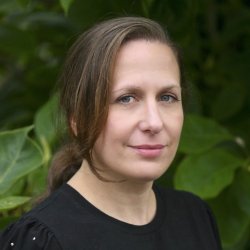
Professor Nophar Geifman
About
Biography
My interests lie in data sciences within healthcare and medicine; extending the use of artificial intelligence and big-data analytics to improve patient-centric predictions, treatment and outcomes, while also enhancing the open sharing of biomedical data. I have developed and employed a wide range of AI methods and applications, from text mining to machine learning, with the overarching goal of producing translational research with real-world impact.
My research centres on patient stratification, and biomarker discovery from large, diverse clinical and ‘omics’ datasets; applying informatics techniques, AI and machine learning for discovery in various areas of medicine, particularly where conventional research methods have over-simplified the inherent complexity of disease and care.
Publications
Background Juvenile idiopathic arthritis (JIA) is a heterogeneous disease, the signs and symptoms of which can be summarised with use of composite disease activity measures, including the clinical Juvenile Arthritis Disease Activity Score (cJADAS). However, clusters of children and young people might experience different global patterns in their signs and symptoms of disease, which might run in parallel or diverge over time. We aimed to identify such clusters in the 3 years after a diagnosis of JIA. The identification of these clusters would allow for a greater understanding of disease progression in JIA, including how physician-reported and patient-reported outcomes relate to each other over the JIA disease course. Methods In this multicentre prospective longitudinal study, we included children and young people recruited before Jan 1, 2015, to the Childhood Arthritis Prospective Study (CAPS), a UK multicentre inception cohort. Participants without a cJADAS score were excluded. To assess groups of children and young people with similar disease patterns in active joint count, physician's global assessment, and patient or parental global evaluation, we used latent profile analysis at initial presentation to paediatric rheumatology and multivariate group-based trajectory models for the following 3 years. Optimal models were selected on the basis of a combination of model fit, clinical plausibility, and model parsimony. Findings Between Jan 1, 2001, and Dec 31, 2014, 1423 children and young people with JIA were recruited to CAPS, 239 of whom were excluded, resulting in a final study population of 1184 children and young people. We identified five clusters at baseline and six trajectory groups using longitudinal follow-up data. Disease course was not well predicted from clusters at baseline; however, in both cross-sectional and longitudinal analyses, substantial proportions of children and young people had high patient or parent global scores despite low or improving joint counts and physician global scores. Participants in these groups were older, and a higher proportion of them had enthesitis-related JIA and lower socioeconomic status, compared with those in other groups. Interpretation Almost one in four children and young people with JIA in our study reported persistent, high patient or parent global scores despite having low or improving active joint counts and physician's global scores. Distinct patient subgroups defined by disease manifestation or trajectories of progression could help to better personalise health-care services and treatment plans for individuals with JIA. Copyright (C) 2020 The Author(s). Published by Elsevier Ltd. This is an Open Access article under the CC BY 4.0 license.
The global COVID-19 pandemic resulted in widespread harms but also rapid advances in vaccine development, diagnostic testing, and treatment. As the disease moves to endemic status, the need to identify characteristic biomarkers of the disease for diagnostics or therapeutics has lessened, but lessons can still be learned to inform biomarker research in dealing with future pathogens. In this work, we test five sets of research-derived biomarkers against an independent targeted and quantitative Liquid Chromatography-Mass Spectrometry metabolomics dataset to evaluate how robustly these proposed panels would distinguish between COVID-19-positive and negative patients in a hospital setting. We further evaluate a crowdsourced panel comprising the COVID-19 metabolomics biomarkers most commonly mentioned in the literature between 2020 and 2023. The best-performing panel in the independent dataset-measured by F1 score (0.76) and AUROC (0.77)-included nine biomarkers: lactic acid, glutamate, aspartate, phenylalanine, & beta;-alanine, ornithine, arachidonic acid, choline, and hypoxanthine. Panels comprising fewer metabolites performed less well, showing weaker statistical significance in the independent cohort than originally reported in their respective discovery studies. Whilst the studies reviewed here were small and may be subject to confounders, it is desirable that biomarker panels be resilient across cohorts if they are to find use in the clinic, highlighting the importance of assessing the robustness and reproducibility of metabolomics analyses in independent populations.
The UK Biobank is a cohort study that collects data on diet, lifestyle, biomarkers, and health to examine diet-disease associations. Based on the UK Biobank, we reviewed 36 studies on diet and three health conditions: type 2 diabetes (T2DM), cardiovascular disease (CVD), and cancer. Most studies used one-time dietary data instead of repeated 24 h recalls, which may lead to measurement errors and bias in estimating diet-disease associations. We also found that most studies focused on single food groups or macronutrients, while few studies adopted a dietary pattern approach. Several studies consistently showed that eating more red and processed meat led to a higher risk of lung and colorectal cancer. The results suggest that high adherence to "healthy" dietary patterns (consuming various food types, with at least three servings/day of whole grain, fruits, and vegetables, and meat and processed meat less than twice a week) slightly lowers the risk of T2DM, CVD, and colorectal cancer. Future research should use multi-omics data and machine learning models to account for the complexity and interactions of dietary components and their effects on disease risk.
There is an unmet need for improved diagnostic testing and risk prediction for cases of prostate cancer (PCa) to improve care and reduce overtreatment of indolent disease. Here we have analysed the serum proteome and lipidome of 262 study participants by liquid chromatography-mass spectrometry, including participants diagnosed with PCa, benign prostatic hyperplasia (BPH), or otherwise healthy volunteers, with the aim of improving biomarker specificity. Although a two class machine learning model separated PCa from controls with sensitivity of 0.82 and specificity of 0.95, adding BPH resulted in a statistically significant decline in specificity for prostate cancer to 0.76, with half of BPH cases being misclassified by the model as PCa. A small number of biomarkers differentiating between BPH and prostate cancer were identified, including proteins in MAP Kinase pathways, as well as in lipids containing oleic acid; these may offer a route to greater specificity. These results highlight, however, that whilst there are opportunities for machine learning, these will only be achieved by use of appropriate training sets that include confounding comorbidities, especially when calculating the specificity of a test.
Background: The anatomical continuity between the uterine cavity and the lower genital tract allows for the exploitation of uterine-derived biomaterial in cervico-vaginal fluid for endometrial cancer detection based on non-invasive sampling methodologies. Plasma is an attractive biofluid for cancer detection due to its simplicity and ease of collection. In this biomarker discovery study, we aimed to identify proteomic signatures that accurately discriminate endometrial cancer from controls in cervico-vaginal fluid and blood plasma. Methods: Blood plasma and Delphi Screener-collected cervico-vaginal fluid samples were acquired from symptomatic post-menopausal women with (n=53) and without (n=65) endometrial cancer. Digitised proteomic maps were derived for each sample using sequential window acquisition of all theoretical mass spectra (SWATH-MS). Machine learning was employed to identify the most discriminatory proteins. The best diagnostic model was determined based on accuracy and model parsimony. Findings: A protein signature derived from cervico-vaginal fluid more accurately discriminated cancer from control samples than one derived from plasma. A 5-biomarker panel of cervico-vaginal fluid derived proteins (HPT, LG3BP, FGA, LY6D and IGHM) predicted endometrial cancer with an AUC of 0.95 (0.91-0.98), sensitivity of 91% (83%-98%), and specificity of 86% (78%-95%). By contrast, a 3-marker panel of plasma proteins (APOD, PSMA7 and HPT) predicted endometrial cancer with an AUC of 0.87(0.81-0.93), sensitivity of 75% (64%-86%), and specificity of 84% (75%-93%). The parsimonious model AUC values for detection of stage I endometrial cancer in cervico-vaginal fluid and blood plasma were 0.92 (0.87-0.97) and 0.88 (0.82-0.95) respectively. Interpretation: Here, we leveraged the natural shed of endometrial tumours to potentially develop an innovative approach to endometrial cancer detection. We show proof of principle that endometrial cancers secrete unique protein signatures that can enable cancer detection via cervico-vaginal fluid assays. Confirmation in a larger independent cohort is warranted.
Byline: Taariq M Salie, Univ of Cape Town, Cape Town, South Africa; Jing Yang, Univ of Manchester, Manchester, United Kingdom; Carlos R Medina, Univ of Manchester, Manchester, United Kingdom; Nophar Geifman, Div of Informatics, Imaging & Data Sciences, Univ of Manchester, Manchester, United Kingdom; Liesl J Zuhlke, Paediatrics, Univ of Cape Town, Institute of Child Health, Red Cross Children's Hosp, Cape Town, South Africa; Simon Frain, Div of Cardiovascular Sciences, Univ of Manchester, Manchester, United Kingdom; Anthony Whetton, Univ of Manchester, Manchester, United Kingdom; Bernard Keavney, Univ of Manchester, Manchester; Mark E Engel, Univ of Cape Town, OBSERVATORY; Introduction: Rheumatic heart disease (RHD) remains a major source of morbidity and mortality in developing countries. A deeper insight into the pathogenetic mechanisms underlying RHD could provide opportunities for drug repurposing, guide recommendations for secondary penicillin prophylaxis, and/or inform development of near-patient diagnostics. Methods: We conducted a proteomic study in 215 African patients with severe RHD and 230 controls, using the SWATH-MS technique. We applied a machine learning (ML) approach to feature selection among the 366 proteins quantifiable in at least 40% of samples, using the Boruta wrapper algorithm. The case-control differences and contribution to AUC of the ROC for each of the 56 proteins identified by the Boruta algorithm were calculated by Logistic Regression adjusted for age, sex and BMI. Biological pathways and functions enriched for proteins were identified using ClueGo pathway analyses. Results: Adiponectin, complement component C7 and fibulin-1, a component of heart valve matrix, were each higher in cases when compared with controls. Ficolin-3, a protein with calcium-independent lectin activity that activates the complement pathway, was lower in cases than controls. The top 6 biomarkers from the Boruta analyses conferred an AUC of 0.90 indicating excellent discriminatory capacity between RHD cases and controls. Conclusions: These results support the presence of an ongoing inflammatory response in RHD, at a time when severe valve disease has developed, and distant from previous episodes of acute rheumatic fever. This biomarker signature could have potential utility in recognizing different degrees of ongoing inflammation in RHD patients, which may in turn be related to prognostic severity.
Abstract Background/Aims The CLUSTER consortium aims to identify biomarkers and strata that improve personalised treatments for JIA/JIA-uveitis. By bringing together knowledge and data, CLUSTER can conduct novel analyses in this rare, heterogeneous disease. Data harmonisation across existing JIA cohorts facilitates new, larger datasets that would otherwise take years to collect; however, challenges exist as datasets are often collected autonomously. Here we present progress towards a large-scale, unique JIA data resource, bringing together treatment data from four real-world JIA treatment studies. Methods Four studies (CAPS, CHARMS, BCRD and BSPAR-ETN; the latter two being part of the UK JIA Biologics register) contributed data into CLUSTER. We created two clinical datasets of JIA patients starting first-line methotrexate (MTX) or tumour necrosis factor inhibitors (TNFi). Variables were selected based on a previously developed core dataset, accounting for different levels of granularity across studies. The same inclusion and exclusion criteria were agreed for both datasets, designed to allow for combined analysis of these. OpenPseudonymiser software encrypted NHS numbers - these were matched cross-study to identify duplicates and checked against known duplicate lists. Errors in NHS numbers and existing duplicate matches were identified and corrected. Each NHS number was assigned a CLUSTER ID, meaning one child has the same ID across all relevant studies such that children contributing similar data across multiple studies could be identified. Results A total of 7013 records (from 5435 individuals) were identified, of which 2882 (41%, corresponding to 1304 individuals) represented the same child across >1 study. 197 individuals had duplicate records within one study, 961 in two studies, 142 in three, and four children had duplicate records in all four studies. After removing 350 MTX and 605 TNFi duplicate entries, the final datasets contain 2899 and 2401 unique MTX and TNFi patients respectively; 1018 are in both datasets having received both treatments. Missingness across core outcome variables ranged from 10% (active joint count MTX timepoint 2) to 60% (physician VAS TNFi timepoint 2) and was not improved through combining datasets with duplicate entries. Specificity in some variables was lost to allow integration by combining data using least common denominators (e.g. ethnicity captured as Caucasian/Non-Caucasian, despite more specific categories available in some studies). Conclusion Combining data across studies has achieved dataset sizes rarely seen in JIA, which is invaluable to progressing research into personalised treatments and disease outcomes. However, losing specificity in some variables and missingness (a known challenge in observational data) and their impact on future analyses requires further consideration. Ongoing work includes identifying patients with both clinical and biological data that can be combined for more in-depth analyses. Both datasets are available for researchers to use via the CLUSTER Consortium Data Management Committee. Disclosure S. Lawson-Tovey: None. L.R. Wedderburn: Consultancies; L.W. reports consulting fees from Pfizer unrelated to this work. Grants/research support; CLUSTER consortium receives support from AbbVie, UCB, Pfizer, Sobi and GSK. N. Geifman: None. M. Barnes: None. K.L. Hyrich: Grants/research support; KLH reports grant income from BMS, UCB, and Pfizer. Other; KLH reports non-personal speaker's fees from Abbvie.
Abstract Background Composite disease scores in juvenile idiopathic arthritis (JIA), such as the clinical Juvenile Arthritis Disease Activity Score (cJADAS), include multiple disease manifestations, presented as a single score. These overall scores aid understanding of disease holistically in each child or young person (CYP), and have been suggested as outcomes for clinical trials and targets in treat to target clinical strategies. However, signs and symptoms of disease may not follow similar patterns following a JIA diagnosis. It is not currently known what the patterns of disease activity are in CYP with JIA and how these cluster over time. Methods CYP with JIA were selected if enrolled in the Childhood Arthritis Prospective Study (CAPS), a UK multicentre inception cohort, before January 2015. cJADAS10 components (active joint count 0-10, physician global, patient/parent global) were collected at diagnosis, six months, one year and then annually to three years. Multivariate group-based trajectory models modelled cJADAS10 component scores using censored-normal (physician and parent global) and zero-inflated Poisson (active joint count) distributions. Within linear, quadratic and cubic polynomials, one to ten trajectories were tested. The optimal models were selected using Bayesian Information Criteria, model parsimony and clinical plausibility. Results Of 1,183 CYP selected, the majority were female (65%) and of white ethnicity (90%) with oligoarticular JIA the most common JIA category (45%). The optimal model identified six multivariate patterns of disease. In four of these clusters, signs and symptoms of disease had similar patterns over time: Low-Remission (32%), Low-Low (20%), High-Low (16%) and High-Low-High (10%). However, in two groups, Low-Chronic (14%) and High Chronic (8%), manifestations of inflammation and wellbeing followed different trajectory severities and shapes over time. These groups demonstrated persistent poor wellbeing despite control of inflammatory signs. Conclusion Disease activity in CYP with JIA does not improve in a uniform manner following initial presentation to paediatric rheumatology. Six latent multivariate trajectories have been identified in young people with JIA, two of which persist with chronic poor wellbeing despite lowered inflammation. Conflicts of Interest The authors declare no conflicts of interest.
Objectives: To identify predictors of overall lupus and lupus nephritis (LN) responses in patients with LN. Methods: Data from the Aspreva Lupus Management Study (ALMS) trial cohort was used to identify baseline predictors of response at 6 months. Endpoints were major clinical response (MCR), improvement, complete renal response (CRR) and partial renal response (PRR). Univariate and multivariate logistic regressions with least absolute shrinkage and selection operator (LASSO) and cross-validation in randomly split samples were utilised. Predictors were ranked by the percentage of times selected by LASSO and prediction performance was assessed by the area under the receiver operating characteristics (AUROC) curve. Results: We studied 370 patients in the ALMS induction trial. Improvement at 6 months was associated with older age (OR=1.03 (95% CI: 1.01 to 1.05) per year), normal haemoglobin (1.85 (1.16 to 2.95) vs low haemoglobin), active lupus (British Isles Lupus Assessment Group A or B) in haematological and mucocutaneous domains (0.61 (0.39 to 0.97) and 0.50 (0.31 to 0.81)), baseline damage (SDI>1 vs =0) (0.38 (0.16 to 0.91)) and 24-hour urine protein (0.63 (0.50 to 0.80)). LN duration 2–4 years (0.43 (0.19 to 0.97) vs
A key trend in current medical research is a shift from a one-size-fit-all to precision treatment strategies, where the focus is on identifying narrow subgroups of the population that would benefit from a given intervention. Precision medicine will greatly benefit from accessible tools that clinicians can use to identify suchsuch subgroups, and to generate novel inferences about the patient population they are treating. We present a novel dashboard app that enables clinician users to explore patient subgroups with varying longitudinal treatment response, using latent class mixed modeling. The dashboard was developed in R Shiny. We present results of our approach applied to an observational study of patients with moderate to severe rheumatoid arthritis (RA) on first-line biologic treatment.
Temporal phenotyping enables clinicians to better under-stand observable characteristics of a disease as it progresses. Modelling disease progression that captures interactions between phenotypes is inherently challenging. Temporal models that capture change in disease over time can identify the key features that characterize disease subtypes that underpin these trajectories. These models will enable clinicians to identify early warning signs of progression in specific sub-types and therefore to make informed decisions tailored to individual patients. In this paper, we explore two approaches to building temporal phenotypes based on the topology of data: topological data analysis and pseudo time-series. Using type 2 diabetes data, we show that the topological data analysis approach is able to identify trajectories representing different temporal phenotypes and that pseudo time-series can infer a state space model characterized by transitions between hidden states that represent distinct temporal phenotypes. Both approaches highlight lipid profiles as key factors in distinguishing the phenotypes.
Sepsis remains a complex medical problem and a major challenge in healthcare. Diagnostics and outcome predictions are focused on physiological parameters with less consideration given to patients' medical background. Given the aging population, not only are diseases becoming increasingly prevalent but occur more frequently in combinations ("multimorbidity"). We hypothesized the existence of patient subgroups in critical care with distinct multimorbidity states. We further hypothesize that certain multimorbidity states associate with higher rates of organ failure, sepsis, and mortality co-occurring with these clinical problems. We analyzed 36,390 patients from the open source Medical Information Mart for Intensive Care III (MIMIC III) dataset. Morbidities were defined based on Elixhauser categories, a well-established scheme distinguishing 30 classes of chronic diseases. We used latent class analysis to identify distinct patient subgroups based on demographics, admission type, and morbidity compositions and compared the prevalence of organ dysfunction, sepsis, and inpatient mortality for each subgroup. We identified six clinically distinct multimorbidity subgroups labeled based on their dominant Elixhauser disease classes. The "cardiopulmonary" and "cardiac" subgroups consisted of older patients with a high prevalence of cardiopulmonary conditions and constituted 6.1% and 26.4% of study cohort respectively. The "young" subgroup included 23.5% of the cohort composed of young and healthy patients. The "hepatic/addiction" subgroup, constituting 9.8% of the cohort, consisted of middle-aged patients (mean age of 52.25, 95% CI 51.85-52.65) with the high rates of depression (20.1%), alcohol abuse (47.75%), drug abuse (18.2%), and liver failure (67%). The "complicated diabetics" and "uncomplicated diabetics" subgroups constituted 9.4% and 24.8% of the study cohort respectively. The complicated diabetics subgroup demonstrated higher rates of end-organ complications (88.3% prevalence of renal failure). Rates of organ dysfunction and sepsis ranged 19.6-69% and 12.5-46.7% respectively in the six subgroups. Mortality co-occurring with organ dysfunction and sepsis ranges was 8.4-23.8% and 11.7-27.4% respectively. These adverse outcomes were most prevalent in the hepatic/addiction subgroup. We identify distinct multimorbidity states that associate with relatively higher prevalence of organ dysfunction, sepsis, and co-occurring mortality. The findings promote the incorporation of multimorbidity in healthcare models and the shift away from the current single-disease paradigm in clinical practice, training, and trial design.
Rheumatic heart disease (RHD) remains a major source of morbidity and mortality in developing countries. A deeper insight into the pathogenetic mechanisms underlying RHD could provide opportunities for drug repurposing, guide recommendations for secondary penicillin prophylaxis, and/or inform development of near-patient diagnostics.We performed quantitative proteomics using Sequential Windowed Acquisition of All Theoretical Fragment Ion Mass Spectrometry (SWATH-MS) to screen protein expression in 215 African patients with severe RHD, and 230 controls. We applied a machine learning (ML) approach to feature selection among the 366 proteins quantifiable in at least 40% of samples, using the Boruta wrapper algorithm. The case-control differences and contribution to area under the Receiver Operating Curve for each of the 56 proteins identified by the Boruta algorithm were calculated by Logistic Regression adjusted for age, sex and BMI. Biological pathways and functions enriched for proteins were identified using ClueGo pathway analyses.Adiponectin, complement component C7 and fibulin-1, a component of heart valve matrix, were significantly higher in cases when compared with controls (Table 1). Ficolin-3, a protein with calcium-independent lectin activity that activates the complement pathway, was lower in cases than controls (Table 1). The top six biomarkers, including adiponectin, complement component C7, quiescin sulfhydryl oxidase 1, insulin like growth factor binding protein acid labile subunit, pregnancy zone protein and phosphatidylinositol-glycan-specific phospholipase D, from the Boruta analyses (Fig. 1a) conferred an AUC of 0.90 indicating excellent discriminatory capacity between RHD cases and controls (Fig. 1b).ClueGo pathway analysis results of these biomarkers support the presence of an ongoing inflammatory response in RHD (Fig. 2), at a time when severe valve disease has developed, and distant from previous episodes of acute rheumatic fever. This biomarker signature could have potential utility in recognizing different degrees of ongoing inflammation in RHD patients, which may, in turn, be related to prognostic severity.Conflict of InterestNone
The use of technologies that provide objective, digital data to clinicians, carers, and service users to improve care and outcomes comes under the unifying term Digital Health. This field, which includes the use of high-tech health devices, telemedicine and health analytics has, in recent years, seen significant growth in the United Kingdom and worldwide. It is clearly acknowledged by multiple stakeholders that digital health innovations are necessary for the future of improved and more economic healthcare service delivery. Here we consider digital health-related research and applications by using an informatics tool to objectively survey the field. We have used a quantitative text-mining technique, applied to published works in the field of digital health, to capture and analyse key approaches taken and the diseases areas where these have been applied. Key areas of research and application are shown to be cardiovascular, stroke, and hypertension; although the range seen is wide. We consider advances in digital health and telemedicine in light of the COVID-19 pandemic.
Introduction: Body mass index (BMI) is often elevated at type 2 diabetes (T2D) diagnosis. Using latent class trajectory modelling (LCTM) of BMI, we examined whether weight loss after diagnosis influenced cancer incidence and all-cause mortality. Methods: From 1995 to 2010, we identified 7,708 patients with T2D from the Salford Integrated Record database (UK) and linked to the cancer registry for information on obesity-related cancer (ORC), non-ORC; and all-cause mortality. Repeated BMIs were used to construct sex-specific latent class trajectories. Hazard ratios (HRs) and 95% confidence intervals (CIs) were estimated using Cox regression models. Results: Four sex-specific BMI classes were identified; stable-overweight, stable-obese, obese-slightly-decreasing, and obese-steeply-decreasing; comprising 41%, 45%, 13%, and 1% of women, and 45%, 37%, 17%, and 1% of men, respectively. In women, the stable-obese class had similar ORC risks as the obese-slightly-decreasing class, whereas the stable-overweight class had lower risks. In men, the obese-slightly-decreasing class had higher risks of ORC (HR = 1.86, 95% CI: 1.05–3.32) than the stable-obese class, while the stable-overweight class had similar risks No associations were observed for non-ORC. Compared to the stable-obese class, women (HR = 1.60, 95% CI: 0.99–2.58) and men (HR = 2.37, 95% CI: 1.66–3.39) in the obese-slightly-decreasing class had elevated mortality. No associations were observed for the stable-overweight classes. Conclusion: Patients who lost weight after T2D diagnosis had higher risks for ORC (in men) and higher all-cause mortality (both genders) than patients with stable obesity.
Background Halting progression of chronic kidney disease (CKD) to established end stage kidney disease is a major goal of global health research. The mechanism of CKD progression involves pro-inflammatory, pro-fibrotic, and vascular pathways, but pathophysiological differentiation is currently lacking. Methods Plasma samples of 414 non-dialysis CKD patients, 170 fast progressors (with ∂ eGFR-3 ml/min/1.73 m2/year or worse) and 244 stable patients (∂ eGFR of − 0.5 to + 1 ml/min/1.73 m2/year) with a broad range of kidney disease aetiologies, were obtained and interrogated for proteomic signals with SWATH-MS. We applied a machine learning approach to feature selection of proteins quantifiable in at least 20% of the samples, using the Boruta algorithm. Biological pathways enriched by these proteins were identified using ClueGo pathway analyses. Results The resulting digitised proteomic maps inclusive of 626 proteins were investigated in tandem with available clinical data to identify biomarkers of progression. The machine learning model using Boruta Feature Selection identified 25 biomarkers as being important to progression type classification (Area Under the Curve = 0.81, Accuracy = 0.72). Our functional enrichment analysis revealed associations with the complement cascade pathway, which is relevant to CKD as the kidney is particularly vulnerable to complement overactivation. This provides further evidence to target complement inhibition as a potential approach to modulating the progression of diabetic nephropathy. Proteins involved in the ubiquitin–proteasome pathway, a crucial protein degradation system, were also found to be significantly enriched. Conclusions The in-depth proteomic characterisation of this large-scale CKD cohort is a step toward generating mechanism-based hypotheses that might lend themselves to future drug targeting. Candidate biomarkers will be validated in samples from selected patients in other large non-dialysis CKD cohorts using a targeted mass spectrometric analysis.
Background Rheumatic heart disease (RHD) remains a major source of morbidity and mortality in developing countries. A deeper insight into the pathogenetic mechanisms underlying RHD could provide opportunities for drug repurposing, guide recommendations for secondary penicillin prophylaxis, and/or inform development of near-patient diagnostics. Methods We performed quantitative proteomics using Sequential Windowed Acquisition of All Theoretical Fragment Ion Mass Spectrometry (SWATH-MS) to screen protein expression in 215 African patients with severe RHD, and 230 controls. We applied a machine learning (ML) approach to feature selection among the 366 proteins quantifiable in at least 40% of samples, using the Boruta wrapper algorithm. The case–control differences and contribution to Area Under the Receiver Operating Curve (AUC) for each of the 56 proteins identified by the Boruta algorithm were calculated by Logistic Regression adjusted for age, sex and BMI. Biological pathways and functions enriched for proteins were identified using ClueGo pathway analyses. Results Adiponectin, complement component C7 and fibulin-1, a component of heart valve matrix, were significantly higher in cases when compared with controls. Ficolin-3, a protein with calcium-independent lectin activity that activates the complement pathway, was lower in cases than controls. The top six biomarkers from the Boruta analyses conferred an AUC of 0.90 indicating excellent discriminatory capacity between RHD cases and controls. Conclusions These results support the presence of an ongoing inflammatory response in RHD, at a time when severe valve disease has developed, and distant from previous episodes of acute rheumatic fever. This biomarker signature could have potential utility in recognizing different degrees of ongoing inflammation in RHD patients, which may, in turn, be related to prognostic severity.
Prostate cancer is the most common malignant tumour in men. Improved testing for di- agnosis, risk prediction, and response to treatment would improve care. Here, we identified a pro- teomic signature of prostate cancer in peripheral blood using data-independent acquisition mass spectrometry combined with machine learning. A highly predictive signature was derived, which was associated with relevant pathways, including the coagulation, complement, and clotting cas- cades, as well as plasma lipoprotein particle remodeling. We further validated the identified bi- omarkers against a second cohort, identifying a panel of five key markers (GP5, SERPINA5, ECM1, IGHG1, and THBS1) which retained most of the diagnostic power of the overall dataset, achieving an AUC of 0.91. Taken together, this study provides a proteomic signature complementary to PSA for the diagnosis of patients with localised prostate cancer, with the further potential for assessing risk of future development of prostate cancer. Data are available via ProteomeXchange with identi- fier PXD025484.
Simple Summary: Prostate cancer is the third most frequent cancer in men worldwide, with a notable increase in prevalence over the past two decades. The PSA is the only well-established protein biomarker for prostate cancer diagnosis, staging, and surveillance. It frequently leads to inaccurate diagnosis and overtreatment since it is an organ-specific biomarker rather than a tumour-specific biomarker. As a result, one of the primary goals of prostate cancer proteome research is to identify novel biomarkers that can be used with or instead of PSA, particularly in non-invasive blood samples. Thousands of peptides or assays were detected in blood samples from patients with low- to high-grade prostate cancer and healthy individuals, allowing data processing of sequential window acquisition of all theoretical mass spectra (SWATH-MS). By assisting in the detection of prostate cancer biomarkers in blood samples, this useful resource will improve our understanding of the role of proteomics in prostate cancer diagnosis and risk assessment. Prostate cancer is the most frequent form of cancer in men, accounting for more than one-third of all cases. Current screening techniques, such as PSA testing used in conjunction with routine procedures, lead to unnecessary biopsies and the discovery of low-risk tumours, resulting in overdiagnosis. SWATH-MS is a well-established data-independent (DI) method requiring prior knowledge of targeted peptides to obtain valuable information from SWATH maps. In response to the growing need to identify and characterise protein biomarkers for prostate cancer, this study explored a spectrum source for targeted proteome analysis of blood samples. We created a comprehensive prostate cancer serum spectral library by combining data-dependent acquisition (DDA) MS raw files from 504 patients with low, intermediate, or high-grade prostate cancer and healthy controls, as well as 304 prostate cancer-related protein in silico assays. The spectral library contains 114,684 transitions, which equates to 18,479 peptides translated into 1227 proteins. The robustness and accuracy of the spectral library were assessed to boost confidence in the identification and quantification of prostate cancer-related proteins across an independent cohort, resulting in the identification of 404 proteins. This unique database can facilitate researchers to investigate prostate cancer protein biomarkers in blood samples. In the real-world use of the spectrum library for biomarker detection, using a signature of 17 proteins, a clear distinction between the validation cohort's pre- and post-treatment groups was observed. Data are available via ProteomeXchange with identifier PXD028651.
Methotrexate (MTX) is the gold-standard first-line disease-modifying anti-rheumatic drug for juvenile idiopathic arthritis (JIA), despite only being either effective or tolerated in half of children and young people (CYP). To facilitate stratified treatment of early JIA, novel methods in machine learning were used to i) identify clusters with distinct disease patterns following MTX initiation; ii) predict cluster membership; and iii) compare clusters to existing treatment response measures. Discovery and verification cohorts included CYP who first initiated MTX before January 2018 in one of four UK multicentre prospective cohorts of JIA within the CLUSTER consortium. JADAS components (active joint count, physician (PGA) and parental (PGE) global assessments, ESR) were recorded at MTX start and over the following year. Clusters of MTX 'response' were uncovered using multivariate group-based trajectory modelling separately in discovery and verification cohorts. Clusters were compared descriptively to ACR Pedi 30/90 scores, and multivariate logistic regression models predicted cluster-group assignment. The discovery cohorts included 657 CYP and verification cohorts 1241 CYP. Six clusters were identified: Fast improvers (11%), Slow Improvers (16%), Improve-Relapse (7%), Persistent Disease (44%), Persistent PGA (8%) and Persistent PGE (13%), the latter two characterised by improvement in all features except one. Factors associated with clusters included ethnicity, ILAR category, age, PGE, and ESR scores at MTX start, with predictive model area under the curve values of 0.65-0.71. Singular ACR Pedi 30/90 scores at 6 and 12 months could not capture speeds of improvement, relapsing courses or diverging disease patterns. Six distinct patterns following initiation of MTX have been identified using methods in artificial intelligence. These clusters demonstrate the limitations in traditional yes/no treatment response assessment (e.g., ACRPedi30) and can form the basis of a stratified medicine programme in early JIA. Medical Research Council, Versus Arthritis, Great Ormond Street Hospital Children's Charity, Olivia's Vision, and the National Institute for Health Research.
In 2016, 13 specific obesity related cancers were identified by IARC. Here, using baseline WHO BMI categories, latent profile analysis (LPA) and latent class trajectory modelling (LCTM) we evaluated the usefulness of one-off measures when predicting cancer risk vs life-course changes. Our results in LPA broadly concurred with the three basic WHO BMI categories, with similar stepwise increase in cancer risk observed. In LCTM, we identified 5 specific trajectories in men and women. Compared to the leanest class, a stepwise increase in risk for obesity related cancer was observed for all classes. When latent class membership was compared to baseline BMI, we found that the trajectories were composed of a range of BMI (baseline) categories. All methods reveal a link between obesity and the 13 cancers identified by IARC. However, the additional information included by LCTM indicates that lifetime BMI may highlight additional group of people that are at risk.
Background Excess body fatness, commonly approximated by a one-off determination of body mass index (BMI), is associated with increased risk of at least 13 cancers. Modelling of longitudinal BMI data may be more informative for incident cancer associations, e.g. using latent class trajectory modelling (LCTM) may offer advantages in capturing changes in patterns with time. Here, we evaluated the variation in cancer risk with LCTMs using specific age recall versus decade recall BMI. Methods We obtained BMI profiles for participants from the Prostate, Lung, Colorectal and Ovarian Cancer Screening Trial. We developed gender-specific LCTMs using recall data from specific ages 20 and 50 years (72,513 M; 74,837 W); decade data from 30s to 70s (42,113 M; 47,352 W) and a combination of both (74,106 M, 76,245 W). Using an established methodological framework, we tested 1:7 classes for linear, quadratic, cubic and natural spline shapes, and modelled associations for obesity-related cancer (ORC) incidence using LCTM class membership. Results Different models were selected depending on the data type used. In specific age recall trajectories, only the two heaviest classes were associated with increased risk of ORC. For the decade recall data, the shapes appeared skewed by outliers in the heavier classes but an increase in ORC risk was observed. In the combined models, at older ages the BMI values were more extreme. Conclusions Specific age recall models supported the existing literature changes in BMI over time are associated with increased ORC risk. Modelling of decade recall data might yield spurious associations.
Summary Background The effectiveness and cost‐effectiveness of biologic therapies for psoriasis are significantly compromised by variable treatment responses. Thus, more precise management of psoriasis is needed. Objectives To identify subgroups of patients with psoriasis treated with biologic therapies, based on changes in their disease activity over time, that may better inform patient management. Methods We applied latent class mixed modelling to identify trajectory‐based patient subgroups from longitudinal, routine clinical data on disease severity, as measured by the Psoriasis Area and Severity Index (PASI), from 3546 patients in the British Association of Dermatologists Biologics and Immunomodulators Register, as well as in an independent cohort of 2889 patients pooled across four clinical trials. Results We discovered four discrete classes of global response trajectories, each characterized in terms of time to response, size of effect and relapse. Each class was associated with differing clinical characteristics, e.g. body mass index, baseline PASI and prevalence of different manifestations. The results were verified in a second cohort of clinical trial participants, where similar trajectories following the initiation of biologic therapy were identified. Further, we found differential associations of the genetic marker HLA‐C*06:02 between our registry‐identified trajectories. Conclusions These subgroups, defined by change in disease over time, may be indicative of distinct endotypes driven by different biological mechanisms and may help inform the management of patients with psoriasis. Future work will aim to further delineate these mechanisms by extensively characterizing the subgroups with additional molecular and pharmacological data. What is already known about this topic? While many patients with psoriasis respond to treatment with biologics, there are those who show little or no response and those who respond initially but then either lose response or suffer from adverse effects. Better characterization of patients who will, or will not, benefit from biologic therapy will facilitate the understanding of relevant biological mechanisms and explain treatment outcome variation in patient cohorts. What does this study add? Using a data‐driven approach, we identified four subgroups of patients with psoriasis defined by global trajectories of response to biologic therapies. Our results were replicated in a second cohort obtained by pooling data from four clinical trials of biologic therapies for psoriasis. We further identified potential human leucocyte antigen biomarkers that help to distinguish between the trajectory‐based subgroups. Linked Comment: L.S. van der Schoot and J.M.P.A. van den Reek. Br J Dermatol 2021; 185:698–699.
Integrating data from different sources into homogeneous dataset increases the opportunities to study human health. However, disparate data collections are often heterogeneous, which complicates their integration. In this paper, we focus on the issue of content heterogeneity in data integration. Traditional approaches for resolving content heterogeneity map all source datasets to a common data model that includes only shared data items, and thus omit all items that vary between datasets. Based on an example of three datasets in Systemic Lupus Erythematosus, we describe and experimentally evaluate a probabilistic data integration approach which propagates the uncertainty resulting from content heterogeneity into statistical inference, avoiding the need to map to a common data model.
This study aimed to demonstrate how to estimate the value of health gain after patients with a multisystem disease achieve a condition-specific composite response endpoint. Data from patients treated in routine practice with an exemplar multisystem disease (systemic lupus erythematosus) were extracted from a national register (British Isles Lupus Assessment Group Biologics Register). Two bespoke composite response endpoints (Major Clinical Response and Improvement) were developed in advance of this study. Difference-in-differences regression compared health utility values (3-level version of EQ-5D; UK tariff) over 6 months for responders and nonresponders. Bootstrapped regression estimated the incremental quality-adjusted life-years (QALYs), probability of QALY gain after achieving the response criteria, and population monetary benefit of response. Within the sample (n = 171), 18.2% achieved Major Clinical Response and 49.1% achieved Improvement at 6 months. Incremental health utility values were 0.0923 for Major Clinical Response and 0.0454 for Improvement. Expected incremental QALY gain at 6 months was 0.020 for Major Clinical Response and 0.012 for Improvement. Probability of QALY gain after achieving the response criteria was 77.6% for Major Clinical Response and 72.7% for Improvement. Population monetary benefit of response was £1 106 458 for Major Clinical Response and £649 134 for Improvement. Bespoke composite response endpoints are becoming more common to measure treatment response for multisystem diseases in trials and observational studies. Health technology assessment agencies face a growing challenge to establish whether these endpoints correspond with improved health gain. Health utility values can generate this evidence to enhance the usefulness of composite response endpoints for health technology assessment, decision making, and economic evaluation.
Objectives. similar to 30% of patients with SLE develop LN. Presence and/or severity of LN are currently assessed by renal biopsy, but biomarkers in serum or urine samples may provide an avenue for non-invasive routine testing. We aimed to validate a urinary protein panel for its ability to predict active renal involvement in SLE. Methods. A total of 197 SLE patients and 48 healthy controls were recruited, and urine samples collected. Seventy-five of the SLE patients had active LN and 104 had no or inactive renal disease. Concentrations of lipocalin-like prostaglandin D synthase (LPGDS), transferrin, alpha-1-acid glycoprotein (AGP-1), ceruloplasmin, monocyte chemoattractant protein 1 (MCP-1) and soluble vascular cell adhesion molecule-1 (sVCAM-1) were quantified by MILLIPLEX (R) Assays using the MAGPIX Luminex platform. Binary logistic regression was conducted to examine whether proteins levels associate with active renal involvement and/or response to rituximab treatment. Results. Urine levels of transferrin (P
Motivation: Data-independent acquisition mass spectrometry allows for comprehensive peptide detection and relative quantification than standard data-dependent approaches. While less prone to missing values, these still exist. Current approaches for handling the so-called missingness have challenges. We hypothesized that non-random missingness is a useful biological measure and demonstrate the importance of analysing missingness for proteomic discovery within a longitudinal study of disease activity. Results: The magnitude of missingness did not correlate with mean peptide concentration. The magnitude of missingness for each protein strongly correlated between collection time points (baseline, 3months, 6months; R=0.95-0.97, confidence interval = 0.94-0.97) indicating little time-dependent effect. This allowed for the identification of proteins with outlier levels of missingness that differentiate between the patient groups characterized by different patterns of disease activity. The association of these proteins with disease activity was confirmed by machine learning techniques. Our novel approach complements analyses on complete observations and other missing value strategies in biomarker prediction of disease activity.
Objectives: Peer review is a powerful tool that steers the education and practice of medical researchers but may allow biased critique by anonymous reviewers. We explored factors unrelated to research quality that may influence peer review reports, and assessed the possibility that sub-types of reviewers exist. Our findings could potentially improve the peer review process. Methods: We evaluated the harshness, constructiveness and positiveness in 596 reviews from journals with open peer review, plus 46 reviews from colleagues' anonymously reviewed manuscripts. We considered possible influencing factors, such as number of authors and seasonal trends, on the content of the review. Finally, using machine-learning we identified latent types of reviewer with differing characteristics. Results: Reviews provided during a northern-hemisphere winter were significantly harsher, suggesting a seasonal effect on language. Reviews for articles in journals with an open peer review policy were significantly less harsh than those with an anonymous review process. Further, we identified three types of reviewers: nurturing, begrudged, and blasé. Conclusion: Nurturing reviews were in a minority and our findings suggest that more widespread open peer reviewing could improve the educational value of peer review, increase the constructive criticism that encourages researchers, and reduce pride and prejudice in editorial processes.
The emergence of novel coronavirus disease 2019 (COVID-19), caused by the SARS-CoV-2 coronavirus, has necessitated the urgent development of new diagnostic and therapeutic strategies. Rapid research and development, on an international scale, has already generated assays for detecting SARS-CoV-2 RNA and host immunoglobulins. However, the complexities of COVID-19 are such that fuller definitions of patient status, trajectory, sequelae, and responses to therapy are now required. There is accumulating evidence-from studies of both COVID-19 and the related disease SARS-that protein biomarkers could help to provide this definition. Proteins associated with blood coagulation (D-dimer), cell damage (lactate dehydrogenase), and the inflammatory response (e.g., C-reactive protein) have already been identified as possible predictors of COVID-19 severity or mortality. Proteomics technologies, with their ability to detect many proteins per analysis, have begun to extend these early findings. To be effective, proteomics strategies must include not only methods for comprehensive data acquisition (e.g., using mass spectrometry) but also informatics approaches via which to derive actionable information from large data sets. Here we review applications of proteomics to COVID-19 and SARS and outline how pipelines involving technologies such as artificial intelligence could be of value for research on these diseases.
Systemic lupus erythematosus (SLE) is a clinically and biologically heterogeneous autoimmune disease. We explored whether the deconvolution of whole blood transcriptomic data could identify differences in predicted immune cell frequency between active SLE patients, and whether these differences are associated with clinical features and/or medication use. Patients with active SLE (BILAG-2004 Index) enrolled in the BILAG-Biologics Registry (BILAG-BR), prior to change in therapy, were studied as part of the MASTERPLANS Stratified Medicine consortium. Whole blood RNA-sequencing (RNA-seq) was conducted at enrolment into the registry. Data were deconvoluted using CIBERSORTx. Predicted immune cell frequencies were compared between active and inactive disease in the nine BILAG-2004 domains and according to immunosuppressant use (current and past). Predicted cell frequency varied between 109 patients. Patients currently, or previously, exposed to mycophenolate mofetil (MMF) had fewer inactivated macrophages (0.435% vs 1.391%, p = 0.001), naïve CD4 T cells (0.961% vs 2.251%, p = 0.002), and regulatory T cells (1.858% vs 3.574%, p = 0.007), as well as a higher proportion of memory activated CD4 T cells (1.826% vs 1.113%, p = 0.015), compared to patients never exposed to MMF. These differences remained statistically significant after adjusting for age, gender, ethnicity, disease duration, renal disease, and corticosteroid use. There were 2607 differentially expressed genes (DEGs) in patients exposed to MMF with over-representation of pathways relating to eosinophil function and erythrocyte development and function. Within CD4 + T cells, there were fewer predicted DEGs related to MMF exposure. No significant differences were observed for the other conventional immunosuppressants nor between patients according disease activity in any of the nine organ domains. MMF has a significant and persisting effect on the whole blood transcriptomic signature in patients with SLE. This highlights the need to adequately adjust for background medication use in future studies using whole blood transcriptomics.
Temporal phenotyping enables clinicians to better understand observable characteristics of a disease as it progresses. Modelling disease progression that captures interactions between phenotypes is inherently challenging. Temporal models that capture change in disease over time can identify the key features that characterize disease subtypes that underpin these trajectories. These models will enable clinicians to identify early warning signs of progression in specific sub-types and therefore to make informed decisions tailored to individual patients. In this paper, we explore two approaches to building temporal phenotypes based on the topology of data: topological data analysis and pseudo time-series. Using type 2 diabetes data, we show that the topological data analysis approach is able to identify disease trajectories and that pseudo time-series can infer a state space model characterized by transitions between hidden states that represent distinct temporal phenotypes. Both approaches highlight lipid profiles as key factors in distinguishing the phenotypes.
Background: Significant evidence suggests that the cholesterol-lowering statins can affect cognitive function and reduce the risk for Alzheimer’s disease (AD) and dementia. These potential effects may be constrained by specific combinations of an individual’s sex and apolipoprotein E (APOE) genotype. Methods: Here we examine data from 252,327 UK Biobank participants, aged 55 or over, and compare the effects of statin use in males and females. We assessed difference in statin treatments taking a matched cohort approach, and identified key stratifiers using regression models and conditional inference trees. Using statistical modeling, we further evaluated the effect of statins on survival, cognitive decline over time, and on AD prevalence. Results: We identified that in the selected population, males were older, had a higher level of education, better cognitive scores, higher incidence of cardiovascular and metabolic diseases, and a higher rate of statin use. We observed that males and those participants with an APOE ε4–positive genotype had higher probabilities of being treated with statins; while participants with an AD diagnosis had slightly lower probabilities. We found that use of statins was not significantly associated with overall higher rates of survival. However, when considering the interaction of statin use with sex, the results suggest higher survival rates in males treated with statins. Finally, examination of cognitive function indicates a potential beneficial effect of statins that is selective for APOE ε4–positive genotypes. Discussion: Our evaluation of the aging population in a large cohort from the UK Biobank confirms sex and APOE genotype as fundamental risk stratifiers for AD and cognitive function, furthermore it extends them to the specific area of statin use, clarifying their specific interactions with treatments. © 2021 The Authors. Alzheimer’s & Dementia: Translational Research & Clinical Interventions published by Wiley Periodicals LLC on behalf of Alzheimer’s Association. Open access journal This item from the UA Faculty Publications collection is made available by the University of Arizona with support from the University of Arizona Libraries. If you have questions, please contact us at repository@u.library.arizona.edu.
•A data analysis pipeline to extract frequent patterns in breast cancer patients using administrative data from EHR.•A Topic Modeling step allows synthesizing the ICD9-CM codes of the procedures carried out during hospitalizations.•Frequent patterns of care are extracted through a careflow mining algorithm.•The results reveal interesting temporal phenotypes, which are different in terms of clinical outcome.•The resulting careflows reflect the clinical practice guidelines enacted at the considered Breast Unit. In this work we describe the application of a careflow mining algorithm to detect the most frequent patterns of care in a cohort of 3000 breast cancer patients. The applied method relies on longitudinal data extracted from electronic health records, recorded from the first surgical procedure after a breast cancer diagnosis. Careflows are mined from events data recorded for administrative purposes, including procedures from ICD9 – CM billing codes and chemotherapy treatments. Events data have been pre-processed with Topic Modelling to create composite events based on concurrent procedures. The results of the careflow mining algorithm allow the discovery of electronic temporal phenotypes across the studied population. These phenotypes are further characterized on the basis of clinical traits and tumour histopathology, as well as in terms of relapses, metastasis occurrence and 5-year survival rates. Results are highly significant from a clinical perspective, since phenotypes describe well characterized pathology classes, and the careflows are well matched with existing clinical guidelines. The analysis thus facilitates deriving real-world evidence that can inform clinicians as well as hospital decision makers.
Weight gain is a common consequence of treatment with antipsychotic drugs in early psychosis, leading to further morbidity and poor treatment adherence. Identifying tools that can predict weight change in early psychosis may contribute to better-individualised treatment and adherence. Recently we showed that proteomic profiling with sequential window acquisition of all theoretical fragment ion spectra (SWATH) mass spectrometry (MS) can identify individuals with pre-diabetes more likely to experience weight change in relation to lifestyle change. We investigated whether baseline proteomic profiles predicted weight change over time using data from the BeneMin clinical trial of the anti-inflammatory antibiotic, minocycline, versus placebo. Expression levels for 844 proteins were determined by SWATH proteomics in 83 people (60 men and 23 women). Hierarchical clustering analysis and principal component analysis of baseline proteomics data did not reveal distinct separation between the proteome profiles of participants in different weight change categories. However, individuals with the highest weight loss had higher Positive and Negative Syndrome Scale (PANSS) scores. Our findings imply that mode of treatment i.e. the pharmacological intervention for psychosis may be the determining factor in weight change after diagnosis, rather than predisposing proteomic dynamics.
Introduction: Establishing efficacy of and molecular pathways for statins has the potential to impact incidence of Alzheimer's and age-related neurodegenerative diseases (NDD). Methods: This retrospective cohort study surveyed US-based Humana claims, which includes prescription and patient records from private-payer and Medicare insurance. Claims from 288,515 patients, aged 45 years and older, without prior history of NDD or neurological surgery, were surveyed for a diagnosis of NDD starting 1 year following statin exposure. Patients were required to be enrolled with claims data for at least 6 months prior to first statin prescription and at least 3 years thereafter. Computational system biology analysis was conducted to determine unique target engagement for each statin. Results: Of the 288,515 participants included in the study, 144,214 patients (mean [standard deviation (SD)] age, 67.22 [3.8] years) exposed to statin therapies, and 144,301 patients (65.97 [3.2] years) were not treated with statins. The mean (SD) follow-up time was 5.1 (2.3) years. Exposure to statins was associated with a lower incidence of Alzheimer's disease (1.10% vs 2.37%; relative risk [RR], 0.4643; 95% confidence interval [CI], 0.44-0.49; P < .001), dementia 3.03% vs 5.39%; RR, 0.56; 95% CI, 0.54-0.58; P < .001), multiple sclerosis (0.08% vs 0.15%; RR, 0.52; 95% CI, 0.410.66; P < .001), Parkinson's disease (0.48% vs 0.92%; RR, 0.53; 95% CI, 0.48-0.58; P < .001), and amyotrophic lateral sclerosis (0.02% vs 0.05%; RR, 0.46; 95% CI, 0.300.69; P < .001). All NDD incidence for all statins, except for fluvastatin (RR, 0.91; 95% CI, 0.65-1.30; P = 0.71), was reduced with variances in individual risk profiles. Pathway analysis indicated unique and common profiles associated with risk reduction efficacy. Discussion: Benefits and risks of statins relative to neurological outcomes should be considered when prescribed for at-risk NDD populations. Common statin activated pathways indicate overarching systems required for risk reduction whereas unique targets could advance a precision medicine approach to prevent neurodegenerative diseases.
The severe acute respiratory syndrome virus SARS-CoV-2, a close relative of the SARS-CoV virus, is the cause of the recent COVID-19 pandemic affecting, to date, over 14 million individuals across the globe and demonstrating relatively high rates of infection and mortality. A third virus, the H5N1, responsible for avian influenza, has caused infection with some clinical similarities to those in COVID-19 infections. Cytokines, small proteins that modulate immune responses, have been directly implicated in some of the severe responses seen in COVID-19 patients, e.g. cytokine storms. Understanding the immune processes related to COVID-19, and other similar infections, could help identify diagnostic markers and therapeutic targets. Here we examine data of cytokine, immune cell types, and disease associations captured from biomedical literature associated with COVID-19, Coronavirus in general, SARS, and H5N1 influenza, with the objective of identifying potentially useful relationships and areas for future research. Cytokine and cell-type associations captured from Medical Subject Heading (MeSH) terms linked to thousands of PubMed records, has identified differing patterns of associations between the four corpuses of publications (COVID-19, Coronavirus, SARS, or H5N1 influenza). Clustering of cytokine-disease co-occurrences in the context of Coronavirus has identified compelling clusters of co-morbidities and symptoms, some of which already known to be linked to COVID-19. Finally, network analysis identified sub-networks of cytokines and immune cell types associated with different manifestations, co-morbidities and symptoms of Coronavirus, SARS, and H5N1. Systematic review of research in medicine is essential to facilitate evidence-based choices about health interventions. In a fast moving pandemic the approach taken here will identify trends and enable rapid comparison to the literature of related diseases.
Background Missing values are a key issue in the statistical analysis of proteomic data. Defining the strategy to address missing values is a complex task in each study, potentially affecting the quality of statistical analyses. Results We have developed OptiMissP, a dashboard to visually and qualitatively evaluate missingness and guide decision making in the handling of missing values in proteomics studies that use data-independent acquisition mass spectrometry. It provides a set of visual tools to retrieve information about missingness through protein densities and topology-based approaches, and facilitates exploration of different imputation methods and missingness thresholds. Conclusions OptiMissP provides support for researchers' and clinicians' qualitative assessment of missingness in proteomic datasets in order to define study-specific strategies for the handling of missing values. OptiMissP considers biases in protein distributions related to the choice of imputation method and helps analysts to balance the information loss caused by low missingness thresholds and the noise introduced by selecting high missingness thresholds. This is complemented by topological data analysis which provides additional insight to the structure of the data and their missingness. We use an example in Chronic Kidney Disease to illustrate the main functionalities of OptiMissP.
Systemic lupus erythematosus (SLE) is a heterogeneous systemic autoimmune condition for which there are limited licensed therapies. Clinical trial design is challenging in SLE due at least in part to imperfect outcome measures. Improved understanding of how disease activity changes over time could inform future trial design. The aim of this study was to determine whether distinct trajectories of disease activity over time occur in patients with active SLE within a clinical trial setting and to identify factors associated with these trajectories. Latent class trajectory models were fitted to a clinical trial dataset of a monoclonal antibody targeting CD22 (Epratuzumab) in patients with active SLE using the numerical BILAG-2004 score (nBILAG). The baseline characteristics of patients in each class and changes in prednisolone over time were identified. Exploratory PK-PD modelling was used to examine cumulative drug exposure in relation to latent class membership. Five trajectories of disease activity were identified, with 3 principal classes: non-responders (NR), slow responders (SR) and rapid-responders (RR). In both the SR and RR groups, significant changes in disease activity were evident within the first 90 days of the trial. The SR and RR patients had significantly higher baseline disease activity, exposure to epratuzumab and activity in specific BILAG domains, whilst NR had lower steroid use at baseline and less change in steroid dose early in the trial. Longitudinal nBILAG scores reveal different trajectories of disease activity and may offer advantages over fixed endpoints. Corticosteroid use however remains an important confounder in lupus trials and can influence early response. Changes in disease activity and steroid dose early in the trial were associated with the overall disease activity trajectory, supporting the feasibility of performing adaptive trial designs in SLE.
To determine whether using a reweighted disease activity score that better reflects joint synovitis, i.e., the 2-component Disease Activity Score in 28 joints (DAS28) (based on swollen joint count and C-reactive protein level), produces more clinically relevant treatment outcome trajectories compared to the standard 4-component DAS28. Latent class mixed modeling of response to biologic treatment was applied to 2,991 rheumatoid arthritis (RA) patients in whom treatment with a biologic disease-modifying antirheumatic drug was being initiated within the Biologics in Rheumatoid Arthritis Genetics and Genomics Study Syndicate cohort, using both 4-component and 2-component DAS28 scores as outcome measures. Patient groups with similar trajectories were compared in terms of pretreatment baseline characteristics (including disability and comorbidities) and follow-up characteristics (including antidrug antibody events, adherence to treatments, and blood drug levels). We compared the trajectories obtained using the 4- and 2-component scores to determine which characteristics were better captured by each. Using the 4-component DAS28, we identified 3 trajectory groups, which is consistent with previous findings. We showed that the 4-component DAS28 captures information relating to depression. Using the 2-component DAS28, 7 trajectory groups were identified; among them, distinct groups of nonresponders had a higher incidence of respiratory comorbidities and a higher proportion of antidrug antibody events. We also identified a group of patients for whom the 2-component DAS28 scores remained relatively low; this group included a high percentage of patients who were nonadherent to treatment. This highlights the utility of both the 4- and 2-component DAS28 for monitoring different components of disease activity. Here we show that the 2-component modified DAS28 defines important biologic and clinical phenotypes associated with treatment outcome in RA and characterizes important underlying response mechanisms to biologic drugs.
Introduction Exacerbation-prone asthma subtype has been reported in studies using data-driven methodologies. However, patterns of severe exacerbations have not been studied. Objective To investigate longitudinal trajectories of severe wheeze exacerbations from infancy to school age. Methods We applied longitudinal k-means clustering to derive exacerbation trajectories among 887 participants from a population-based birth cohort with severe wheeze exacerbations confirmed in healthcare records. We examined early-life risk factors of the derived trajectories, and their asthma-related outcomes and lung function in adolescence. Results 498/887 children (56%) had physician-confirmed wheeze by age 8 years, of whom 160 had at least one severe exacerbation. A two-cluster model provided the optimal solution for severe exacerbation trajectories among these 160 children: "Infrequent exacerbations (IE)" (n = 150, 93.7%) and "Early-onset frequent exacerbations (FE)" (n = 10, 6.3%). Shorter duration of breastfeeding was the strongest early-life risk factor for FE (weeks, median [IQR]: FE, 0 [0-1.75] vs. IE, 6 [0-20], P < .001). Specific airway resistance (sR(aw)) was significantly higher in FE compared with IE trajectory throughout childhood. We then compared children in the two exacerbation trajectories with those who have never wheezed (NW, n = 389) or have wheezed but had no severe exacerbations (WNE, n = 338). At age 8 years, FEV1/FVC was significantly lower and FeNO significantly higher among FE children compared with all other groups. By adolescence (age 16), subjects in FE trajectory were significantly more likely to have current asthma (67% FE vs. 30% IE vs. 13% WNE, P < .001) and use inhaled corticosteroids (77% FE vs. 15% IE vs. 18% WNE, P < .001). Lung function was significantly diminished in the FE trajectory (FEV1/FVC, mean [95%CI]: 89.9% [89.3-90.5] vs. 88.1% [87.3-88.8] vs. 85.1% [83.4-86.7] vs. 74.7% [61.5-87.8], NW, WNE, IE, FE respectively, P < .001). Conclusion We have identified two distinct trajectories of severe exacerbations during childhood with different early-life risk factors and asthma-related outcomes in adolescence.
In recent years, high-throughput sequencing technologies provide unprecedented opportunity to depict cancer samples at multiple molecular levels. The integration and analysis of these multi-omics datasets is a crucial and critical step to gain actionable knowledge in a precision medicine framework. This paper explores recent data-driven methodologies that have been developed and applied to respond major challenges of stratified medicine in oncology, including patients' phenotyping, biomarker discovery, and drug repurposing. We systematically retrieved peer-reviewed journals published from 2014 to 2019, select and thoroughly describe the tools presenting the most promising innovations regarding the integration of heterogeneous data, the machine learning methodologies that successfully tackled the complexity of multi-omics data, and the frameworks to deliver actionable results for clinical practice. The review is organized according to the applied methods: Deep learning, Network-based methods, Clustering, Features Extraction, and Transformation, Factorization. We provide an overview of the tools available in each methodological group and underline the relationship among the different categories. Our analysis revealed how multi-omics datasets could be exploited to drive precision oncology, but also current limitations in the development of multi-omics data integration.
Background Individual clinical trials and cohort studies are a useful source of data, often under-utilised once a study has ended. Pooling data from multiple sources could increase sample sizes and allow for further investigation of treatment effects; even if the original trial did not meet its primary goals. Through the MASTERPLANS (MAximizing Sle ThERapeutic PotentiaL by Application of Novel and Stratified approaches) national consortium, focused on Systemic Lupus Erythematosus (SLE), we have gained valuable real-world experiences in aligning, harmonising and combining data from multiple studies and trials, specifically where standards for data capture, representation and documentation, were not used or were unavailable. This was not without challenges arising both from the inherent complexity of the disease and from differences in the way data were captured and represented across different studies. Main body Data were, unavoidably, aligned by hand, matching up equivalent or similar patient variables across the different studies. Heterogeneity-related issues were tackled and data were cleaned, organised and combined, resulting in a single large dataset ready for analysis. Overcoming these hurdles, often seen in large-scale data harmonization and integration endeavours of legacy datasets, was made possible within a realistic timescale and limited resource by focusing on specific research questions driven by the aims of MASTERPLANS. Here we describe our experiences tackling the complexities in the integration of large, diverse datasets, and the lessons learned. Conclusions Harmonising data across studies can be complex, and time and resource consuming. The work carried out here highlights the importance of using standards for data capture, recording, and representation, to facilitate both the integration of large datasets and comparison between studies. Where standards are not implemented at the source harmonisation is still possible by taking a flexible approach, with systematic preparation, and a focus on specific research questions.
Novel machine learning methods open the door to advances in rheumatology through application to complex, high-dimensional data, otherwise difficult to analyse. Results from such efforts could provide better classification of disease, decision support for therapy selection, and automated interpretation of clinical images. Nevertheless, such data-driven approaches could potentially model noise, or miss true clinical phenomena. One proposed solution to ensure clinically meaningful machine learning models is to involve primary stakeholders in their development and interpretation. Including patient and health care professionals' input and priorities, in combination with statistical fit measures, allows for any resulting models to be well fit, meaningful, and fit for practice in the wider rheumatological community. Here we describe outputs from workshops that involved healthcare professionals, and young people from the Your Rheum Young Person's Advisory Group, in the development of complex machine learning models. These were developed to better describe trajectory of early juvenile idiopathic arthritis disease, as part of the CLUSTER consortium. We further provide key instructions for reproducibility of this process.Involving people living with, and managing, a disease investigated using machine learning techniques, is feasible, impactful and empowering for all those involved.
ObjectiveSystemic lupus erythematosus (SLE) is a clinically and biologically heterogenous autoimmune disease. We aimed to investigate the plasma proteome of patients with active SLE to identify novel subgroups, or endotypes, of patients.MethodPlasma was collected from patients with active SLE who were enrolled in the British Isles Lupus Assessment Group Biologics Registry (BILAG-BR). The plasma proteome was analysed using a data-independent acquisition method, Sequential Window Acquisition of All theoretical mass spectra mass spectrometry (SWATH-MS). Unsupervised, data-driven clustering algorithms were used to delineate groups of patients with a shared proteomic profile.ResultsIn 223 patients, six clusters were identified based on quantification of 581 proteins. Between the clusters, there were significant differences in age (p = 0.012) and ethnicity (p = 0.003). There was increased musculoskeletal disease activity in cluster 1 (C1), 19/27 (70.4%) (p = 0.002) and renal activity in cluster 6 (C6) 15/24 (62.5%) (p = 0.051). Anti-SSa/Ro was the only autoantibody that significantly differed between clusters (p = 0.017). C1 was associated with p21-activated kinases (PAK) and Phospholipase C (PLC) signalling. Within C1 there were two sub-clusters (C1A and C1B) defined by 49 proteins related to cytoskeletal protein binding. C2 and C6 demonstrated opposite Rho family GTPase and Rho GDI signalling. Three proteins (MZB1, SND1 and AGL) identified in C6 increased the classification of active renal disease although this did not reach statistical significance (p = 0.0617).ConclusionsUnsupervised proteomic analysis identifies clusters of patients with active SLE, that are associated with clinical and serological features, which may facilitate biomarker discovery. The observed proteomic heterogeneity further supports the need for a personalised approach to treatment in SLE.
Background CLUSTER is a UK consortium focussed on precision medicine research in JIA/JIA-Uveitis. As part of this programme, a large-scale JIA data resource was created by harmonizing and pooling existing real-world studies. Here we present challenges and progress towards creation of this unique large JIA dataset. Methods Four real-world studies contributed data; two clinical datasets of JIA patients starting first-line methotrexate (MTX) or tumour necrosis factor inhibitors (TNFi) were created. Variables were selected based on a previously developed core dataset, and encrypted NHS numbers were used to identify children contributing similar data across multiple studies. Results Of 7013 records (from 5435 individuals), 2882 (1304 individuals) represented the same child across studies. The final datasets contain 2899 (MTX) and 2401 (TNFi) unique patients; 1018 are in both datasets. Missingness ranged from 10% to 60% and was not improved through harmonisation. Conclusions Combining data across studies has achieved dataset sizes rarely seen in JIA, invaluable to progressing research. Losing variable specificity and missingness, and their impact on future analyses requires further consideration.
Treatments for COVID-19 infections have improved dramatically since the beginning of the pandemic, and glucocorticoids have been a key tool in improving mortality rates. The UK’s National Institute for Health and Care Excellence guidance is for treatment to be targeted only at those requiring oxygen supplementation, however, and the interactions between glucocorticoids and COVID-19 are not completely understood. In this work, a multi-omic analysis of 98 inpatient-recruited participants was performed by quantitative metabolomics (using targeted liquid chromatography-mass spectrometry) and data-independent acquisition proteomics. Both ‘omics datasets were analysed for statistically significant features and pathways differentiating participants whose treatment regimens did or did not include glucocorticoids. Metabolomic differences in glucocorticoid-treated patients included the modulation of cortisol and bile acid concentrations in serum, but no alleviation of serum dyslipidemia or increased amino acid concentrations (including tyrosine and arginine) in the glucocorticoid-treated cohort relative to the untreated cohort. Proteomic pathway analysis indicated neutrophil and platelet degranulation as influenced by glucocorticoid treatment. These results are in keeping with the key role of platelet-associated pathways and neutrophils in COVID-19 pathogenesis and provide opportunity for further understanding of glucocorticoid action. The findings also, however, highlight that glucocorticoids are not fully effective across the wide range of ‘omics dysregulation caused by COVID-19 infections.
Latent class trajectory models (LCTMs) are often used to identify subgroups of patients that are clinically meaningful in terms of longitudinal exposure and outcome, e.g. drug response patterns. These models are increasingly applied in medicine and epidemiology. However, in many published studies, it is not clear whether the chosen models, where subgroups of patients are identified, represent real heterogeneity in the population, or whether any associations with clinically meaningful characteristics are accidental. In particular, we note an apparent over-reliance on lowest AIC or BIC values. While these are objective measures of goodness of fit, and can help identify the optimal number of subgroups, they are not sufficient on their own to fully evaluate a given trajectory model. Here we demonstrate how longitudinal latent class models can substantially change by making small modifications in model specification, and the impact of this on the relationship to clinical outcomes. We show that the predicted trajectory patterns and outcome probabilities differ when pre-specified cubic versus linear shapes are tested on the same data. However, both could be interpreted to be the " correct " model. We emphasise that LCTMs, like all unsupervised approaches, are hypotheses generating, and should not be directly implemented in clinical practice without significant testing and validation.
Additional publications
John Reynolds, Jen Prattely, Nophar Geifman, Mark Lunt, MASTERPLANS Consortium, Caroline Gordon, and Ian Bruce. Distinct patterns of disease activity over time in patients with active SLE revealed using latent class trajectory models. Arthritis Research & Therapy (2021)
Stephanie JW Shoop-Worrall, Katherine Cresswell, Imogen Bolger, Beth Dillon, Kimme L Hyrich, and Nophar Geifman. Nothing about us without us: involving patient collaborations for machine learning applications in rheumatology. Annals of the Rheumatic Diseases (2021)
Charlotte Watson, Andrew G Renehan, and Nophar Geifman; Associations of specific-age and decade recall body mass index trajectories with obesity-related cancer. BMC Cancer (2021)
Nophar Geifman, Narges Azadbakht, Jiaping Zeng, Toby Wilkinson, Nick Dand, Catherine H. Smith, Iain Buchan, Deborah Stocken, Nick J. Reynolds, Michael R. Barnes, Richard B. Warren, Jonathan Barker, Christopher E. M. Griffiths, Niels Peek, and the BADBIR Study Group, on behalf of the PSORT Consortium. Defining Treatment Response Trajectories in Psoriasis using Large-scale Patient-level Data. British Journal of Dermatology (2021).
Angelica Arioli, Arianna Dagliati, Niels Peek, Philip Kalra, Anthony D. Whetton, and Nophar Geifman; OptiMissP: a dashboard to assess missingness in proteomic data-independent acquisition mass spectrometry. PlosOne (2021).
Arianna Dagliati, Roberta Diaz-Brinton, Niels Peek, and Nophar Geifman; Sex and APOE genotype differences related to statin use in the aging population. Alzheimer’s & Dementia: Translational research & Clinical Interventions (2021)
Stephanie JW Shoop-Worrall, Kimme L Hyrich, Lucy R Wedderburn, Wendy Thomson, and Nophar Geifman; on behalf of CAPS and the CLUSTER consortium. Patient-reported wellbeing and clinical disease measures over time captured by multivariate trajectories of disease activity in individuals with juvenile idiopathic arthritis in the UK: a multicentre prospective longitudinal study. Lancet Rheumatology (2021)
Georgina Torrandell-Haro, Gregory L. Branigan, Francesca Vitali, Nophar Geifman, Julie M. Zissimopoulos, and Roberta Diaz Brinton; Statin therapy and risk of Alzheimer's and age‐related neurodegenerative diseases. Alzheimer's & Dementia – Translational Research & Clinical Interventions (2020)
Adrian Heald, Narges Azadbakht, Bethany Geary, Nophar Geifman, Helene Fachim, Oliver Howes, Anthony Whetton, and Bill Deakin. Application of SWATH mass spectrometry in the identification of circulating proteins that predict future weight gain in early psychosis. Clinical Proteomics (2020)
Nophar Geifman and Anthony D. Whetton; A consideration of publication-derived immune-related associations in Coronavirus and related lung damaging diseases. Journal of Translational Medicine (2020)
Anthony D. Whetton, George W. Preston, Semira Abubeker, and Nophar Geifman; Proteomics and informatics for understanding phases and identifying biomarkers in COVID-19 disease. Journal of Proteome Research (2020)
Helen Le Sueur, Ian Bruce, and Nophar Geifman. The challenges in data integration – heterogeneity and complexity in clinical trials and patient registries. BMC Medical Research Methodology (2020)
Helen Le Sueur, Arianna Dagliati, Iain Buchan, Anthony D. Whetton, Glen P. Martin, Tim Dornan, and Nophar Geifman; Pride and Prejudice – what can we learn from peer review? Medical Teacher (2020)
Arianna Dagliati, Nophar Geifman, Niels Peek, John Holmes Lucia Sacchi, Riccardo Bellazzi, Seyed Erfan Sajjadi, and Allan Tucker. Using Topological Data Analysis and Pseudo Time Series to Infer Temporal Phenotypes from Electronic Health Records. Artificial Intelligence in Medicine (2020)
Francesca Vitali, Giovanna Nicora, Arianna Dagliati, Nophar Geifman and Riccardo Bellazzi; Integrated multi-omics analyses in oncology: a review of machine learning methods and tools. Frontiers in Oncology (2020)
Arianna Dagliati, Darren Plant, Nisha Nair, Meghna Jani, Beatrice Amico, Niels Peek, Anne Morgan, John Isaacs, Anthony Wilson, Kimme Hyrich, Nophar Geifman§, and Anne Barton§. Latent Class Trajectory Modeling of 2-Component Disease Activity Score in 28 Joints Identifies Multiple Rheumatoid Arthritis Phenotypes of Response to Biologic Disease-Modifying Antirheumatic Drugs. § joint last-author. Arthritis & Rheumatology (2020)
Lorenzo Chiudinelli, Arianna Dagliati, Valentina Tibollo, Sara Albasini, Nophar Geifman, Niels Peek, John H. Holmes, Fabio Corsi, Riccardo Bellazzi, Lucia Sacchi; Mining post-surgical care processes in breast cancer patients. Artificial Intelligence in Medicine, special issue on AI in Medicine and The Breast (2020)
Beatrice Amico, Arianna Dagliati, Darren Plant, Anne Barton, Niels Peek, and Nophar Geifman; A Dashboard for Latent Class Trajectory Modelling: application in Rheumatoid Arthritis. Studies in health technology and informatics, 264, pp.911-915 (2019)
Kathryn A. McGurk, Arianna Dagliati, Davide Chiasserini, Dave Lee, Darren Plant, Ivona Baricevic-Jones, Janet Kelsall, Rachael Eineman, Rachel Reed, Bethany Geary, Richard D. Unwin, Anna Nicolaou, Bernard D. Keavney, Anne Barton, Anthony D. Whetton, and Nophar Geifman; The use of missing values in proteomic data-independent acquisition mass spectrometry to enable disease activity discrimination. Bioinformatics (2019)
Matea Deliu, Sara Fontanella, Sadia Haider, Matthew Sperrin, Nophar Geifman, Clare Murray, Angela Simpson, and Adnan Custovic. Longitudinal trajectories of severe wheeze exacerbations from infancy to school age and their association with early-life risk factors and late asthma outcomes. Clinical and Experimental Allergy (2019)
Toby Wilkinson, Siddharth Sinha, Niels Peek, and Nophar Geifman; Clinical trial data reuse – overcoming the complexities in trial design and data sharing. BMC Trials (2019).
Zsolt Zador, Alex Landry, Michael Cusimano, and Nophar Geifman; Multimorbidity states associated with higher mortality rates in organ dysfunction and sepsis: a data-driven analysis in critical care. Critical Care (2019).
Nophar Geifman and Eitan Rubin. The Age-Phenome Database. SpringerPlus, 1.1: p1-8 (2012).