objects. By jointly training for compositing and subject-driven generation, also known as customization, we achieve a more balanced integration of textual and visual inputs for text-driven object compositing. As a result, we obtain a versatile model with state-of-the-art performance in both tasks. We further present a data generation pipeline leveraging visual and language models to effortlessly synthesize multimodal, aligned training data.
This paper introduces a novel approach to temporal action localization (TAL) in few-shot learning. Our work addresses the inherent limitations of conventional single-prompt learning methods that often lead to overfitting due to the inability to generalize across varying contexts in real-world videos. Recognizing the diversity of camera views, backgrounds, and objects in videos, we propose a multi-prompt learning framework enhanced with optimal transport. This design allows the model to learn a set of diverse prompts for each action, capturing general characteristics more effectively and distributing the representation to mitigate the risk of overfitting. Furthermore, by employing optimal transport theory , we efficiently align these prompts with action features, optimizing for a comprehensive representation that adapts to the multifaceted nature of video data. Our experiments demonstrate significant improvements in action localization accuracy and robustness in few-shot settings on the standard challenging datasets of THUMOS-14 and EpicKitchens100, highlighting the efficacy of our multi-prompt optimal transport approach in overcoming the challenges of conventional few-shot TAL methods.
Detecting actions in videos, particularly within cluttered scenes, poses significant challenges due to the limitations of 2D frame analysis from a camera perspective. Unlike human vision, which benefits from 3D understanding, recognizing actions in such environments can be difficult. This research introduces a novel approach integrating 3D features and depth maps alongside RGB features to enhance action recognition accuracy. Our method involves processing estimated depth maps through a separate branch from the RGB feature encoder and fusing the features to understand the scene and actions comprehensively. Using the Side4Video framework and VideoMamba, which employ CLIP and VisionMamba for spatial feature extraction, our approach outperformed our implementation of the Side4Video network on the Something-Something V2 dataset. Our code is available at: https://github.com/SadeghRahmaniB/DEAR
We present a new method for learning a fine-grained representation of visual style. Representation learning aims to discover individual salient features of a domain in a compact and descriptive form that strongly identifies the unique characteristics of that domain. Prior visual style representation works attempt to disentangle style (i.e. appearance) from content (i.e. semantics) yet a complete separation has yet to be achieved. We present a technique to learn a representation of visual style more strongly disentangled from the semantic content depicted in an image. We use Neural Style Transfer (NST) to measure and drive the learning signal and achieve state-of-the-art representation learning on explicitly disentangled metrics. We show that strongly addressing the disentanglement of style and content leads to large gains in style-specific metrics, encoding far less semantic information and achieving state-of-the-art accuracy in downstream style matching (retrieval) and zero-shot style tagging tasks.
This paper introduces ViscoNet, a novel method that enhances text-to-image human generation models with visual prompting. Unlike existing methods that rely on lengthy text descriptions to control the image structure, ViscoNet allows users to specify the visual appearance of the target object with a reference image. ViscoNet disentangles the object's appearance from the image background and injects it into a pre-trained latent diffusion model (LDM) model via a ControlNet branch. This way, ViscoNet mitigates the style mode collapse problem and enables precise and flexible visual control. We demonstrate the effectiveness of ViscoNet on human image generation, where it can manipulate visual attributes and artistic styles with text and image prompts. We also show that ViscoNet can learn visual conditioning from small and specific object domains while preserving the generative power of the LDM backbone.
We present PDFed, a decentralized, aggregator-free, and asynchronous federated learning protocol for training image diffusion models using a public blockchain. In general, diffusion models are prone to memorization of training data, raising privacy and ethical concerns (e.g., regurgitation of private training data in generated images). Federated learning (FL) offers a partial solution via collaborative model training across distributed nodes that safeguard local data privacy. PDFed proposes a novel sample-based score that measures the novelty and quality of generated samples, incorporating these into a blockchain-based federated learning protocol that we show reduces private data memorization in the collaboratively trained model. In addition, PDFed enables asynchronous collaboration among participants with varying hardware capabilities, facilitating broader participation. The protocol records the provenance of AI models, improving transparency and auditability, while also considering automated incentive and reward mechanisms for participants. PDFed aims to empower artists and creators by protecting the privacy of creative works and enabling decentralized, peer-to-peer collaboration. The protocol positively impacts the creative economy by opening up novel revenue streams and fostering innovative ways for artists to benefit from their contributions to the AI space.
Existing person image generative models can do either image generation or pose transfer but not both. We propose a unified diffusion model, UPGPT to provide a universal solution to perform all the person image tasks - generative, pose transfer, and editing. With fine-grained multimodality and disentanglement capabilities, our approach offers fine-grained control over the generation and the editing process of images using a combination of pose, text, and image, all without needing a semantic segmentation mask which can be challenging to obtain or edit. We also pioneer the parameterized body SMPL model in pose-guided person image generation to demonstrate new capability - simultaneous pose and camera view interpolation while maintaining a person's appearance. Results on the benchmark DeepFashion dataset show that UPGPT is the new state-of-the-art while simultaneously pioneering new capabilities of edit and pose transfer in human image generation.
We present ALADIN (All Layer AdaIN); a novel architecture for searching images based on the similarity of their artistic style. Representation learning is critical to visual search, where distance in the learned search embedding reflects image similarity. Learning an embedding that discriminates fine-grained variations in style is hard, due to the difficulty of defining and labelling style. ALADIN takes a weakly supervised approach to learning a representation for fine-grained style similarity of digital artworks, leveraging BAM-FG, a novel large-scale dataset of user generated content groupings gathered from the web. ALADIN sets a new state of the art accuracy for style-based visual search over both coarse labelled style data (BAM) and BAM-FG; a new 2.62 million image dataset of 310,000 fine-grained style groupings also contributed by this work.
Text-to-image models (T2I) such as StableDiffusion have been used to generate high quality images of people. However, due to the random nature of the generation process, the person has a different appearance e.g. pose, face, and clothing, despite using the same text prompt. The appearance inconsistency makes T2I unsuitable for pose transfer. We address this by proposing a multimodal diffusion model that accepts text, pose, and visual prompting. Our model is the first unified method to perform all person image tasks-generation, pose transfer, and mask-less edit. We also pioneer using small dimensional 3D body model parameters directly to demonstrate new capability - simultaneous pose and camera view interpolation while maintaining the person's appearance.
Despite its popularity and the substantial betting it attracts, horse racing has seen limited research in machine learning. Some studies have tackled related challenges, such as adapting multi-object tracking to the unique geometry of horse tracks [3] and tracking jockey caps during complex manoeuvres [2]. Our research aims to create a helmet detector framework as a preliminary step for re-identification using a limited dataset. Specifically , we detected jockeys' helmets throughout a 205-second race with six disjointed outdoor cameras, addressing challenges like occlusion and varying illumination. Occlusion is a significant challenge in horse racing , often more pronounced than in other sports. Jockeys race in close groups, causing substantial overlap between jockeys and horses in the camera's view, complicating detection and segmentation. Additionally, motion blur, especially in the race's final stretch, and the multi-camera broadcast capturing various angles—front, back, and sides—further complicate detection and consecutively re-identification (Re-ID). To address these issues, we focus on helmet identification rather than detecting all horses or jockeys. We believe helmets, with their simple shapes and consistent appearance even when rotated, offer a more reliable target for detection to make the Re-ID downstream task more achievable. The Architecture: A summary of the architecture is presented in Figure 1. The system focuses on helmet detection and classification. A multi-class Convolutional Neural Network (ConvNet) based on ResNet-18 is trained on labelled helmet classes using a cross-entropy loss function. For helmet detection and segmentation, we employ Grounded-SAM [4] with the prompt "helmet" to accurately detect and segment jockeys' helmets, even capturing partially visible helmets in cases of mild occlusion. These segmented helmets are then cropped from the images to create our dataset. Based on the ResNet-18 architecture, the classification model processes these cropped helmet images and is trained using cross-entropy loss and the Adam optimizer. After training, we apply a confidence threshold to filter out false positives. Finally, we use the detected helmets to annotate the original images with colour-coded bounding boxes corresponding to each class. Dataset: The racing broadcast company Racetech [1] provided the data and industry context that enabled the research presented in this paper. The dataset comprises a single outdoor competitive horse race, captured by six moving cameras, with a total duration of 205 seconds and 1026 frames sampled at 5 FPS. The race features 12 jockeys, and we developed a proof-of-concept labelled dataset focusing on 5 jockeys (or classes) across the six cameras. For semi-automated ground truth labelling, the cropped helmet images were sorted by primary colour, followed by a manual review to remove misclassifications. The model was trained on 80% of the samples from camera 1 and tested on the remaining samples from camera 1 and unseen data from cameras 2-6. Results: The accuracy of the helmet detector across the 6 cameras is illustrated in Figure 2, highlighting the model's overall performance. Helmets with simple, solid-coloured designs, such as classes 1 and 2, achieved higher accuracy. However, helmets with intricate designs, like those in Figure 1: The model segments helmets from RGB images and utilizes a multi-class classifier to train the helmet detector. The final output is an annotated MP4 video, where helmet bounding boxes are colour-coded to represent the five distinct classes. Figure 2: Confusion matrix for individual classes on each angle. Values are percentages of total frames that the class has been successfully annotated. After running through the model, these values were manually verified to remove false positives but do not account for frames where the class is not present. classes 4 and 5, faced challenges, particularly in wide shots where lower resolution impacted detection. Additionally, cameras positioned around turns (such as cameras 4 and 5) were less successful due to issues with occlusion and blurring, which adversely affected both ResNet feature extraction and the initial helmet detection. However, there is a good performance in the classification of helmets despite only using examples from a single camera view to train the model. The camera view 1 helmet examples used to train this model ranged from 70 to 160 images per class, which, in conjunction with the single-angle training set and relatively simple model, shows that there is a prospect of success in this technique. For instance, the most successful class (class 1) had a mean of 79.1% successful detection and classification during the race. Overall, we see this technology as helpful in augmenting the broadcast footage and possibly retrieving specific jockey footage of past races. The near 80% is an excellent initial figure, but we'd expect this to be needed to have far few false negatives and positives for broadcast. Conclusions: We initially selected five jockeys with distinct helmets to simplify dataset creation. However, expanding the dataset revealed challenges in manually classifying similar helmets. Future research will combine distinct helmet detections with jockey or horse tracking to improve classification, acknowledging that not all helmets in a race will be unique. Further exploration of the data requirements for effective helmet detection is also recommended.
Compositing an object into an image involves multiple non-trivial sub-tasks such as object placement and scaling, color/lighting harmonization, viewpoint/geometry adjustment, and shadow/reflection generation. Recent generative image compositing methods leverage diffusion models to handle multiple sub-tasks at once. However, existing models face limitations due to their reliance on masking the original object \sooye{during} training, which constrains their generation to the input mask. Furthermore, obtaining an accurate input mask specifying the location and scale of the object in a new image can be highly challenging. To overcome such limitations, we define a novel problem of \textit{unconstrained generative object compositing}, i.e., the generation is not bounded by the mask, and train a diffusion-based model on a synthesized paired dataset. Our first-of-its-kind model is able to generate object effects such as shadows and reflections that go beyond the mask, enhancing image realism. Additionally, if an empty mask is provided, our model automatically places the object in diverse natural locations and scales, accelerating the compositing workflow. Our model outperforms existing object placement and compositing models in various quality metrics and user studies.
Long-term Action Quality Assessment (AQA) evaluates the execution of activities in videos. However, the length presents challenges in fine-grained interpretability, with current AQA methods typically producing a single score by averaging clip features, lacking detailed semantic meanings of individual clips. Long-term videos pose additional difficulty due to the complexity and diversity of actions, exacerbating interpretability challenges. While query-based transformer networks offer promising long-term modelling capabilities, their interpretability in AQA remains unsatisfactory due to a phenomenon we term Temporal Skipping, where the model skips self-attention layers to prevent output degradation. To address this, we propose an attention loss function and a query initialization method to enhance performance and interpretability. Additionally, we introduce a weight-score regression module designed to approximate the scoring patterns observed in human judgments and replace conventional single-score regression, improving the rationality of interpretability. Our approach achieves state-of-the-art results on three real-world, long-term AQA benchmarks.
This paper demonstrates a self-supervised approach for learning semantic video representations. Recent vision studies show that a masking strategy for vision and natural language supervision has contributed to developing transferable visual pretraining. Our goal is to achieve a more semantic video representation by leveraging the text related to the video content during the pretraining in a fully self-supervised manner. To this end, we present FILS, a novel Self-Supervised Video Feature Prediction In Semantic Language Space (FILS). The vision model can capture valuable structured information by correctly predicting masked feature semantics in language space. It is learned using a patch-wise video-text contrastive strategy, in which the text representations act as prototypes for transforming vision features into a language space, which are then used as targets for semantically meaningful feature prediction using our masked encoder-decoder structure. FILS demonstrates remarkable transferability on downstream action recognition tasks, achieving state-of-the-art on challenging egocentric datasets, like Epic-Kitchens, Something-SomethingV2, Charades-Ego, and EGTEA, using ViT-Base. Our efficient method requires less computation and smaller batches compared to previous works.
In this work, we present an end-to-end network for stereo-consistent image inpainting with the objective of inpainting large missing regions behind objects. The proposed model consists of an edge-guided UNet-like network using Partial Convolutions. We enforce multi-view stereo consistency by introducing a disparity loss. More importantly, we develop a training scheme where the model is learned from realistic stereo masks representing object occlusions, instead of the more common random masks. The technique is trained in a supervised way. Our evaluation shows competitive results compared to previous state-of-the-art techniques.
Broadcast television traditionally employs a unidirectional transmission path to deliver low latency, high-quality media to viewers. To expand their viewing choices, audiences now demand internet OTT (Over The Top) streamed media with the same quality of experience they have become accustomed to with traditional broadcasting. Media streaming over the internet employs elephant flow characteristics and suffers long delays due to the inherent and variable latency of TCP/IP. Early detection of media streams (elephant flows) as they enter the network allows the controller in a software-defined network to re-route the elephant flows so that the probability of congestion is reduced and the latency-sensitive mice flows can be given priority. This paper proposes to perform rapid elephant flow detection, and hence media flow detection, on IP networks within 200ms using a data-driven temporal sequence prediction model, reducing the existing detection time by half. We propose a two-stage machine learning method that encodes the inherent and non-linear temporal data and volume characteristics of the sequential network packets using an ensemble of Long Short-Term Memory (LSTM) layers, followed by a Mixture Density Network (MDN) to model uncertainty, thus determining when an elephant flow (media stream) is being sent within 200ms of the flow starting. We demonstrate that on two standard datasets, we can rapidly identify elephant flows and signal them to the controller within 200ms, improving the current count-minsketch method that requires more than 450ms of data to achieve comparable results.
Style transfer is the task of reproducing the semantic contents of a source image in the artistic style of a second target image. In this paper, we present NeAT, a new state-of-the art feed-forward style transfer method. We re-formulate feed-forward style transfer as image editing, rather than image generation, resulting in a model which improves over the state-of-the-art in both preserving the source content and matching the target style. An important component of our model's success is identifying and fixing "style halos", a commonly occurring artefact across many style transfer techniques. In addition to training and testing on standard datasets, we introduce the BBST-4M dataset, a new, large scale, high resolution dataset of 4M images. As a component of curating this data, we present a novel model able to classify if an image is stylistic. We use BBST-4M to improve and measure the generalization of NeAT across a huge variety of styles. Not only does NeAT offer state-of-the-art quality and generalization, it is designed and trained for fast inference at high resolution.
The field of Action Recognition has seen a large increase in activity in recent years. Much of the progress has been through incorporating ideas from single-frame object recognition and adapting them for temporal-based action recognition. Inspired by the success of interest points in the 2D spatial domain, their 3D (space-time) counterparts typically form the basic components used to describe actions, and in action recognition the features used are often engineered to fire sparsely. This is to ensure that the problem is tractable; however, this can sacrifice recognition accuracy as it cannot be assumed that the optimum features in terms of class discrimination are obtained from this approach. In contrast, we propose to initially use an overcomplete set of simple 2D corners in both space and time. These are grouped spatially and temporally using a hierarchical process, with an increasing search area. At each stage of the hierarchy, the most distinctive and descriptive features are learned efficiently through data mining. This allows large amounts of data to be searched for frequently reoccurring patterns of features. At each level of the hierarchy, the mined compound features become more complex, discriminative, and sparse. This results in fast, accurate recognition with real-time performance on high-resolution video. As the compound features are constructed and selected based upon their ability to discriminate, their speed and accuracy increase at each level of the hierarchy. The approach is tested on four state-of-the-art data sets, the popular KTH data set to provide a comparison with other state-of-the-art approaches, the Multi-KTH data set to illustrate performance at simultaneous multiaction classification, despite no explicit localization information provided during training. Finally, the recent Hollywood and Hollywood2 data sets provide challenging complex actions taken from commercial movie sequences. For all four data sets, the proposed hierarchical approa- h outperforms all other methods reported thus far in the literature and can achieve real-time operation.
In this paper, we aim to tackle the problem of recognising temporal sequences in the context of a multi-class problem. In the past, the representation of sequential patterns was used for modelling discriminative temporal patterns for different classes. Here, we have improved on this by using the more general representation of episodes, of which sequential patterns are a special case. We then propose a novel tree structure called a MultI-Class Episode Tree (MICE-Tree) that allows one to simultaneously model a set of different episodes in an efficient manner whilst providing labels for them. A set of MICE-Trees are then combined together into a MICE-Forest that is learnt in a Boosting framework. The result is a strong classifier that utilises episodes for performing classification of temporal sequences. We also provide experimental evidence showing that the MICE-Trees allow for a more compact and efficient model compared to sequential patterns. Additionally, we demonstrate the accuracy and robustness of the proposed method in the presence of different levels of noise and class labels.
This paper introduces ViscoNet, a novel method that enhances text-to-image human generation models with visual prompting. Unlike existing methods that rely on lengthy text descriptions to control the image structure, ViscoNet allows users to specify the visual appearance of the target object with a reference image. ViscoNet disentangles the object's appearance from the image background and injects it into a pre-trained latent diffusion model (LDM) model via a ControlNet branch. This way, ViscoNet mitigates the style mode collapse problem and enables precise and flexible visual control. We demonstrate the effectiveness of ViscoNet on human image generation, where it can manipulate visual attributes and artistic styles with text and image prompts. We also show that ViscoNet can learn visual conditioning from small and specific object domains while preserving the generative power of the LDM backbone.
Figure 1: DECORAIT enables creatives to register consent (or not) for Generative AI training using their content, as well as to receive recognition and reward for that use. Provenance is traced via visual matching, and consent and ownership registered using a distributed ledger (blockchain). Here, a synthetic image is generated via the Dreambooth[32] method using prompt "a photo of [Subject]" and concept images (left). The red cross indicates images whose creatives have opted out of AI training via DECORAIT, which when taken into account leads to a significant visual change (right). DECORAIT also determines credit apportionment across the opted-in images and pays a proportionate reward to creators via crypto-currency micropyament. ABSTRACT We present DECORAIT; a decentralized registry through which content creators may assert their right to opt in or out of AI training as well as receive reward for their contributions. Generative AI (GenAI) enables images to be synthesized using AI models trained on vast amounts of data scraped from public sources. Model and content creators who may wish to share their work openly without sanctioning its use for training are thus presented with a data gov-ernance challenge. Further, establishing the provenance of GenAI training data is important to creatives to ensure fair recognition and reward for their such use. We report a prototype of DECO-RAIT, which explores hierarchical clustering and a combination of on/off-chain storage to create a scalable decentralized registry to trace the provenance of GenAI training data in order to determine training consent and reward creatives who contribute that data. DECORAIT combines distributed ledger technology (DLT) with visual fingerprinting, leveraging the emerging C2PA (Coalition for Content Provenance and Authenticity) standard to create a secure, open registry through which creatives may express consent and data ownership for GenAI.
Text-to-image models (T2I) such as StableDiffusion have been used to generate high quality images of people. However , due to the random nature of the generation process, the person has a different appearance e.g. pose, face, and clothing, despite using the same text prompt. The appearance inconsistency makes T2I unsuitable for pose transfer. We address this by proposing a multimodal diffusion model that accepts text, pose, and visual prompting. Our model is the first unified method to perform all person image tasks-generation, pose transfer, and mask-less edit. We also pioneer using small dimensional 3D body model parameters directly to demonstrate new capability-simultaneous pose and camera view interpolation while maintaining the per-son's appearance.
Neural Style Transfer (NST) is the field of study applying neural techniques to modify the artistic appearance of a content image to match the style of a reference style image. Traditionally, NST methods have focused on texture-based image edits, affecting mostly low level information and keeping most image structures the same. However, style-based deformation of the content is desirable for some styles, especially in cases where the style is abstract or the primary concept of the style is in its deformed rendition of some content. With the recent introduction of diffusion models, such as Stable Diffusion, we can access far more powerful image generation techniques, enabling new possibilities. In our work, we propose using this new class of models to perform style transfer while enabling deformable style transfer, an elusive capability in previous models. We show how leveraging the priors of these models can expose new artistic controls at inference time, and we document our findings in exploring this new direction for the field of style transfer.
We propose an approach to accurately esti- mate 3D human pose by fusing multi-viewpoint video (MVV) with inertial measurement unit (IMU) sensor data, without optical markers, a complex hardware setup or a full body model. Uniquely we use a multi-channel 3D convolutional neural network to learn a pose em- bedding from visual occupancy and semantic 2D pose estimates from the MVV in a discretised volumetric probabilistic visual hull (PVH). The learnt pose stream is concurrently processed with a forward kinematic solve of the IMU data and a temporal model (LSTM) exploits the rich spatial and temporal long range dependencies among the solved joints, the two streams are then fused in a final fully connected layer. The two complemen- tary data sources allow for ambiguities to be resolved within each sensor modality, yielding improved accu- racy over prior methods. Extensive evaluation is per- formed with state of the art performance reported on the popular Human 3.6M dataset [26], the newly re- leased TotalCapture dataset and a challenging set of outdoor videos TotalCaptureOutdoor. We release the new hybrid MVV dataset (TotalCapture) comprising of multi- viewpoint video, IMU and accurate 3D skele- tal joint ground truth derived from a commercial mo- tion capture system. The dataset is available online at http://cvssp.org/data/totalcapture/.
Representation learning aims to discover individual salient features of a domain in a compact and descriptive form that strongly identifies the unique characteristics of a given sample respective to its domain. Existing works in visual style representation literature have tried to disentangle style from content during training explicitly. A complete separation between these has yet to be fully achieved. Our paper aims to learn a representation of visual artistic style more strongly disentangled from the semantic content depicted in an image. We use Neural Style Transfer (NST) to measure and drive the learning signal and achieve state-of-the-art representation learning on explicitly disentangled metrics. We show that strongly addressing the disentanglement of style and content leads to large gains in style-specific metrics, encoding far less semantic information and achieving state-of-the-art accuracy in downstream multimodal applications.
In this chapter, we present a generic classifier for detecting spatio-temporal interest points within video, the premise being that, given an interest point detector, we can learn a classifier that duplicates its functionality and which is both accurate and computationally efficient. This means that interest point detection can be achieved independent of the complexity of the original interest point formulation. We extend the naive Bayesian classifier of Randomised Ferns to the spatio-temporal domain and learn classifiers that duplicate the functionality of common spatio-temporal interest point detectors. Results demonstrate accurate reproduction of results with a classifier that can be applied exhaustively to video at frame-rate, without optimisation, in a scanning window approach. © 2010, IGI Global.
We present a generic, efficient and iterative algorithm for interactively clustering classes of images and videos. The approach moves away from the use of large hand labelled training datasets, instead allowing the user to find natural groups of similar content based upon a handful of “seed” examples. Two efficient data mining tools originally developed for text analysis; min-Hash and APriori are used and extended to achieve both speed and scalability on large image and video datasets. Inspired by the Bag-of-Words (BoW) architecture, the idea of an image signature is introduced as a simple descriptor on which nearest neighbour classification can be performed. The image signature is then dynamically expanded to identify common features amongst samples of the same class. The iterative approach uses APriori to identify common and distinctive elements of a small set of labelled true and false positive signatures. These elements are then accentuated in the signature to increase similarity between examples and “pull” positive classes together. By repeating this process, the accuracy of similarity increases dramatically despite only a few training examples, only 10% of the labelled groundtruth is needed, compared to other approaches. It is tested on two image datasets including the caltech101 [9] dataset and on three state-of-the-art action recognition datasets. On the YouTube [18] video dataset the accuracy increases from 72% to 97% using only 44 labelled examples from a dataset of over 1200 videos. The approach is both scalable and efficient, with an iteration on the full YouTube dataset taking around 1 minute on a standard desktop machine.
A real-time full-body motion capture system is presented which uses input from a sparse set of inertial measurement units (IMUs) along with images from two or more standard video cameras and requires no optical markers or specialized infra-red cameras. A real-time optimization-based framework is proposed which incorporates constraints from the IMUs, cameras and a prior pose model. The combination of video and IMU data allows the full 6-DOF motion to be recovered including axial rotation of limbs and drift-free global position. The approach was tested using both indoor and outdoor captured data. The results demonstrate the effectiveness of the approach for tracking a wide range of human motion in real time in unconstrained indoor/outdoor scenes.
We describe a non-parametric algorithm for multiple-viewpoint video inpainting. Uniquely, our algorithm addresses the domain of wide baseline multiple-viewpoint video (MVV) with no temporal look-ahead in near real time speed. A Dictionary of Patches (DoP) is built using multi-resolution texture patches reprojected from geometric proxies available in the alternate views. We dynamically update the DoP over time, and a Markov Random Field optimisation over depth and appearance is used to resolve and align a selection of multiple candidates for a given patch, this ensures the inpainting of large regions in a plausible manner conserving both spatial and temporal coherence. We demonstrate the removal of large objects (e.g. people) on challenging indoor and outdoor MVV exhibiting cluttered, dynamic backgrounds and moving cameras.
Self-supervised learning (SSL) techniques have recently produced outstanding results in learning visual representations from unlabeled videos. However, despite the importance of motion in supervised learning techniques for action recognition, SSL methods often do not explicitly consider motion information in videos. To address this issue, we propose MOFO (MOtion FOcused), a novel SSL method for focusing representation learning on the motion area of a video for action recognition. MOFO automatically detects motion areas in videos and uses these to guide the self-supervision task. We use a masked autoencoder that randomly masks out a high proportion of the input sequence and forces a specified percentage of the inside of the motion area to be masked and the remainder from outside. We further incorporate motion information into the finetuning step to emphasise motion in the downstream task. We demonstrate that our motion-focused innovations can significantly boost the performance of the currently leading SSL method (VideoMAE) for action recognition. Our proposed approach significantly improves the performance of the current SSL method for action recognition, indicating the importance of explicitly encoding motion in SSL.
Since 2010, ImageCLEF has run a scalable image annotation task, to promote research into the annotation of images using noisy web page data. It aims to develop techniques to allow computers to describe images reliably, localise di erent concepts depicted and generate descriptions of the scenes. The primary goal of the challenge is to encourage creative ideas of using web page data to improve image annotation. Three subtasks and two pilot teaser tasks were available to participants; all tasks use a single mixed modality data source of 510,123 web page items for both training and test. The dataset included raw images, textual features obtained from the web pages on which the images appeared, as well as extracted visual features. Extracted from the Web by querying popular image search engines, the dataset was formed. For the main subtasks, the development and test sets were both taken from the ____training set". For the teaser tasks, 200,000 web page items were reserved for testing, and a separate development set was provided. The 251 concepts were chosen to be visual objects that are localizable and that are useful for generating textual descriptions of the visual content of images and were mined from the texts of our extensive database of image-webpage pairs. This year seven groups participated in the task, submitting over 50 runs across all subtasks, and all participants also provided working notes papers. In general, the groups' performance is impressive across the tasks, and there are interesting insights into these very relevant challenges.
Transformers have recently been shown to generate high quality images from text input. However, the existing method of pose conditioning using skeleton image tokens is computationally inefficient and generate low quality images. Therefore we propose a new method; Keypoint Pose Encoding (KPE); KPE is 10× more memory efficient and over 73% faster at generating high quality images from text input conditioned on the pose. The pose constraint improves the image quality and reduces errors on body extremities such as arms and legs. The additional benefits include invariance to changes in the target image domain and image resolution, making it easily scalable to higher resolution images. We demonstrate the versatility of KPE by generating photorealistic multiperson images derived from the DeepFashion dataset [1].We also introduce a evaluation method People Count Error (PCE) that is effective in detecting error in generated human images. (a) (b) Figure 1: (a) Our pose constrained text-to-image model supports partial and full pose view, multiple people, different genders, at different scales. (b) The Architectural diagram of our pose-guided text-to-image generation model. The text, pose keypoints and image are encoded into tokens and go into an transformer. *The target image encoding section is required only for training and is not needed in inference.
•Representation and method for evolutionary neural architecture search of encoder-decoder architectures for Deep Image prior,•Leveraging a state-of-the-art perceptual metric to guide the optimization.•State of the art DIP results for inpainting, denoising, up-scaling, beating the hand-optimized DIP architectures proposed.•Demonstrated the content- style dependency of DIP architectures. We present a neural architecture search (NAS) technique to enhance image denoising, inpainting, and super-resolution tasks under the recently proposed Deep Image Prior (DIP). We show that evolutionary search can automatically optimize the encoder-decoder (E-D) structure and meta-parameters of the DIP network, which serves as a content-specific prior to regularize these single image restoration tasks. Our binary representation encodes the design space for an asymmetric E-D network that typically converges to yield a content-specific DIP within 10-20 generations using a population size of 500. The optimized architectures consistently improve upon the visual quality of classical DIP for a diverse range of photographic and artistic content.
Pure vision transformer architectures are highly effective for short video classification and action recognition tasks. However, due to the quadratic complexity of self attention and lack of inductive bias, transformers are resource intensive and suffer from data inefficiencies. Long form video understanding tasks amplify data and memory efficiency problems in transformers making current approaches unfeasible to implement on data or memory restricted domains. This paper introduces an efficient Spatio-Temporal Attention Network (STAN) which uses a two-stream transformer architecture to model dependencies between static image features and temporal contextual features. Our proposed approach can classify videos up to two minutes in length on a single GPU, is data efficient, and achieves SOTA performance on several long video understanding tasks.
We present an approach to iteratively cluster images and video in an efficient and intuitive manor. While many techniques use the traditional approach of time consuming groundtruthing large amounts of data [10, 16, 20, 23], this is increasingly infeasible as dataset size and complexity increase. Furthermore it is not applicable to the home user, who wants to intuitively group his/her own media without labelling the content. Instead we propose a solution that allows the user to select media that semantically belongs to the same class and use machine learning to "pull" this and other related content together. We introduce an "image signature" descriptor and use min-Hash and greedy clustering to efficiently present the user with clusters of the dataset using multi-dimensional scaling. The image signatures of the dataset are then adjusted by APriori data mining identifying the common elements between a small subset of image signatures. This is able to both pull together true positive clusters and push apart false positive examples. The approach is tested on real videos harvested from the web using the state of the art YouTube dataset [18]. The accuracy of correct group label increases from 60.4% to 81.7% using 15 iterations of pulling and pushing the media around. While the process takes only 1 minute to compute the pair wise similarities of the image signatures and visualise the youtube whole dataset. © 2011. The copyright of this document resides with its authors.
Content-aware image completion or in-painting is a fundamental tool for the correction of defects or removal of objects in images. We propose a non-parametric in-painting algorithm that enforces both structural and aesthetic (style) consistency within the resulting image. Our contributions are two-fold: 1) we explicitly disentangle image structure and style during patch search and selection to ensure a visually consistent look and feel within the target image. 2) we perform adaptive stylization of patches to conform the aesthetics of selected patches to the target image, so harmonizing the integration of selected patches into the final composition. We show that explicit consideration of visual style during in-painting delivers excellent qualitative and quantitative results across the varied image styles and content, over the Places2 scene photographic dataset and a challenging new in-painting dataset of artwork derived from BAM!
We present an approach to automatically expand the annotation of images using the internet as an additional information source. The novelty of the work is in the expansion of image tags by automatically introducing new unseen complex linguistic labels which are collected unsupervised from associated webpages. Taking a small subset of existing image tags, a web based search retrieves additional textual information. Both a textual bag of words model and a visual bag of words model are combined and symbolised for data mining. Association rule mining is then used to identify rules which relate words to visual contents. Unseen images that fit these rules are re-tagged. This approach allows a large number of additional annotations to be added to unseen images, on average 12.8 new tags per image, with an 87.2% true positive rate. Results are shown on two datasets including a new 2800 image annotation dataset of landmarks, the results include pictures of buildings being tagged with the architect, the year of construction and even events that have taken place there. This widens the tag annotation impact and their use in retrieval. This dataset is made available along with tags and the 1970 webpages and additional images which form the information corpus. In addition, results for a common state-of-the-art dataset MIRFlickr25000 are presented for comparison of the learning framework against previous works. © 2013 Springer-Verlag.
This paper presents a scalable solution to the problem of tracking objects across spatially separated, uncalibrated, non-overlapping cameras. Unlike other approaches this technique uses an incremental learning method to create the spatio-temporal links between cameras, and thus model the posterior probability distribution of these links. This can then be used with an appearance model of the object to track across cameras. It requires no calibration or batch preprocessing and becomes more accurate over time as evidence is accumulated.
A real-time full-body motion capture system is presented which uses input from a sparse set of inertial measurement units (IMUs) along with images from two or more standard video cameras and requires no optical markers or specialized infra-red cameras. A real-time optimization-based framework is proposed which incorporates constraints from the IMUs, cameras and a prior pose model. The combination of video and IMU data allows the full 6-DOF motion to be recovered including axial rotation of limbs and drift-free global position. The approach was tested using both indoor and outdoor captured data. The results demonstrate the effectiveness of the approach for tracking a wide range of human motion in real time in unconstrained indoor/outdoor scenes.
© Springer International Publishing Switzerland 2015. In recent years, dense trajectories have shown to be an efficient representation for action recognition and have achieved state-of-the art results on a variety of increasingly difficult datasets. However, while the features have greatly improved the recognition scores, the training process and machine learning used hasn’t in general deviated from the object recognition based SVM approach. This is despite the increase in quantity and complexity of the features used. This paper improves the performance of action recognition through two data mining techniques, APriori association rule mining and Contrast Set Mining. These techniques are ideally suited to action recognition and in particular, dense trajectory features as they can utilise the large amounts of data, to identify far shorter discriminative subsets of features called rules. Experimental results on one of the most challenging datasets, Hollywood2 outperforms the current state-of-the-art.
This paper presents a generic method for recognising and localising human actions in video based solely on the distribution of interest points. The use of local interest points has shown promising results in both object and action recognition. While previous methods classify actions based on the appearance and/or motion of these points, we hypothesise that the distribution of interest points alone contains the majority of the discriminatory information. Motivated by its recent success in rapidly detecting 2D interest points, the semi-naive Bayesian classification method of Randomised Ferns is employed. Given a set of interest points within the boundaries of an action, the generic classifier learns the spatial and temporal distributions of those interest points. This is done efficiently by comparing sums of responses of interest points detected within randomly positioned spatio-temporal blocks within the action boundaries. We present results on the largest and most popular human action dataset using a number of interest point detectors, and demostrate that the distribution of interest points alone can perform as well as approaches that rely upon the appearance of the interest points.
This paper presents an approach to hand pose estimation that combines discriminative and model-based methods to leverage the advantages of both. Randomised Decision Forests are trained using real data to provide fast coarse segmentation of the hand. The segmentation then forms the basis of constraints applied in model fitting, using an efficient projected Gauss-Seidel solver, which enforces temporal continuity and kinematic limitations. However, when fitting a generic model to multiple users with varying hand shape, there is likely to be residual errors between the model and their hand. Also, local minima can lead to failures in tracking that are difficult to recover from. Therefore, we introduce an error regression stage that learns to correct these instances of optimisation failure. The approach provides improved accuracy over the current state of the art methods, through the inclusion of temporal cohesion and by learning to correct from failure cases. Using discriminative learning, our approach performs guided optimisation, greatly reducing model fitting complexity and radically improves efficiency. This allows tracking to be performed at over 40 frames per second using a single CPU thread.
Movie genre classification is an active research area in machine learning. However, due to the limited labels available, there can be large semantic variations between movies within a single genre definition. We expand these 'coarse' genre labels by identifying 'fine-grained' semantic information within the multi-modal content of movies. By leveraging pre-trained 'expert' networks, we learn the influence of different combinations of modes for multi-label genre classification. Using a contrastive loss, we continue to fine-tune this 'coarse' genre classification network to identify high-level intertextual similarities between the movies across all genre labels. This leads to a more 'fine-grained' and detailed clustering, based on semantic similarities while still retaining some genre information. Our approach is demonstrated on a newly introduced multi-modal 37,866,450 frame, 8,800 movie trailer dataset, MMX-Trailer-20, which includes pre-computed audio, location, motion, and image embeddings.
This paper presents an overview of the ImageCLEF 2016 evaluation campaign, an event that was organized as part of the CLEF (Conference and Labs of the Evaluation Forum) labs 2016. ImageCLEF is an ongoing initiative that promotes the evaluation of technologies for annotation, indexing and retrieval for providing information access to collections of images in various usage scenarios and domains. In 2016, the 14th edition of ImageCLEF, three main tasks were proposed: 1) identi cation, multi-label classi cation and separation of compound gures from biomedical literature; 2) automatic annotation of general web images; and 3) retrieval from collections of scanned handwritten documents. The handwritten retrieval task was the only completely novel task this year, although the other two tasks introduced several modi cations to keep the proposed tasks challenging.
This paper presents an approach to the categorisation of spatio-temporal activity in video, which is based solely on the relative distribution of feature points. Introducing a Relative Motion Descriptor for actions in video, we show that the spatio-temporal distribution of features alone (without explicit appearance information) effectively describes actions, and demonstrate performance consistent with state-of-the-art. Furthermore, we propose that for actions where noisy examples exist, it is not optimal to group all action examples as a single class. Therefore, rather than engineering features that attempt to generalise over noisy examples, our method follows a different approach: We make use of Random Sampling Consensus (RANSAC) to automatically discover and reject outlier examples within classes. We evaluate the Relative Motion Descriptor and outlier rejection approaches on four action datasets, and show that outlier rejection using RANSAC provides a consistent and notable increase in performance, and demonstrate superior performance to more complex multiple-feature based approaches.
"Actions in the wild" is the term given to examples of human motion that are performed in natural settings, such as those harvested from movies [1] or Internet databases [2]. This paper presents an approach to the categorisation of such activity in video, which is based solely on the relative distribution of spatio-temporal interest points. Presenting the Relative Motion Descriptor, we show that the distribution of interest points alone (without explicitly encoding their neighbourhoods) effectively describes actions. Furthermore, given the huge variability of examples within action classes in natural settings, we propose to further improve recognition by automatically detecting outliers, and breaking complex action categories into multiple modes. This is achieved using a variant of Random Sampling Consensus (RANSAC), which identifies and separates the modes. We employ a novel reweighting scheme within the RANSAC procedure to iteratively reweight training examples, ensuring their inclusion in the final classification model. We demonstrate state-of-the-art performance on five human action datasets. © 2014 Elsevier Inc. All rights reserved.
This paper introduces a novel approach to social behaviour recognition governed by the exchange of non-verbal cues between people. We conduct experiments to try and deduce distinct rules that dictate the social dynamics of people in a conversation, and utilise semi-supervised computer vision techniques to extract their social signals such as laughing and nodding. Data mining is used to deduce frequently occurring patterns of social trends between a speaker and listener in both interested and not interested social scenarios. The confidence values from rules are utilised to build a Social Dynamic Model (SDM), that can then be used for classification and visualisation. By visualising the rules generated in the SDM, we can analyse distinct social trends between an interested and not interested listener in a conversation. Results show that these distinctions can be applied generally and used to accurately predict conversational interest.
The use of sparse invariant features to recognise classes of actions or objects has become common in the literature. However, features are often ”engineered” to be both sparse and invariant to transformation and it is assumed that they provide the greatest discriminative information. To tackle activity recognition, we propose learning compound features that are assembled from simple 2D corners in both space and time. Each corner is encoded in relation to its neighbours and from an over complete set (in excess of 1 million possible features), compound features are extracted using data mining. The final classifier, consisting of sets of compound features, can then be applied to recognise and localise an activity in real-time while providing superior performance to other state-of-the-art approaches (including those based upon sparse feature detectors). Furthermore, the approach requires only weak supervision in the form of class labels for each training sequence. No ground truth position or temporal alignment is required during training.
We present StyleBabel, a unique open access dataset of natural language captions and free-form tags describing the artistic style of over 135K digital artworks, collected via a novel participatory method from experts studying at specialist art and design schools. StyleBabel was collected via an iterative method, inspired by `Grounded Theory': a qualitative approach that enables annotation while co-evolving a shared language for fine-grained artistic style attribute description. We demonstrate several downstream tasks for StyleBabel, adapting the recent ALADIN architecture for fine-grained style similarity, to train cross-modal embeddings for: 1) free-form tag generation; 2) natural language description of artistic style; 3) fine-grained text search of style. To do so, we extend ALADIN with recent advances in Visual Transformer (ViT) and cross-modal representation learning, achieving a state of the art accuracy in fine-grained style retrieval.
We present DECORAIT; a decentralized registry through which content creators may assert their right to opt in or out of AI training as well as receive reward for their contributions. Generative AI (GenAI) enables images to be synthesized using AI models trained on vast amounts of data scraped from public sources. Model and content creators who may wish to share their work openly without sanctioning its use for training are thus presented with a data governance challenge. Further, establishing the provenance of GenAI training data is important to creatives to ensure fair recognition and reward for their such use. We report a prototype of DECORAIT, which explores hierarchical clustering and a combination of on/off-chain storage to create a scalable decentralized registry to trace the provenance of GenAI training data in order to determine training consent and reward creatives who contribute that data. DECORAIT combines distributed ledger technology (DLT) with visual fingerprinting, leveraging the emerging C2PA (Coalition for Content Provenance and Authenticity) standard to create a secure, open registry through which creatives may express consent and data ownership for GenAI.
We present HyperNST; a neural style transfer (NST) technique for the artistic stylization of images, based on Hyper-networks and the StyleGAN2 architecture. Our contribution is a novel method for inducing style transfer parameterized by a metric space, pre-trained for style-based visual search (SBVS). We show for the first time that such space may be used to drive NST, enabling the application and interpolation of styles from an SBVS system. The technical contribution is a hyper-network that predicts weight updates to a StyleGAN2 pre-trained over a diverse gamut of artistic content (portraits), tailoring the style parameterization on a per-region basis using a semantic map of the facial regions. We show HyperNST to exceed state of the art in content preservation for our stylized content while retaining good style transfer performance
There is a clear trend in the use of robots to accomplish services that can help humans. In this paper, robots acting in urban environments are considered for the task of person guiding. Nowadays, it is common to have ubiquitous sensors integrated within the buildings, such as camera networks, and wireless communications like 3G or WiFi. Such infrastructure can be directly used by robotic platforms. The paper shows how combining the information from the robots and the sensors allows tracking failures to be overcome, by being more robust under occlusion, clutter, and lighting changes. The paper describes the algorithms for tracking with a set of fixed surveillance cameras and the algorithms for position tracking using the signal strength received by a wireless sensor network (WSN). Moreover, an algorithm to obtain estimations on the positions of people from cameras on board robots is described. The estimate from all these sources are then combined using a decentralized data fusion algorithm to provide an increase in performance. This scheme is scalable and can handle communication latencies and failures. We present results of the system operating in real time on a large outdoor environment, including 22 nonoverlapping cameras,WSN, and several robots. © Institut Mines-Télécom and Springer-Verlag 2012.
This paper presents an overview of the ImageCLEF 2015 evaluation campaign, an event that was organized as part of the CLEF labs 2015. ImageCLEF is an ongoing initiative that promotes the evaluation of technologies for annotation, indexing and retrieval for providing information access to databases of images in various usage scenarios and domains. In 2015, the 13th edition of ImageCLEF, four main tasks were proposed: 1) automatic concept annotation, localization and sentence description generation for general images; 2) identification, multi-label classification and separation of compound figures from biomedical literature; 3) clustering of x-rays from all over the body; and 4) prediction of missing radiological annotations in reports of liver CT images. The x-ray task was the only fully novel task this year, although the other three tasks introduced modifications to keep up relevancy of the proposed challenges. The participation was considerably positive in this edition of the lab, receiving almost twice the number of submitted working notes papers as compared to previous years.
Within the field of action recognition, features and descriptors are often engineered to be sparse and invariant to transformation. While sparsity makes the problem tractable, it is not necessarily optimal in terms of class separability and classification. This paper proposes a novel approach that uses very dense corner features that are spatially and temporally grouped in a hierarchical process to produce an overcomplete compound feature set. Frequently reoccurring patterns of features are then found through data mining, designed for use with large data sets. The novel use of the hierarchical classifier allows real time operation while the approach is demonstrated to handle camera motion, scale, human appearance variations, occlusions and background clutter. The performance of classification, outperforms other state-of-the-art action recognition algorithms on the three datasets; KTH, multi-KTH, and Hollywood. Multiple action localisation is performed, though no groundtruth localisation data is required, using only weak supervision of class labels for each training sequence. The Hollywood dataset contain complex realistic actions from movies, the approach outperforms the published accuracy on this dataset and also achieves real time performance. ©2009 IEEE.
Movie genre classification is an active research area in machine learning; however, the content of movies can vary widely within a single genre label. We expand these 'coarse' genre labels by identifying 'fine-grained' contextual relationships within the multi-modal content of videos. By leveraging pre-trained 'expert' networks, we learn the influence of different combinations of modes for multi-label genre classification. Then, we continue to fine-tune this 'coarse' genre classification network self-supervised to sub-divide the genres based on the multi-modal content of the videos. Our approach is demonstrated on a new multi-moda137,866,450 frame, 8,800 movie trailer dataset, MMX-Trailer-20, which includes pre-computed audio, location, motion, and image embeddings.
In this work, we present an end-to-end network for stereo-consistent image inpainting with the objective of inpainting large missing regions behind objects. The proposed model consists of an edge-guided UNet-like network using Partial Convolutions. We enforce multi-view stereo consistency by introducing a disparity loss. More importantly, we develop a training scheme where the model is learned from realistic stereo masks representing object occlusions, instead of the more common random masks. The technique is trained in a supervised way. Our evaluation shows competitive results compared to previous state-of-the-art techniques.
In this paper, we propose a computational model for social interaction between three people in a conversation, and demonstrate results using human video motion synthesis. We utilised semi-supervised computer vision techniques to label social signals between the people, like laughing, head nod and gaze direction. Data mining is used to deduce frequently occurring patterns of social signals between a speaker and a listener in both interested and not interested social scenarios, and the mined confidence values are used as conditional probabilities to animate social responses. The human video motion synthesis is done using an appearance model to learn a multivariate probability distribution, combined with a transition matrix to derive the likelihood of motion given a pose configuration. Our system uses social labels to more accurately define motion transitions and build a texture motion graph. Traditional motion synthesis algorithms are best suited to large human movements like walking and running, where motion variations are large and prominent. Our method focuses on generating more subtle human movement like head nods. The user can then control who speaks and the interest level of the individual listeners resulting in social interactive conversational agents.
We present a method for simultaneously estimating 3D hu- man pose and body shape from a sparse set of wide-baseline camera views. We train a symmetric convolutional autoencoder with a dual loss that enforces learning of a latent representation that encodes skeletal joint positions, and at the same time learns a deep representation of volumetric body shape. We harness the latter to up-scale input volumetric data by a factor of 4X, whilst recovering a 3D estimate of joint positions with equal or greater accuracy than the state of the art. Inference runs in real-time (25 fps) and has the potential for passive human behaviour monitoring where there is a requirement for high fidelity estimation of human body shape and pose.
We present a convolutional autoencoder that enables high fidelity volumetric reconstructions of human performance to be captured from multi-view video comprising only a small set of camera views. Our method yields similar end-to-end reconstruction error to that of a prob- abilistic visual hull computed using significantly more (double or more) viewpoints. We use a deep prior implicitly learned by the autoencoder trained over a dataset of view-ablated multi-view video footage of a wide range of subjects and actions. This opens up the possibility of high-end volumetric performance capture in on-set and prosumer scenarios where time or cost prohibit a high witness camera count.
Automatic image annotation is the task of automatically assigning some form of semantic label to images, such as words, phrases or sentences describing the objects, attributes, actions, and scenes depicted in the image. In this chapter, we present an overview of the various automatic image annotation tasks that were organized in conjunction with the ImageCLEF track at CLEF between 2009–2016. Throughout the eight years, the image annotation tasks have evolved from annotating Flickr photos by learning from clean data to annotating web images by learning from large-scale noisy web data. The tasks are divided into three distinct phases, and this chapter will provide a discussion for each of these phases.We will also compare and contrast other related benchmarking challenges, and provide some insights into the future of automatic image annotation.
We present EKILA; a decentralized framework that enables creatives to receive recognition and reward for their contributions to generative AI (GenAI). EKILA proposes a robust visual attribution technique and combines this with an emerging content provenance standard (C2PA) to address the problem of synthetic image provenance – determining the generative model and training data responsible for an AI-generated image. Furthermore, EKILA extends the non-fungible token (NFT) ecosystem to introduce a tokenized representation for rights, enabling a triangular relationship between the asset’s Ownership, Rights, and Attribution (ORA). Leveraging the ORA relationship enables creators to express agency over training consent and, through our attribution model, to receive apportioned credit, including royalty payments for the use of their assets in GenAI.
It is known that relative feature location is important in representing objects, but assumptions that make learning tractable often simplify how structure is encoded e.g. spatial pooling or star models. For example, techniques such as spatial pyramid matching (SPM), in-conjunction with machine learning techniques perform well [13]. However, there are limitations to such spatial encoding schemes which discard important information about the layout of features. In contrast, we propose to use the object itself to choose the basis of the features in an object centric approach. In doing so we return to the early work of geometric hashing [18] but demonstrate how such approaches can be scaled-up to modern day object detection challenges in terms of both the number of examples and their variability. We apply a two stage process, initially filtering background features to localise the objects and then hashing the remaining pairwise features in an affine invariant model. During learning, we identify class-wise key feature predictors. We validate our detection and classification of objects on the PASCAL VOC'07 and' 11 [6] and CarDb [21] datasets and compare with state of the art detectors and classifiers. Importantly we demonstrate how structure in features can be efficiently identified and how its inclusion can increase performance. This feature centric learning technique allows us to localise objects even without object annotation during training and the resultant segmentation provides accurate state of the art object localization, without the need for annotations.
Video activity recognition by deep neural networks is impressive for many classes. However, it falls short of human performance, especially for challenging to discriminate activities. Humans differentiate these complex activities by recognising critical spatio-temporal relations among explicitly recognised objects and parts, for example, an object entering the aperture of a container. Deep neural networks can struggle to learn such critical relationships effectively. Therefore we propose a more human-like approach to activity recognition, which interprets a video in sequential temporal phases and extracts specific relationships among objects and hands in those phases. Random forest classifiers are learnt from these extracted relationships. We apply the method to a challenging subset of the something-something dataset and achieve a more robust performance against neural network baselines on challenging activities.
Often within the field of tracking people within only fixed cameras are used. This can mean that when the the illumination of the image changes or object occlusion occurs, the tracking can fail. We propose an approach that uses three simultaneous separate sensors. The fixed surveillance cameras track objects of interest cross camera through incrementally learning relationships between regions on the image. Cameras and laser rangefinder sensors onboard robots also provide an estimate of the person. Moreover, the signal strength of mobile devices carried by the person can be used to estimate his position. The estimate from all these sources are then combined using data fusion to provide an increase in performance. We present results of the fixed camera based tracking operating in real time on a large outdoor environment of over 20 non-overlapping cameras. Moreover, the tracking algorithms for robots and wireless nodes are described. A decentralized data fusion algorithm for combining all these information is presented.
This paper presents a scalable solution to the problem of tracking objects across spatially separated, uncalibrated, non-overlapping cameras. Unlike other approaches this technique uses an incremental learning method to create the spatio-temporal links between cameras, and thus model the posterior probability distribution of these links. This can then be used with an appearance model of the object to track across cameras. It requires no calibration or batch preprocessing and becomes more accurate over time as evidence is accumulated.
This paper presents a solution to the problem of tracking people within crowded scenes. The aim is to maintain individual object identity through a crowded scene which contains complex interactions and heavy occlusions of people. Our approach uses the strengths of two separate methods; a global object detector and a localised frame by frame tracker. A temporal relationship model of torso detections built during low activity period, is used to further disambiguate during periods of high activity. A single camera with no calibration and no environmental information is used. Results are compared to a standard tracking method and groundtruth. Two video sequences containing interactions, overlaps and occlusions between people are used to demonstrate our approach. The results show that our technique performs better that a standard tracking method and can cope with challenging occlusions and crowd interactions.
We present an algorithm for fusing multi-viewpoint video (MVV) with inertial measurement unit (IMU) sensor data to accurately estimate 3D human pose. A 3-D convolutional neural network is used to learn a pose embedding from volumetric probabilistic visual hull data (PVH) derived from the MVV frames. We incorporate this model within a dual stream network integrating pose embeddings derived from MVV and a forward kinematic solve of the IMU data. A temporal model (LSTM) is incorporated within both streams prior to their fusion. Hybrid pose inference using these two complementary data sources is shown to resolve ambiguities within each sensor modality, yielding improved accuracy over prior methods. A further contribution of this work is a new hybrid MVV dataset (TotalCapture) comprising video, IMU and a skeletal joint ground truth derived from a commercial motion capture system. The dataset is available online at http://cvssp.org/data/totalcapture/.
Temporal Action Localization (TAL) aims to identify actions' start, end, and class labels in untrimmed videos. While recent advancements using transformer networks and Feature Pyramid Networks (FPN) have enhanced visual feature recognition in TAL tasks, less progress has been made in the integration of audio features into such frameworks. This paper introduces the Multi-Resolution Audio-Visual Feature Fusion (MRAV-FF), an innovative method to merge audio-visual data across different temporal resolutions. Central to our approach is a hierarchical gated cross-attention mechanism, which discerningly weighs the importance of audio information at diverse temporal scales. Such a technique not only refines the precision of regression boundaries but also bolsters classification confidence. Importantly, MRAV-FF is versatile, making it compatible with existing FPN TAL architectures and offering a significant enhancement in performance when audio data is available.
The ImageCLEF 2015 Scalable Image Annotation, Localization and Sentence Generation task was the fourth edition of a challenge aimed at developing more scalable image annotation systems. In particular this year the focus of the three subtasks available to participants had the goal to develop techniques to allow computers to reliably describe images, localize the different concepts depicted in the images and generate a description of the scene. All three tasks use a single mixed modality data source of 500,000 web page items which included raw images, textual features obtained from the web pages on which the images appeared, as well as various visual features extracted from the images themselves. Unlike previous years the test set was also the training set and in this edition of the task hand-labelled data has been allowed. The images were obtained from the Web by querying popular image search engines. The development and subtasks 1 and 2 test sets were both taken from the “training set” and had 1,979 and 3,070 samples, and the subtask 3 track had 500 and 450 samples. The 251 concepts this year were chosen to be visual objects that are localizable and that are useful for generating textual descriptions of visual content of images and were mined from the texts of our large database of image-webpage pairs. This year 14 groups participated in the task, submitting a total of 122 runs across the 3 subtasks and 11 of the participants also submitted working notes papers. This result is very positive, in fact if compared to the 11 participants and 58 submitted runs of the last year it is possible to see how the interest in this topic is still very high.
This paper proposes Sparse View Synthesis. This is a view synthesis problem where the number of reference views is limited, and the baseline between target and reference view is significant.Under these conditions, current radiance field methods fail catastrophically due to inescapable artifacts such 3d floating blobs, blurring and structural duplication, whenever the number of reference views is limited, or the target view diverges significantly from the reference views. Advances in network architecture and loss regularisation are unable to satisfactorily remove these artifacts. The occlusions within the scene ensure that the true contents of these regions is simply not available to the model.In this work, we instead focus on hallucinating plausible scene contents within such regions. To this end we unify radiance field models with adversarial learning and perceptual losses. The resulting system provides up to 60% improvement in perceptual accuracy compared to current state-of-the-art radiance field models on this problem.
In the field of media production, video editing techniques play a pivotal role. Recent approaches have had great success at performing novel view image synthesis of static scenes. But adding temporal information adds an extra layer of complexity. Previous models have focused on implicitly representing static and dynamic scenes using NeRF. These models achieve impressive results but are costly at training and inference time. They overfit an MLP to describe the scene implicitly as a function of position. This paper proposes ZeST-NeRF, a new approach that can produce temporal NeRFs for new scenes without retraining. We can accurately reconstruct novel views using multi-view synthesis techniques and scene flow-field estimation, trained only with unrelated scenes. We demonstrate how existing state-of-the-art approaches from a range of fields cannot adequately solve this new task and demonstrate the efficacy of our solution. The resulting network improves quantitatively by 15% and produces significantly better visual results.
We propose a human performance capture system employing convolutional neural networks (CNN) to estimate human pose from a volumetric representation of a performer derived from multiple view-point video (MVV).We compare direct CNN pose regression to the performance of an affine invariant pose descriptor learned by a CNN through a classification task. A non-linear manifold embedding is learned between the descriptor and articulated pose spaces, enabling regression of pose from the source MVV. The results are evaluated against ground truth pose data captured using a Vicon marker-based system and demonstrate good generalisation over a range of human poses, providing a system that requires no special suit to be worn by the performer.
It is known that relative feature location is important in representing objects, but assumptions that make learning tractable often simplify how structure is encoded e.g. spatial pooling or star models. For example, techniques such as spatial pyramid matching (SPM), in-conjunction with machine learning techniques perform well [13]. However, there are limitations to such spatial encoding schemes which discard important information about the layout of features. In contrast, we propose to use the object itself to choose the basis of the features in an object centric approach. In doing so we return to the early work of geometric hashing [18] but demonstrate how such approaches can be scaled-up to modern day object detection challenges in terms of both the number of examples and their variability. We apply a two stage process; initially filtering background features to localise the objects and then hashing the remaining pairwise features in an affine invariant model. During learning, we identify class-wise key feature predictors. We validate our detection and classification of objects on the PASCAL VOC’07 and ’11 [6] and CarDb [21] datasets and compare with state of the art detectors and classifiers. Importantly we demonstrate how structure in features can be efficiently identified and how its inclusion can increase performance. This feature centric learning technique allows us to localise objects even without object annotation during training and the resultant segmentation provides accurate state of the art object localization, without the need for annotations.
We present a novel human performance capture technique capable of robustly estimating the pose (articulated joint positions) of a performer observed passively via multiple view-point video (MVV). An affine invariant pose descriptor is learned using a convolutional neural network (CNN) trained over volumetric data extracted from a MVV dataset of diverse human pose and appearance. A manifold embedding is learned via Gaussian Processes for the CNN descriptor and articulated pose spaces enabling regression and so estimation of human pose from MVV input. The learned descriptor and manifold are shown to generalise over a wide range of human poses, providing an efficient performance capture solution that requires no fiducials or other markers to be worn. The system is evaluated against ground truth joint configuration data from a commercial marker-based pose estimation system
Intelligent visual surveillance is an important application area for computer vision. In situations where networks of hundreds of cameras are used to cover a wide area, the obvious limitation becomes the users’ ability to manage such vast amounts of information. For this reason, automated tools that can generalise about activities or track objects are important to the operator. Key to the users’ requirements is the ability to track objects across (spatially separated) camera scenes. However, extensive geometric knowledge about the site and camera position is typically required. Such an explicit mapping from camera to world is infeasible for large installations as it requires that the operator know which camera to switch to when an object disappears. To further compound the problem the installation costs of CCTV systems outweigh those of the hardware. This means that geometric constraints or any form of calibration (such as that which might be used with epipolar constraints) is simply not realistic for a real world installation. The algorithms cannot afford to dictate to the installer. This work attempts to address this problem and outlines a method to allow objects to be related and tracked across cameras without any explicit calibration, be it geometric or colour.
Self-supervised learning (SSL) techniques have recently produced outstanding results in learning visual representations from unlabeled videos. Despite the importance of motion in supervised learning techniques for action recognition, SSL methods often do not explicitly consider motion information in videos. To address this issue, we propose MOFO (MOtion FOcused), a novel SSL method for focusing representation learning on the motion area of a video, for action recognition. MOFO automatically detects motion areas in videos and uses these to guide the self-supervision task. We use a masked autoencoder which randomly masks out a high proportion of the input sequence; we force a specified percentage of the inside of the motion area to be masked and the remainder from outside. We further incorporate motion information into the finetuning step to emphasise motion in the downstream task. We demonstrate that our motion-focused innovations can significantly boost the performance of the currently leading SSL method (VideoMAE) for action recognition. Our method improves the recent self-supervised Vision Transformer (ViT), VideoMAE, by achieving +2.6%, +2.1%, +1.3% accuracy on Epic-Kitchens verb, noun and action classification, respectively, and +4.7% accuracy on Something-Something V2 action classification. Our proposed approach significantly improves the performance of the current SSL method for action recognition, indicating the importance of explicitly encoding motion in SSL.
Within the eld of image and video recognition, the traditional approach is a dataset split into xed training and test partitions. However, the labelling of the training set is time-consuming, especially as datasets grow in size and complexity. Furthermore, this approach is not applicable to the home user, who wants to intuitively group their media without tirelessly labelling the content. Consequently, we propose a solution similar in nature to an active learning paradigm, where a small subset of media is labelled as semantically belonging to the same class, and machine learning is then used to pull this and other related content together in the feature space. Our interactive approach is able to iteratively cluster classes of images and video. We reformulate it in an online learning framework and demonstrate competitive performance to batch learning approaches using only a fraction of the labelled data. Our approach is based around the concept of an image signature which, unlike a standard bag of words model, can express co-occurrence statistics as well as symbol frequency. We e ciently compute metric distances between signatures despite their inherent high dimensionality and provide discriminative feature selection, to allow common and distinctive elements to be identi ed from a small set of user labelled examples. These elements are then accentuated in the image signature to increase similarity between examples and pull correct classes together. By repeating this process in an online learning framework, the accuracy of similarity increases dramatically despite labelling only a few training examples. To demonstrate that the approach is agnostic to media type and features used, we evaluate on three image datasets (15 scene, Caltech101 and FG-NET), a mixed text and image dataset (ImageTag), a dataset used in active learning (Iris) and on three action recognition datasets (UCF11, KTH and Hollywood2). On the UCF11 video dataset, the accuracy is 86.7% despite using only 90 labelled examples from a dataset of over 1200 videos, instead of the standard 1122 training videos. The approach is both scalable and e cient, with a single iteration over the full UCF11 dataset of around 1200 videos taking approximately 1 minute on a standard desktop machine.
We aim to simultaneously estimate the 3D articulated pose and high fidelity volumetric occupancy of human performance, from multiple viewpoint video (MVV) with as few as two views. We use a multi-channel symmetric 3D convolutional encoder-decoder with a dual loss to enforce the learning of a latent embedding that enables inference of skeletal joint positions and a volumetric reconstruction of the performance. The inference is regularised via a prior learned over a dataset of view-ablated multi-view video footage of a wide range of subjects and actions, and show this to generalise well across unseen subjects and actions. We demonstrate improved reconstruction accuracy and lower pose estimation error relative to prior work on two MVV performance capture datasets: Human 3.6M and TotalCapture.
This paper proposes a generic approach combining a bottom-up (low-level) visual detector with a top-down (high-level) fuzzy first-order logic (FOL) reasoning framework in order to detect pedestrians from a moving vehicle. Detections from the low-level visual corner based detector are fed into the logical reasoning framework as logical facts. A set of FOL clauses utilising fuzzy predicates with piecewise linear continuous membership functions associates a fuzzy confidence (a degree-of-truth) to each detector input. Detections associated with lower confidence functions are deemed as false positives and blanked out, thus adding top-down constraints based on global logical consistency of detections. We employ a state of the art visual detector on a challenging pedestrian detection dataset, and demonstrate an increase in detection performance when used in a framework that combines bottom-up detections with (fuzzy FOL-based) top-down constraints. © 2012 ICPR Org Committee.