A Bayesian approach to complex land use change modelling
Start date
October 2019End date
May 2020Overview
The UK is currently facing a housing shortage and the government plan is to increase house building to a rate of 300,000 per year in the mid-2020s. This is largely supported through policy instruments related to improving access to personal finance and reducing planning barriers. According to the government, over 40 per cent of local planning authorities do not have a plan that meets the projected growth in households in their area. Against this backdrop of announced substantial, poorly understood and more liberally regulated land use change, there is a clear need to understand possible patterns of future urban growth.
There exists computational models that take economic and demographic forecasts and use these to explore possible trajectories of land use change. A particular family of such models are cellular automata (CA) land use models; these models are complex in a mathematical sense as they represent the chaotic and spatially uneven processes by which cities and towns can grow. A major hurdle preventing the widespread use of these models is that the better performing models are calibrated based on 'expert judgement'. This reliance on manual intervention is not only resource intensive but also casts a shadow on the objective reliability of model results.
Aims and objectives
In this project we will investigate the use of a statistical technique of approximate Bayesian computation to automatically calibrate CA land use models. We aim to deliver a proof-of-concept demonstrating the methods on a CA model that is stripped down to its fundamental essentials and applied to a variety of UK and European urban areas.
A further advantage of the Bayesian approach is that it will not just give the best-fitting set of parameters, but also the associated uncertainty. This will allow analysts to use the model to support decisions with confidence based on a range of possible outcomes and their likelihood.
Funder
Team
Investigators
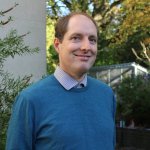
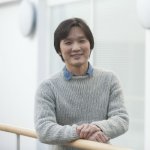
Collaborators
- Department of Civil and Environmental Engineering, University of Surrey
- School of Mathematics and Physics, University of Surrey.