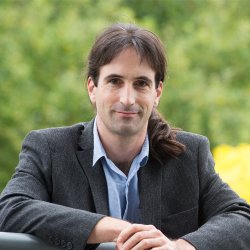
Dr Daniel Abasolo
Academic and research departments
Centre for Biomedical Engineering, School of Mechanical Engineering Sciences, Faculty of Engineering and Physical Sciences.About
Biography
Dr Daniel Abásolo MEng PhD is Senior Lecturer in Biomedical Engineering at the Centre for Biomedical Engineering, Department of Mechanical Engineering Sciences within the Faculty of Engineering and Physical Sciences at the University of Surrey since 2010.
He is an engineer by background, holding an Engineering degree (MEng Telecommunication Engineering, 2001) from the University of Valladolid, where he also carried doctoral research and was conferred the PhD degree - Summa Cum Laude - with a thesis on advanced biomedical signal processing of the electroencephalogram (EEG) in Alzheimer's disease in 2006.
Dr Abásolo started his research career working on the development of non-linear techniques for the analysis of brain activity recorded in EEGs for the diagnosis of Alzheimer's disease. He has subsequently applied this work to the characterisation of other biomedical signals (such as intracranial pressure, oxygen saturation and electrocardiogram recordings, among others) with many different advanced signal processing algorithms. His work has led to the publication of several highly cited papers in indexed journals (h-index of 24 based on Thomson Reuters, February 2018, or 26 based on Google Scholar, February 2018) and he has also been instrumental in securing research grants throughout his academic career.
Currently, Dr Abásolo's research interests lie mainly in non-linear biomedical signal processing, in particular the development of algorithms that can be applied to Alzheimer's disease diagnosis, the characterisation of healthy and pathological ageing using brain signals, EEG analysis in sleep studies, and electrocardiogram analysis in atrial fibrillation. He is also interested in deep learning techniques for EEG analysis and the identification of circadian and ultradian rhythms with advanced signal processing algorithms.
Dr Daniel Abásolo is a passionate advocate for biomedical engineering and has been championing the discipline both at the University of Surrey, for example as a member of the Engineering for Health project, and nationally, as an active member of the IET Healthcare Technologies Network Executive Committee. He is currently the Chairman of this committee and also represents the IET at the IMechE Biomedical Engineering Division.
He is also particularly enthusiastic about nurturing students' talent with research-intensive final year projects. This has led to significant success for the University of Surrey nationally, with medical/biomedical engineering students working under his supervision winning the IET Dennis Hill Award for best MEng/MSc project for four consecutive years and other IMechE Medical Engineering student awards also going to students from the University of Surrey.
University roles and responsibilities
- Programme Leader for BEng/MEng Biomedical Engineering
Affiliations and memberships
News
ResearchResearch interests
- Biomedical Signal Processing
- Non-linear Analysis
- Deep Learning
- Electroencephalogram
- Magnetoencephalogram
- Alzheimer's Disease
- Healthy and Pathological Ageing
- Sleep
- Intracranial Pressure
- Normal Pressure Hydrocephalus
- Electrocardiogram
- Atrial Fibrillation
- Circadian and Ultradian Rhythms
Research collaborations
Dr Raphaelle Winsky-Sommerer, University of Surrey, UK
Dr Jonathan Johnston, University of Surrey, UK
Dr Daan van der Veen, University of Surrey, UK
Prof Christopher James, University of Warwick, UK
Prof Chris Fry, University of Bristol, UK
Dr Javier Escudero, University of Edinburgh, UK
Dr Vladyslav Vyazovskiy University of Oxford, UK
Dr Herbert Jelinek, Charles Sturt University, Australia
Dr Alberto Fernández, Universidad Complutense Madrid, Spain
Research interests
- Biomedical Signal Processing
- Non-linear Analysis
- Deep Learning
- Electroencephalogram
- Magnetoencephalogram
- Alzheimer's Disease
- Healthy and Pathological Ageing
- Sleep
- Intracranial Pressure
- Normal Pressure Hydrocephalus
- Electrocardiogram
- Atrial Fibrillation
- Circadian and Ultradian Rhythms
Research collaborations
Dr Raphaelle Winsky-Sommerer, University of Surrey, UK
Dr Jonathan Johnston, University of Surrey, UK
Dr Daan van der Veen, University of Surrey, UK
Prof Christopher James, University of Warwick, UK
Prof Chris Fry, University of Bristol, UK
Dr Javier Escudero, University of Edinburgh, UK
Dr Vladyslav Vyazovskiy University of Oxford, UK
Dr Herbert Jelinek, Charles Sturt University, Australia
Dr Alberto Fernández, Universidad Complutense Madrid, Spain
Teaching
- FHEQ Level 6 (Year 3 BEng/MEng Medical Engineering): Biomedical Signal Processing (ENG3186)
- FHEQ Level 7 (MSc Biomedical Engineering): Instrumentation (ENGM186)
- FHEQ Level 7 (MSc Biomedical Engineering): Computer Methods in Biomedical Engineering Research (ENGM259)
- Supervision of final year projects (BEng/MEng Medical Engineering, MSc Biomedical Engineering)
Publications
Brain clocks track the deviations between predicted brain age and chronological age (brain age gaps, BAGs). These BAGs can be used to measure accelerated aging, monitoring deviations from the healthy brain trajectories associated with brain diseases and different cumulative burdens. However, the underlying biophysical mechanisms associated with BAGs in aging and dementia remain unclear. Here, we combine source space connectivity (EEG) with generative brain modeling in healthy controls (HCs) from the global south and north, alongside Alzheimer’s disease (AD) and behavioral variant frontotemporal dementia (bvFTD) patients (N=1,399). BAGs in aging were influenced by geography (south>north), income (low>high), sex (female>male), and education (low>high), with larger BAGs in patients, especially females with AD. Biophysical modeling revealed BAGs related to hyperexcitability and structural disintegration in aging, while hypoexcitability and severe disintegration were linked to dementia. Our work sheds light on the biophysical mechanisms of accelerated aging and dementia in diverse populations
Brain clocks, which quantify discrepancies between brain age and chronological age, hold promise for understanding brain health and disease. However, the impact of multimodal diversity (geographical, socioeconomic, sociodemographic, sex, neurodegeneration) on the brain age gap (BAG) is unknown. Here, we analyzed datasets from 5,306 participants across 15 countries (7 Latin American countries -LAC, 8 non-LAC). Based on higher-order interactions in brain signals, we developed a BAG deep learning architecture for functional magnetic resonance imaging (fMRI=2,953) and electroencephalography (EEG=2,353). The datasets comprised healthy controls, and individuals with mild cognitive impairment, Alzheimer's disease, and behavioral variant frontotemporal dementia. LAC models evidenced older brain ages (fMRI: MDE=5.60, RMSE=11.91; EEG: MDE=5.34, RMSE=9.82) compared to non-LAC, associated with frontoposterior networks. Structural socioeconomic inequality and other disparity-related factors (pollution, health disparities) were influential predictors of increased brain age gaps, especially in LAC (R2=0.37, F2=0.59, RMSE=6.9). A gradient of increasing BAG from controls to mild cognitive impairment to Alzheimer's disease was found. In LAC, we observed larger BAGs in females in control and Alzheimer's disease groups compared to respective males. Results were not explained by variations in signal quality, demographics, or acquisition methods. Findings provide a quantitative framework capturing the multimodal diversity of accelerated brain aging.Brain clocks, which quantify discrepancies between brain age and chronological age, hold promise for understanding brain health and disease. However, the impact of multimodal diversity (geographical, socioeconomic, sociodemographic, sex, neurodegeneration) on the brain age gap (BAG) is unknown. Here, we analyzed datasets from 5,306 participants across 15 countries (7 Latin American countries -LAC, 8 non-LAC). Based on higher-order interactions in brain signals, we developed a BAG deep learning architecture for functional magnetic resonance imaging (fMRI=2,953) and electroencephalography (EEG=2,353). The datasets comprised healthy controls, and individuals with mild cognitive impairment, Alzheimer's disease, and behavioral variant frontotemporal dementia. LAC models evidenced older brain ages (fMRI: MDE=5.60, RMSE=11.91; EEG: MDE=5.34, RMSE=9.82) compared to non-LAC, associated with frontoposterior networks. Structural socioeconomic inequality and other disparity-related factors (pollution, health disparities) were influential predictors of increased brain age gaps, especially in LAC (R2=0.37, F2=0.59, RMSE=6.9). A gradient of increasing BAG from controls to mild cognitive impairment to Alzheimer's disease was found. In LAC, we observed larger BAGs in females in control and Alzheimer's disease groups compared to respective males. Results were not explained by variations in signal quality, demographics, or acquisition methods. Findings provide a quantitative framework capturing the multimodal diversity of accelerated brain aging.
Back pain lifetime incidence is 60%-70%, while 12%-20% of older women have vertebral fractures (VFs), often with back pain. We aimed to provide objective evidence, currently lacking, regarding whether back pain and VFs affect physical activity (PA). We recruited 69 women with recent back pain (age 74.5 +/- 5.4 years). Low- (0.5 < g < 1.0), medium- (1.0 = 1.5) PA and walking time were measured (100 Hz for 7 days, hip-worn accelerometer). Linear mixed-effects models assessed associations between self-reported pain and PA, and group differences (VFs from spine radiographs/no-VF) in PA. Higher daily pain was associated with reduced low (beta = -0.12, 95% confidence interval, [-0.22, -0.03], p =.013) and medium-impact PA (beta = -0.11, 95% confidence interval, [-0.21, -0.01], p =.041), but not high-impact PA or walking time (p >.11). VFs were not associated with PA (all p >.2). Higher daily pain levels but not VFs were associated with reduced low- and medium-impact PA, which could increase sarcopenia and falls risk in older women with back pain.
Background: Nonlinear dynamical measures, such as fractal dimension (FD), entropy, and Lempel-Ziv complexity (LZC), have been extensively investigated individually for detecting information content in magnetoencephalograms (MEGs) from patients with Alzheimer’s dementia (AD). Objective: To compare systematically the performance of twenty conventional and recently introduced nonlinear dynamical measures in studying AD vs. mild cognitive impairment (MCI) and healthy control (HC) subjects using MEG. Methods: We compared twenty nonlinear measures to distinguish MEG recordings from 36 AD participants (mean age=74.06±6.95 years); 18 MCI participants (mean age=74.89±5.57 years, and 26 HC subjects (mean age=71.77±6.38 years) in different brain regions and also evaluated the effect of the length of MEG epochs on their performance. We also studied the correlation between these measures and cognitive performance based on the Mini Mental State Examination (MMSE). Results: The results obtained by LZC, zero-crossing rate (ZCR), FD, and dispersion entropy (DispEn) measures showed significant differences among the three groups. There was no significant difference between HC and MCI. The highest Hedge’s g effect sizes for HC vs. AD and MCI vs. AD were respectively obtained by Higuchi’s FD (HFD) and fuzzy DispEn (FuzDispEn) in the whole brain and was most prominent in left lateral. The results obtained by HFD and FuzDispEn had a significant correlation with the MMSE scores. DispEn-based techniques, LZC, and ZCR, compared with HFD, were less sensitive to epoch length in distinguishing HC form AD. Conclusions: FuzDispEn was the most consistent technique to distinguish MEG dynamical patterns in AD compared with HC and MCI.
Objective Functional seizures (FS) look like epileptic seizures but are characterized by a lack of epileptic activity in the brain. Approximately one in five referrals to epilepsy clinics are diagnosed with this condition. FS are diagnosed by recording a seizure using video-electroencephalography (EEG), from which an expert inspects the semiology and the EEG. However, this method can be expensive and inaccessible and can present significant patient burden. No single biomarker has been found to diagnose FS. However, the current limitations in FS diagnosis could be improved with machine learning to classify signal features extracted from EEG, thus providing a potentially very useful aid to clinicians. Methods The current study has investigated the use of seizure-free EEG signals with machine learning to identify subjects with FS from those with epilepsy. The dataset included interictal and preictal EEG recordings from 48 subjects with FS (mean age = 34.76 ± 10.55 years, 14 males) and 29 subjects with epilepsy (mean age = 38.95 ± 13.93 years, 18 males) from which various statistical, temporal, and spectral features from the five EEG frequency bands were extracted then analyzed with threshold accuracy, five machine learning classifiers, and two feature importance approaches. Results The highest classification accuracy reported from thresholding was 60.67%. However, the temporal features were the best performing, with the highest balanced accuracy reported by the machine learning models: 95.71% with all frequency bands combined and a support vector machine classifier. Significance Machine learning was much more effective than using individual features and could be a powerful aid in FS diagnosis. Furthermore, combining the frequency bands improved the accuracy of the classifiers in most cases, and the lowest performing EEG bands were consistently delta and gamma.
Psychogenic non-epileptic seizures (PNES) may resemble epileptic seizures but are not caused by epileptic activity. However, the analysis of electroencephalogram (EEG) signals with entropy algorithms could help identify patterns that differentiate PNES and epilepsy. Furthermore, the use of machine learning could reduce the current diagnosis costs by automating classification. The current study extracted the approximate sample, spectral, singular value decomposition, and Renyi entropies from interictal EEGs and electrocardiograms (ECG)s of 48 PNES and 29 epilepsy subjects in the broad, delta, theta, alpha, beta, and gamma frequency bands. Each feature-band pair was classified by a support vector machine (SVM), k-nearest neighbour (kNN), random forest (RF), and gradient boosting machine (GBM). In most cases, the broad band returned higher accuracy, gamma returned the lowest, and combining the six bands together improved classifier performance. The Renyi entropy was the best feature and returned high accuracy in every band. The highest balanced accuracy, 95.03%, was obtained by the kNN with Renyi entropy and combining all bands except broad. This analysis showed that entropy measures can differentiate between interictal PNES and epilepsy with high accuracy, and improved performances indicate that combining bands is an effective improvement for diagnosing PNES from EEGs and ECGs.
Background Structural income inequality – the uneven income distribution across regions or countries – could affect brain structure and function, beyond individual differences. However, the impact of structural income inequality on the brain dynamics and the roles of demographics and cognition in these associations remains unexplored. Methods Here, we assessed the impact of structural income inequality, as measured by the Gini coefficient on multiple EEG metrics, while considering the subject-level effects of demographic (age, sex, education) and cognitive factors. Resting-state EEG signals were collected from a diverse sample (countries = 10; healthy individuals = 1394 from Argentina, Brazil, Colombia, Chile, Cuba, Greece, Ireland, Italy, Turkey and United Kingdom). Complexity (fractal dimension, permutation entropy, Wiener entropy, spectral structure variability), power spectral and aperiodic components (1/f slope, knee, offset), as well as graph-theoretic measures were analysed. Findings Despite variability in samples, data collection methods, and EEG acquisition parameters, structural inequality systematically predicted electrophysiological brain dynamics, proving to be a more crucial determinant of brain dynamics than individual-level factors. Complexity and aperiodic activity metrics captured better the effects of structural inequality on brain function. Following inequality, age and cognition emerged as the most influential predictors. The overall results provided convergent multimodal metrics of biologic embedding of structural income inequality characterised by less complex signals, increased random asynchronous neural activity, and reduced alpha and beta power, particularly over temporoposterior regions. Conclusion These findings might challenge conventional neuroscience approaches that tend to overemphasise the influence of individual-level factors, while neglecting structural factors. Results pave the way for neuroscience-informed public policies aimed at tackling structural inequalities in diverse populations.
Hip-worn triaxial accelerometers are widely used to assess physical activity in terms of energy expenditure. Methods for classification in terms of different types of activity of relevance to the skeleton in populations at risk of osteoporosis are not currently available. This publication aims to assess the accuracy of four machine learning models on binary (standing and walking) and tertiary (standing, walking, and jogging) classification tasks in postmenopausal women. Eighty women performed a shuttle test on an indoor track, of which thirty performed the same test on an indoor treadmill. The raw accelerometer data were pre-processed, converted into eighteen different features and then combined into nine unique feature sets. The four machine learning models were evaluated using three different validation methods. Using the leave-one-out validation method, the highest average accuracy for the binary classification model, 99.61%, was produced by a k-NN Manhattan classifier using a basic statistical feature set. For the tertiary classification model, the highest average accuracy, 94.04%, was produced by a k-NN Manhattan classifier using a feature set that included all 18 features. The methods and classifiers within this study can be applied to accelerometer data to more accurately characterize weight-bearing activity which are important to skeletal health.
Hydrocephalus includes a range of conditions characterized by clinical symptoms, enlarged ventricles and disorders in cerebrospinal fluid (CSF) circulation. Infusion tests can be used to analyze CSF dynamics in patients with hydrocephalus. In infusion tests, intracranial pressure (ICP) is artificially raised, while the resulting ICP is measured in order to detect CSF circulation alterations. In this study, we analyzed 77 ICP signals recorded during infusion tests using the spectral turbulence (ST). Each signal was divided into four artifact-free epochs. The mean ST, , and the standard deviation of ST, SD[ST], were calculated for each epoch. Statistically significant differences were found between the basal phase of the infusion test and the remaining phases using and SD[ST] (p
The analysis of P-wave variability from the electrocardiogram (ECG) has been suggested as an early predictor of the onset of paroxysmal atrial fibrillation (PAF). Hence, a preventive treatment could be used to avoid the loss of normal sinus rhythm, thus minimising health risks and improving the patient's quality of life. In these previous studies the variability of different temporal and morphological P-wave features has been only analysed in a linear fashion. However, the electrophysiological alteration occurring in the atria before the onset of PAF has to be considered as an inherently complex, chaotic and non-stationary process. This work analyses the presence of non-linear dynamics in the P-wave progression before the onset of PAF through the application of the central tendency measure (CTM), which is a non-linear metric summarising the degree of variability in a time series. Two hour-length ECG intervals just before the arrhythmia onset belonging to 46 different PAF patients were analysed. In agreement with the invasively observed inhomogeneous atrial conduction preceding the onset of PAF, CTM for all the considered P-wave features showed higher variability when the arrhythmia was closer to its onset. A diagnostic accuracy around 80% to discern between ECG segments far from PAF and close to PAF was obtained with the CTM of the metrics considered. This result was similar to previous P-wave variability methods based on linear approaches. However, the combination of linear and non-linear methods with a decision tree improved considerably their discriminant ability up to 90%, thus suggesting that both dynamics could coexist at the same time in the fragmented depolarisation of the atria preceding the arrhythmia.
Diabetes is a disease of great and rising prevalence, with the obesity epidemic being a significant contributing risk factor. Duodenal–jejunal bypass liner (DJBL) is a reversible implant that mimics the effects of more aggressive surgical procedures, such as gastric bypass, to induce weight loss. We hypothesized that DJBL also influences the glucose dynamics in type II diabetes, based on the induced changes already demonstrated in other physiological characteristics and parameters. In order to assess the validity of this assumption, we conducted a quantitative analysis based on several nonlinear algorithms (Lempel–Ziv Complexity, Sample Entropy, Permutation Entropy, and modified Permutation Entropy), well suited to the characterization of biomedical time series. We applied them to glucose records drawn from two extreme cases available of DJBL implantation: before and after 10 months. The results confirmed the hypothesis and an accuracy of 86.4% was achieved with modified Permutation Entropy. Other metrics also yielded significant classification accuracy results, all above 70%, provided a suitable parameter configuration was chosen. With the Leave–One–Out method, the results were very similar, between 72% and 82% classification accuracy. There was also a decrease in entropy of glycaemia records during the time interval studied. These findings provide a solid foundation to assess how glucose metabolism may be influenced by DJBL implantation and opens a new line of research in this field.
The Cluster-Span Threshold (CST) is a recently introduced unbiased threshold for functional connectivity networks. This binarisation technique offers a natural trade-off of sparsity and density of information by balancing the ratio of closed to open triples in the network topology. Here we present findings comparing it with the Union of Shortest Paths (USP), another recently proposed objective method. We analyse standard network metrics of binarised networks for sensitivity to clinical Alzheimer’s disease in the Beta band of Electroencephalogram activity. We find that the CST outperforms the USP, as well as subjective thresholds based on fixing the network density, as a sensitive threshold for distinguishing differences in the functional connectivity between Alzheimer’s disease patients and control. This study provides the first evidence of the usefulness of the CST for clinical research purposes.
Alzheimer’s disease (AD) is neurodegenerative, caused by the progressive death of brain cells over time. One non-invasive approach to investigate AD is to use electroencephalogram (EEG) signals. The data are usually non-stationary with a strong background activity and noise which makes the analysis difficult leading to low performance in many real world applications including the detection of AD. In this study, we present a method based on local texture changes of EEG signals to differentiate AD patients from the healthy ones, using one-dimensional local binary patterns (1D-LBPs) coupled with support vector machines (SVM). Our proposed method maps the EEG data into a less detailed representation which is less sensitive to noise. A 10 fold cross validation performed at both the epoch and subject level show the discriminancy power of 1D-LBP feature vectors with application to AD data.
The aim of the present study was to analyze resting-state brain activity in patients with Parkinson's disease (PD), a degenerative disorder of the nervous system. Magnetoencephalography (MEG) signals were recorded with a 151-channel whole-head radial gradiometer MEG system in 18 early-stage untreated PD patients and 20 age-matched control subjects. Artifact-free epochs of 4 s (1250 samples) were analyzed with Lempel-Ziv complexity (LZC), applying two- and three-symbol sequence conversion methods. The results showed that MEG signals from PD patients are less complex than control subjects' recordings. We found significant group differences (p-values
Hydrocephalus includes a number of disorders characterised by clinical symptoms, enlarged ventricles (observable using neuroimaging techniques) and altered cerebrospinal fluid (CSF) dynamics. Infusion tests are one of the available procedures to study CSF circulation in patients with clinical and radiological features of hydrocephalus. In them, intracranial pressure (ICP) is deliberately raised and CSF circulation disorders evaluated through measurements of the resulting ICP. In this study, we analysed seventy-seven ICP signals recorded during infusion tests using four spectral-based parameters: median frequency (MF) and relative power (RP) in three frequency bands. These measures provide a novel perspective for the analysis of ICP signals in the frequency domain. Each signal was divided into four artefact-free epochs (corresponding to the basal, early infusion, plateau and recovery phases of the infusion study). The four spectral parameters were calculated for each epoch. We analysed differences between epochs of the infusion test and correlations between these epochs and patient data. Statistically significant differences (p
Hydrocephalus is a condition characterized by altered cerebrospinal fluid (CSF) dynamics and chronic rises in intracranial pressure (ICP). However, the reason why hydrocephalic physiologies fail to inhibit dangerously high ICP levels is not known. Infusion studies are used to raise ICP and evaluate CSF circulation disorders. In this pilot study, ICP signals recorded during infusion tests from 33 patients with normal pressure hydrocephalus and 36 patients having developed a secondary form of normal pressure hydrocephalus were characterized using Permutation Entropy (PE), a symbolic non-linear method to quantify complexity. Each ICP signal was divided into four epochs - baseline (before infusion begins), infusion, plateau, and recovery (after infusion has stopped) - and the mean PE was calculated for each epoch. Statistically significant differences were found between PE for most epochs (p
The aim of this study was to explore the ability of several spectral entropies to discriminate between spontaneous magnetoencephalographic (MEG) oscillations from 20 Alzheimer's disease (AD) patients and 21 controls. Hence, the relative spectral power (RSP) in classical frequency bands was calculated from the averaged power spectral density. Given the fact that the RSP can be viewed as a probability distribution function, the Shannon spectral entropy, Tsallis spectral entropy, generalized escort-Tsallis spectral entropy and Rényi spectral entropy were calculated from the RSP. Significant differences for each parameter were assessed with Mann-Whitney U test, whereas classification performance was studied using binary logistic regression. Results revealed an increase in the RSP of control subjects at beta and gamma bands, while AD patients showed an increase in the RSP values at delta and theta bands. Entropies obtained statistically significant lower values for AD patients than for controls. This issue suggests a significant decrease in irregularity of AD patients' MEG activity.
The aim of this study was to analyse the electroencephalogram (EEG) background activity in Alzheimer's disease (AD) with the Detrended Moving Average (DMA) method, a new approach to quantify correlation properties in non-stationary signals with underlying trends. EEGs were recorded from the 19 scalp loci of the international 10-20 system in 11 AD patients and 11 age-matched controls. Our results showed two scaling regions in all subjects' channels, with a clear bend when their corresponding slopes (alpha(1) and alpha(2)) were distinctly different. With the exception of electrode T4, the alpha(1) values were lower in control subjects than in AD patients, with significant differences at TS, P3, P4 and O1 (p < 0.01, Student's t-test). On the other hand, alpha(2) values were higher in control subjects than in AD patients, with significant differences only at F4. Furthermore, we evaluated the ability of alpha(2) to discriminate AD patients from control subjects at these electrodes using ROC plots. We obtained a maximum accuracy of 81.82% at O1 with alpha(1) and at F4 with alpha(2). These findings suggest that the scaling behaviour of the EEG is sensitive to AD and that the DMA method could help to increase our insight into brain dysfunction in AD.
Background: Down syndrome (DS) is considered the most frequent cause of early-onset Alzheimer’s disease (AD), and the typical pathophysiological signs are present in almost all individuals with DS by the age of 40. Despite of this evidence, the investigation on the pre-dementia stages in DS is scarce. In the present study we analyzed the complexity of brain oscillatory patterns and neuropsychological performance for the characterization of mild cognitive impairment (MCI) in DS. Materials and methods: Lempel-Ziv complexity (LZC) values from resting-state magnetoencephalography recordings and the neuropsychological performance in 28 patients with DS [control DS group (CN-DS) (n = 14), MCI group (MCI-DS) (n = 14)] and 14 individuals with typical neurodevelopment (CN-no-DS) were analyzed. Results: Lempel-Ziv complexity was lowest in the frontal region within the MCI-DS group, while the CN-DS group showed reduced values in parietal areas when compared with the CN-no-DS group. Also, the CN-no-DS group exhibited the expected pattern of significant increase of LZC as a function of age, while MCI-DS cases showed a decrease. The combination of reduced LZC values and a divergent trajectory of complexity evolution with age, allowed the discrimination of CN-DS vs. MCI-DS patients with a 92.9% of sensitivity and 85.7% of specificity. Finally, a pattern of mnestic and praxic impairment was significantly associated in MCI-DS cases with the significant reduction of LZC values in frontal and parietal regions (p = 0.01). Conclusion: Brain signal complexity measured with LZC is reduced in DS and its development with age is also disrupted. The combination of both features might assist in the detection of MCI within this population.
BACKGROUND: Nonlinear dynamical measures, such as fractal dimension (FD), entropy, and Lempel-Ziv complexity (LZC), have been extensively investigated individually for detecting information content in magnetoencephalograms (MEGs) from patients with Alzheimer's disease (AD).OBJECTIVETo compare systematically the performance of twenty conventional and recently introduced nonlinear dynamical measures in studying AD versus mild cognitive impairment (MCI) and healthy control (HC) subjects using MEG.METHODSWe compared twenty nonlinear measures to distinguish MEG recordings from 36 AD (mean age = 74.06±6.95 years), 18 MCI (mean age = 74.89±5.57 years), and 26 HC subjects (mean age = 71.77±6.38 years) in different brain regions and also evaluated the effect of the length of MEG epochs on their performance. We also studied the correlation between these measures and cognitive performance based on the Mini-Mental State Examination (MMSE).RESULTSThe results obtained by LZC, zero-crossing rate (ZCR), FD, and dispersion entropy (DispEn) measures showed significant differences among the three groups. There was no significant difference between HC and MCI. The highest Hedge's g effect sizes for HC versus AD and MCI versus AD were respectively obtained by Higuchi's FD (HFD) and fuzzy DispEn (FuzDispEn) in the whole brain and was most prominent in left lateral. The results obtained by HFD and FuzDispEn had a significant correlation with the MMSE scores. DispEn-based techniques, LZC, and ZCR, compared with HFD, were less sensitive to epoch length in distinguishing HC form AD.CONCLUSIONSFuzDispEn was the most consistent technique to distinguish MEG dynamical patterns in AD compared with HC and MCI.
Sample entropy (SampEn) is a nonlinear regularity index that requires the a priori selection of three parameters: the length of the sequences to be compared, m, the patterns similarity tolerance, r, and the number of samples under analysis, N. Appropriate values for m, r and N have been recommended and widely used in the literature for the application of SampEn to some physiological time series, such as heart rate, hormonal data, etc. However, no guidelines exist for the selection of that values in other cases. Therefore, an optimal parameters study should be required for the application of SampEn to not previously analyzed biomedical signals. In the present work, a thorough analysis on the optimal values for m, r and N is presented within the context of atrial fibrillation (AF) organization estimation, computed from surface electrocardiogram recordings. Recently, the evaluation of AF organization through SampEn, has revealed clinically useful information that could be used for a better treatment of this arrhythmia. The present study analyzed optimal SampEn parameter values within two different scenarios of AF organization estimation, such as the prediction of paroxysmal AF termination and the electrical cardioversion outcome in persistent AF. As a result, interesting recommendations about the selection of m, r and N, together with the relationship between N and the sampling rate (f(s)) were obtained. More precisely, (i) the proportion between N and f(s) should be higher than 1s and f(s) >= 256 Hz, (ii) overlapping between adjacent N-length windows does not improve AF organization estimation with respect to the analysis of non-overlapping windows, and (iii) values of m and r maximizing successful classification for the analyzed AF databases should be considered within a range wider than the proposed in the literature for heart rate analysis, i.e. m = 1 and m = 2 and r between 0.1 and 0.25 times the standard deviation of the data. (C) 2010 Elsevier Ireland Ltd. All rights reserved.
The aim of this study was to analyze the electroencephalogram (EEG) background activity in Alzheimer's disease (AD) with two non-linear methods: Approximate Entropy (ApEn) and Auto Mutual Information (AMI). ApEn quantifies the regularity in data, while AMI detects linear and non-linear dependencies in time series. EEGs were recorded from the 19 scalp loci of the international 10-20 system in 11 AD patients and 11 age-matched controls. ApEn was significantly lower in AD patients at electrodes O1, O2, P3 and P4 (p
Nocturnal oximetry is an attractive option for the diagnosis of obstructive sleep apnoea (OSA) syndrome because of its simplicity and low cost compared to polysomnography (PSG). The present study assesses nonlinear analysis of blood oxygen saturation (SaO(2)) from nocturnal oximetry as a diagnostic test to discriminate between OSA positive and OSA negative patients. A sample of 187 referred outpatients, clinically suspected of having OSA, was studied using nocturnal oximetry performed simultaneously with complete PSG. A positive OSA diagnosis was found for 111 cases, while the remaining 76 cases were classified as OSA negative. The following oximetric indices were obtained: cumulative time spent below a saturation of 90% (CT90), oxygen desaturation indices of 4% (ODI4), 3% (ODI3) and 2% (ODI2) and the delta index (Delta index). SaO(2) records were subsequently processed applying two nonlinear methods: central tendency measure (CTM) and Lempel-Ziv (LZ) complexity. Significant differences (p < 0.01) were found between OSA positive and OSA negative patients. Using CTM we obtained a sensitivity of 90.1% and a specificity of 82.9%, while with LZ the sensitivity was 86.5% and the specificity was 77.6%. CTM and LZ accuracies were higher than those provided by ODI4, ODI3, ODI2 and CT90. The results suggest that nonlinear analysis of SaO(2) signals from nocturnal oximetry could yield useful information in OSA diagnosis.
The analysis of resting-state brain activity recording in magnetoencephalograms (MEGs) with new algorithms of symbolic dynamics analysis could help obtain a deeper insight into the functioning of the brain and identify potential differences between males and females. Permutation Lempel-Ziv complexity (PLZC), a recently introduced non-linear signal processing algorithm based on symbolic dynamics, was used to evaluate the complexity of MEG signals in source space. PLZC was estimated in a broad band of frequencies (2–45 Hz), as well as in narrow bands (i.e., theta (4–8 Hz), alpha (8–12 Hz), low beta (12–20 Hz), high beta (20–30 Hz), and gamma (30–45 Hz)) in a sample of 98 healthy elderly subjects (49 males, 49 female) aged 65–80 (average age of 72.71 ± 4.22 for males and 72.67 ± 4.21 for females). PLZC was significantly higher for females than males in the high beta band at posterior brain regions including the precuneus, and the parietal and occipital cortices. Further statistical analyses showed that higher complexity values over highly overlapping regions than the ones mentioned above were associated with larger hippocampal volumes only in females. These results suggest that sex differences in healthy aging can be identified from the analysis of magnetoencephalograms with novel signal processing methods.
The aim of this study was to improve the diagnosis of Alzheimer's disease (AD) patients applying a blind source separation (BSS) and component selection procedure to their magnetoencephalogram (MEG) recordings. MEGs from 18 AD patients and 18 control subjects were decomposed with the algorithm for multiple unknown signals extraction. MEG channels and components were characterized by their mean frequency, spectral entropy, approximate entropy, and Lempel-Ziv complexity. Using Student's t-test, the components which accounted for the most significant differences between groups were selected. Then, these relevant components were used to partially reconstruct the MEG channels. By means of a linear discriminant analysis, we found that the BSS-preprocessed MEGs classified the subjects with an accuracy of 80.6%, whereas 72.2% accuracy was obtained without the BSS and component selection procedure.
The characterisation of healthy ageing of the brain could help create a fingerprint of normal ageing that might assist in the early diagnosis of neurodegenerative conditions. This study examined changes in resting state MEG permutation entropy due to age and gender in a sample of 220 healthy participants (98 males and 122 females, ages ranging between 7 and 84). Entropy was quantified using normalised permutation entropy and modified permutation entropy, with an embedding dimension of 5 and a lag of 1 as the input parameters for both algorithms. Effects of age were observed over the 5 regions of the brain i.e. anterior, central, posterior, and left and right lateral, with the anterior and central regions containing the highest permutation entropy. Statistically significant differences due to age were observed in the different brain regions for both genders, with the evolutions described using the fitting of polynomial regressions. Nevertheless, no significant differences between the genders were observed across all ages. These results suggest that the evolution of entropy in the background brain activity, quantified with permutation entropy algorithms, might be considered an alternative illustration of a ‘nominal’ physiological rhythm.
In this pilot study, a symbolic sequence decomposition method was used in conjunction with Shannon’s entropy to investigate the changes in electroencephalogram signals of 11 patients with Alzheimer’s disease and 11 age-matched control subjects. Results were statistically analysed by student t-test and later classified with receiver operating curves. Statistically significant differences between both groups were found at electrodes Fp1, O2, P3, T4 and T5. Sensitivity (defined as percentages of correctly classified patients) and specificity (defined as correctly classified controls) were evaluated using the receiver operating curves method. Accuracy of the methods was calculated according to sensitivity and specificity measures of electrodes showing statistically significant differences between the control group and Alzheimer's disease patients and ranged between 72.73-77.27%. These accuracy values were in agreement with previously published entropy studies on this data set. Although combining these methods did not provide any greater accuracy over previous findings, using a symbolic sequence decomposition method enhanced the data processing.
We appreciate the interest of Dr Tang in our article. Certainly, our previous results should be taken with caution due to the small database size. Nevertheless, it must be noted that this limitation was clearly recognized in our article. Furthermore, our hypothesis is completely justified from the current state of the art in the analysis of electroencephalogram (EEG) signals from Alzheimer's disease (AD) patients. We evaluated whether the multiscale entropy (MSE) analysis of the EEG background activity was useful to distinguish AD patients and controls. We do believe that further discussions about risk factors or related clinicophysiological protein aspects are clearly beyond the scope of our article. For the sake of completeness, we now detail some results that complement our previous analysis. They suggest that the MSE analysis can provide relevant information about the dynamics of AD patients' EEG data. Thus, we must reaffirm our conclusions, although we again acknowledge that further studies are needed.
The analysis of electroencephalograms (EEGs) of patients with Alzheimer’s disease (AD) could contribute to the diagnosis of this dementia. In this study, a new non-linear signal processing metric, distance-based Lempel-Ziv complexity (dLZC), is introduced to characterise changes between pairs of electrodes in EEGs in AD. When complexity in each signal arises from different sub-sequences dLZC would be greater than when similar sub-sequences are present in each signal. EEGs from 11 AD patients and 11 age-matched control subjects were analysed. The dLZC values for AD patients were lower than for control subjects for most electrode pairs, with statistically significant differences (p
The aim of this preliminary study was to asses whether a time series analysis from overnight pulse oximetry by means of Approximate Entropy (ApEn) could yield essential information on the diagnosis of obstructive sleep apnea (OSA) syndrome. We analyzed the oxygen saturation (SaO(2)) signals of 74 patients (44 with a positive diagnosis of OSA and 30 with a negative diagnosis of OSA) by means of ApEn, which quantified the regularity (or complexity) of time series. Results showed that recurrence of apnea events in patients with OSA determined a significant increase in ApEn values with a mean standard deviation (SD) of 1.07 +/- 0.30. A mean +/- SD ApEn value of 0.47 +/- 0.25 was estimated in patients without OSA. We obtained an area under the ROC curve of 0.94. The optimum threshold was selected at 0.81, where we achieved a 79,5% sensitivity and 90% specificity. Further analyses are necessary with new and larger data set to test the potential value of our methodology prospectively.
Conference Title: 2022 44th Annual International Conference of the IEEE Engineering in Medicine & Biology Society (EMBC) Conference Start Date: 2022, July 11 Conference End Date: 2022, July 15 Conference Location: Glasgow, Scotland, United KingdomPsychogenic non-epileptic seizures (PNES) are attacks that resemble epilepsy but are not associated with epileptic brain activity and are regularly misdiagnosed. The current gold standard method of diagnosis is expensive and complex. Electroencephalogram (EEG) analysis with machine learning could improve this. A k-nearest neighbours (kNN) and support vector machine (SVM) were used to classify EEG connectivity measures from 48 patients with PNES and 29 patients with epilepsy. The synchronisation method - correlation or coherence - and the binarisation threshold were defined through experimentation. Ten network parameters were extracted from the synchronisation matrix. The broad, delta, theta, alpha, beta, gamma, and combined ‘all’ frequency bands were compared along with three feature selection methods: the full feature set (no selection), light gradient boosting machine (LGBM) and k-Best. Coherence was the highest performing synchronisation method and 0.6 was the best coherence threshold. The highest balanced accuracy was 89.74%, produced by combining all six frequency bands and selecting features with LGBM, classified by the SVM. This method returned a comparatively high accuracy but at a high computation cost. Future research should focus on identifying specific frequency bands and network parameters to reduce this cost. Clinical relevance - This study found that EEG connectivity and machine learning methods can be used to differentiate PNES from epilepsy using interictal recordings to a high accuracy. Thus, this method could be an effective tool in assisting clinicians in PNES diagnosis without a video- EEG recording of a habitual seizure.
In the present study an attempt was made to quantify and characterise the changes in the intracranial pressure (ICP) waveform over the wide pressure range covered during infusion studies by means of the central tendency measure (CTM). CTM is a non-linear approach using continuous chaotic modelling that summarises the degree of variability in a signal.
Biomedical signals are measurable time series that describe a physiological state of a biological system. Entropy algorithms have been previously used to quantify the complexity of biomedical signals, but there is a need to understand the relationship of entropy to signal processing concepts. In this study, ten synthetic signals that represent widely encountered signal structures in the field of signal processing were created to interpret permutation, modified permutation, sample, quadratic sample and fuzzy entropies. Subsequently, the entropy algorithms were applied to two different databases containing electroencephalogram (EEG) signals from epilepsy studies. Transitions from randomness to periodicity were successfully detected in the synthetic signals, while significant differences in EEG signals were observed based on different regions and states of the brain. In addition, using results from one entropy algorithm as features and the k-nearest neighbours algorithm, maximum classification accuracies in the first EEG database ranged from 63% to 73.5%, while these values increased by approximately 20% when using two different entropies as features. For the second database, maximum classification accuracy reached 62.5% using one entropy algorithm, while using two algorithms as features further increased that by 10%. Embedding entropies (sample, quadratic sample and fuzzy entropies) are found to outperform the rest of the algorithms in terms of sensitivity and show greater potential by considering the fine-tuning possibilities they offer. On the other hand, permutation and modified permutation entropies are more consistent across different input parameter values and considerably faster to calculate.
The aim of this pilot study was to assess the usefulness of independent component analysis (ICA) to detect cardiac artifacts and power line interferences in magnetoencephalogram (MEG) recordings. We recorded MEG signals from six subjects with, a 148-channel whole-head magnetometer (MAGNES 2500 WH, 4D Neuroimaging). Epochs of 50 s with power line noise, cardiac, and ocular artifacts were selected for analysis. We applied a statistical criterion to determine the number of sources, and a robust ICA algorithm to decompose the MEG epochs. Skewness, kurtosis, and a spectral metric were used to mark the studied artifacts. We found that the power fine interference could be easily detected by its frequency characteristics. Moreover, skewness outperformed kurtosis when identifying the cardiac artifact.
•Age, sex, education, and cognition modulate electrophysiological brain dynamics•Age and cognition are the most robust predictors of EEG signals•Education and sex have a lesser influence as predictors of EEG signals•Periodic spectral and graph-theoretic measures best captured individual differences Diversity in brain health is influenced by individual differences in demographics and cognition. However, most studies on brain health and diseases have typically controlled for these factors rather than explored their potential to predict brain signals. Here, we assessed the role of individual differences in demographics (age, sex, and education; n = 1,298) and cognition (n = 725) as predictors of different metrics usually used in case-control studies. These included power spectrum and aperiodic (1/f slope, knee, offset) metrics, as well as complexity (fractal dimension estimation, permutation entropy, Wiener entropy, spectral structure variability) and connectivity (graph-theoretic mutual information, conditional mutual information, organizational information) from the source space resting-state EEG activity in a diverse sample from the global south and north populations. Brain-phenotype models were computed using EEG metrics reflecting local activity (power spectrum and aperiodic components) and brain dynamics and interactions (complexity and graph-theoretic measures). Electrophysiological brain dynamics were modulated by individual differences despite the varied methods of data acquisition and assessments across multiple centers, indicating that results were unlikely to be accounted for by methodological discrepancies. Variations in brain signals were mainly influenced by age and cognition, while education and sex exhibited less importance. Power spectrum activity and graph-theoretic measures were the most sensitive in capturing individual differences. Older age, poorer cognition, and being male were associated with reduced alpha power, whereas older age and less education were associated with reduced network integration and segregation. Findings suggest that basic individual differences impact core metrics of brain function that are used in standard case-control studies. Considering individual variability and diversity in global settings would contribute to a more tailored understanding of brain function.
Analysis of electrophysiological signals recorded from the brain with Lempel-Ziv (LZ) complexity, a measure based on coarse-graining of the signal, can provide valuable insights into understanding brain activity. LZ complexity of local field potential signals recorded from the neocortex of 11 adult male Wistar-Kyoto rats in different vigilance states - waking, non-rapid-eye movement (NREM) and REM sleep - was estimated with different coarse-graining techniques (median, LZCm, and k-means, LZCkm). Furthermore, surrogate data were used to test the hypothesis that LZ complexity results reveal effects accounted for by temporal structure of the signal, rather than merely its frequency content. LZ complexity values were significantly lower in NREM sleep as compared to waking and REM sleep, for both real and surrogate signals. LZCkm and LZCm values were similar, although in NREM sleep the values deviated in some epochs, where signals also differed significantly in terms of temporal structure and spectral content. Thus, the interpretation of LZ complexity results should take into account the specific algorithm used to coarse-grain the signal. Moreover, the occurrence of high amplitude slow waves during NREM sleep determines LZ complexity to a large extent, but characteristics such as the temporal sequence of slow waves or cross-frequency interactions might also play a role. © Springer International Publishing Switzerland 2014.
Understanding the dynamics of brain activity manifested in the EEG, local field potentials (LFP), and neuronal spiking is essential for explaining their underlying mechanisms and physiological significance. Much has been learned about sleep regulation using conventional EEG power spectrum, coherence, and period-amplitude analyses, which focus primarily on frequency and amplitude characteristics of the signals and on their spatio-temporal synchronicity. However, little is known about the effects of ongoing brain state or preceding sleep-wake history on the nonlinear dynamics of brain activity. Recent advances in developing novel mathematical approaches for investigating temporal structure of brain activity based on such measures, as Lempel-Ziv complexity (LZC) can provide insights that go beyond those obtained with conventional techniques of signal analysis. Here, we used extensive data sets obtained in spontaneously awake and sleeping adult male laboratory rats, as well as during and after sleep deprivation, to perform a detailed analysis of cortical LFP and neuronal activity with LZC approach. We found that activated brain states-waking and rapid eye movement (REM) sleep are characterized by higher LZC compared with non-rapid eye movement (NREM) sleep. Notably, LZC values derived from the LFP were especially low during early NREM sleep after sleep deprivation and toward the middle of individual NREM sleep episodes. We conclude that LZC is an important and yet largely unexplored measure with a high potential for investigating neurophysiological mechanisms of brain activity in health and disease.
Objective: We propose a novel complexity measure to overcome the deficiencies of the widespread and powerful multiscale entropy (MSE), including, MSE values may be undefined for short signals, and MSE is slow for real-time applications. Methods: We introduce multiscale dispersion entropy (DisEn - MDE) as a very fast and powerful method to quantify the complexity of signals. MDE is based on our recently developed DisEn, which has a computation cost of O(N), compared with O(N2) for sample entropy used in MSE. We also propose the refined composite MDE (RCMDE) to improve the stability of MDE. Results: We evaluate MDE, RCMDE, and refined composite MSE (RCMSE) on synthetic signals and three biomedical datasets. The MDE, RCMDE, and RCMSE methods show similar results, although the MDE and RCMDE are faster, lead to more stable results, and discriminate different types of physiological signals better than MSE and RCMSE. Conclusion: For noisy short and long time series, MDE and RCMDE are noticeably more stable than MSE and RCMSE, respectively. For short signals, MDE and RCMDE, unlike MSE and RCMSE, do not lead to undefined values. The proposed MDE and RCMDE are significantly faster than MSE and RCMSE, especially for long signals, and lead to larger differences between physiological conditions known to alter the complexity of the physiological recordings.
Alzheimer's disease (AD) is one of the most prominent neurodegenerative disorders. The aim of this study is to analyze the magnetoencephalogram (MEG) background activity in AD patients using sample entropy (SampEn) and multiscale entropy (MSE). The former quantifies the signal regularity, while the latter is a complexity measure. These concepts, irregularity and complexity, are linked although the relationship is not straightforward. Five minutes of recording were acquired with a 148-channel whole-head magnetometer in 20 patients with probable AD and 21 control subjects. Our results show that MEG recordings are less complex and more regular in AD patients than in control subjects. Significant differences between both groups were found in some MEG channels with both methods (p
The goal of this study was to analyze the magnetoencephalogram (MEG) background activity in patients,with Alzheimer's disease (AD) using the auto mutual information (AMI). Applied to time series, AMI provides a measure of future points predictability from past points. Five minutes of recording were acquired with a 148-channel wholehead magnetometer (AIIAGNES 2500 NVH, 413 Neuroimaging) in 12 patients with probable AD and 12 elderly control subjects. Artifact-free epochs of 20 seconds (3392 points, sample frequency of 169.6 Hz) were selected for our study. Our results showed that the absolute values of the averaged decline rate of AIM were lower in AD patients than in control subjects for all channels. In addition, there were statistically significant differences (p < 0.01, Student's t-test) in most channels. These preliminary results suggest that neuronal dysfunction in AD is associated with differences in the dynamical processes underlying the MEG recording.
Conference Title: 2022 44th Annual International Conference of the IEEE Engineering in Medicine & Biology Society (EMBC) Conference Start Date: 2022, July 11 Conference End Date: 2022, July 15 Conference Location: Glasgow, Scotland, United KingdomAlzheimer's Disease (AD) is the most common form of dementia. Mild Cognitive Impairment (MCI) is the term given to the stage describing prodromal AD and represents a ‘risk factor’ in early-stage AD diagnosis from normal cognitive decline due to ageing. The electroencephalogram (EEG) has been studied extensively for AD characterization, but reliable early-stage diagnosis continues to present a challenge. The aim of this study was to introduce a novel way of classifying between AD patients, MCI subjects, and age-matched healthy control (HC) subjects using EEG-derived feature images and deep learning techniques. The EEG recordings of 141 age-matched subjects (52 AD, 37 MCI, 52 HC) were converted into 2D greyscale images representing the Pearson correlation coefficients and the distance Lempel-Ziv Complexity (dLZC) between the 21 EEG channels. Each feature type was computed from EEG epochs of 1s, 2s, 5s and 10s segmented from the original recording. The CNN architecture AlexNet was modified and employed for this three-way classification task and a 70/30 split was used for training and validation with each of the different epoch lengths and EEG-derived images. Whilst a maximum classification accuracy of 73.49% was obtained using dLZC-derived images from 10s epochs as input to the model, the classification accuracy reached 98.13% using the images obtained from Pearson correlation coefficients and 5s epochs. Clinical Relevance— The preliminary findings from this study show that deep learning applied to the analysis of the EEG can classify subjects with accuracies close to 100%
Non-linear analysis of the electroencephalogram (EEG) background activity can help to obtain a better understanding of abnormal dynamics in the brain. The aim of this study was to analyze the regularity of the EEG time series of Alzheimer's disease (AD) Patients to test the hypothesis that the irregularity of the AD patients' EEG is lower than that of age-matched controls. We recorded the EEG from 19 scalp electrodes in 11 AD patients and 11 age-matched controls and estimated the Approximate Entropy (ApEn). ApEn is a non-linear method that can be used to quantify the irregularity of a time series. Larger values correspond to more irregularity. We evaluated different values for input parameters m and r to estimate ApEn and concluded that m=1 and r=0.25 times the SD of the time series were the optimum choices. With these parameters, ApEn was significantly lower in the AD patients at the P3, P41 O1 and O2 (p < 0.01) electrodes. The decreased irregularity found in the EEG of AD patients in the parietal and occipital regions leads us to think that regularity analysis of the EEG with ApEn could be a useful tool to increase our insight into brain dysfunction in Alzheimer's disease.
The goal of this study was to analyze the magnetoencephalogram (MEG) background activity in patients with Alzheimer's disease (AD) using a nonlinear forecasting measure. It is a nonparametric method to quantify the predictability of time series. Five minutes of recording were acquired with a 148-channel whole-head magnetometer in 15 patients with probable AD and 15 elderly control subjects. Stationary epochs of 5 seconds (848 points, sample frequency of 169.55 Hz) were selected. Our results showed that AD patients' MEGs were more predictable than controls' recordings. Additionally, an accuracy of 76.7% (80.0% sensitivity; 73.3% specificity) was reached using a receiver operating characteristic curve. These preliminary results suggest the usefulness of nonlinear forecasting to gain a better understanding of dynamical processes underlying the MEG recording.
The complexity of the intracranial pressure (ICP) signal decreases with intracranial hypertension in children with acute brain injury as well as during infusion studies in adults with hydrocephalus. In this study we have analysed the pressure signal obtained in the lumbar subarachnoid space during infusion testing. The pulse amplitude rises when the ICP is increased by additional external volume. Our objective was to determine the relative influence of the pressure range and pulse amplitude on the loss of complexity observed during infusion-related intracranial hypertension.
Sample Entropy (SampEn) is a nonlinear regularity index that requires the a priori selection of three parameters: the length of the sequences to be compared, m, the patterns similarity tolerance, r, and the number of samples under analysis, N. Appropriate values for m, r and N have been recommended in some cases, such as heart rate, hormonal data, etc., but no guidelines exist for the selection of that values. Hence, an optimal parameters study should be required for the application of SampEn to not previously analyzed biomedical signals. In this work, a thorough analysis on the optimal SampEn parameter values within two different scenarios of AF organization estimation, such as the prediction of paroxysmal AF termination and the electrical cardioversion outcome in persistent AF, is presented. Results indicated that, (i) the proportion between N and the sampling rate (f) should be higher than one second and f ≥ 256 Hz, (ii) overlapping between adjacent N-length windows does not improve organization estimation and (iii) values of m and r maximizing classification should be considered within a range wider than the proposed in the literature for heart rate analysis.
A Real-time Treadmill Speed Control Algorithm (RTSCA) has been developed for gait rehabilitation of children with cerebral palsy (CP). The objective of the work described in this paper was to investigate the feasibility of the RTSCA prior to use by children with CP. Thirteen healthy subjects aged between 19 and 25 were recruited to walk on the treadmill using conventional speed buttons without the virtual reality (VR) environment, and the RTSCA with and without VR. The participants were asked to undertake three treadmill tests and to complete a questionnaire to provide feedback on the control of the treadmill. The descriptive results show that for 10 participants changing walking speed from stationary when using the RTSCA was similar or more comfortable to using conventional treadmill speed control buttons. For those who found it less comfortable the core issue was insufficient time to practise with the system. All the participants were satisfied with the safety and the performance of the RTSCA when incorporated into the VR scenario. A Wilcoxon test was conducted to examine whether there was a significant difference between walking speeds on the treadmill when using the conventional speed buttons and the RTSCA. The results showed that participants walked at significantly higher speeds when using the RTSCA. This may suggest that they walked more naturally or confidently on the treadmill when using the RTSCA as compared to the use of conventional treadmill speed control buttons.
In this proof-of-principle study we analyzed intracranial electroencephalogram recordings in patients with intractable focal epilepsy. We contrast two implementations of Independent Component Analysis (ICA) - Ensemble (or spatial) ICA (E-ICA) and Space-Time ICA (ST-ICA) in separating out the ictal components underlying the measurements. In each case we assess the outputs of the ICA algorithms by means of a non-linear method known as the Lempel-Ziv (LZ) complexity. LZ complexity quantifies the complexity of a time series and is well suited to the analysis of non-stationary biomedical signals of short length. Our results show that for small numbers of intracranial recordings, standard E-ICA results in marginal improvements in the separation as measured by the LZ complexity changes. ST-ICA using just 2 recording channels both near and far from the epileptic focus result in more distinct ictal components--although at this stage there is a subjective element to the separation process for ST-ICA. Our results are promising showing that it is possible to extract meaningful information from just 2 recording electrodes through ST-ICA, even if they are not directly over the seizure focus. This work is being further expanded for seizure onset analysis.
Maturation and ageing, which can be characterised by the dynamic changes in brain morphology, can have an impact on the physiology of the brain. As such, it is possible that these changes can have an impact on the magnetic activity of the brain recorded using magnetoencephalography. In this study changes in the resting state brain (magnetic) activity due to healthy ageing were investigated by estimating the complexity of magnetoencephalogram (MEG) signals. The main aim of this study was to identify if the complexity of background MEG signals changed significantly across the human lifespan for both males and females. A sample of 177 healthy participants (79 males and 98 females aged between 21 and 80 and grouped into 3 categories i.e., early-, mid- and late-adulthood) was used in this investigation. This investigation also extended to evaluating if complexity values remained relatively stable during the 5 min recording. Complexity was estimated using permutation Lempel-Ziv complexity, a recently introduced complexity metric, with a motif length of 5 and a lag of 1. Effects of age and gender were investigated in the MEG channels over 5 brain regions, i.e., anterior, central, left lateral, posterior, and, right lateral, with highest complexity values observed in the signals recorded by the channels over the anterior and central regions of the brain. Results showed that while changes due to age had a significant effect on the complexity of the MEG signals recorded over 5 brain regions, gender did not have a significant effect on complexity values in all age groups investigated. Moreover, although some changes in complexity were observed between the different minutes of recording, due to the small magnitude of the changes it was concluded that practical significance might outweigh statistical significance in this instance. The results from this study can contribute to form a fingerprint of the characteristics of healthy ageing in MEGs that could be useful when investigating changes to the resting state activity due to pathology.
Neuronal outputs are complex signals of dynamically integrated excitatory and inhibitory components. Decreased synaptic inhibition in a neuronal network increases excitability and multiple spiking in neurons. Synchronized multiple spiking among a neuronal population further generates rhythmic field potentials and this epileptiform activity can propagate in the brain and cause seizures. Pharmacological interventions that reduce rhythmicity of epileptiform activity may have antiepileptic potentials. We evaluated the Lempel-Ziv (LZ) complexity for identifying rhythmicity in population spikes recorded in granule cells of the murine dentate gyrus in vitro. Blocking synaptic inhibition by the GABAA receptor antagonist, bicuculline, caused epileptiform population spikes, and we found that the LZ complexity of the signal was significantly reduced. Moreover, the GABAB receptor agonist, baclofen, reduced the amplitude of the epileptiform population spike and we found that it increased LZ complexity. The results show that LZ complexity is sensitive to pharmacological interventions that apparently alter rhythmicity of neuronal outputs by desynchronizing neuronal population firing. This novel approach in neuronal signal processing may be used to identify new antiepileptic targets. © Springer International Publishing Switzerland 2014.
Alzheimer's disease (AD) is the most frequent form of dementia in western countries. An early detection would be beneficial, but currently diagnostic accuracy is relatively poor. In this study, differences in information content between cortical areas in 12 AD patients and 11 control subjects were assessed with Kullback-Leibler (KL) entropy. KL entropy measures the degree of similarity between two probability distributions. EEGs were recorded from 19 scalp electrodes and KL entropy values of the EEGs in both groups were estimated for the local, distant and interhemispheric electrodes. KL entropy values were lower in AD patients than in age-matched control subjects, with significant effects for diagnosis and brain region (p < 0.05, two-way ANOVA). No significant interaction for diagnosis X region was found (p = 0.7671). Additionally a one-way ANOVA showed that KL entropy values were significantly lower in AD patients (p < 0.05) for the distant electrodes on the right hemisphere. These results suggest that KL entropy highlights information content changes in the EEG due to AD. However, further studies are needed to address the possible usefulness of KL entropy in the characterisation and early detection of AD.
This study assesses the connectivity alterations caused by Alzheimer's disease (AD) and mild cognitive impairment (MCI) in magnetoencephalogram (MEG) background activity. Moreover, a novel methodology to adaptively extract brain rhythms from the MEG is introduced. This methodology relies on the ability of empirical mode decomposition to isolate local signal oscillations and constrained blind source separation to extract the activity that jointly represents a subset of channels. Inter-regional MEG connectivity was analysed for 36 AD, 18 MCI and 26 control subjects in δ, θ, α and β bands over left and right central, anterior, lateral and posterior regions with magnitude squared coherence—c(f). For the sake of comparison, c(f) was calculated from the original MEG channels and from the adaptively extracted rhythms. The results indicated that AD and MCI cause slight alterations in the MEG connectivity. Computed from the extracted rhythms, c(f) distinguished AD and MCI subjects from controls with 69.4% and 77.3% accuracies, respectively, in a full leave-one-out cross-validation evaluation. These values were higher than those obtained without the proposed extraction methodology.
The spurious increase in coherence of electroencephalogram (EEG) signals between distant electrode points has long been understood to be due to volume conduction of the EEG signal. Reducing the volume conduction components of EEG recordings in pre-processing attenuates this. However, the effect of volume conduction on non-linear signal processing of EEG signals is yet to be fully described. This pilot study aimed to investigate the impact of volume conduction on results calculated with a distance based, bivariate form of Lempel-Ziv Complexity (dLZC) by analyzing EEG signals from Alzheimer's disease (AD) patients and healthy age-matched controls with and without pre-processing with Current Source Density (CSD) transformation. Spurious statistically significant differences between AD patients and control's EEG signals seen without CSD pre-processing were not seen with CSD volume conduction mitigation. There was, however, overlap in the region of electrodes which were seen to hold this statistically significant information. These results show that, while previously published findings are still valid, volume conduction mitigation is required to ensure non-linear signal processing methods identify changes in signals only due to the purely local signal alone.
Symbolic dynamic analysis (SDA) methods have been applied to biomedical signals and have been proven efficient in characterising differences in the electroencephalogram (EEG) in various conditions (e.g., epilepsy, Alzheimer’s and Parkinson’s diseases). In this study, we investigated the use of SDA on EEGs recorded during sleep. Lempel-Ziv Complexity (LZC), Permutation Entropy (PE), Permutation Lempel-Ziv Complexity (PLZC), as well as power spectral analysis based on the fast Fourier transform (FFT), were applied to 8-h sleep EEG recordings in healthy men (n=31) and women (n=29), aged 20-74 years. The results of the SDA methods and FFT analysis were compared and the effects of age and sex were investigated. Surrogate data were used to determine whether the findings with SDA methods truly reflected changes in non-linear dynamics of the EEG and not merely changes in the power spectrum. The surrogate data analysis showed that LZC merely reflected spectral changes in EEG activity, whereas PE and PLZC reflected genuine changes in the non-linear dynamics of the EEG. All three SDA techniques distinguished the vigilance states (i.e. wakefulness, REM sleep, NREM sleep and its sub stages: stage 1, stage 2 and slow wave sleep). Complexity of the sleep EEG increased with ageing. Sex on the other hand did not affect the complexity values assessed with any of these three SDA methods, even though FFT detected sex differences. This study shows that SDA provides additional insights into the dynamics of sleep EEG and how it is affected by ageing.
The advent of new signal processing methods, such as non-linear analysis techniques, represents a new perspective which adds further value to brain signals' analysis. Particularly, Lempel-Ziv's Complexity (LZC) has proven to be useful in exploring the complexity of the brain electromagnetic activity. However, an important problem is the lack of knowledge about the physiological determinants of these measures. Although a correlation between complexity and connectivity has been proposed, this hypothesis was never tested in vivo. Thus, the correlation between the microstructure of the anatomic connectivity and the functional complexity of the brain needs to be inspected. In this study we analyzed the correlation between LZC and fractional anisotropy (FA), a scalar quantity derived from diffusion tensors that is particularly useful as an estimate of the functional integrity of myelinated axonal fibers, in a group of sixteen healthy adults (all female, mean age 65.56±6.06 years, intervals 58-82). Our results showed a positive correlation between FA and LZC scores in regions including clusters in the splenium of the corpus callosum, cingulum, parahipocampal regions and the sagittal stratum. This study supports the notion of a positive correlation between the functional complexity of the brain and the microstructure of its anatomical connectivity. Our investigation proved that a combination of neuroanatomical and neurophysiological techniques may shed some light on the underlying physiological determinants of brain's oscillations.
Hydrocephalus is a condition characterised by enlarged cerebral ventricles, which in turn affects intracranial pressure (ICP); however, the mechanisms regulating ICP are not fully understood. A non-linear signal processing approach was applied to ICP signals measured during infusion studies from patients with two forms of hydrocephalus, in a bid to compare the differences. This is the first study of its kind. The two forms of hydrocephalus were idiopathic normal pressure hydrocephalus (iNPH) and secondary normal pressure hydrocephalus (SH). Following infusion tests, the Lempel-Ziv (LZ) complexity was calculated from the iNPH and SH ICP signals. The LZ complexity values were averaged for the baseline, infusion, plateau and recovery stages of the tests. It was found that as the ICP increased from basal levels, the LZ complexities decreased, reaching their lowest during the plateau stage. However, the complexities computed from the SH ICP signals decreased to a lesser extent when compared to the iNPH ICP signals. Furthermore, statistically significant differences were found between the plateau and recovery stage complexities when comparing the iNPH and SH results (p
The auto mutual information function (AMIF) evaluates the signal predictability by assessing linear and non-linear dependencies between two measurements taken from a single time series. Furthermore, the AMIF rate of decrease (AMIFRD) is correlated with signal entropy. This metric has been used to analyze biomedical data, including cardiac and brain activity recordings. Hence, the AMIFRD can be a relevant parameter in the context of biomedical signal analysis. Thus, in this pilot study, we have analyzed a synthetic sequence (a Lorenz system) and real biosignals (electroencephalograms recorded with eyes open and closed) with the AMIFRD. We aimed at illustrating the application of this parameter to biomedical time series. Our results show that the AMIFRD can detect changes in the non-linear dynamics of a sequence and that it can distinguish different physiological conditions.
The Surrey Virtual Rehabilitation System (SVRS) was developed for clinical use in physiotherapy for children with cerebral palsy (CP). The overall aim of this work was to evaluate the practicality of the SVRS for three simple lower extremity exercises. Two children with CP, two clinical engineering trainees and a physiotherapist performed the tests whilst parents and a paediatric physiotherapist observed the exercise sessions. The feedback collected during an open-discussion and the descriptive analysis of responses to 15 closed-ended questions suggest that the participants were satisfied with the practicality of the SVRS. Outcome measures derived from data collected during the sessions indicate that the SVRS may provide clinically relevant feedback on the performance of patients for themselves and their treating clinicians. In conclusion, the SVRS appears practical for rehabilitation purposes and is worthy of further evaluation and development. Copyright © 2014 Inderscience Enterprises Ltd.
Hydrocephalus includes a range of disorders characterized by clinical symptoms, abnormal brain imaging and altered cerebrospinal fluid (CSF) dynamics. Infusion tests can be used to study CSF circulation in patients with hydrocephalus. In them, intracranial pressure (ICP) is deliberately raised and CSF circulation disorders evaluated through measurements of the resulting ICP. In this study, we analyzed 77 ICP signals recorded during infusion tests using the spectral entropy (SE). Each signal was divided into four artifact-free epochs. The mean SE, , and the standard deviation of SE, SD[SE], were calculated for each epoch. Statistically significant differences were found between phases of the infusion test using and SD[SE] (p
Alzheimer's disease (AD) is one of the most prominent neurodegenerative disorders. The aim of this research work is to study the magnetoencephalogram (MEG) background activity in AD patients using two scaling analysis methods: detrended fluctuation analysis (DFA) and backward detrended moving average (BDMA). Both measures have been designed to quantify correlations in noisy and non-stationary signals. Five minutes of recording were acquired with a 148-channel whole-head magnetometer in 15 patients with probable AD and 15 control subjects. Both DFA and BDMA exhibited two scaling regions with different slopes. Significant differences between both groups were found in the second region of DFA and in the first region of BDMA (p < 0.01, Student's t-test). Using receiver operating characteristic curves, accuracies of 83.33% with DFA and of 80% with BDMA were reached. Our findings show the usefulness of these scaling analysis methods to increase our insight into AD.
The goal of this study was to analyze the magnetoencephalogram (MEG) background activity in patients with Alzheimer's disease (AD) using a regularity measure: approximate entropy (ApEn). This measure was computed for a broad band (0.5-40 Hz) as well as typical frequency bands (delta, theta, alpha, beta and gamma). Five minutes of recording were acquired with a 148-channel whole-head magnetometer in 15 patients with probable AD and 15 elderly control subjects. Our results showed that AD patients' MEGs were more regular than controls' recordings at all frequency bands, with the exception of beta. Additionally, there were statistically significant differences (p ⟨ 0.01, Student's t-test) at the broad and delta bands. Using receiver operating characteristic curves, the highest accuracy (83.33%) was reached at delta band. These results suggest the usefulness of ApEn to gain a better understanding of dynamical processes underlying the MEG recording.
Functional connectivity has proven useful to characterise electroencephalogram (EEG) activity in Alzheimer’s disease (AD). However, most current functional connectivity analyses have been static, disregarding any potential variability of the connectivity with time. In this pilot study, we compute short-time resting state EEG functional connectivity based on the imaginary part of coherency for 12 AD patients and 11 controls. We derive binary unweighted graphs using the cluster-span threshold, an objective binary threshold. For each short-time binary graph, we calculate its local clustering coefficient (Cloc), degree (K), and efficiency (E). The distribution of these graph metrics for each participant is then characterised with four statistical moments: mean, variance, skewness, and kurtosis. The results show significant differences between groups in the mean of K and E, and the kurtosis of Cloc and K. Although not significant when considered alone, the skewness of Cloc is the most frequently selected feature for the discrimination of subject groups. These results suggest that the variability of EEG functional connectivity may convey useful information about AD.
Sample Entropy (SampEn) is a nonlinear regularity index that requires the a priori selection of three parameters: the length of the sequences to be compared, m, the patterns similarity tolerance, r, and the number of samples under analysis, N. Appropriate values for m, r and N have been recommended in some cases, such as heart rate, hormonal data, etc., but no guidelines exist for the selection of that values. Hence, an optimal parameters study should be required for the application of SampEn to not previously analyzed biomedical signals. In this work, a thorough analysis on the optimal SampEn parameter values within two different scenarios of AF organization estimation, such as the prediction of paroxysmal AF termination and the electrical cardioversion outcome in persistent AF, is presented. Results indicated that, (i) the proportion between N and the sampling rate (ƒ(s)) should be higher than one second and ƒ(s) ≥ 256 Hz, (ii) overlapping between adjacent N-length windows does not improve organization estimation and (iii) values of m and r maximizing classification should be considered within a range wider than the proposed in the literature for heart rate analysis, i. e. m = 1 and m = 2 and r between 0.1 and 0.25 times the standard deviation of the data.
Characterization of the complexity of electroencephalogram (EEG) responses has provided important insights in cognitive function as well as in the brain bases of consciousness and vigilance. Whether brain response complexity changes during prolonged wakefulness and sleep deprivation –when vigilance level considerably varies– is not fully elucidated yet. In the present study, we repeatedly assessed EEG responses to transcranial magnetic stimulation (TMS) over 34 h of sleep deprivation under constant routine conditions in healthy younger (N = 13; 5 women; 18–30 y) and older (N = 12; 6 women; 50–70 y) individuals, while they were performing a vigilance task. Response complexity was computed both at the global (all scalp sensors) and local (sensors surrounding TMS hotspot) levels using the Lempel-Ziv algorithm. Response complexity was significantly higher in the older compared to the young volunteers over the entire protocol. Global complexity response significantly changed with time spent awake, with an increasing trend from the beginning to the middle of the biological night, followed by a decreasing trend from the middle of the biological night to the following afternoon. An unexpected different link between vigilance performance and brain response complexity was detected across age groups: higher response complexity was associated with lower performance in the older group, particularly in the morning sessions. These findings show that cortical activity complexity changes with vigilance variation, as experienced during sleep deprivation and circadian misalignment, in two age groups, with no evident time course difference across age-groups. Aside from classical linear EEG analyses, computation of Lempel-Ziv complexity provides additional insights on the neurophysiology of the processes associated with vigilance and their modifications throughout ageing.
This pilot study applied three distance-based bivariate Lempel-Ziv complexity (LZC) measures to investigate the changes in electroencephalogram (EEG) signals between 11 patients with Alzheimer's disease (AD) and 11 age matched controls. These methods measure richness of complexity between pairs of signals. Complexity of control subjects' EEGs was richer, i.e. signals were made from a greater number and greater range of subsequences, than those from AD patients in almost all cases in two non-normalized distance- based methods. Only some pairs including electrode T4 (2.1% of the total) occasionally showed the reverse result. Statistically significant differences were found with these two methods in 21 and 18 of 120 tested electrode pairs, respectively (Student's t test, p
Epileptic seizures are generated by an abnormal synchronization of neurons unforeseeable for the patients. In this study we analyzed invasive electroencephalogram (EEG) recordings in patients suffering from medically intractable focal epilepsy with two non-linear methods, Approximate Entropy (ApEn) and Lempel-Ziv (LZ) complexity. ApEn and LZ complexity quantify the regularity and complexity of a time series, respectively, and are well suited to the analysis of non-stationary biomedical signals of short length. Our results show an increase in ApEn and LZ complexity values during seizures at the focal electrodes. These changes could also be seen at some extra focal electrodes. After the seizure ends, the values of both non-linear metrics return to values lower than those before the seizure. Moreover, we quantified the changes in LZ complexity, showing the complexity increase during the seizure and its notable decrease after its end. Our results suggest that these techniques are useful to detect changes due to epileptic seizures in the EEG.
Alzheimer's disease (AD) is the main cause of dementia in western countries. Although a definite diagnosis of this illness is only possible by necropsy, the analysis of nonlinear dynamics in electroencephalogram (EEG) signals could help physicians in this difficult task In this study we have applied Approximate Entropy (ApEn) to analyze the EEG background activity of patients with a clinical diagnosis of Alzheimer's disease and control subjects. ApEn is a newly introduced statistic that can be used to quantify the complexity (or irregularity) of a time series. We have divided the EEG data into frames to calculate their ApEn. Our results show that the degree of complexity of EEGs from control subjects is higher. Applying the ANOVA test, we have verified that there was a significant difference (p < 0.05) between the EEGs of these groups.
Early detection of cardiac autonomic neuropathy (CAN) in patients with diabetes mellitus (DM) is of prime importance, as it will facilitate the prevention of its serious consequences. In the present work, the non-linear dynamics of electrocardiogram (ECG) recordings in 41 Type 2 DM patients with early CAN and 40 controls without clinical signs and symptoms of CAN were analysed with different implementations of Lempel-Ziv (LZ) complexity. LZ complexity is a non-linear analysis method that estimates the complexity of time series of finite length and reflects the arising rate of new patterns along the sequence. Results suggest that ECG traces are less complex in patients with early CAN than in those with no CAN. Differences were statistically significant (p < 0.05, Kruskal-Wallis test) when the LZ complexity was implemented with a three symbol conversion and the mean used to define the thresholds. Furthermore, the discriminative abilities of the different LZ complexity implementations in the context of CAN were evaluated with ROC curves. Accuracies over 65% were obtained when the mean was used to define the thresholds, with a sensitivity of 75.61% with a two symbol conversion. Our results suggest that LZ complexity might be a useful tool for an early detection of CAN from ECG recordings. Nevertheless, further studies are needed to address the possible usefulness of this methodology in the characterisation and early detection of CAN in type 2 DM patients.
Approximate entropy (ApEn) is a family of statistics introduced as a quantification of regularity in time series without any a priori knowledge about the system generating them. The aim of this preliminary study was to assess whether a time series analysis of arterial oxygen saturation (SaO2) signals from overnight pulse oximetry by means of ApEn could yield essential information on the diagnosis of obstructive sleep apnea (OSA) syndrome. We analyzed SaO2 signals from 187 subjects: 111 with a positive diagnosis of OSA and 76 with a negative diagnosis of OSA. We divided our data in a training set (44 patients with OSA Positive and 30 patients with OSA Negative) and a test set (67 patients with OSA Positive and 46 patients with OSA Negative). The training set was used for algorithm development and optimum threshold selection. Results showed that recurrence of apnea events in patients with OSA determined a significant increase in ApEn values. This method was assessed prospectively using the test dataset, where we obtained 82.09% sensitivity and 86.96% specificity. We conclude that ApEn analysis of SaO2 from pulse oximetric recording could be useful in the study of OSA.
The Lempel-Ziv (LZ) complexity is a non-linear time series analysis metric that reflects the arising rate of new patterns along with the sequence. Thus, it captures its temporal sequence and, quite conveniently, it can be computed with short data segments. In the present work, a detailed analysis on LZ complexity is presented within the context of atrial fibrillation (AF) organization estimation. As the analysed time series depend on the original sampling rate (fs), we evaluated the relationship between LZ complexity and fs. Furthermore, different implementations of LZ complexity were tested. Our results show the usefulness of LZ complexity to estimate AF organization and suggest that the signals from a terminating paroxysmal AF group are more organized (i.e. less complex) than those from the non-terminating paroxysmal AF group. However, the diagnostic accuracy was not as high as that obtained with sample entropy (SampEn), another non-linear metric, with the same database in a previous study (92% vs. 96%). Nevertheless, the LZ complexity analysis of AF organization with sampling frequencies higher than 2048 Hz, or even its combination with SampEn or other non-linear metrics, might improve the prediction of spontaneous AF termination.
Objective: This study aimed to produce a novel Deep Learning (DL) model for the classification of subjects with Alzheimer's Disease (AD), Mild Cognitive Impairment (MCI) subjects and Healthy Ageing (HA) subjects using resting-state scalp EEG signals. Approach: The raw EEG data were pre-processed to remove unwanted artefacts and sources of noise. The data were then processed with the Continuous Wavelet Transform (CWT), using the Morse mother wavelet, to create time-frequency graphs with a wavelet coefficient scale range of 0 to 600. The graphs were combined into tiled topographical maps governed by the 10-20 system orientation for scalp electrodes. The application of this processing pipeline was used on a data set of resting-state EEG samples from age-matched groups of 52 AD subjects (82.3 ± 4.7 years of age), 37 MCI subjects (78.4 ± 5.1 years of age) and 52 HA subjects (79.6 ± 6.0 years of age). This resulted in the formation of a data set of 16,197 topographical images. This image data set was then split into training, validation and test images and used as input to an AlexNet DL model. This model was comprised of 5 hidden convolutional layers and optimised for various parameters such as learning rate, learning rate schedule, optimiser, and batch size. Main Results: The performance was assessed by a 10-fold cross-validation strategy, which produced an average accuracy result of 98.9% ± 0.4% for the three-class classification of AD vs. MCI vs. HA. The results showed minimal overfitting and bias between classes, further indicating the strength of the model produced. Significance: These results provide significant improvement for this classification task compared to previous studies in this field and suggest that DL could contribute to the diagnosis of AD from EEG recordings.
The work outlined in this paper aimed to evaluate the practicality of the Surrey Virtual Rehabilitation System (SVRS) during simple hip and knee flexion-extension exercises. Two clinical engineering trainees, a physiotherapist and two children with CP performed two tests using the SVRS whilst parents and a paediatric physiotherapist observed the tests. Feedback collected using both questionnaires and an open-discussion suggests that the SVRS is practical for rehabilitation purposes during both physical exercises. © 2013 IEEE.
Paroxysmal atrial fibrillation (PAF) is the most common cardiac arrhythmia, conveying a stroke risk comparable to persistent AF. It poses a significant diagnostic challenge given its intermittency and potential brevity, and absence of symptoms in most patients. This pilot study introduces a novel biomarker for early PAF detection, based upon analysis of sinus rhythm ECG waveform complexity. Sinus rhythm ECG recordings were made from 52 patients with ( n = 28) or without ( n = 24) a subsequent diagnosis of PAF. Subjects used a handheld ECG monitor to record 28-second periods, twice-daily for at least 3 weeks. Two independent ECG complexity indices were calculated using a Lempel-Ziv algorithm: R-wave interval variability (beat detection, BD) and complexity of the entire ECG waveform (threshold crossing, TC). TC, but not BD, complexity scores were significantly greater in PAF patients, but TC complexity alone did not identify satisfactorily individual PAF cases. However, a composite complexity score ( h -score) based on within-patient BD and TC variability scores was devised. The h -score allowed correct identification of PAF patients with 85% sensitivity and 83% specificity. This powerful but simple approach to identify PAF sufferers from analysis of brief periods of sinus-rhythm ECGs using hand-held monitors should enable easy and low-cost screening for PAF with the potential to reduce stroke occurrence.
Alzheimer’s disease (AD) is a degenerative brain disorder leading to memory loss and changes in other cognitive abilities. The complexity of electroencephalogram (EEG) signals may help to characterise AD. To this end, we propose an extension of multiscale entropy based on variance (MSEσ2) to multichannel signals, termed multivariate MSEσ2 (mvMSEσ2), to take into account both the spatial and time domains of time series. Then, we investigate the mvMSEσ2 of EEGs at different frequency bands, including the broadband signals filtered between 1 and 40Hz, θ, α, and β bands, and compare it with the previously proposed multiscale entropy based on mean (MSEµ), multivariate MSEµ (mvMSEµ), and MSEσ2, to distinguish different kinds of dynamical properties of spread and mean in the signals. Results from 11 AD patients and 11 age-matched controls suggest that the presence of broadband activity of EEGs is required for a proper evaluation of complexity. MSEσ2 and mvMSEσ2 results, showing a loss of complexity in AD signals, led to smaller p-values in comparison with MSEµ and mvMSEµ ones, suggesting that the variance-based MSE and mvMSE can characterise changes in EEGs as a result of AD in a more detailed way. The p-values for the slope values of mvMSE curves were smaller than for MSE at large scale factors, also showing the possible usefulness of multivariate techniques.
OBJECTIVE: To determine whether decomplexification of intracranial pressure dynamics occurs during periods of severe intracranial hypertension (intracranial pressure >25 mm Hg for >5 mins in the absence of external noxious stimuli) in pediatric patients with intracranial hypertension. DESIGN: Retrospective analysis of clinical case series over a 30-month period from April 2000 through January 2003. SETTING: Multidisciplinary 16-bed pediatric intensive care unit. PATIENTS: Eleven episodes of intracranial hypertension from seven patients requiring ventriculostomy catheter for intracranial pressure monitoring and/or cerebral spinal fluid drainage. INTERVENTIONS: None. MEASUREMENTS AND MAIN RESULTS: We measured changes in the intracranial pressure complexity, estimated by the approximate entropy (ApEn), as patients progressed from a state of normal intracranial pressure (
The aim of the present study was to analyse the magnetoencephalogram (MEG) background activity in patients with Alzheimer's disease (AD) using the Lempel-Ziv (LZ) complexity. This non-linear method measures the complexity of finite sequences and is related to the number of distinct substrings and the rate of their occurrence along the sequence. The MEGs were recorded with a 148-channel whole-head magnetometer (MAGNES 2500 WH, 4D Neuroimaging) in 21 patients with AD and in 21 age-matched control subjects. Artefact-free epochs were selected for complexity analysis. Results showed that MEG signals from AD patients had lower complexity than control subjects' MEGs and the differences were statistically significant (p
Objective To analyse magnetoencephalogram (MEG) signals with Lempel-Ziv Complexity (LZC) to identify the regions of the brain showing changes related to cognitive decline and Alzheimeŕs Disease (AD). Methods LZC was used to study MEG signals in the source space from 99 participants (36 male, 63 female, average age: 71.82 ± 4.06) in three groups (33 subjects per group): healthy (control) older adults, older adults with subjective cognitive decline (SCD), and adults with mild cognitive impairment (MCI). Analyses were performed in broadband (2–45 Hz) and in classic narrow bands (theta (4–8 Hz), alpha (8–12 Hz), low beta (12–20 Hz), high beta (20–30 Hz), and, gamma (30–45 Hz)). Results LZC was significantly lower in subjects with MCI than in those with SCD. Moreover, subjects with MCI had significantly lower MEG complexity than controls and SCD subjects in the beta frequency band. Lower complexity was correlated with smaller hippocampal volumes. Conclusions Brain complexity – measured with LZC – decreases in MCI patients when compared to SCD and healthy controls. This decrease is associated with a decrease in hippocampal volume, a key feature in AD progression. Significance This is the first study to date characterising the changes of brain activity complexity showing the specific spatial pattern of the alterations as well as the morphological correlations throughout preclinical stages of AD.
Currently accepted input parameter limitations in entropy-based, non-linear signal processing methods, for example, sample entropy (SampEn), may limit the information gathered from tested biological signals. The ability of quadratic sample entropy (QSE) to identify changes in electroencephalogram (EEG) signals of 11 patients with a diagnosis of Alzheimer's disease (AD) and 11 age-matched, healthy controls is investigated. QSE measures signal regularity, where reduced QSE values indicate greater regularity. The presented method allows a greater range of QSE input parameters to produce reliable results than SampEn. QSE was lower in AD patients compared with controls with significant differences (p < 0.01) for different parameter combinations at electrodes P3, P4, O1 and O2. Subject- and epoch-based classifications were tested with leave-one-out linear discriminant analysis. The maximum diagnostic accuracy and area under the receiver operating characteristic curve were 77.27 and more than 80%, respectively, at many parameter and electrode combinations. Furthermore, QSE results across all r values were consistent, suggesting QSE is robust for a wider range of input parameters than SampEn. The best results were obtained with input parameters outside the acceptable range for SampEn, and can identify EEG changes between AD patients and controls. However, caution should be applied because of the small sample size.
Alzheimer’s disease (AD) is the most prevalent form of dementia in the world, which is characterised by the loss of neurones and the build-up of plaques in the brain, causing progressive symptoms of memory loss and confusion. Although definite diagnosis is only possible by necropsy, differential diagnosis with other types of dementia is still needed. An electroencephalogram (EEG) is a cheap, portable, non-invasive method to record brain signals. Previous studies with non-linear signal processing methods have shown changes in the EEG due to AD, which is characterised reduced complexity and increased regularity. EEGs from 11 AD patients and 11 age-matched control subjects were analysed with Fuzzy Entropy (FuzzyEn), a non-linear method that was introduced as an improvement over the frequently used Approximate Entropy (ApEn) and Sample Entropy (SampEn) algorithms. AD patients had significantly lower FuzzyEn values than control subjects (p < 0.01) at electrodes T6, P3, P4, O1, and O2. Furthermore, when diagnostic accuracy was calculated using Receiver Operating Characteristic (ROC) curves, FuzzyEn outperformed both ApEn and SampEn, reaching a maximum accuracy of 86.36%. These results suggest that FuzzyEn could increase the insight into brain dysfunction in AD, providing potentially useful diagnostic information. However, results depend heavily on the input parameters that are used to compute FuzzyEn.
This paper presents the game Myo-Pong, a simple graphical table-tennis game included in the portable UVa Neuromuscular Training System (UVa-NTS). Myo-Pong demonstrates the capabilities of the UVa-NTS as a myoelectric real-time system for training and for playing by means of myoelectric control.
The aim of the present research is to study the magnetoencephalogram (MEG) background activity in patients with Alzheimer's disease (AD) using the Lempel-Ziv (LZ) complexity. We recorded the MEG with a 148-channel whole-head magnetometer (MAGNES 2500 WH, 4D Neuroimaging) in 10 patients with probable AD and 10 age-matched control subjects, during five minutes. Artefact-free epochs were selected for the non-linear analysis. In all MEG channels, the AD patients had lower complexity than control subjects. In 77 of them the differences were statistically significant (p < 0.01). These preliminary results suggest that cognitive dysfunction in AD is associated with a decreased complexity in certain regions of the brain.
PURPOSE: Nonlinear dynamics has enhanced the diagnostic abilities of some physiological signals. Recent studies have shown that the complexity of the intracranial pressure waveform decreases during periods of intracranial hypertension in paediatric patients with acute brain injury. We wanted to assess changes in the complexity of the cerebrospinal fluid (CSF) pressure signal over the large range covered during the study of CSF circulation with infusion studies. METHODS: We performed 37 infusion studies in patients with hydrocephalus of various types and origin (median age 71 years; interquartile range 60-77 years). After 5 min of baseline measurement, infusion was started at a rate of 1.5 ml/min until a plateau was reached. Once the infusion finished, CSF pressure was recorded until it returned to baseline. We analysed CSF pressure signals using the Lempel-Ziv (LZ) complexity measure. To characterise more accurately the behaviour of LZ complexity, the study was segmented into four periods: basal, early infusion, plateau and recovery. RESULTS: The LZ complexity of the CSF pressure decreased in the plateau of the infusion study compared to the basal complexity (p=0.0018). This indicates loss of complexity of the CSF pulse waveform with intracranial hypertension. We also noted that the level of complexity begins to increase when the infusion is interrupted and CSF pressure drops towards the initial values. CONCLUSIONS: The LZ complexity decreases when CSF pressure reaches the range of intracranial hypertension during infusion studies. This finding provides further evidence of a phenomenon of decomplexification in the pulsatile component of the pressure signal during intracranial hypertension.
This study focuses on the resting state network analysis of the brain, as well as how these networks change both in topology and location throughout life. The magnetoencephalogram (MEG) background activity from 220 healthy volunteers (age 7-84 years), was analysed combining complex network analysis principles of graph theory with both linear and non-linear methods to evaluate the changes in the brain. Granger Causality (GC) (linear method) and Phase Slope Index (PSI) (non-linear method) were used to observe the connectivity in the brain during rest, and as a function of age by analysing the degree, clustering coefficient, efficiency, betweenness, modularity and maximised modularity of the observed complex brain networks. Our results showed that GC showed little linear causal activity in the brain at rest, with small world topology, while PSI showed little information flow in the brain, with random network topology. However, both analyses produced complementary results pertaining to the resting state of the brain.
. This pilot study applied Lempel-Ziv Complexity (LZC) to 22 resting EEG signals, collected using the 10-20 international system, from 11 patients with Alzheimer’s disease (AD) and 11 age-matched controls. This allowed for frequency band analysis as the EEG signals were first prefiltered with a third order Hamming window in the ranges F to F+WHz with both F and W equal to 1-30Hz respectively. Control subjects were found to have a greater signal complexity than AD patients with statistically significant bands seen at various ranges in all 16 electrodes. The maximum statistical significance (Student’s t test, p
Symbolic dynamic analysis (SDA) methods have been applied to biomedical signals and have been proven efficient in characterising differences in the electroencephalogram (EEG) in various conditions (e.g., epilepsy, Alzheimer’s, and Parkinson’s diseases). In this study, we investigated the use of SDA on EEGs recorded during sleep. Lempel-Ziv complexity (LZC), permutation entropy (PE), and permutation Lempel-Ziv complexity (PLZC), as well as power spectral analysis based on the fast Fourier transform (FFT), were applied to 8-h sleep EEG recordings in healthy men (n=31) and women (n=29), aged 20-74 years. The results of the SDA methods and FFT analysis were compared and the effects of age and sex were investigated. Surrogate data were used to determine whether the findings with SDA methods truly reflected changes in nonlinear dynamics of the EEG and not merely changes in the power spectrum. The surrogate data analysis showed that LZC merely reflected spectral changes in EEG activity, whereas PE and PLZC reflected genuine changes in the nonlinear dynamics of the EEG. All three SDA techniques distinguished the vigilance states (i.e., wakefulness, REM sleep, NREM sleep, and its sub-stages: stage 1, stage 2, and slow wave sleep). Complexity of the sleep EEG increased with ageing. Sex on the other hand did not affect the complexity values assessed with any of these three SDA methods, even though FFT detected sex differences. This study shows that SDA provides additional insights into the dynamics of sleep EEG and how it is affected by ageing.
The magnetoencephalogram (MEG) is contaminated with undesired signals, which are called artifacts. Some of the most important ones are the cardiac and the ocular artifacts (CA and OA, respectively), and the power line noise (PLN). Blind source separation (BSS) has been used to reduce the influence of the artifacts in the data. There is a plethora of BSS-based artifact removal approaches, but few comparative analyses. In this study, MEG background activity from 26 subjects was processed with five widespread BSS (AMUSE, SOBI, JADE, extended Infomax, and FastICA) and one constrained BSS (cBSS) techniques. Then, the ability of several combinations of BSS algorithm, epoch length, and artifact detection metric to automatically reduce the CA, OA, and PLN were quantified with objective criteria. The results pinpointed to cBSS as a very suitable approach to remove the CA. Additionally, a combination of AMUSE or SOBI and artifact detection metrics based on entropy or power criteria decreased the OA. Finally, the PLN was reduced by means of a spectral metric. These findings confirm the utility of BSS to help in the artifact removal for MEG background activity.
Alzheimer’s disease (AD) is a neurodegenerative disease caused by the progressive death of brain cells over time. It represents the most frequent cause of dementia in the western world, and affects an individual’s cognitive ability and psychological capacity. While clinical diagnoses of AD are made primarily on the basis of clinical evaluation and mental health tests, diagnostic certainty is only possible through necropsy. One non-invasive approach to investigating AD is to use electroencephalograms (EEGs), which reflect brain electrical activity and so can be used to detect electrical abnormalities in brain signals with non-invasive cranial surface electrodes. Generally EEGs in AD patients show a shift to lower frequencies in spectral analysis and display less complexity and contain more regular patterns compared to those of control subjects. Here we present a method for differentiating AD patients from healthy ones based on their EEG signals using Benford’s law and support vector machines (SVMs) with a radial basis function (RBF) kernel. EEG signals from eleven AD and eleven age-matched controls were divided into artefact-free 5-sec epochs and used to train an SVM. 10 fold cross validation was performed at both the epochand subject-level to evaluate the importance of each electrode in discriminating between AD and healthy subjects. Substantive variability was seen across the different electrodes, with electrodes O1, O2 and C4 particularly being important. Performance across the electrodes was reduced when subject-level cross validation was performed, but relative performance across the electrodes was consistent with that found using epoch-level cross validation.
The aim of this study was to assess whether independent component analysis (ICA) could be valuable to remove power line noise, cardiac, and ocular artifacts from magnetoencephalogram (MEG) background activity. The MEGs were recorded from 11 subjects with a 148-channel whole-head magnetometer. We used a statistical criterion to estimate the number of independent components. Then, a robust ICA algorithm decomposed the MEG epochs and several methods were applied to detect those artifacts. The whole process had been previously tested on synthetic data. We found that the line noise components could be easily detected by their frequency spectrum. In addition, the ocular artifacts could be identified by their frequency characteristics and scalp topography. Moreover, the cardiac artifact was better recognized by its skewness value than by its kurtosis one. Finally, the MEG signals were compared before and after artifact rejection to evaluate our method.
Specific patterns of brain activity during sleep and waking are recorded in the electroencephalogram (EEG). Time-frequency analyses methods have been widely used to analyse the EEG and identified characteristic oscillations for each vigilance state (VS) i.e., wakefulness, rapid-eye movement (REM) and non-rapid-eye movement (NREM) sleep. However, other aspects such as change of patterns associated with brain dynamics may not be captured unless a non-linear-based analysis method is used. In this pilot study, Permutation Lempel-Ziv Complexity (PLZC), a novel symbolic dynamics analysis method, was used to characterise the changes in the EEG in sleep and wakefulness during baseline and recovery from sleep deprivation. The results obtained with PLZC were contrasted with a related non-linear method, Lempel-Ziv complexity (LZC). Both measure the emergence of new patterns. However, LZC is dependent on the absolute amplitude of the EEG, while PLZC is only dependent on the relative amplitude due to symbolisation procedure and thus, more resistant to noise. We showed that PLZC discriminates activated brain states associated with wakefulness and REM sleep, which both displayed higher complexity, compared to NREM sleep. Additionally, significantly lower PLZC values were measured in NREM sleep during the recovery period following SD compared to baseline, suggesting a reduced emergence of new activity patterns in the EEG. These findings were validated using PLZC on surrogate data. By contrast, LZC was merely reflecting changes in the spectral composition of the EEG. Overall, this study implies that PLZC is a robust non-linear complexity measure, which is not dependent on amplitude variations in the signal, and which may be useful to further assess EEG alterations induced by environmental or pharmacological manipulations.
Research into binary network analysis of brain function faces a methodological challenge in selecting an appropriate threshold to binarise edge weights. For EEG phase-based functional connectivity, we test the hypothesis that such binarisation should take into account the complex hierarchical structure found in functional connectivity. We explore the density range suitable for such structure and provide a comparison of state-of-the-art binarisation techniques, the recently proposed Cluster-Span Threshold (CST), minimum spanning trees, efficiency-cost optimisation and union of shortest path graphs, with arbitrary proportional thresholds and weighted networks. We test these techniques on weighted complex hierarchy models by contrasting model realisations with small parametric differences. We also test the robustness of these techniques to random and targeted topological attacks.We find that the CST performs consistenty well in state-of-the-art modelling of EEG network topology, robustness to topological PLOS 1/31 network attacks, and in three real datasets, agreeing with our hypothesis of hierarchical complexity. This provides interesting new evidence into the relevance of considering a large number of edges in EEG functional connectivity research to provide informational density in the topology.
—Alzheimer's Disease (AD) is the most common form of dementia. Mild Cognitive Impairment (MCI) is the term given to the stage describing prodromal AD and represents a 'risk factor' in early-stage AD diagnosis from normal cognitive decline due to ageing. The electroencephalogram (EEG) has been studied extensively for AD characterization, but reliable early-stage diagnosis continues to present a challenge. The aim of this study was to introduce a novel way of classifying between AD patients, MCI subjects, and age-matched healthy control (HC) subjects using EEG-derived feature images and deep learning techniques. The EEG recordings of 141 age-matched subjects (52 AD, 37 MCI, 52 HC) were converted into 2D greyscale images representing the Pearson correlation coefficients and the distance Lempel-Ziv Complexity (dLZC) between the 21 EEG channels. Each feature type was computed from EEG epochs of 1s, 2s, 5s and 10s segmented from the original recording. The CNN architecture AlexNet was modified and employed for this three-way classification task and a 70/30 split was used for training and validation with each of the different epoch lengths and EEG-derived images. Whilst a maximum classification accuracy of 73.49% was obtained using dLZC-derived images from 10s epochs as input to the model, the classification accuracy reached 98.13% using the images obtained from Pearson correlation coefficients and 5s epochs. Clinical Relevance— The preliminary findings from this study show that deep learning applied to the analysis of the EEG can classify subjects with accuracies close to 100%.
Brain clocks, which quantify discrepancies between brain age and chronological age, hold promise for understanding brain health and disease. However, the impact of diversity (including geographical, socioeconomic, sociodemographic, sex and neurodegeneration) on the brain-age gap is unknown. We analyzed datasets from 5,306 participants across 15 countries (7 Latin American and Caribbean countries (LAC) and 8 non-LAC countries). Based on higher-order interactions, we developed a brain-age gap deep learning architecture for functional magnetic resonance imaging (2,953) and electroencephalography (2,353). The datasets comprised healthy controls and individuals with mild cognitive impairment, Alzheimer disease and behavioral variant frontotemporal dementia. LAC models evidenced older brain ages (functional magnetic resonance imaging: mean directional error = 5.60, root mean square error (r.m.s.e.) = 11.91; electroencephalography: mean directional error = 5.34, r.m.s.e. = 9.82) associated with frontoposterior networks compared with non-LAC models. Structural socioeconomic inequality, pollution and health disparities were influential predictors of increased brain-age gaps, especially in LAC (R² = 0.37, F² = 0.59, r.m.s.e. = 6.9). An ascending brain-age gap from healthy controls to mild cognitive impairment to Alzheimer disease was found. In LAC, we observed larger brain-age gaps in females in control and Alzheimer disease groups compared with the respective males. The results were not explained by variations in signal quality, demographics or acquisition methods. These findings provide a quantitative framework capturing the diversity of accelerated brain aging.Brain clocks, which quantify discrepancies between brain age and chronological age, hold promise for understanding brain health and disease. However, the impact of diversity (including geographical, socioeconomic, sociodemographic, sex and neurodegeneration) on the brain-age gap is unknown. We analyzed datasets from 5,306 participants across 15 countries (7 Latin American and Caribbean countries (LAC) and 8 non-LAC countries). Based on higher-order interactions, we developed a brain-age gap deep learning architecture for functional magnetic resonance imaging (2,953) and electroencephalography (2,353). The datasets comprised healthy controls and individuals with mild cognitive impairment, Alzheimer disease and behavioral variant frontotemporal dementia. LAC models evidenced older brain ages (functional magnetic resonance imaging: mean directional error = 5.60, root mean square error (r.m.s.e.) = 11.91; electroencephalography: mean directional error = 5.34, r.m.s.e. = 9.82) associated with frontoposterior networks compared with non-LAC models. Structural socioeconomic inequality, pollution and health disparities were influential predictors of increased brain-age gaps, especially in LAC (R² = 0.37, F² = 0.59, r.m.s.e. = 6.9). An ascending brain-age gap from healthy controls to mild cognitive impairment to Alzheimer disease was found. In LAC, we observed larger brain-age gaps in females in control and Alzheimer disease groups compared with the respective males. The results were not explained by variations in signal quality, demographics or acquisition methods. These findings provide a quantitative framework capturing the diversity of accelerated brain aging.
Abstract - Psychogenic non-epileptic seizures (PNES) are attacks that resemble epilepsy but are not associated with epileptic brain activity and are regularly misdiagnosed. The current gold standard method of diagnosis is expensive and complex. Electroencephalogram (EEG) analysis with machine learning could improve this. A k-nearest neighbours (kNN) and support vector machine (SVM) were used to classify EEG connectivity measures from 48 patients with PNES and 29 patients with epilepsy. The synchronisation method-correlation or coherence-and the binarisation threshold were defined through experimentation. Ten network parameters were extracted from the synchronisation matrix. The broad, delta, theta, alpha, beta, gamma, and combined 'all' frequency bands were compared along with three feature selection methods: the full feature set (no selection), light gradient boosting machine (LGBM) and k-Best. Coherence was the highest performing synchronisation method and 0.6 was the best coherence threshold. The highest balanced accuracy was 89.74%, produced by combining all six frequency bands and selecting features with LGBM, classified by the SVM. This method returned a comparatively high accuracy but at a high computation cost. Future research should focus on identifying specific frequency bands and network parameters to reduce this cost. Clinical relevance - This study found that EEG connectivity and machine learning methods can be used to differentiate PNES from epilepsy using interictal recordings to a high accuracy. Thus, this method could be an effective tool in assisting clinicians in PNES diagnosis without a video-EEG recording of a habitual seizure.