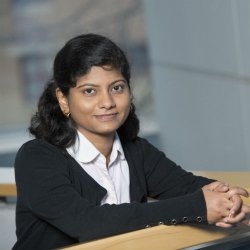
Dr Suparna De
Academic and research departments
Computer Science Research Centre, Surrey Institute for People-Centred Artificial Intelligence (PAI), School of Computer Science and Electronic Engineering.About
Biography
Suparna De is a Lecturer in the Department of Computer Science, a Surrey AI Fellow in the Surrey Institute for People-Centred AI and an Honorary Senior Research Fellow in the Social Research Institute at UCL, UK. She is also a visiting lecturer at the University of Granada, Spain, on the Master's degree in Software Development, Data Processing and Analysis - Representation and Processing of Information and Semantic Web (RTIWS: Representación y Tratamiento de la Información y Web Semántica).
She is a member of the Distributed and Networked Systems and Nature Inspired Computing and Engineering (NICE) research groups.
She completed her Ph.D. and MSc. (with distinction) degrees at the Dept. of Electronic Engineering from the University of Surrey.
She serves on the editorial board of Nature Scientific Reports (Computational Science division) and Elsevier High-Confidence Computing journals.
Areas of specialism
University roles and responsibilities
- Computer Science Admissions Tutor (UG)
Affiliations and memberships
News
In the media
ResearchResearch interests
Suparna's research applies machine learning and Semantic Web technologies to the broad domain of knowledge and data engineering, including deep learning for text data (derived from social networks and longitudinal social science datasets), semantic modelling and search and IoT data analytics.
Her current work focusses on researching machine comprehension and information extraction for longitudinal text, applied to metadata extraction and uplift for social science questionnaires, and adaptive privacy-preserving models for online social networks.
Previous work has included research in Big Data analytics, visualisation and data fusion techniques for understanding city dynamics from multimodal spatio-temporal data.
Research projects
EPSRC-funded, Co-Investigator (Co-I), April 2022 - March 2025.
This three-year, £3.4 million project will produce Privacy-Enhancing Technologies (PETs) to support the online privacy and safety of people navigating significant life transitions. The researchers on the project are comprised of a multi-disciplinary team of experts in Cybersecurity, Psychology, Law, Business, and Criminology from the Universities of Cambridge, Edge Hill, Edinburgh, Queen Mary University of London, Strathclyde and Surrey.
KTP - Predictive Maintenance for Rail InfrastructureInnovateUK-funded, Co-Principal Investigator (PI) and Academic Lead, August 2023 - Jan 2026. Grant number: 10054741
This 30-month, £212k Knowledge Transfer partnership (KTP) grant, funded by Innovate UK, will develop novel machine learning algorithms and models for a cloud-based predictive maintenance platform that also enables real-time monitoring and risk prediction of IoT multi-sensors that sense critical parts of the rail infrastructure (i.e. tracks, bridges, structures supporting overhead lines).
Understanding the multiple dimensions of prediction of concepts in social and biomedical science questionnairesScience and Technology Facilities (STFC) DiRAC-funded, PI, Oct. '21 - March '22.
Part of Grant Number: ST/S003916/1
This grant is a collaboration with Jon Johnson at IoE, UCL, and RITS, UCL. The project investigates various dimensions of concept prediction: against a range of different types of unseen data from UK Data Archive's longitudinal studies, e.g. social science vs biomedical, 1995 vs 2015; and to build up an understanding of different predictions rate by category. Hierarchical approaches for topic classification against the European Language Social Science Thesaurus (ELSST) thesaurus were developed.
ESRC-funded, PI, Feb. 2021 - Feb. 2022.
Total funding amount: £81,500. Part of Grant Number: ES/K000357/1
This grant is a collaboration with Jon Johnson at the Institute of Education, UCL, London. The project investigates automated extraction of metadata from the Cohort and Longitudinal Studies Enhancement Resources (CLOSER) UK Data Archive. Automation of question extraction from paper questionnaires will form part of a pipeline to populate question banks and other metadata repositories and provide a low cost solution to the manual processes undertaken as part of the CLOSER project and UKDA (and other archives) to enhance survey metadata alongside the data description.
Science and Technology Facilities (STFC) DiRAC-funded, PI, Feb. - Dec. 2021. Part of Grant Number: ST/S003916/1.
This grant is a collaboration with Jon Johnson at IoE, UCL, and RITS, UCL. The project will utilise the questions (question text and response domains) and linked concepts (based on the European Language Social Science Thesaurus (ELSST)) held in CLOSER Discovery, the CLOSER metadata store. The aim is to create a model that will be able to classify existing questions (and predict from new questions) to these existing concepts in ELSST.
TagItSmart (2015-18) is a Smart Tags driven service platform for enabling ecosystems of connected objects that dynamically change their status in response to a variety of environmental factors and be seamlessly tracked during their lifecycle. TagItSmart is developing smart tags that combine the power of functional inks with the pervasiveness of digital and electronic markers, e.g. dynamic QR codes, NFC tags, in order to capture new contextual information. Beside this, the ubiquitous presence of smartphones with their cameras and NFC readers facilitates seamless observation measurements and lifecycle tracking of smart tag big data.
I design and develop semantic models and reasoning algorithms for capturing the characteristics of the Smart Tags and to provide decision-support mechanisms for connecting their lifecycle data to semantically-enabled workflows.
The EU H2020 frontierCities2 project (December 2016 - November 2018) is a acceleration and incubation programme that aims to accelerate market update of the FIWARE generic enablers in the Internet of Things and Smart Cities domain by targeting SMEs and startups to develop FIWARE-enabled smart mobility and smart city solutions.
I work together with the FIWARE Foundation to deliver technical coaching to the startups and lead the work-package tasked with further developing the FIWARE enablers and support mechanisms.
The iKaaS project (Oct 2015 - Oct 2017), jointly funded by the EC H2020 programme and MIC, Japan, delivers a secure, robust and scalable multi-cloud platform that brings together the paradigms of IoT, Big Data and the Cloud.
I researched aspects of data analysis in smart city platforms built on heterogeneous cloud platforms. As part of this, we developed novel anomaly detection algorithms for city environmental features (such as measured air pollution levels) and real-time detection of city social events by analysing Twitter feeds. The research delivered tools to discover correlation between large-scale city events and anomalies detected in pollution levels.
The IoT.est project (2011-14), funded by the EU FP7 Programme, developed a test-driven service creation environment for Internet of Things enabled business services. I served as the work-package leader for semantic annotation and large-scale discovery of IoT services, as well as the University of Surrey technical coordinator. I was also in-charge of the proof-of-concept project demonstrator that integrated modules from project partners.
The iCore project (2011-14), funded by the EU FP7 Programme, defined the concept of Virtual Objects (VO) to abstract the technological heterogeneity derived from the vast amounts of heterogeneous objects and devices forming part of the IoT. I researched the dynamic association derivation between ICT and real-world objects and service workflow composition in iCore.
The IoT-A project (2010-13), funded by the EU FP7 Programme, was the lighthouse EU project that defined a reference architecture and model for the IoT, including defining its constituent concepts such as entity, resource and IoT service. I was the deputy leader of the WP that researched various mechanisms for resolution framewoks for the IoT.
MVCE Core 4I worked as a PhD researcher on the Mobile VCE Core 4 Programme on Ubiquitous Services (funded by the UK Technology Strategy Board), work area: ontology-based context management for mobile systems.
Indicators of esteem
Scholarships:
- Overseas Research Student Sponsorship for PhD research: University of Surrey and MobileVCE Core 4 programme
- DFIDSSS Scholarship: jointly funded by the University of Surrey and the British Commonwealth Scholarship Commission for MSc programme.
Awards:
- Cable and Wireless Award: University of Surrey, for the best overall performance from a student graduating with an MSc in Satellite Communication Engineering or Communications Networks and Software
- IET Certificate in recognition of significant contribution to IET On Campus at the University of Surrey
Research Themes
Data analytics for Cyber-Physical and Social Streams
This research theme focusses on deriving latent patterns in CPSS data streams by developing novel ML algorithms; as well as fusion of social network data with sensor data streams. Our work looks at analysing social network data to extract real-world event information and human movements, in conjunction with sensor data and open datasets, to achieve outcomes related to quantifying their influence on smart city dynamics, such as traffic flows, measured pollution levels, redefining inner-city boundaries etc.
Related publications:
- Exploring the Effectiveness of Service Decomposition in Fog Computing Architecture for the Internet of Things, IEEE Transactions on Sustainable Computing, March 2019
- A Survey on an Emerging Area: Deep Learning for Smart City Data, IEEE Transactions on Emerging Topics in Computational Intelligence, May 2019
- Data-driven Air Quality Characterisation for Urban Environments: a Case Study, IEEE Access, December 2018
- Missing Data Estimation in Mobile Sensing Environments, IEEE Access 6(1), October 2018
- Cyber–Physical–Social Frameworks for Urban Big Data Systems: A Survey. MDPI Applied Sciences, 2017, 7 (10) (slideshare)
- Real world city event extraction from Twitter data streams, Procedia Computer Science, 2016, 98, pp. 443-448 (dataset)
Large scale sensor and data discovery and ranking
This research theme focuses on scalable, distributed discovery mechanisms supporting the sensor-as-a-service paradigm. Based on the particular localization characteristics of IoT deployments, our work has developed novel methods using geospatial indexing techniques and semantic service technologies for both sensor and sensor data discovery.
Related publications:
- Designing the Sensing as a Service Ecosystem for the Internet of Things, IEEE Internet of Things Magazine,1(2), December 2018
- Spatial Indexing for Data Searching in Mobile Sensing Environments. Sensors 2017, 17(6), 1427
- Search Techniques for the Web of Things: A Taxonomy and Survey, Sensors 2016, 16(5), 600
- An Experimental Study on Geospatial Indexing for Sensor Service Discovery, Expert Systems with Applications, Elsevier, May 2015 (ontology, dataset)
- A Ranking Method for Sensor Services based on Estimation of Service Access Cost, Information Sciences, Elsevier, October 2015 (code and dataset)
- Enabling Query of Frequently Updated Data from Mobile Sensing Sources, The 13th IEEE International Conferences on Ubiquitous Computing and Communications (IUCC2014), December 2014.
Semantic Models for the Internet of Things
This research theme focusses on semantic models for the main abstractions and concepts that underlie the IoT domain. The developed ontologies enable fine-grained semantic annotations, and to create Linked sensor data for service and data discovery.
Related publications:
- SmartTags: IoT Product Passport for Circular Economy Based on Printed Sensors and Unique Item-Level Identifiers, Sensors 2019, 19(3), 586.
- Knowledge Representation in the Internet of Things: Semantic Modelling and its Applications. Automatika – Journal for Control, Measurement, Electronics, Computing and Communications, December 2013.
- An Internet of Things Platform for Real-World and Digital Objects'. Scalable Computing: Practice and Experience, May 2012. (platform demo)
- Service modelling for the Internet of Things, IEEE Federated Conference on Computer Science and Information Systems (FEDCSIS), September 2011 (ontology)
Research interests
Suparna's research applies machine learning and Semantic Web technologies to the broad domain of knowledge and data engineering, including deep learning for text data (derived from social networks and longitudinal social science datasets), semantic modelling and search and IoT data analytics.
Her current work focusses on researching machine comprehension and information extraction for longitudinal text, applied to metadata extraction and uplift for social science questionnaires, and adaptive privacy-preserving models for online social networks.
Previous work has included research in Big Data analytics, visualisation and data fusion techniques for understanding city dynamics from multimodal spatio-temporal data.
Research projects
EPSRC-funded, Co-Investigator (Co-I), April 2022 - March 2025.
This three-year, £3.4 million project will produce Privacy-Enhancing Technologies (PETs) to support the online privacy and safety of people navigating significant life transitions. The researchers on the project are comprised of a multi-disciplinary team of experts in Cybersecurity, Psychology, Law, Business, and Criminology from the Universities of Cambridge, Edge Hill, Edinburgh, Queen Mary University of London, Strathclyde and Surrey.
InnovateUK-funded, Co-Principal Investigator (PI) and Academic Lead, August 2023 - Jan 2026. Grant number: 10054741
This 30-month, £212k Knowledge Transfer partnership (KTP) grant, funded by Innovate UK, will develop novel machine learning algorithms and models for a cloud-based predictive maintenance platform that also enables real-time monitoring and risk prediction of IoT multi-sensors that sense critical parts of the rail infrastructure (i.e. tracks, bridges, structures supporting overhead lines).
Science and Technology Facilities (STFC) DiRAC-funded, PI, Oct. '21 - March '22.
Part of Grant Number: ST/S003916/1
This grant is a collaboration with Jon Johnson at IoE, UCL, and RITS, UCL. The project investigates various dimensions of concept prediction: against a range of different types of unseen data from UK Data Archive's longitudinal studies, e.g. social science vs biomedical, 1995 vs 2015; and to build up an understanding of different predictions rate by category. Hierarchical approaches for topic classification against the European Language Social Science Thesaurus (ELSST) thesaurus were developed.
ESRC-funded, PI, Feb. 2021 - Feb. 2022.
Total funding amount: £81,500. Part of Grant Number: ES/K000357/1
This grant is a collaboration with Jon Johnson at the Institute of Education, UCL, London. The project investigates automated extraction of metadata from the Cohort and Longitudinal Studies Enhancement Resources (CLOSER) UK Data Archive. Automation of question extraction from paper questionnaires will form part of a pipeline to populate question banks and other metadata repositories and provide a low cost solution to the manual processes undertaken as part of the CLOSER project and UKDA (and other archives) to enhance survey metadata alongside the data description.
Science and Technology Facilities (STFC) DiRAC-funded, PI, Feb. - Dec. 2021. Part of Grant Number: ST/S003916/1.
This grant is a collaboration with Jon Johnson at IoE, UCL, and RITS, UCL. The project will utilise the questions (question text and response domains) and linked concepts (based on the European Language Social Science Thesaurus (ELSST)) held in CLOSER Discovery, the CLOSER metadata store. The aim is to create a model that will be able to classify existing questions (and predict from new questions) to these existing concepts in ELSST.
TagItSmart (2015-18) is a Smart Tags driven service platform for enabling ecosystems of connected objects that dynamically change their status in response to a variety of environmental factors and be seamlessly tracked during their lifecycle. TagItSmart is developing smart tags that combine the power of functional inks with the pervasiveness of digital and electronic markers, e.g. dynamic QR codes, NFC tags, in order to capture new contextual information. Beside this, the ubiquitous presence of smartphones with their cameras and NFC readers facilitates seamless observation measurements and lifecycle tracking of smart tag big data.
I design and develop semantic models and reasoning algorithms for capturing the characteristics of the Smart Tags and to provide decision-support mechanisms for connecting their lifecycle data to semantically-enabled workflows.
The EU H2020 frontierCities2 project (December 2016 - November 2018) is a acceleration and incubation programme that aims to accelerate market update of the FIWARE generic enablers in the Internet of Things and Smart Cities domain by targeting SMEs and startups to develop FIWARE-enabled smart mobility and smart city solutions.
I work together with the FIWARE Foundation to deliver technical coaching to the startups and lead the work-package tasked with further developing the FIWARE enablers and support mechanisms.
The iKaaS project (Oct 2015 - Oct 2017), jointly funded by the EC H2020 programme and MIC, Japan, delivers a secure, robust and scalable multi-cloud platform that brings together the paradigms of IoT, Big Data and the Cloud.
I researched aspects of data analysis in smart city platforms built on heterogeneous cloud platforms. As part of this, we developed novel anomaly detection algorithms for city environmental features (such as measured air pollution levels) and real-time detection of city social events by analysing Twitter feeds. The research delivered tools to discover correlation between large-scale city events and anomalies detected in pollution levels.
The IoT.est project (2011-14), funded by the EU FP7 Programme, developed a test-driven service creation environment for Internet of Things enabled business services. I served as the work-package leader for semantic annotation and large-scale discovery of IoT services, as well as the University of Surrey technical coordinator. I was also in-charge of the proof-of-concept project demonstrator that integrated modules from project partners.
The iCore project (2011-14), funded by the EU FP7 Programme, defined the concept of Virtual Objects (VO) to abstract the technological heterogeneity derived from the vast amounts of heterogeneous objects and devices forming part of the IoT. I researched the dynamic association derivation between ICT and real-world objects and service workflow composition in iCore.
The IoT-A project (2010-13), funded by the EU FP7 Programme, was the lighthouse EU project that defined a reference architecture and model for the IoT, including defining its constituent concepts such as entity, resource and IoT service. I was the deputy leader of the WP that researched various mechanisms for resolution framewoks for the IoT.
I worked as a PhD researcher on the Mobile VCE Core 4 Programme on Ubiquitous Services (funded by the UK Technology Strategy Board), work area: ontology-based context management for mobile systems.
Indicators of esteem
Scholarships:
- Overseas Research Student Sponsorship for PhD research: University of Surrey and MobileVCE Core 4 programme
- DFIDSSS Scholarship: jointly funded by the University of Surrey and the British Commonwealth Scholarship Commission for MSc programme.
Awards:
- Cable and Wireless Award: University of Surrey, for the best overall performance from a student graduating with an MSc in Satellite Communication Engineering or Communications Networks and Software
- IET Certificate in recognition of significant contribution to IET On Campus at the University of Surrey
Research Themes
Data analytics for Cyber-Physical and Social Streams
This research theme focusses on deriving latent patterns in CPSS data streams by developing novel ML algorithms; as well as fusion of social network data with sensor data streams. Our work looks at analysing social network data to extract real-world event information and human movements, in conjunction with sensor data and open datasets, to achieve outcomes related to quantifying their influence on smart city dynamics, such as traffic flows, measured pollution levels, redefining inner-city boundaries etc.
Related publications:
- Exploring the Effectiveness of Service Decomposition in Fog Computing Architecture for the Internet of Things, IEEE Transactions on Sustainable Computing, March 2019
- A Survey on an Emerging Area: Deep Learning for Smart City Data, IEEE Transactions on Emerging Topics in Computational Intelligence, May 2019
- Data-driven Air Quality Characterisation for Urban Environments: a Case Study, IEEE Access, December 2018
- Missing Data Estimation in Mobile Sensing Environments, IEEE Access 6(1), October 2018
- Cyber–Physical–Social Frameworks for Urban Big Data Systems: A Survey. MDPI Applied Sciences, 2017, 7 (10) (slideshare)
- Real world city event extraction from Twitter data streams, Procedia Computer Science, 2016, 98, pp. 443-448 (dataset)
Large scale sensor and data discovery and ranking
This research theme focuses on scalable, distributed discovery mechanisms supporting the sensor-as-a-service paradigm. Based on the particular localization characteristics of IoT deployments, our work has developed novel methods using geospatial indexing techniques and semantic service technologies for both sensor and sensor data discovery.
Related publications:
- Designing the Sensing as a Service Ecosystem for the Internet of Things, IEEE Internet of Things Magazine,1(2), December 2018
- Spatial Indexing for Data Searching in Mobile Sensing Environments. Sensors 2017, 17(6), 1427
- Search Techniques for the Web of Things: A Taxonomy and Survey, Sensors 2016, 16(5), 600
- An Experimental Study on Geospatial Indexing for Sensor Service Discovery, Expert Systems with Applications, Elsevier, May 2015 (ontology, dataset)
- A Ranking Method for Sensor Services based on Estimation of Service Access Cost, Information Sciences, Elsevier, October 2015 (code and dataset)
- Enabling Query of Frequently Updated Data from Mobile Sensing Sources, The 13th IEEE International Conferences on Ubiquitous Computing and Communications (IUCC2014), December 2014.
Semantic Models for the Internet of Things
This research theme focusses on semantic models for the main abstractions and concepts that underlie the IoT domain. The developed ontologies enable fine-grained semantic annotations, and to create Linked sensor data for service and data discovery.
Related publications:
- SmartTags: IoT Product Passport for Circular Economy Based on Printed Sensors and Unique Item-Level Identifiers, Sensors 2019, 19(3), 586.
- Knowledge Representation in the Internet of Things: Semantic Modelling and its Applications. Automatika – Journal for Control, Measurement, Electronics, Computing and Communications, December 2013.
- An Internet of Things Platform for Real-World and Digital Objects'. Scalable Computing: Practice and Experience, May 2012. (platform demo)
- Service modelling for the Internet of Things, IEEE Federated Conference on Computer Science and Information Systems (FEDCSIS), September 2011 (ontology)
Supervision
Completed postgraduate research projects I have supervised
Yuchao Zhou (co-supervisor): Data-driven Cyber-Physical-Social System for Knowledge Discovery in Smart Cities.
Qi Chen (external collaborative supervisor): Distributed Intelligence for Big Smart City Data Processing, Xi’an Jiaotong-Liverpool University, China.
Postgraduate research supervision
Principal PhD Supervisor for Zeqiang Wang, "Natural Language Processing for Longitudinal Social and Biomedical Science Datasets", (October 2023 - present).
External collaborative PhD supervisor for Yuqi Wang at the Xi’an Jiaotong-Liverpool University, China (October 2021 - present).
Teaching
2023-24, Spring semester:
Parallel Computing: module lead.
Computer Networks: module lead.
Professional Project (BSc final year project) and MSc dissertation: academic supervisor