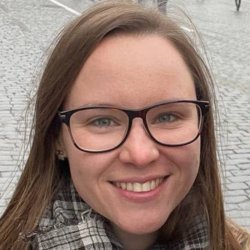
Dr Yulia Gryaditskaya
Academic and research departments
Centre for Vision, Speech and Signal Processing (CVSSP), Surrey Institute for People-Centred Artificial Intelligence (PAI).About
Biography
Dr. Yulia Gryaditskaya is a Lecturer in Artificial Intelligence at Surrey Institute for People-Centred AI and CVSSP, UK. Prior to that, she was a Senior Research Fellow (2020-2022) in Computer Vision and Machine Learning at the Centre for Vision Speech and Signal Processing (CVSSP), in the SketchX group, led by Prof. Yi-Zhe Song.
Before joining CVSSP, she was a postdoctoral researcher (2017-2020) at Inria, GraphDeco, under the guidance of Dr. Adrien Bousseau. She had the opportunity to visit MIT and collaborate with Prof. Fredo Durand, MIT, CSAIL, and Prof. Alla Sheffer, UBC, British Columbia. She received her PhD (2012-2016) from MPI Informatik, Germany, advised by Prof. Karol Myszkowski and Prof. Hans-Peter Seidel.
While working on her PhD (2014), she did a research internship in the Color and HDR group in Technicolor R&D, Rennes, France, under the guidance of Dr. Erik Reinhard. She received a degree (2007-2012) in Applied Mathematics and Computer Science with a specialization in Operation Research and System Analysis from Lomonosov Moscow State University, Russia.
News
In the media
ResearchResearch interests
Yulia explores how AI can be used to assists people through the creative process, increasing people's creativity and productivity. Her main research interests are in sketching and 3D modeling. She works on the tasks of sketch understanding, sketch and 3D shapes analysis and generation.
Research interests
Yulia explores how AI can be used to assists people through the creative process, increasing people's creativity and productivity. Her main research interests are in sketching and 3D modeling. She works on the tasks of sketch understanding, sketch and 3D shapes analysis and generation.
Teaching
Undergraduate:
- EEE2041 Computer Vision and Graphics, Department of Electrical and Electronic Engineering, University of Surrey
Masters:
- EEEM067 AR, VR and the Metaverse, Department of Electrical and Electronic Engineering, University of Surrey
Publications
This dataset contains training, validation and test data used in the paper: "Open Vocabulary Scene Sketch Semantic Understanding" by Ahmed Bourouis, Judith Ellen Fan, Yulia Gryaditskaya, CVPR, 2024.It contains our split of the sketches from the FSCOCO dataset into training, validation and test sets. For the validation and test sets, we provide stroke-level annotations into different categories, as shown in the images above. The details are provided below.
We conduct a detailed study of the ability of pretrained on pretext tasks ViT and ResNet feature layers to quantify the similarity between pairs of 2D sketch views of individual 3D shapes. We assess the performance in terms of the models' abilities to retrieve similar views and ground-truth 3D shapes. Going beyond naive zero-shot performance study, we investigate alternative fine-tuning strategies on one or several shape classes, and their generalization to other shape classes. Leveraging progress in NPR (Non-Photo Realistic) rendering, we generate synthetic sketch views in several styles which we use to fine-tune pretrained foundation models using contrastive learning. We study how the scale of an object in a sketch affects the similarity of features at different network layers. We observe that depending on the scale, different feature layers can be more indicative of shape similarities in sketch views. However, we find that similar object scales result in the best performance of ViT and ResNet. In summary, we show that careful selection of a fine-tuning strategy allows us to obtain consistent improvement in zero-shot shape retrieval accuracy. We believe that our work will have a significant impact on research in the sketch domain, providing insights and guidance on how to adopt large pretrained models as perceptual losses.
Designing real and virtual garments is becoming extremely demanding with rapidly changing fashion trends and increasing need for synthesizing realistically dressed digital humans for various applications. However, traditionally designing real and virtual garments has been time-consuming. Sketch based modeling aims to bring the ease and immediacy of drawing to the 3D world thereby motivating faster iterations. We propose a novel sketch-based garment modeling framework that is specifically targeted to synchronize with the iterative process of garment ideation, e.g., adding or removing details from different views in each iteration. At the core of our learning based approach is a view-aware feature aggregation module that fuses the features from the latest sketch with the thus far aggregated features to effective refine the generated 3D shape. We evaluate our approach on a wide variety of garment types and iterative refinement scenarios. We also provide comparisons to alternative feature aggregation methods and demonstrate favorable results. The code is available at https://github.com/pinakinathc/multiviewsketch-garment.
We present the first competitive drawing agent Pixelor that exhibits human-level performance at a Pictionary-like sketching game, where the participant whose sketch is recognized first is a winner. Our AI agent can autonomously sketch a given visual concept, and achieve a recognizable rendition as quickly or faster than a human competitor. The key to victory for the agent’s goal is to learn the optimal stroke sequencing strategies that generate the most recognizable and distinguishable strokes first. Training Pixelor is done in two steps. First, we infer the stroke order that maximizes early recognizability of human training sketches. Second, this order is used to supervise the training of a sequence-to-sequence stroke generator. Our key technical contributions are a tractable search of the exponential space of orderings using neural sorting; and an improved Seq2Seq Wasserstein (S2S-WAE) generator that uses an optimal-transport loss to accommodate the multi-modal nature of the optimal stroke distribution. Our analysis shows that Pixelor is better than the human players of the Quick, Draw! game, under both AI and human judging of early recognition. To analyze the impact of human competitors’ strategies, we conducted a further human study with participants being given unlimited thinking time and training in early recognizability by feedback from an AI judge. The study shows that humans do gradually improve their strategies with training, but overall Pixelor still matches human performance. The code and the dataset are available at http://sketchx.ai/pixelor.
We present the first one-shot personalized sketch segmentation method. We aim to segment all sketches belonging to the same category provisioned with a single sketch with a given part annotation while (i) preserving the parts semantics embedded in the exemplar, and (ii) being robust to input style and abstraction. We refer to this scenario as personalized . With that, we importantly enable a much-desired personalization capability for downstream fine-grained sketch analysis tasks. To train a robust segmentation module, we deform the exemplar sketch to each of the available sketches of the same category. Our method generalizes to sketches not observed during training. Our central contribution is a sketch-specific hierarchical deformation network. Given a multi-level sketch-strokes encoding obtained via a graph convolutional network, our method estimates rigid-body transformation from the target to the exemplar, on the upper level. Finer deformation from the exemplar to the globally warped target sketch is further obtained through stroke-wise deformations, on the lower-level. Both levels of deformation are guided by mean squared distances between the keypoints learned without supervision, ensuring that the stroke semantics are preserved. We evaluate our method against the state-of-the-art segmentation and perceptual grouping baselines re-purposed for the one-shot setting and against two few-shot 3D shape segmentation methods. We show that our method outperforms all the alternatives by more than 10% on average. Ablation studies further demonstrate that our method is robust to personalization : changes in input part semantics and style differences.
We present the first algorithm capable of automatically lifting real-world, vector-format, industrial design sketches into 3D. Targeting real-world sketches raises numerous challenges due to inaccuracies, use of overdrawn strokes, and construction lines. In particular, while construction lines convey important 3D information, they add significant clutter and introduce multiple accidental 2D intersections. Our algorithm exploits the geometric cues provided by the construction lines and lifts them to 3D by computing their intended 3D intersections and depths. Once lifted to 3D, these lines provide valuable geometric constraints that we leverage to infer the 3D shape of other artist drawn strokes. The core challenge we address is inferring the 3D connectivity of construction and other lines from their 2D projections by separating 2D intersections into 3D intersections and accidental occlusions. We efficiently address this complex combinatorial problem using a dedicated search algorithm that leverages observations about designer drawing preferences , and uses those to explore only the most likely solutions of the 3D intersection detection problem. We demonstrate that our separator outputs are of comparable quality to human annotations, and that the 3D structures we recover enable a range of design editing and visualization applications, including novel view synthesis and 3D-aware scaling of the depicted shape.
We advance sketch research to scenes with the first dataset of freehand scene sketches, FS-COCO. With practical applications in mind, we collect sketches that convey scene content well but can be sketched within a few minutes by a person with any sketching skills. Our dataset comprises 10,000 freehand scene vector sketches with per point space-time information by 100 non-expert individuals, offering both object- and scene-level abstraction. Each sketch is augmented with its text description. Using our dataset, we study for the first time the problem of fine-grained image retrieval from freehand scene sketches and sketch captions. We draw insights on: (i) Scene salience encoded in sketches using the strokes temporal order; (ii) Performance comparison of image retrieval from a scene sketch and an image caption; (iii) Complementarity of information in sketches and image captions, as well as the potential benefit of combining the two modalities. In addition, we extend a popular vector sketch LSTM-based encoder to handle sketches with larger complexity than was supported by previous work. Namely, we propose a hierarchical sketch decoder, which we leverage at a sketch-specific ``pretext" task. Our dataset enables for the first time research on freehand scene sketch understanding and its practical applications. We release the dataset under CC BY-NC 4.0 license: https://fscoco.github.io
3D shape modeling is labor-intensive and time-consuming and requires years of expertise. Recently, 2D sketches and text inputs were considered as conditional modalities to 3D shape generation networks to facilitate 3D shape modeling. However, text does not contain enough fine-grained information and is more suitable to describe a category or appearance rather than geometry, while 2D sketches are ambiguous, and depicting complex 3D shapes in 2D again requires extensive practice. Instead, we explore virtual reality sketches that are drawn directly in 3D. We assume that the sketches are created by novices, without any art training, and aim to reconstruct physically-plausible 3D shapes. Since such sketches are potentially ambiguous, we tackle the problem of the generation of multiple 3D shapes that follow the input sketch structure. Limited in the size of the training data, we carefully design our method, training the model step-by-step and leveraging multi-modal 3D shape representation. To guarantee the plausibility of generated 3D shapes we leverage the normalizing flow that models the distribution of the latent space of 3D shapes. To encourage the fidelity of the generated 3D models to an input sketch, we propose a dedicated loss that we deploy at different stages of the training process. We plan to make our code publicly available.
Deep image-based modeling received lots of attention in recent years, yet the parallel problem of sketch-based modeling has only been briefly studied, often as a potential application. In this work, for the first time, we identify the main differences between sketch and image inputs: (i) style variance, (ii) imprecise perspective, and (iii) sparsity. We discuss why each of these differences can pose a challenge, and even make a certain class of image-based methods inapplicable. We study alternative solutions to address each of the difference. By doing so, we drive out a few important insights: (i) sparsity commonly results in an incorrect prediction of foreground versus background, (ii) diversity of human styles, if not taken into account, can lead to very poor generalization properties, and finally (iii) unless a dedicated sketching interface is used, one can not expect sketches to match a perspective of a fixed viewpoint. Finally, we compare a set of representative deep single-image modeling solutions and show how their performance can be improved to tackle sketch input by taking into consideration the identified critical differences.
We present the first competitive drawing agent Pixelor that exhibits human-level performance at a Pictionary-like sketching game, where the participant whose sketch is recognized first is a winner. Our AI agent can autonomously sketch a given visual concept, and achieve a recognizable rendition as quickly or faster than a human competitor. The key to victory for the agent’s goal is to learn the optimal stroke sequencing strategies that generate the most recognizable and distinguishable strokes first. Training Pixelor is done in two steps. First, we infer the stroke order that maximizes early recognizability of human training sketches. Second, this order is used to supervise the training of a sequence-to-sequence stroke generator. Our key technical contributions are a tractable search of the exponential space of orderings using neural sorting; and an improved Seq2Seq Wasserstein (S2S-WAE) generator that uses an optimal-transport loss to accommodate the multi-modal nature of the optimal stroke distribution. Our analysis shows that Pixelor is better than the human players of the Quick, Draw! game, under both AI and human judging of early recognition. To analyze the impact of human competitors’ strategies, we conducted a further human study with participants being given unlimited thinking time and training in early recognizability by feedback from an AI judge. The study shows that humans do gradually improve their strategies with training, but overall Pixelor still matches human performance. The code and the dataset are available at http://sketchx.ai/pixelor.
Deep image-based modeling has received a lot of attention in recent years. Sketch-based modeling in particular has gained popularity given the ubiquitous nature of touchscreen devices. In this paper, we (i) study and compare diverse single-image reconstruction methods on sketch input, comparing the different 3D shape representations: multi-view, voxel- and point-cloud-based, mesh-based and implicit ones; and (ii) analyze the main challenges and requirements of sketch-based modeling systems. We introduce the regression loss and provide two variants of its formulation for the two most promising 3D shape representations: point clouds and signed distance functions. We show that this loss can increase general reconstruction accuracy, and the view- and style-robustness of the reconstruction methods. Moreover, we demonstrate that this loss can benefit the disentanglement of latent space to view invariant and view-specific information, resulting in further improved performance. To address the figure-ground ambiguity typical for sparse freehand sketches, we propose a two-branch architecture that exploits sparse user labeling. We hope that our work will inform future research on sketch-based modeling.
In this paper we study, for the first time, the problem of fine-grained sketch-based 3D shape retrieval. We advocate the use of sketches as a fine-grained input modality to retrieve 3D shapes at instance-level - e.g., given a sketch of a chair, we set out to retrieve a specific chair from a gallery of all chairs. Fine-grained sketch-based 3D shape retrieval (FG-SBSR) has not been possible till now due to a lack of datasets that exhibit one-to-one sketch-3D correspondences. The first key contribution of this paper is two new datasets, consisting a total of 4,680 sketch-3D pairings from two object categories. Even with the datasets, FG-SBSR is still highly challenging because (i) the inherent domain gap between 2D sketch and 3D shape is large, and (ii) retrieval needs to be conducted at the instance level instead of the coarse category level matching as in traditional SBSR. Thus, the second contribution of the paper is the first cross-modal deep embedding model for FG-SBSR, which specifically tackles the unique challenges presented by this new problem. Core to the deep embedding model is a novel cross-modal view attention module which automatically computes the optimal combination of 2D projections of a 3D shape given a query sketch.
Recently, encoders like ViT (vision transformer) and ResNet have been trained on vast datasets and utilized as perceptual metrics for comparing sketches and images, as well as multi-domain encoders in a zero-shot setting. However, there has been limited effort to quantify the granularity of these encoders. Our work addresses this gap by focusing on multi-modal 2D projections of individual 3D instances. This task holds crucial implications for retrieval and sketch-based modeling. We show that in a zero-shot setting (without retraining on a specific shape category or sketch style), the more abstract the sketch, the higher the likelihood of incorrect image matches. Even within the same sketch domain, sketches of the same object drawn in different styles, for example by distinct individuals, might not be accurately matched. One of the key findings of our research is that meticulous fine-tuning on one class of 3D shapes leads to improved performance on other shape classes (fine-tuned but zero-shot), reaching or surpassing the accuracy of supervised methods. We compare and discuss several fine-tuning strategies. Additionally, we delve deeply into how the scale of an object in a sketch influences the similarity of features at different network layers, helping us identify which network layers provide the most accurate matching. Significantly, we discover that ViT and ResNet perform best when dealing with similar object scales. We believe that our work will have a significant impact on research in the sketch domain, providing insights and guidance on how to adopt large pretrained models as perceptual losses.
3D shape modeling is labor-intensive, time-consuming, and requires years of expertise. To facilitate 3D shape modeling, we propose a 3D shape generation network that takes a 3D VR sketch as a condition. We assume that sketches are created by novices without art training and aim to reconstruct geometrically realistic 3D shapes of a given category. To handle potential sketch ambiguity, our method creates multiple 3D shapes that align with the original sketch’s structure. We carefully design our method, training the model step-by-step and leveraging multi-modal 3D shape representation to support training with limited training data. To guarantee the realism of generated 3D shapes we leverage the normalizing flow that models the distribution of the latent space of 3D shapes. To encourage the fidelity of the generated 3D shapes to an input sketch, we propose a dedicated loss that we deploy at different stages of the training process. The code is available at https://github.com/Rowl1ng/3Dsketch2shape.
With the improvement of both acquisition techniques, and computational and storage capabilities, we are witnessing an increasing presence of multidimensional scene representations. Two-dimensional, conventional images are gradually losing their hegemony, leaving room for novel formats. Among these, light fields are gaining importance, further propelled by the recent reappearance of virtual reality. Content generation is one of the stumbling blocks in this realm, and light fields are one of the main input sources of content. As their use becomes more common, a key challenge is the ability to edit or modify the appearance of the objects in the light field. This paper presents a method for manipulating the appearance of gloss in light fields. In particular, we propose a multidimensional filtering approach in which the specular highlights are filtered in the spatial and angular domains to target a desired increase of the material roughness. The filtering kernel is computed based on surface normals and view direction. Our technique generates angularly-coherent plausible edits in both synthetic and captured light fields.
We study the practical task of fine-grained 3D-VRsketch-based 3D shape retrieval. This task is of particular interest as 2D sketches were shown to be effective queries for 2D images. However, due to the domain gap, it remains hard to achieve strong performance in 3D shape retrieval from 2D sketches. Recent work demonstrated the advantage of 3D VR sketching on this task. In our work, we focus on the challenge caused by inherent inaccuracies in 3D VR sketches. We observe that retrieval results obtained with a triplet loss with a fixed margin value, commonly used for retrieval tasks, contain many irrelevant shapes and often just one or few with a similar structure to the query. To mitigate this problem, we for the first time draw a connection between adaptive margin values and shape similarities. In particular, we propose to use a triplet loss with an adaptive margin value driven by a ‘fitting gap’, which is the similarity of two shapes under structure-preserving deformations. We also conduct a user study which confirms that this fitting gap is indeed a suitable criterion to evaluate the structural similarity of shapes. Furthermore, we introduce a dataset of 202 VR sketches for 202 3D shapes drawn from memory rather than from observation. The code and data are available at https://github.com/Rowl1ng/Structure-Aware-VR-Sketch-Shape-Retrieval
This paper, for the very first time, introduces human sketches to the landscape of XAI (Explainable Artificial Intelligence). We argue that sketch as a "human-centered" data form, represents a natural interface to study explainability. We focus on cultivating sketch-specific explainability designs. This starts by identifying strokes as a unique building block that offers a degree of flexibility in object construction and manipulation impossible in photos. Following this, we design a simple explainability-friendly sketch encoder that accommodates the intrinsic properties of strokes: shape, location, and order. We then define the first ever XAI task for sketch, that of stroke location inversion (SLI). Just as we have heat maps for photos and correlation matrices for text, SLI offers an explainability angle to sketch by asking a network how well it can recover stroke locations of an unseen sketch. We provide qualitative results for readers to interpret as snapshots of the SLI process in the paper and as GIFs on the project page. A minor but exciting note is that thanks to its sketch-specific design, our sketch encoder also yields the best sketch recognition accuracy to date while having the smallest number of parameters. The code is available at https://sketchxai.github.io.
Mobile phones and tablets are rapidly gaining significance as omnipresent image and video capture devices. In this context we present an algorithm that allows such devices to capture high dynamic range (HDR) video. The design of the algorithm was informed by a perceptual study that assesses the relative importance of motion and dynamic range. We found that ghosting artefacts are more visually disturbing than a reduction in dynamic range, even if a comparable number of pixels is affected by each. We incorporated these findings into a real-time, adaptive metering algorithm that seamlessly adjusts its settings to take exposures that will lead to minimal visual artefacts after recombination into an HDR sequence. It is uniquely suitable for real-time selection of exposure settings. Finally, we present an off-line HDR reconstruction algorithm that is matched to the adaptive nature of our real-time metering approach.
Product designers extensively use sketches to create and communicate 3D shapes and thus form an ideal audience for sketch-based modeling, nonphotorealistic rendering and sketch filtering. However, sketching requires significant expertise and time, making design sketches a scarce resource for the research community. We introduce OpenSketch, a dataset of product design sketches aimed at offering a rich source of information for a variety of computer-aided design tasks. OpenSketch contains more than 400 sketches representing 12 man-made objects drawn by 7 to 15 product designers of varying expertise. We provided participants with front, side and top views of these objects, and instructed them to draw from two novel perspective viewpoints. This drawing task forces designers to construct the shape front their mental vision rather than directly copy what they see. They achieve this task by employing a variety of sketching techniques and methods not observed in prior datasets. Together with industrial design teachers, we distilled a taxonomy of line types and used it to label each stroke of the 214 sketches drawn from one of the two viewpoints. While some of these lines have long been known in computer graphics, others remain to be reproduced algorithmically or exploited for shape inference. In addition, we also asked participants to produce clean presentation drawings from each of their sketches, resulting in aligned pairs of drawings of different styles. Finally, we registered each sketch to its reference 3D model by annotating sparse correspondences. We provide an analysis of our annotated sketches, which reveals systematic drawing strategies over time and shapes, as well as a positive correlation between presence of construction lines and accuracy. Our sketches, in combination with provided annotations, fonts challenging benchmarks for existing algorithms as well as a great source of inspiration for future developments. We illustrate the versatility of our data by using it to test a 3D reconstruction deep network trained on synthetic drawings, as well as to train a filtering network to convert concept sketches into presentation drawings. We distribute our dataset under the Creative Commons CC0 license: https://nsinria.fr/d3/OpenSketch.
Image calibration requires both linearization of pixel values and scaling so that values in the image correspond to real-world luminances. In this paper we focus on the latter and rather than rely on camera characterization, we calibrate images by analysing their content and metadata, obviating the need for expensive measuring devices or modeling of lens and camera combinations. Our analysis correlates sky pixel values to luminances that would be expected based on geographical metadata. Combined with high dynamic range (HDR) imaging, which gives us linear pixel data, our algorithm allows us to find absolute luminance values for each pixel—effectively turning digital cameras into absolute light meters. To validate our algorithm we have collected and annotated a calibrated set of HDR images and compared our estimation with several other approaches, showing that our approach is able to more accurately recover absolute luminance. We discuss various applications and demonstrate the utility of our method in the context of calibrated color appearance reproduction and lighting design.
In this paper, for the first time, we investigate the problem of generating 3D shapes from professional 2D sketches via deep learning. We target sketches done by professional artists, as these sketches are likely to contain more details than the ones produced by novices, and thus the reconstruction from such sketches poses a higher demand on the level of detail in the reconstructed models. This is importantly different to previous work, where the training and testing was conducted on either synthetic sketches or sketches done by novices. Novices sketches often depict shapes that are physically unrealistic, while models trained with synthetic sketches could not cope with the level of abstraction and style found in real sketches. To address this problem, we collected the first large-scale dataset of professional sketches, where each sketch is paired with a reference 3D shape, with a total of 1,500 professional sketches collected across 500 3D shapes. The dataset is available at http://sketchx.ai/downloads/. We introduce two bespoke designs within a deep adversarial network to tackle the imprecision of human sketches and the unique figure/ground ambiguity problem inherent to sketch-based reconstruction. We show that existing 3D shapes generation methods designed for images fail to be naively applied to our problem, and demonstrate the effectiveness of our method both qualitatively and quantitatively.
With the improvement of both acquisition techniques, and computational and storage capabilities, we are witnessing an increasing presence of multidimensional scene representations. Two-dimensional, conventional images are gradually losing their hegemony, leaving room for novel formats. Among these, light fields are gaining importance, further propelled by the recent reappearance of virtual reality. Content generation is one of the stumbling blocks in this realm, and light fields are one of the main input sources of content. As their use becomes more common, a key challenge is the ability to edit or modify the appearance of the objects in the light field. This paper presents a method for manipulating the appearance of gloss in light fields. In particular, we propose a multidimensional filtering approach in which the specular highlights are filtered in the spatial and angular domains to target a desired increase of the material roughness. The filtering kernel is computed based on surface normals and view direction. Our technique generates angularly-coherent plausible edits in both synthetic and captured light fields
Concept sketches, ubiquitously used in industrial design, are inherently imprecise yet highly effective at communicating 3D shape to human observers. We present a new symmetry-driven algorithm for recovering designer-intended 3D geometry from concept sketches. We observe that most concept sketches of human-made shapes are structured around locally symmetric building blocks, defined by triplets of orthogonal symmetry planes. We identify potential building blocks using a combination of 2D symmetries and drawing order. We reconstruct each such building block by leveraging a combination of perceptual cues and observations about designer drawingchoices. We cast this reconstruction as an integer programming problem where we seek to identify, among the large set of candidate symmetry correspondences formed by approximate pen strokes, the subset that results in the most symmetric and well-connected shape. We demonstrate the robustness of our approach by reconstructing 82 sketches, which exhibit significant over-sketching, inaccurate perspective, partial symmetry, and other imperfections. In a comparative study, participants judged our results as superior to the state-of-the-art by a ratio of 2:1.
Deep image-based modeling received lots of attention in recent years, yet the parallel problem of sketch-based modeling has only been briefly studied, often as a potential application. In this work, for the first time, we identify the main differences between sketch and image inputs: (i) style variance, (ii) imprecise perspective, and (iii) sparsity. We discuss why each of these differences can pose a challenge, and even make a certain class of image-based methods inapplicable. We study alternative solutions to address each of the difference. By doing so, we drive out a few important insights: (i) sparsity commonly results in an incorrect prediction of foreground versus background, (ii) diversity of human styles, if not taken into account, can lead to very poor generalization properties, and finally (iii) unless a dedicated sketching interface is used, one can not expect sketches to match a perspective of a fixed viewpoint. Finally, we compare a set of representative deep single-image modeling solutions and show how their performance can be improved to tackle sketch input by taking into consideration the identified critical differences.
Designing real and virtual garments is becoming extremely demanding with rapidly changing fashion trends and the increasing need for synthesizing realistically dressed digital humans for various applications. However, traditionally designing real and virtual garments has been time-consuming. Sketch-based modeling aims to bring the ease and immediacy of drawing to the 3D world thereby motivating faster iterations. We propose a novel sketch-based garment modeling framework specifically targeted to synchronize with the iterative process of garment ideation, e.g., adding or removing details from different views in each iteration. At the core of our learning-based approach is a view-aware feature aggregation module that fuses the features from the latest sketch with the thus far aggregated features to effectively refine the generated 3D shape. We evaluate our approach on a wide variety of garment types and iterative refinement scenarios. We also provide comparisons to alternative feature aggregation methods and demonstrate favorable results. The code is available at https://github.com/pinakinathc/multiviewsketch-garment.
Growing free online 3D shapes collections dictated research on 3D retrieval. Active debate has however been had on (i) what the best input modality is to trigger retrieval, and (ii) the ultimate usage scenario for such retrieval. In this paper, we offer a different perspective towards answering these questions - we study the use of 3D sketches as an input modality and advocate a VR-scenario where retrieval is conducted. Thus, the ultimate vision is that users can freely retrieve a 3D model by air-doodling in a VR environment. As a first stab at this new 3D VR-sketch to 3D shape retrieval problem, we make four contributions. First, we code a VR utility to collect 3D VR-sketches and conduct retrieval. Second, we collect the first set of 167 3D VRsketches on two shape categories from ModelNet. Third, we propose a novel approach to generate a synthetic dataset of human-like 3D sketches of different abstract levels to train deep networks. At last, we compare the common multi-view and volumetric approaches: We show that, in contrast to 3D shape to 3D shape retrieval, volumetric point-based approaches exhibit superior performance on 3D sketch to 3D shape retrieval due to the sparse and abstract nature of 3D VR-sketches. We believe these contributions will collectively serve as enablers for future attempts at this problem. The VR interface, code and datasets are available at https://tinyurl.com/3DSketch3DV.
We present the first fine-grained dataset of 1,497 3D VR sketch and 3D shape pairs of a chair category with large shapes diversity. Our dataset supports the recent trend in the sketch community on fine-grained data analysis, and extends it to an actively developing 3D domain. We argue for the most convenient sketching scenario where the sketch consists of sparse lines and does not require any sketching skills, prior training or time-consuming accurate drawing. We then, for the first time, study the scenario of fine-grained 3D VR sketch to 3D shape retrieval, as a novel VR sketching application and a proving ground to drive out generic insights to inform future research. By experimenting with carefully selected combinations of design factors on this new problem, we draw important conclusions to help follow-on work. We hope our dataset will enable other novel applications, especially those that require a fine-grained angle such as fine-grained 3D shape reconstruction. The dataset is available at tinyurl.com/ VRSketch3DV21.