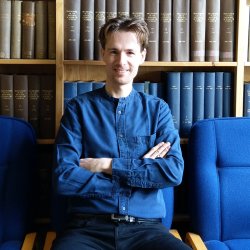
Dr Tibor Auer
About
Biography
My research interests include assessing and improving reproducibility in neuroimaging, as well as developing approaches and tools to characterise the robustness of research findings and facilitate the adoption of best practices.
I received my undergraduate degree in medicine (MD) and my PhD in clinical neuroscience from the University of Pécs, Hungary, where I implemented a broad spectrum of in vivo MR techniques in a clinical environment. Then, I joined the methods-oriented Biomedizinische NMR Forschungs GmbH in Max-Planck Institute for Biophysical Chemistry in Göttingen, studied the assumptions and mechanisms underlying neurofeedback training (NFT), a non-invasive intervention, and optimised the experimental setup and protocol. At the MRC Cognition and Brain Sciences Unit in Cambridge and the Royal Holloway University of London in Egham, I led the development of Automatic Analysis, a reproducible and scalable neuroimaging analysis pipeline, and contributed to international harmonisation initiatives, including the Brain Imaging Data Structure and the Neuroimaging Data Model. Combining my commitment to clinically applicable research and my keen interest in powerful and reliable methods, I joined the University of Surrey, School of Psychology to investigate neurocognitive changes during healthy ageing and assess and optimise electric and neurofeedback-based neuromodulation.
Areas of specialism
Affiliations and memberships
News
In the media
ResearchResearch interests
Reproducibility and methodological variation
I am aware of the huge gap between the volume of neuroimaging findings and their translation into mental health research and practice, a gap that can be partially attributed to the lack of reproducibility and confidence in the findings. My primary research interest is to assess and mitigate the effect of methodological variation in neuroimaging to improve its translation potential.
Methods development and Open Science
I am enthusiastic about understanding upcoming methodological improvements in data acquisition, processing, and analysis, to combine and develop new approaches. I lead the international group developing Automatic Analysis, a Matlab framework to process multimodal neuroimaging analysis pipelines and co-develop OpenNFT, a Python/Matlab framework for real-time fMRI neurofeedback. I am also a strong supporter of the harmonisation of neuroimaging approaches, and I am involved in international initiatives such as the Brain Imaging Data Structure and Neuroimaging Data Model providing a standard for organising neuroimaging data and analysis.
Neuromodulation
I am interested in optimising the experimental setup and understanding the assumptions and mechanisms underlying non-invasive neuromodulation, including fMRI-based neurofeedback training (NFT) and transcranial alternating current stimulation (tACS). I combine cutting-edge neuroimaging methods, including EEG, fMRI and machine learning, to understand neural signals and identify them as candidate neuromarkers for personalising neuromodulation.
Research interests
Reproducibility and methodological variation
I am aware of the huge gap between the volume of neuroimaging findings and their translation into mental health research and practice, a gap that can be partially attributed to the lack of reproducibility and confidence in the findings. My primary research interest is to assess and mitigate the effect of methodological variation in neuroimaging to improve its translation potential.
Methods development and Open Science
I am enthusiastic about understanding upcoming methodological improvements in data acquisition, processing, and analysis, to combine and develop new approaches. I lead the international group developing Automatic Analysis, a Matlab framework to process multimodal neuroimaging analysis pipelines and co-develop OpenNFT, a Python/Matlab framework for real-time fMRI neurofeedback. I am also a strong supporter of the harmonisation of neuroimaging approaches, and I am involved in international initiatives such as the Brain Imaging Data Structure and Neuroimaging Data Model providing a standard for organising neuroimaging data and analysis.
Neuromodulation
I am interested in optimising the experimental setup and understanding the assumptions and mechanisms underlying non-invasive neuromodulation, including fMRI-based neurofeedback training (NFT) and transcranial alternating current stimulation (tACS). I combine cutting-edge neuroimaging methods, including EEG, fMRI and machine learning, to understand neural signals and identify them as candidate neuromarkers for personalising neuromodulation.
Publications
Highlights
Clinical and Cognitive Neuroscience
- Auer, T., et al. (2008). Identifying seizure-onset zone and visualizing seizure spread by fMRI: a case report. Epileptic Disord.
One of my most challenging PhD projects was the analysis of an fMRI capturing an epileptic seizure. I combined neurological considerations and adaptive data exploration and analytics and implemented a “travelling wave” approach to identify seizure onset and visualise seizure spread intuitively.
- Auer, T., et al. (2009). Does obstetric brachial plexus injury influence speech dominance? Annals of Neurology.
I investigated the causality between handedness and language lateralisation confronting the dominant theory that handedness is secondary. Acquiring fMRI of a vulnerable sample, such as children with obstetric brachial plexus injury, and using a correlation technique, I demonstrated that hand usage could influence language lateralisation.
- Auer, T., Schweizer, R., & Frahm, J. (2015). Training Efficiency and Transfer Success in an Extended Real-Time Functional MRI Neurofeedback Training of the Somatomotor Cortex of Healthy Subjects. Front Hum Neurosci
My first paper on neurofeedback training (NFT) identified and defined training efficiency and transfer success as two specific performance metrics. Moreover, I found training efficiency highly correlated to and predictive of transfer success; therefore, I could provide some practical guidance for NFTs.
- Auer, T., et al. (2018). Higher-order Brain Areas Associated with Real-time Functional MRI Neurofeedback Training of the Somato-motor Cortex. Neuroscience
Investigating the functional connectivity during NFT, I emphasised the role of cognitive control and skill learning rather than conditioning as the main mechanisms underlying fMRI-based NFT. I also identified the anterior midcingulate cortex (aMCC) as the “mediator” of cognitive control in NFT.
- Auer, T., Lorenz, R., Violante, I. (2022). Auer, Tibor; Lorenz, Romy; Violante, Ines (2022): Decoding frequency-specific modulation of fMRI network connectivity: a tACS study. Figshare.
I employed uni- and multivariate analyses and demonstrated that tACS has differentiable effects on both the magnitude of the fMRI activity and the effective connectivity. I also proved that effective connectivity in the dorsal attention and control networks is the most robust in decoding the stimulation frequency.
Neuroinformatics
- Cusack R., Vicente-Grabovetsky A., Mitchell D. J., Wild C. J., Auer T., et al. (2015) Automatic analysis (aa): Efficient neuroimaging workflows and parallel processing using Matlab and XML. Frontiers in Neuroinformatics
I took over the coordination of aa’s development in 2014. I improved its versatility and robustness by implementing parallel processing and integrating foundational tools for functional connectivity, multivariate analysis, diffusion tensor and kurtosis imaging, and M/EEG. I have redesigned the results reporting with quality assurance and implemented provenance capturing.
- Taylor, J. R., Williams, N., Cusack, R., Auer, T., et al. (2017). The Cambridge Centre for Ageing and Neuroscience (Cam-CAN) data repository: Structural and functional MRI, MEG, and cognitive data from a cross-sectional adult lifespan sample. Neuroimage
I employed and developed Automatic Analysis (aa) as the central processing pipeline for the Cambridge Centre for Ageing and Neuroscience (CamCAN) project, which demonstrated aa’s efficiency. As an outcome, I provided data, both raw and preprocessed according to the best practices, thus triggering and supporting more than a hundred studies.
- Gorgolewski, K. J., Auer, T., et al. (2016). The brain imaging data structure, a format for organizing and describing outputs of neuroimaging experiments. Scientific Data
I faced several informatics issues and challenges while working on the CamCAN project. Therefore, I joined the International Neuroinformatics Coordinating Facility (INCF) Neuroimaging Data Sharing Task Force. In collaboration with the Center for Reproducible Neuroscience, I worked on the BIDS standard, which became the gold standard for neuroimaging data management.
- Maumet, C., Auer, T., et al. (2016). Sharing brain mapping statistical results with the neuroimaging data model. Scientific Data
I joined the initiative to harmonise the description of the results and the provenance of neuroimaging analyses. I contributed to the data model by defining several terms and their semantic relationships. The major foundation tools, including SPM, FSL, and AFNI, have adopted the data model.
- Karakuzu, A., Appelhoff, S., Auer, T., et al. (2022). qMRI-BIDS: An extension to the brain imaging data structure for quantitative magnetic resonance imaging data. Scientific Data
I contributed to the extension of BIDS to provide clear guidance on how to store and share novel quantitative imaging data and tools.
This paper describes the data repository for the Cambridge Centre for Ageing and Neuroscience (Cam-CAN) initial study cohort. The Cam-CAN Stage 2 repository contains multi-modal (MRI, MEG, and cognitive-behavioural) data from a large (approximately N = 700), cross-sectional adult lifespan (18–87 years old) population-based sample. The study is designed to characterise age-related changes in cognition and brain structure and function, and to uncover the neurocognitive mechanisms that support healthy cognitive ageing. The database contains raw and preprocessed structural MRI, functional MRI (active tasks and resting state), and MEG data (active tasks and resting state), as well as derived scores from cognitive behavioural experiments spanning five broad domains (attention, emotion, action, language, and memory), and demographic and neuropsychological data. The dataset thus provides a depth of neurocognitive phenotyping that is currently unparalleled, enabling integrative analyses of age-related changes in brain structure, brain function, and cognition, and providing a testbed for novel analyses of multi-modal neuroimaging data.
Transcranial electrical stimulation (tES) can be used in combination with functional MRI (fMRI) to study brain functions. This Consensus Statement provides a checklist for reporting concurrent tES-fMRI research. Low-intensity transcranial electrical stimulation (tES), including alternating or direct current stimulation, applies weak electrical stimulation to modulate the activity of brain circuits. Integration of tES with concurrent functional MRI (fMRI) allows for the mapping of neural activity during neuromodulation, supporting causal studies of both brain function and tES effects. Methodological aspects of tES-fMRI studies underpin the results, and reporting them in appropriate detail is required for reproducibility and interpretability. Despite the growing number of published reports, there are no consensus-based checklists for disclosing methodological details of concurrent tES-fMRI studies. The objective of this work was to develop a consensus-based checklist of reporting standards for concurrent tES-fMRI studies to support methodological rigor, transparency and reproducibility (ContES checklist). A two-phase Delphi consensus process was conducted by a steering committee (SC) of 13 members and 49 expert panelists through the International Network of the tES-fMRI Consortium. The process began with a circulation of a preliminary checklist of essential items and additional recommendations, developed by the SC on the basis of a systematic review of 57 concurrent tES-fMRI studies. Contributors were then invited to suggest revisions or additions to the initial checklist. After the revision phase, contributors rated the importance of the 17 essential items and 42 additional recommendations in the final checklist. The state of methodological transparency within the 57 reviewed concurrent tES-fMRI studies was then assessed by using the checklist. Experts refined the checklist through the revision and rating phases, leading to a checklist with three categories of essential items and additional recommendations: (i) technological factors, (ii) safety and noise tests and (iii) methodological factors. The level of reporting of checklist items varied among the 57 concurrent tES-fMRI papers, ranging from 24% to 76%. On average, 53% of checklist items were reported in a given article. In conclusion, use of the ContES checklist is expected to enhance the methodological reporting quality of future concurrent tES-fMRI studies and increase methodological transparency and reproducibility.
This study investigated the level of self-regulation of the somatomotor cortices (SMCs) attained by an extended functional magnetic resonance imaging (fMRI) neurofeedback training. Sixteen healthy subjects performed 12 real-time functional magnetic resonance imaging neurofeedback training sessions within 4 weeks, involving motor imagery of the dominant right as well as the non-dominant left hand. Target regions of interests in the SMC were individually localized prior to the training by overt finger movements. The feedback signal (FS) was defined as the difference between fMRI activation in the contra-and ipsilateral SMC and visually presented to the subjects. Training efficiency was determined by an off-line general linear model analysis determining the fMRI percent signal changes in the SMC target areas accomplished during the neurofeedback training. Transfer success was assessed by comparing the pre- and post-training transfer task, i.e., the neurofeedback paradigm without the presentation of the FS. Group results show a distinct increase in feedback performance (FP) in the transfer task for the trained group compared to a matched untrained control group, as well as an increase in the time course of the training, indicating an efficient training and a successful transfer. Individual analysis revealed that the training efficiency was not only highly correlated to the transfer success but also predictive. Trainings with at least 12 efficient training runs were associated with a successful transfer outcome. A group analysis of the hemispheric contributions to the FP showed that it is mainly driven by increased fMRI activation in the contralateral SMC, although some individuals relied on ipsilateral deactivation. Training and transfer results showed no difference between left-and right-hand imagery, with a slight indication of more ipsilateral deactivation in the early right-hand trainings.
The Brain Imaging Data Structure (BIDS) established community consensus on the organization of data and metadata for several neuroimaging modalities. Traditionally, BIDS had a strong focus on functional magnetic resonance imaging (MRI) datasets and lacked guidance on how to store multimodal structural MRI datasets. Here, we present and describe the BIDS Extension Proposal 001 (BEP001), which adds a range of quantitative MRI (qMRI) applications to the BIDS. In general, the aim of qMRI is to characterize brain microstructure by quantifying the physical MR parameters of the tissue via computational, biophysical models. By proposing this new standard, we envision standardization of qMRI through multicenter dissemination of interoperable datasets. This way, BIDS can act as a catalyst of convergence between qMRI methods development and application-driven neuroimaging studies that can help develop quantitative biomarkers for neural tissue characterization. In conclusion, this BIDS extension offers a common ground for developers to exchange novel imaging data and tools, reducing the entrance barrier for qMRI in the field of neuroimaging.
Neurofeedback training has been shown to influence behavior in healthy participants as well as to alleviate clinical symptoms in neurological, psychosomatic, and psychiatric patient populations. However, many real-time fMRI neurofeedback studies report large inter-individual differences in learning success. The factors that cause this vast variability between participants remain unknown and their identification could enhance treatment success. Thus, here we employed a meta-analytic approach including data from 24 different neurofeedback studies with a total of 401 participants, including 140 patients, to determine whether levels of activity in target brain regions during pretraining functional localizer or no-feedback runs (i.e., self-regulation in the absence of neurofeedback) could predict neurofeedback learning success. We observed a slightly positive correlation between pretraining activity levels during a functional localizer run and neurofeedback learning success, but we were not able to identify common brain-based success predictors across our diverse cohort of studies. Therefore, advances need to be made in finding robust models and measures of general neurofeedback learning, and in increasing the current study database to allow for investigating further factors that might influence neurofeedback learning.
Neurofeedback (NFB) allows subjects to learn self-regulation of neuronal brain activation based on information about the ongoing activation. The implementation of real-time functional magnetic resonance imaging (rt-fMRI) for NFB training now facilitates the investigation into underlying processes. Our study involved 16 control and 16 training right-handed subjects, the latter performing an extensive rt-fMRI NFB training using motor imagery. A previous analysis focused on the targeted primary somato-motor cortex (SMC). The present study extends the analysis to the supplementary motor area (SMA), the next higher brain area within the hierarchy of the motor system. We also examined transfer-related functional connectivity using a whole-volume psycho-physiological interaction (PPI) analysis to reveal brain areas associated with learning. The ROI analysis of the pre- and post-training fMRI data for motor imagery without NFB (transfer) resulted in a significant training-specific increase in the SMA. It could also be shown that the contralateral SMA exhibited a larger increase than the ipsilateral SMA in the training and the transfer runs, and that the right-hand training elicited a larger increase in the transfer runs than the left-hand training. The PPI analysis revealed a training-specific increase in transfer-related functional connectivity between the left SMA and frontal areas as well as the anterior midcingulate cortex (aMCC) for right- and left-hand trainings. Moreover, the transfer success was related with training-specific increase in functional connectivity between the left SMA and the target area SMC. Our study demonstrates that NFB training increases functional connectivity with non-targeted brain areas. These are associated with the training strategy (i.e., SMA) as well as with learning the NFB skill (i.e., aMCC and frontal areas). This detailed description of both the system to be trained and the areas involved in learning can provide valuable information for further optimization of NFB trainings.
The development of magnetic resonance imaging (MRI) techniques has defined modern neuroimaging. Since its inception, tens of thousands of studies using techniques such as functional MRI and diffusion weighted imaging have allowed for the non-invasive study of the brain. Despite the fact that MRI is routinely used to obtain data for neuroscience research, there has been no widely adopted standard for organizing and describing the data collected in an imaging experiment. This renders sharing and reusing data (within or between labs) difficult if not impossible and unnecessarily complicates the application of automatic pipelines and quality assurance protocols. To solve this problem, we have developed the Brain Imaging Data Structure (BIDS), a standard for organizing and describing MRI datasets. The BIDS standard uses file formats compatible with existing software, unifies the majority of practices already common in the field, and captures the metadata necessary for most common data processing operations.
Only a tiny fraction of the data and metadata produced by an fMRI study is finally conveyed to the community. This lack of transparency not only hinders the reproducibility of neuroimaging results but also impairs future meta-analyses. In this work we introduce NIDM-Results, a format specification providing a machine-readable description of neuroimaging statistical results along with key image data summarising the experiment. NIDM-Results provides a unified representation of mass univariate analyses including a level of detail consistent with available best practices. This standardized representation allows authors to relay methods and results in a platform-independent regularized format that is not tied to a particular neuroimaging software package. Tools are available to export NIDM-Result graphs and associated files from the widely used SPM and FSL software packages, and the NeuroVault repository can import NIDM-Results archives. The specification is publically available at: nidm.nidash.org/specs/nidm-results.html.
Aim. To visualize by ictal, functional MRI, the initial haemodynamic change (i.e. putative seizure-onset zone) and subsequent seizure spread during an epileptic seizure. Methods. A 20-year-old woman was investigated during a simple partial seizure consisting of right-sided mouth clonus. An internal reference curve, correlated with signal change pixelwise, was applied to obtain correlation coefficient maps. The reference curve was shifted scan by scan to examine the correlation at each time point. To demonstrate seizure onset and propagation, a lag time map was produced showing the temporal sequence of activation in various brain regions. Results. fMRI analysis showed that the lower part of the insular cortex was activated first, and its signal alteration preceded the clinical beginning of the seizure (i.e. mouth clonus) by more than one minute. Most of the activations started before clinical seizure onset. The activation corresponding to the motor area of the right face showed only a 7.5 second-long, pre-ictal phase. BOLD signal alterations were also observed in the left caudate nucleus, left thalamus, along with various areas of the left cerebral and cerebellum hemispheres. Conclusions. The present study demonstrates a whole-brain activity simultaneously in time and space, during an epileptic seizure. Our results also support the existence of the pre-ictal state in epilepsy. Replication of our results would be of major interest for presurgical evaluation of patients with drug-resistant epilepsy.
Objective Right-handedness and left-sided language lateralization is an unresolved mystery with unknown cause/effect relations. Most studies suggest that the language lateralization is related to a fundamental brain asymmetry: right-handedness may be secondary. We analyzed the possibility of an opposite cause/effect relation: whether asymmetric hand usage (as a cause) can influence language lateralization (as a consequence). Methods We determined language lateralization by functional magnetic resonance imaging in 15 subjects whose upper limb (UL) had been injured at birth because of unilateral damage of the brachial plexus. These subjects were able to use only one (the noninjured) UL perfectly. Results We found correlation between the severity of right-sided UL injuries and hand usage dysfunction and the degree of left-to-right shift of language lateralization. There was, however, not a complete switch of language lateralization. Interpretation Right-sided UL injury can induce a left-to-right shift in language lateralization, suggesting that hand usage can influence language lateralization. These findings may contradict the broadly accepted theory that right-handedness is a secondary phenomenon caused by left-sided hemispheric language lateralization. However, the cause/effect problem between asymmetric hand usage and language lateralization is not resolved in this study. Our findings may support the theory that gestures had a crucial role in human language evolution and is a part of the language system even today.
Automatic Analysis is a pipeline system for neuroimaging written primarily in MATLAB. It supports SPM 12, as well as selected functions from other software packages (e.g., FSL, Freesurfer). The goal is to facilitate automatic, flexible, and replicable neuroimaging analyses through a comprehensive pipeline system.
Real-time fMRI neurofeedback is an increasingly popular neuroimaging technique that allows an individual to gain control over his/her own brain signals, which can lead to improvements in behavior in healthy participants as well as to improvements of clinical symptoms in patient populations. However, a considerably large ratio of participants undergoing neurofeedback training do not learn to control their own brain signals and, consequently, do not benefit from neurofeedback interventions, which limits clinical efficacy of neurofeedback interventions. As neurofeedback success varies between studies and participants, it is important to identify factors that might influence neurofeedback success. Here, for the first time, we employed a big data machine learning approach to investigate the influence of 20 different design-specific (e.g. activity vs. connectivity feedback), region of interest specific (e.g. cortical vs. subcortical) and subject-specific factors (e.g. age) on neurofeedback performance and improvement in 608 participants from 28 independent experiments. With a classification accuracy of 60% (considerably different from chance level), we identified two factors that significantly influenced neurofeedback performance: Both the inclusion of a pre-training no-feedback run before neurofeedback training and neurofeedback training of patients as compared to healthy participants were associated with better neurofeedback performance. The positive effect of pre-training no-feedback runs on neurofeedback performance might be due to the familiarization of participants with the neurofeedback setup and the mental imagery task before neurofeedback training runs. Better performance of patients as compared to healthy participants might be driven by higher motivation of patients, higher ranges for the regulation of dysfunctional brain signals, or a more extensive piloting of clinical experimental paradigms. Due to the large heterogeneity of our dataset, these findings likely generalize across neurofeedback studies, thus providing guidance for designing more efficient neurofeedback studies specifically for improving clinical neurofeedback-based interventions. To facilitate the development of data-driven recommendations for specific design details and subpopulations the field would benefit from stronger engagement in open science research practices and data sharing.