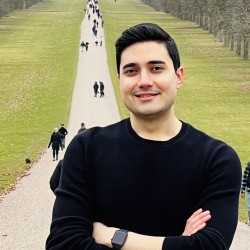
Hesam Shokouh Alaei
Academic and research departments
Centre for Biomedical Engineering, School of Mechanical Engineering Sciences, Faculty of Engineering and Physical Sciences.About
My research project
Diagnosis of Psychogenic non-Epileptic Seizures from Epileptic Seizures Using Electroencephalograms and ElectrocardiogramsPsychogenic non-epileptic seizures (PNES) resembling the symptoms of epileptic seizures are a common diagnostic challenge. An incorrect diagnosis of epilepsy can result in inappropriate treatments such as antiepileptic drugs and unnecessary hospital admissions, which may deteriorate health or lead to a financial burden on health services. In this project, we will use a multi-modal model approach to distinguish the PNES from epileptic seizures based on electroencephalograms and electrocardiograms. This approach includes advanced signal processing and machine learning algorithms based on deep learning. We expect that this research work will address the following question: “How can we design an intelligent system to help neurologists and epileptologists in making the correct diagnosis of the seizures?”
Supervisors
Psychogenic non-epileptic seizures (PNES) resembling the symptoms of epileptic seizures are a common diagnostic challenge. An incorrect diagnosis of epilepsy can result in inappropriate treatments such as antiepileptic drugs and unnecessary hospital admissions, which may deteriorate health or lead to a financial burden on health services. In this project, we will use a multi-modal model approach to distinguish the PNES from epileptic seizures based on electroencephalograms and electrocardiograms. This approach includes advanced signal processing and machine learning algorithms based on deep learning. We expect that this research work will address the following question: “How can we design an intelligent system to help neurologists and epileptologists in making the correct diagnosis of the seizures?”
ResearchResearch interests
- Biomedical Signal Processing
- Machine Learning
- Deep Learning
- Feature Engineering
- Electroencephalogram
- Electrocardiogram
Research interests
- Biomedical Signal Processing
- Machine Learning
- Deep Learning
- Feature Engineering
- Electroencephalogram
- Electrocardiogram
Publications
Living conditions of patients with refractory epilepsy will be significantly improved by a successful prediction of epileptic seizures. A proper warning impending seizure system should be resulted not only in high accuracy and low false positive alarms but also in suitable prediction time. In this study, the mean phase coherence index was used as a reliable indicator for identifying the pre-ictal period of 21-patient Freiburg dataset. In order to predict the seizures on-line, an adaptive Neuro-fuzzy model named ENFM (evolving Neuro-fuzzy model) was used to classify the extracted features. The ENFM was trained by a new class labeling method based on the temporal properties of a prediction characterized by two time intervals, seizure prediction horizon (SPH) and seizure occurrence period (SOP), which are subsequently applied in evaluation method. It is evident that increasing the SPH duration can be more beneficial to patients in preventing irreparable consequences of the seizure, as well as providing adequate time to deal with the seizure. In addition, a reduction in SOP duration can reduce the patient’s stress in SOP interval. These two theories motivated us to design Mamdani fuzzy inference system considering sensitivity and FPR of the prediction result in order to find optimal SOP and SPH for each patient. 10-patient dataset assigned for optimizing the fuzzy system, while the rest of data was used to test the model. The results showed that mean SOP by 6 min and mean SPH by 27 min provided the best outcome, so that last seizure as well as about 15-h inter-ictal period of each patient were predicted on-line without false negative alarms, yielding on average 100% sensitivity, 0.13 per hour FPR, 86.95% precision and 92.5% accuracy.
In this research, the mean phase coherence index used as a reliable indicator for identifying the preictal period of the 14-patient Freiburg EEG dataset. In order to predict the seizures on-line, an adaptive Neuro-fuzzy model named ENFM (evolving neuro-fuzzy model) was used to classify the extracted features. The ENFM trained by a new class labeling method based on the temporal properties of a prediction characterized by two time intervals, seizure prediction horizon (SPH), and seizure occurrence period (SOP), which subsequently applied in the evaluation method. It is evident that an increase in the duration of the SPH can be more useful for the subject in preventing the irreparable consequences of the seizure, and provides adequate time to deal with the seizure. Also, a reduction in duration of the SOP can reduce the patient’s stress in the SOP interval. In this study, the optimal SOP and SPH obtained for each patient using Mamdani fuzzy inference system considering sensitivity, false-positive rate (FPR), and the two mentioned points, which generally ignored in most studies.
Patients with anxious depression have more severe symptoms, more side effects, and higher resistance to treatment than patients with non-anxious depression; therefore, it is crucial to clarify the differences between these two types of patients. In this study, a 5-minute resting EEG was recorded in 15 patients with anxious depression and 9 patients with non-anxious depression under eyes open and closed conditions. Sixty-eight subcortical regions were extracted using exact low resolution brain electromagnetic tomography (eLORETA). The directed transfer function was then used to construct brain networks. Specific features based on graph theory including the strength of connectivity and betweenness centrality (BC) were calculated from the networks. Finally, significant features were selected using the Mann-Whitney U test, and patients were classified into anxious and non-anxious depressive groups using the Support Vector Machine (SVM). Results showed that features of outward connectivity strength led to the highest accuracy, F-score, and specificity with 91.66%, 87. 5%, and 100% in the eyes-closed state, respectively. Moreover, we found that the strength of connectivity in both directions increased for the anxious depressive group during the eyes-open state. In particular, higher outward connectivity was observed in the right hemisphere for the anxious depressive group. Further findings also revealed that features with the most significant difference were mainly associated with the beta band. In addition, significant increased inward and outward connectivity and decreased nodal centrality were observed in the posterior regions of the default mode network. These preliminary findings might provide new insights into the recognition of anxious depressed patients.