9am - 10am
Wednesday 29 May 2024
Fine-Grained Vision-Language Learning
PhD Viva Open Presentation for Brandon Han
Online event - All Welcome!
Free
Guildford
Surrey
GU2 7XH
This event has passed
Fine-Grained Vision-Language Learning
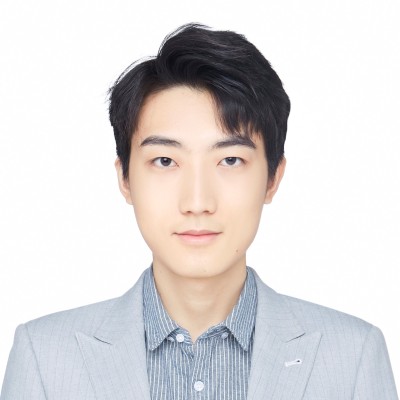
Abstract:
In the ever-evolving landscape of Vision-Language (V+L) learning, the synergy between visual and textual information has proven pivotal for a multitude of tasks, ranging from discriminative to generative objectives. Nevertheless, in specific fine-grained contexts within practical applications, such as e-commerce and human-related modeling, the intricate characteristics of individual instances are difficult to represent, distinguish, and generate. Generic V+L methods often struggle in these nuanced situations due to the lack of specialized designs to address the unique attributes inherent to fine-grained tasks. In light of these challenges, this thesis delves into the world of fine-grained vision-language learning, proposing innovative solutions for typical fine-grained V+L cases to propel the field forward.
Three contributions are made in this thesis. First, we investigate how to learn better fine-grained V+L representations. We present novel pre-training objectives specifically tailored to the unique attributes of the fashion domain, along with a flexible and versatile pretraining architecture. This approach is designed to offer more discriminative and generalizable features, enhancing the performance of a wide range of downstream tasks in the fashion domain. Second, we study how to parameter-efficiently unify fine-grained heterogeneous V+L tasks in a multi-task model. We propose two lightweight adapters and a stable optimization strategy to support simultaneously training a V+L model across multiple heterogeneous tasks, which outperforms independently trained single-task models in discriminative and generative downstream tasks (incl. cross-modal matching, multi-modal recognition, and image-to-text generation) with significant parameter saving. Finally, we explore how to use natural language to create fine-grained visual content – 3D head avatars. Building upon the foundation of 2D text-to-image diffusion models, we enhance the diffusion process by incorporating 3D awareness of head priors and enable fine-grained editing through the proposed identity-aware score distillation method, resulting in superior fidelity and editing capabilities.
Featured Academics
Visitor information
Find out how to get to the University, make your way around campus and see what you can do when you get here.