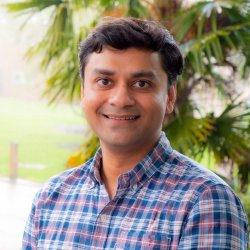
Dr Anjan Dutta
Academic and research departments
Surrey Institute for People-Centred Artificial Intelligence (PAI), Centre for Vision, Speech and Signal Processing (CVSSP), School of Veterinary Medicine.About
Biography
Dr. Anjan Dutta is a Senior Lecturer (Assistant Professor) in Artificial Intelligence at the University of Surrey in United Kingdom. He received a PhD in Computer Science from the Autonomous University of Barcelona (UAB) in 2014, which was awarded with an Excellent Cum Laude (highest grade) qualification with International mention. Moreover, he is a recipient of the Extraordinary PhD Thesis Award for the year 2013-14 by the UAB. Before his PhD, he obtained MSc in Computer Vision and Artificial Intelligence also from the UAB, MCA in Computer Applications from the Maulana Abul Kalam Azad University of Technology and a BSc in Mathematics (Honours) from the University of Calcutta respectively in the year of 2010, 2009 and 2006. His main research interests revolve around computer vision and machine learning. Specifically, he works on deep multi-modal embedding, zero-shot learning, graph neural network for various computer vision tasks.
Areas of specialism
My qualifications
Previous roles
Sustainable development goals
My research interests are related to the following:
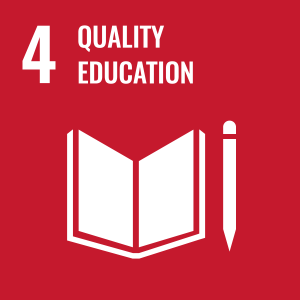
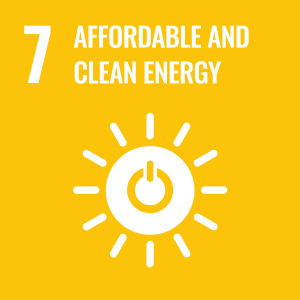
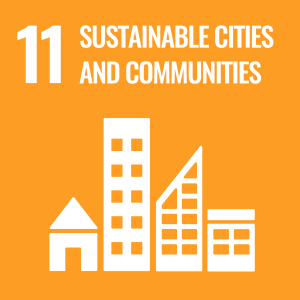
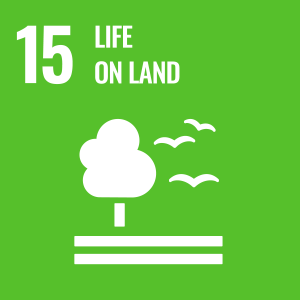
Publications
Invariance learning algorithms that conditionally filter out domain-specific random variables as distractors, do so based only on the data semantics, and not the target domain under evaluation. We show that a provably optimal and sample-efficient way of learning conditional invariances is by relaxing the invariance criterion to be non-commutatively directed towards the target domain. Under domain asymmetry, i.e., when the target domain contains semantically relevant information absent in the source, the risk of the encoder $\varphi^*$ that is optimal on average across domains is strictly lower-bounded by the risk of the target-specific optimal encoder $\Phi^*_\tau$. We prove that non-commutativity steers the optimization towards $\Phi^*_\tau$ instead of $\varphi^*$, bringing the $\mathcal{H}$-divergence between domains down to zero, leading to a stricter bound on the target risk. Both our theory and experiments demonstrate that non-commutative invariance (NCI) can leverage source domain samples to meet the sample complexity needs of learning $\Phi^*_\tau$, surpassing SOTA invariance learning algorithms for domain adaptation, at times by over $2\%$, approaching the performance of an oracle. Implementation is available at https://github.com/abhrac/nci.
Object counting is pivotal for understanding the composition of scenes. Previously, this task was dominated by class-specific methods, which have gradually evolved into more adaptable class-agnostic strategies. However, these strategies come with their own set of limitations, such as the need for manual exemplar input and multiple passes for multiple categories, resulting in significant inefficiencies. This paper introduces a more practical approach enabling simultaneous counting of multiple object categories using an open-vocabulary framework. Our solution, OmniCount, stands out by using semantic and geometric insights (priors) from pre-trained models to count multiple categories of objects as specified by users, all without additional training. OmniCount distinguishes itself by generating precise object masks and leveraging varied interactive prompts via the Segment Anything Model for efficient counting. To evaluate OmniCount, we created the OmniCount-191 benchmark, a first-of-its-kind dataset with multi-label object counts, including points, bounding boxes, and VQA annotations. Our comprehensive evaluation in OmniCount-191, alongside other leading benchmarks, demonstrates OmniCount's exceptional performance, significantly outpacing existing solutions.
In this paper, we investigate the problem of zero-shot sketch-based image retrieval (ZS-SBIR), where human sketches are used as queries to conduct retrieval of photos from unseen categories. We importantly advance prior arts by proposing a novel ZS-SBIR scenario that represents a firm step forward in its practical application. The new setting uniquely recognizes two important yet often neglected challenges of practical ZS-SBIR, (i) the large domain gap between amateur sketch and photo, and (ii) the necessity for moving towards large-scale retrieval. We first contribute to the community a novel ZS-SBIR dataset, QuickDraw-Extended, that consists of 330,000 sketches and 204,000 photos spanning across 110 categories. Highly abstract amateur human sketches are purposefully sourced to maximize the domain gap, instead of ones included in existing datasets that can often be semi-photorealistic. We then formulate a ZS-SBIR framework to jointly model sketches and photos into a common embedding space. A novel strategy to mine the mutual information among domains is specifically engineered to alleviate the domain gap. External semantic knowledge is further embedded to aid semantic transfer. We show that, rather surprisingly, retrieval performance significantly outperforms that of state-of-the-art on existing datasets that can already be achieved using a reduced version of our model. We further demonstrate the superior performance of our full model by comparing with a number of alternatives on the newly proposed dataset. The new dataset, plus all training and testing code of our model, will be publicly released to facilitate future research.
Existing action recognition methods are typically actor-specific due to the intrinsic topological and apparent differences among the actors. This requires actor-specific pose estimation (e.g., humans vs. animals), leading to cumbersome model design complexity and high maintenance costs. Moreover, they often focus on learning the visual modality alone and single-label classification whilst neglecting other available information sources (e.g., class name text) and the concurrent occurrence of multiple actions. To overcome these limitations, we propose a new approach called 'actor-agnostic multi-modal multi-label action recognition,' which offers a unified solution for various types of actors, including humans and animals. We further formulate a novel Multi-modal Semantic Query Network (MSQNet) model in a transformer-based object detection framework (e.g., DETR), characterized by leveraging visual and textual modalities to represent the action classes better. The elimination of actor-specific model designs is a key advantage, as it removes the need for actor pose estimation altogether. Extensive experiments on five publicly available benchmarks show that our MSQNet consistently outperforms the prior arts of actor-specific alternatives on human and animal single- and multi-label action recognition tasks by up to 50%. Code will be released at https://github.com/mondalanindya/MSQNet.
Existing action recognition methods are typically actor-specific due to the intrinsic topological and apparent differences among the actors. This requires actor-specific pose estimation (e.g., humans vs. animals), leading to cumbersome model design complexity and high maintenance costs. Moreover, they often focus on learning the visual modality alone and single-label classification whilst neglecting other available information sources (e.g., class name text) and the concurrent occurrence of multiple actions. To overcome these limitations, we propose a new approach called 'actor-agnostic multi-modal multi-label action recognition,' which offers a unified solution for various types of actors, including humans and animals. We further formulate a novel Multi-modal Semantic Query Network (MSQNet) model in a transformer-based object detection framework (e.g., DETR), characterized by leveraging visual and textual modalities to represent the action classes better. The elimination of actor-specific model designs is a key advantage, as it removes the need for actor pose estimation altogether. Extensive experiments on five publicly available benchmarks show that our MSQNet consistently outperforms the prior arts of actor-specific alternatives on human and animal single- and multi-label action recognition tasks by up to 50%. Code is made available at https://github.com/mondalanindya/MSQNet.
Neural networks trained on biased datasets tend to inadvertently learn spurious correlations, hindering generalization. We formally prove that (1) samples that exhibit spurious correlations lie on a lower rank manifold relative to the ones that do not; and (2) the depth of a network acts as an implicit regularizer on the rank of the attribute subspace that is encoded in its representations. Leveraging these insights, we present DeNetDM, a novel debiasing method that uses network depth modulation as a way of developing robustness to spurious correlations. Using a training paradigm derived from Product of Experts, we create both biased and debiased branches with deep and shallow architectures and then distill knowledge to produce the target debiased model. Our method requires no bias annotations or explicit data augmentation while performing on par with approaches that require either or both. We demonstrate that DeNetDM outperforms existing debiasing techniques on both synthetic and real-world datasets by 5%. Source code will be available upon acceptance.
Rising concerns about privacy and anonymity preservation of deep learning models have facilitated research in data-free learning (DFL). For the first time, we identify that for data-scarce tasks like Sketch-Based Image Retrieval (SBIR), where the difficulty in acquiring paired photos and hand-drawn sketches limits data-dependent cross-modal learning algorithms, DFL can prove to be a much more practical paradigm. We thus propose Data-Free (DF)-SBIR, where, unlike existing DFL problems, pre-trained, single-modality classification models have to be leveraged to learn a cross-modal metric-space for retrieval without access to any training data. The widespread availability of pre-trained classification models, along with the difficulty in acquiring paired photo-sketch datasets for SBIR justify the practicality of this setting. We present a methodology for DF-SBIR, which can leverage knowledge from models independently trained to perform classification on photos and sketches. We evaluate our model on the Sketchy, TU-Berlin, and QuickDraw benchmarks, designing a variety of baselines based on state-of-the-art DFL literature, and observe that our method surpasses all of them by significant margins. Our method also achieves mAPs competitive with data-dependent approaches, all the while requiring no training data. Implementation is available at https://github.com/abhrac/data-free-sbir.
Neural networks trained on biased datasets tend to inadvertently learn spurious correlations, hindering generalization. We formally prove that (1) samples that exhibit spurious correlations lie on a lower rank manifold relative to the ones that do not; and (2) the depth of a network acts as an implicit regularizer on the rank of the attribute subspace that is encoded in its representations. Leveraging these insights, we present DeNetDM, a novel debiasing method that uses network depth modulation as a way of developing robustness to spurious correlations. Using a training paradigm derived from Product of Experts, we create both biased and debiased branches with deep and shallow architectures and then distill knowledge to produce the target debiased model. Our method requires no bias annotations or explicit data augmentation while performing on par with approaches that require either or both. We demonstrate that DeNetDM outperforms existing debiasing techniques on both synthetic and real-world datasets by 5%.
Visual categories that largely share the same set of local parts cannot be discriminated based on part information alone, as they mostly differ in the way the local parts relate to the overall global structure of the object. We propose Relational Proxies , a novel approach that leverages the relational information between the global and local views of an object for encoding its semantic label, even for categories it has not encountered during training. Starting with a rigorous formalization of the notion of distinguishability between categories that share attributes, we prove the necessary and sufficient conditions that a model must satisfy in order to learn the underlying decision boundaries to tell them apart. We design Relational Proxies based on our theoretical findings and evaluate it on seven challenging fine-grained benchmark datasets and achieve state-of-the-art results on all of them, surpassing the performance of all existing works with a margin exceeding 4% in some cases. We additionally show that Relational Proxies also generalizes to the zero-shot setting, where it can efficiently leverage emergent relationships among attributes and image views to generalize to unseen categories, surpassing current state-of-the-art in both the non-generative and generative settings. Implementation will be made public upon acceptance.
Recent advances in fine-grained representation learning leverage local-to-global (emergent) relationships for achieving state-of-the-art results. The relational representations relied upon by such methods, however, are abstract. We aim to deconstruct this abstraction by expressing them as interpretable graphs over image views. We begin by theoretically showing that abstract relational representations are nothing but a way of recovering transitive relationships among local views. Based on this, we design Transitivity Recovering Decompositions (TRD), a graph-space search algorithm that identifies interpretable equivalents of abstract emergent relationships at both instance and class levels, and with no post-hoc computations. We additionally show that TRD is provably robust to noisy views, with empirical evidence also supporting this finding. The latter allows TRD to perform at par or even better than the state-of-the-art, while being fully interpretable. Implementation is available at https://github.com/abhrac/trd.
Low-shot sketch-based image retrieval is an emerging task in computer vision, allowing to retrieve natural images relevant to hand-drawn sketch queries that are rarely seen during the training phase. Related prior works either require aligned sketch-image pairs that are costly to obtain or inefficient memory fusion layer for mapping the visual information to a semantic space. In this paper, we address any-shot,i.e. zero-shot and few-shot, sketch-based image retrieval (SBIR) tasks, where we introduce the few-shot setting for SBIR. For solving these tasks, we propose a semantically aligned paired cycle-consistent generative adversarial network (SEM-PCYC) for any-shot SBIR, where each branch of the generative adversarial network maps the visual information from sketch and image to a common semantic space via adversarial training. Each of these branches maintains cycle consistency that only requires supervision at the category level, and avoids the need of aligned sketch-image pairs. A classification criteria on the generators' outputs ensures the visual to semantic space mapping to be class-specific. Furthermore, we propose to combine textual and hierarchical side information via an auto-encoder that selects discriminating side information within a same end-to-end model. Our results demonstrate a significant boost in any-shot SBIR performance over the state-of-the-art on the extended version of the challenging Sketchy, TU-Berlin and QuickDraw datasets.
Fine-grained categories that largely share the same set of parts cannot be discriminated based on part information alone, as they mostly differ in the way the local parts relate to the overall global structure of the object. We propose Relational Proxies , a novel approach that leverages the relational information between the global and local views of an object for encoding its semantic label. Starting with a rigorous formalization of the notion of distinguishability between fine-grained categories, we prove the necessary and sufficient conditions that a model must satisfy in order to learn the underlying decision boundaries in the fine-grained setting. We design Relational Proxies based on our theoretical findings and evaluate it on seven challenging fine-grained benchmark datasets and achieve state-of-the-art results on all of them, surpassing the performance of all existing works with a margin exceeding 4% in some cases. We also experimentally validate our theory on fine-grained dis-tinguishability and obtain consistent results across multiple benchmarks. Implementation is available at https://github.com/abhrac/relational-proxies.
Representation learning for sketch-based image retrieval has mostly been tackled by learning embeddings that discard modality-specific information. As instances from different modalities can often provide complementary information describing the underlying concept, we propose a cross-attention framework for Vision Transformers (XModalViT) that fuses modality-specific information instead of discarding them. Our framework first maps paired datapoints from the individual photo and sketch modalities to fused representations that unify information from both modalities. We then decouple the input space of the aforementioned modality fusion network into independent encoders of the individual modalities via contrastive and relational cross-modal knowledge distillation. Such encoders can then be applied to downstream tasks like cross-modal retrieval. We demonstrate the expressive capacity of the learned representations by performing a wide range of experiments and achieving state-of-the-art results on three fine-grained sketch-based image retrieval benchmarks: Shoe-V2, Chair-V2 and Sketchy. Implementation is available at https://github.com/abhrac/xmodal-vit.
Zero-Shot Learning (ZSL) aims to recognise unseen object classes which are not observed during the training phase. Most of the existing methods on ZSL focus on learning a compatibility function between the image representation and class semantic information. Few others concentrate on learning image representation by combining local and global features. However, the existing approaches still fail to address the bias issue towards the seen classes. This paper proposes implicit and explicit attention mechanisms to address the existing bias problem in generalised ZSL models. We formulate the implicit attention mechanism with a self-supervised image angle rotation task, which focuses on specific image features aiding in solving the task. On the other hand, the explicit attention mechanism is composed via the consideration of a multi-headed self-attention mechanism in the Vision Transformer model, which learns to attend important image locations and map global image features to semantic space during the training stage. We have conducted comprehensive experiments on three popular benchmarks: AWA2, CUB and SUN, where the effectiveness of our proposed attention mechanisms is shown in both discriminative and generative settings. Our extensive experiments show that our method has achieved state-of-the-art performance obtaining the highest harmonic mean on all three datasets, which is very encouraging to consider the ViT-based attention mechanisms for ZSL tasks in the future.
Existing self-supervised learning methods learn representation by means of pretext tasks which are either (1) discriminating that explicitly specify which features should be separated or (2) aligning that precisely indicate which features should be closed together, but ignore the fact how to jointly and principally define which features to be repelled and which ones to be attracted. In this work, we combine the positive aspects of the discriminating and aligning methods, and design a hybrid method that addresses the above issue. Our method explicitly specifies the repulsion and attraction mechanism respectively by discriminative predictive task and concurrently maximizing mutual information between paired views sharing redundant information. We qualitatively and quantitatively show that our proposed model learns better features that are more effective for the diverse downstream tasks ranging from classification to semantic segmentation. Our experiments on nine established benchmarks show that the proposed model consistently outperforms the existing state-of-the-art results of self-supervised and transfer learning protocol.
The efficacy of zero-shot sketch-based image retrieval (ZS-SBIR) models is governed by two challenges. The immense distributions-gap between the sketches and the images requires a proper domain alignment. Moreover, the fine-grained nature of the task and the high intra-class variance of many categories necessitates a class-wise discriminative mapping among the sketch, image, and the semantic spaces. Under this premise, we propose BDA-SketRet, a novel ZS-SBIR framework performing a bi-level domain adaptation for aligning the spatial and semantic features of the visual data pairs progressively. In order to highlight the shared features and reduce the effects of any sketch or image-specific artifacts, we propose a novel symmetric loss function based on the notion of information bottleneck for aligning the semantic features while a cross-entropy-based adversarial loss is introduced to align the spatial feature maps. Finally, our CNN-based model confirms the discriminativeness of the shared latent space through a novel topology-preserving semantic projection network. Experimental results on the extended Sketchy, TU-Berlin, and QuickDraw datasets exhibit sharp improvements over the literature.
Rising concerns about privacy and anonymity preservation of deep learning models have facilitated research in data-free learning (DFL). For the first time, we identify that for data-scarce tasks like Sketch-Based Image Retrieval (SBIR), where the difficulty in acquiring paired photos and hand-drawn sketches limits data-dependent cross-modal learning algorithms, DFL can prove to be a much more practical paradigm. We thus propose Data-Free (DF)-SBIR, where, unlike existing DFL problems, pre-trained, single-modality classification models have to be leveraged to learn a cross-modal metric-space for retrieval without access to any training data. The widespread availability of pre-trained classification models, along with the difficulty in acquiring paired photo-sketch datasets for SBIR justify the practicality of this setting. We present a methodology for DF-SBIR, which can leverage knowledge from models independently trained to perform classification on photos and sketches. We evaluate our model on the Sketchy, TU-Berlin, and QuickDraw benchmarks, designing a variety of baselines based on state-of-the-art DFL literature, and observe that our method surpasses all of them by significant margins. Our method also achieves mAPs competitive with data-dependent approaches, all the while requiring no training data. Implementation is available at \url{https://github.com/abhrac/data-free-sbir}.
The introduction of the MUStARD dataset, and its emotion recognition extension MUStARD++, have identified sarcasm to be a multi-modal phenomenon -- expressed not only in natural language text, but also through manners of speech (like tonality and intonation) and visual cues (facial expression). With this work, we aim to perform a rigorous benchmarking of the MUStARD++ dataset by considering state-of-the-art language, speech, and visual encoders, for fully utilizing the totality of the multi-modal richness that it has to offer, achieving a 2\% improvement in macro-F1 over the existing benchmark. Additionally, to cure the imbalance in the `sarcasm type' category in MUStARD++, we propose an extension, which we call \emph{MUStARD++ Balanced}, benchmarking the same with instances from the extension split across both train and test sets, achieving a further 2.4\% macro-F1 boost. The new clips were taken from a novel source -- the TV show, House MD, which adds to the diversity of the dataset, and were manually annotated by multiple annotators with substantial inter-annotator agreement in terms of Cohen's kappa and Krippendorf's alpha. Our code, extended data, and SOTA benchmark models are made public.
Most of the existing Zero-Shot Learning (ZSL) methods focus on learning a compatibility function between the image representation and class attributes. Few others concentrate on learning image representation combining local and global features. However, the existing approaches still fail to address the bias issue towards the seen classes. In this paper, we propose implicit and explicit attention mechanisms to address the existing bias problem in ZSL models. We formulate the implicit attention mechanism with a self-supervised image angle rotation task, which focuses on specific image features aiding to solve the task. The explicit attention mechanism is composed with the consideration of a multi-headed self-attention mechanism via Vision Transformer model, which learns to map image features to semantic space during the training stage. We conduct comprehensive experiments on three popular benchmarks: AWA2, CUB and SUN. The performance of our proposed attention mechanisms has proved its effectiveness, and has achieved the state-of-the-art harmonic mean on all the three datasets.
Fine-grained categories that largely share the same set of parts cannot be discriminated based on part information alone, as they mostly differ in the way the local parts relate to the overall global structure of the object. We propose Relational Proxies, a novel approach that leverages the relational information between the global and local views of an object for encoding its semantic label. Starting with a rigorous formalization of the notion of distinguishability between fine-grained categories, we prove the necessary and sufficient conditions that a model must satisfy in order to learn the underlying decision boundaries in the fine-grained setting. We design Relational Proxies based on our theoretical findings and evaluate it on seven challenging fine-grained benchmark datasets and achieve state-of-the-art results on all of them, surpassing the performance of all existing works with a margin exceeding 4% in some cases. We also experimentally validate our theory on fine-grained distinguishability and obtain consistent results across multiple benchmarks. Implementation is available at https://github.com/abhrac/relational-proxies.
Humans show high-level of abstraction capabilities in games that require quickly communicating object information. They decompose the message content into multiple parts and communicate them in an interpretable protocol. Toward equipping machines with such capabilities, we propose the Primitive-based Sketch Abstraction task where the goal is to represent sketches using a fixed set of drawing primitives under the influence of a budget. To solve this task, our Primitive-Matching Network (PMN), learns interpretable abstractions of a sketch in a self supervised manner. Specifically, PMN maps each stroke of a sketch to its most similar primitive in a given set, predicting an affine transformation that aligns the selected primitive to the target stroke. We learn this stroke-to-primitive mapping end-to-end with a distance-transform loss that is minimal when the original sketch is precisely reconstructed with the predicted primitives. Our PMN abstraction empirically achieves the highest performance on sketch recognition and sketch-based image retrieval given a communication budget, while at the same time being highly interpretable. This opens up new possibilities for sketch analysis, such as comparing sketches by extracting the most relevant primitives that define an object category.
The information bottleneck principle provides an information-theoretic method for representation learning, by training an encoder to retain all information which is relevant for predicting the label while minimizing the amount of other, excess information in the representation. The original formulation, however, requires labeled data to identify the superfluous information. In this work, we extend this ability to the multi-view unsupervised setting, where two views of the same underlying entity are provided but the label is unknown. This enables us to identify superfluous information as that not shared by both views. A theoretical analysis leads to the definition of a new multi-view model that produces state-of-the-art results on the Sketchy dataset and label-limited versions of the MIR-Flickr dataset. We also extend our theory to the single-view setting by taking advantage of standard data augmentation techniques, empirically showing better generalization capabilities when compared to common unsupervised approaches for representation learning.
Despite being very successful within the pattern recognition and machine learning community, graph-based methods are often unusable because of the lack of mathematical operations defined in graph domain. Graph embedding, which maps graphs to a vectorial space, has been proposed as a way to tackle these difficulties enabling the use of standard machine learning techniques. However, it is well known that graph embedding functions usually suffer from the loss of structural information. In this paper, we consider the hierarchical structure of a graph as a way to mitigate this loss of information. The hierarchical structure is constructed by topologically clustering the graph nodes and considering each cluster as a node in the upper hierarchical level. Once this hierarchical structure is constructed, we consider several configurations to define the mapping into a vector space given a classical graph embedding, in particular, we propose to make use of the stochastic graphlet embedding (SGE). Broadly speaking, SGE produces a distribution of uniformly sampled low-to-high-order graphlets as a way to embed graphs into the vector space. In what follows, the coarse-to-fine structure of a graph hierarchy and the statistics fetched by the SGE complements each other and includes important structural information with varied contexts. Altogether, these two techniques substantially cope with the usual information loss involved in graph embedding techniques, obtaining a more robust graph representation. This fact has been corroborated through a detailed experimental evaluation on various benchmark graph datasets, where we outperform the state-of-the-art methods.
Humans show high-level of abstraction capabilities in games that require quickly communicating object information. They decompose the message content into multiple parts and communicate them in an interpretable protocol. Toward equipping machines with such capabilities , we propose the Primitive-based Sketch Abstraction task where the goal is to represent sketches using a fixed set of drawing primi-tives under the influence of a budget. To solve this task, our Primitive-Matching Network (PMN), learns interpretable abstractions of a sketch in a self supervised manner. Specifically, PMN maps each stroke of a sketch to its most similar primitive in a given set, predicting an affine transformation that aligns the selected primitive to the target stroke. We learn this stroke-to-primitive mapping end-to-end with a distance-transform loss that is minimal when the original sketch is precisely reconstructed with the predicted primitives. Our PMN abstraction empirically achieves the highest performance on sketch recognition and sketch-based image retrieval given a communication budget, while at the same time being highly interpretable. This opens up new possibilities for sketch analysis, such as comparing sketches by extracting the most relevant primitives that define an object category. Code is available at https://github.com/ExplainableML/sketch-primitives.