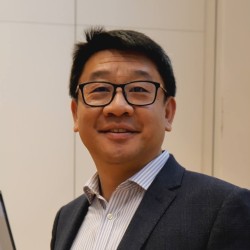
Professor Yi-Zhe Song
Academic and research departments
Centre for Vision, Speech and Signal Processing (CVSSP), School of Computer Science and Electronic Engineering.About
Biography
Yi-Zhe Song is a Professor of Computer Vision and Machine Learning, at the Centre for Vision Speech and Signal Processing (CVSSP), one of the UK's oldest and largest research centres on Artificial Intelligence.
He leads the SketchX Lab within CVSSP - a large research group of 3 academics, 2 postdocs, and 14 full-time PhD students. His vision for SketchX is understanding how seeing can be explained by drawing. In other words, how better understanding of human sketch data can be translated to insights of how human visual systems operate, and in turn how such insights can benefit computer vision and cognitive science at large.
He is an Associate Editor of the IEEE Transactions on Pattern Analysis and Machine Intelligence (TPAMI), the world's top-ranked journal in computer vision
and machine learning in terms of impact factor (16.389), and a Programme Chair for British Machine Vision Conference (BMVC) 2021. He is also an Associate Editor of Frontiers in Computer Science – Computer Vision, and regularly serves as Area Chair (AC) for flagship computer vision and machine learning conferences, most recently as AC for ECCV'22, CVPR'22, and ICCV'21.
SketchX publishes consistently in top-tier conferences* (CVPR, ICCV, ECCV, SIGGRAPH Asia, ICML, BMVC) and journals (IJCV, TIP, TVCG, TCSVT), including a Best Paper Award at British Machine Vision Conference 2015. (*41 x CVPR, 11 x ICCV, 11 x ECCV, 3 x SIGGRAPH Asia, 3 x ICLR, 1 x ICML, 3 x NeurIPS as of June 2023)
He founded, and currently leads the MSc in AI programme at Surrey, having previously established an MSc in AI programme at Queen Mary University of London.
He obtained a PhD in 2008 on Computer Vision and Machine Learning from the University of Bath, a MSc (with Best Dissertation Award) in 2004 from the University of Cambridge, and a Bachelor's Degree (First Class Honours) in 2003 from the University of Bath.
He is a Senior Member of IEEE, a Fellow of the Higher Education Academy (HEA), as well as full member of the EPSRC review college. He also reviews for other international funding bodies, such as Czech Science Foundation, and São Paulo Research Foundation of Brazil.
News
Publications
The study of neural generative models of human sketches is a fascinating contemporary modeling problem due to the links between sketch image generation and the human drawing process. The landmark SketchRNN provided breakthrough by sequentially generating sketches as a sequence of waypoints. However this leads to low-resolution image generation, and failure to model long sketches. In this paper we present B´ezierSketch, a novel generative model for fully vector sketches that are automatically scalable and high-resolution. To this end, we first introduce a novel inverse graphics approach to stroke embedding that trains an encoder to embed each stroke to its best fit B´ezier curve. This enables us to treat sketches as short sequences of paramaterized strokes and thus train a recurrent sketch generator with greater capacity for longer sketches, while producing scalable high-resolution results. We report qualitative and quantitative results on the Quick, Draw! benchmark.
Sketches are highly expressive, inherently capturing subjective and fine-grained visual cues. The exploration of such innate properties of human sketches has, however, been limited to that of image retrieval. In this paper, for the first time, we cultivate the expressiveness of sketches but for the fundamental vision task of object detection. The end result is a sketch-enabled object detection framework that detects based on what \textit{you} sketch -- \textit{that} ``zebra'' (e.g., one that is eating the grass) in a herd of zebras (instance-aware detection), and only the \textit{part} (e.g., ``head" of a ``zebra") that you desire (part-aware detection). We further dictate that our model works without (i) knowing which category to expect at testing (zero-shot) and (ii) not requiring additional bounding boxes (as per fully supervised) and class labels (as per weakly supervised). Instead of devising a model from the ground up, we show an intuitive synergy between foundation models (e.g., CLIP) and existing sketch models build for sketch-based image retrieval (SBIR), which can already elegantly solve the task -- CLIP to provide model generalisation, and SBIR to bridge the (sketch$\rightarrow$photo) gap. In particular, we first perform independent prompting on both sketch and photo branches of an SBIR model to build highly generalisable sketch and photo encoders on the back of the generalisation ability of CLIP. We then devise a training paradigm to adapt the learned encoders for object detection, such that the region embeddings of detected boxes are aligned with the sketch and photo embeddings from SBIR. Evaluating our framework on standard object detection datasets like PASCAL-VOC and MS-COCO outperforms both supervised (SOD) and weakly-supervised object detectors (WSOD) on zero-shot setups. Project Page: \url{https://pinakinathc.github.io/sketch-detect}
Human sketch has already proved its worth in various visual understanding tasks (e.g., retrieval, segmentation, image-captioning, etc). In this paper, we reveal a new trait of sketches - that they are also salient. This is intuitive as sketching is a natural attentive process at its core. More specifically, we aim to study how sketches can be used as a weak label to detect salient objects present in an image. To this end, we propose a novel method that emphasises on how "salient object" could be explained by hand-drawn sketches. To accomplish this, we introduce a photo-to-sketch generation model that aims to generate sequential sketch coordinates corresponding to a given visual photo through a 2D attention mechanism. Attention maps accumulated across the time steps give rise to salient regions in the process. Extensive quantitative and qualitative experiments prove our hypothesis and delineate how our sketch-based saliency detection model gives a competitive performance compared to the state-of-the-art.
In this paper, we extend scene understanding to include that of human sketch. The result is a complete trilogy of scene representation from three diverse and complementary modalities -- sketch, photo, and text. Instead of learning a rigid three-way embedding and be done with it, we focus on learning a flexible joint embedding that fully supports the ``optionality" that this complementarity brings. Our embedding supports optionality on two axes: (i) optionality across modalities -- use any combination of modalities as query for downstream tasks like retrieval, (ii) optionality across tasks -- simultaneously utilising the embedding for either discriminative (e.g., retrieval) or generative tasks (e.g., captioning). This provides flexibility to end-users by exploiting the best of each modality, therefore serving the very purpose behind our proposal of a trilogy in the first place. First, a combination of information-bottleneck and conditional invertible neural networks disentangle the modality-specific component from modality-agnostic in sketch, photo, and text. Second, the modality-agnostic instances from sketch, photo, and text are synergised using a modified cross-attention. Once learned, we show our embedding can accommodate a multi-facet of scene-related tasks, including those enabled for the first time by the inclusion of sketch, all without any task-specific modifications. Project Page: \url{http://www.pinakinathc.me/scenetrilogy}
Given an abstract, deformed, ordinary sketch from untrained amateurs like you and me, this paper turns it into a photorealistic image - just like those shown in Fig. 1(a), all non-cherry-picked. We differ significantly from prior art in that we do not dictate an edgemap-like sketch to start with, but aim to work with abstract free-hand human sketches. In doing so, we essentially democratise the sketch-to-photo pipeline, "picturing" a sketch regardless of how good you sketch. Our contribution at the outset is a decoupled encoder-decoder training paradigm, where the decoder is a StyleGAN trained on photos only. This importantly ensures that generated results are always photorealistic. The rest is then all centred around how best to deal with the abstraction gap between sketch and photo. For that, we propose an autoregressive sketch mapper trained on sketch-photo pairs that maps a sketch to the StyleGAN latent space. We further introduce specific designs to tackle the abstract nature of human sketches, including a fine-grained discriminative loss on the back of a trained sketch-photo retrieval model, and a partial-aware sketch augmentation strategy. Finally, we showcase a few downstream tasks our generation model enables, amongst them is showing how fine-grained sketch-based image retrieval, a well-studied problem in the sketch community, can be reduced to an image (generated) to image retrieval task, surpassing state-of-the-arts. We put forward generated results in the supplementary for everyone to scrutinise.
Unsupervised domain adaptation aims to leverage labeled data from a source domain to learn a classifier for an unlabeled target domain. Among its many variants, open set domain adaptation (OSDA) is perhaps the most challenging, as it further assumes the presence of unknown classes in the target domain. In this paper, we study OSDA with a particular focus on enriching its ability to traverse across larger domain gaps. Firstly, we show that existing state-of-the-art methods suffer a considerable performance drop in the presence of larger domain gaps, especially on a new dataset (PACS) that we re-purposed for OSDA. We then propose a novel framework to specifically address the larger domain gaps. The key insight lies with how we exploit the mutually beneficial information between two networks; (a) to separate samples of known and unknown classes, (b) to maximize the domain confusion between source and target domain without the influence of unknown samples. It follows that (a) and (b) will mutually supervise each other and alternate until convergence. Extensive experiments are conducted on Office-31, Office-Home, and PACS datasets, demonstrating the superiority of our method in comparison to other state-of-the-arts. Code available at https://github.com/dongliangchang/Mutual-to-Separate/
This paper advances the fine-grained sketch-based image retrieval (FG-SBIR) literature by putting forward a strong baseline that overshoots prior state-of-the-arts by ~11%. This is not via complicated design though, but by addressing two critical issues facing the community (i) the gold standard triplet loss does not enforce holistic latent space geometry, and (ii) there are never enough sketches to train a high accuracy model. For the former, we propose a simple modification to the standard triplet loss, that explicitly enforces separation amongst photos/sketch instances. For the latter, we put forward a novel knowledge distillation module can leverage photo data for model training. Both modules are then plugged into a novel plug-n-playable training paradigm that allows for more stable training. More specifically, for (i) we employ an intra-modal triplet loss amongst sketches to bring sketches of the same instance closer from others, and one more amongst photos to push away different photo instances while bringing closer a structurally augmented version of the same photo (offering a gain of ~4-6%). To tackle (ii), we first pre-train a teacher on the large set of unlabelled photos over the aforementioned intra-modal photo triplet loss. Then we distill the contextual similarity present amongst the instances in the teacher's embedding space to that in the student's embedding space, by matching the distribution over inter-feature distances of respective samples in both embedding spaces (delivering a further gain of ~4-5%). Apart from outperforming prior arts significantly, our model also yields satisfactory results on generalising to new classes. Project page: https://aneeshan95.github.io/Sketch_PVT/
The main challenge for fine-grained few-shot image classification is to learn feature representations with higher inter-class and lower intra-class variations, with a mere few labelled samples. Conventional few-shot learning methods however cannot be naively adopted for this fine-grained setting -- a quick pilot study reveals that they in fact push for the opposite (i.e., lower inter-class variations and higher intra-class variations). To alleviate this problem, prior works predominately use a support set to reconstruct the query image and then utilize metric learning to determine its category. Upon careful inspection, we further reveal that such unidirectional reconstruction methods only help to increase inter-class variations and are not effective in tackling intra-class variations. In this paper, we for the first time introduce a bi-reconstruction mechanism that can simultaneously accommodate for inter-class and intra-class variations. In addition to using the support set to reconstruct the query set for increasing inter-class variations, we further use the query set to reconstruct the support set for reducing intra-class variations. This design effectively helps the model to explore more subtle and discriminative features which is key for the fine-grained problem in hand. Furthermore, we also construct a self-reconstruction module to work alongside the bi-directional module to make the features even more discriminative. Experimental results on three widely used fine-grained image classification datasets consistently show considerable improvements compared with other methods. Codes are available at: https://github.com/PRIS-CV/Bi-FRN.
Unsupervised domain adaptation aims to leverage labeled data from a source domain to learn a classifier for an unlabeled target domain. Amongst its many variants, open set domain adaptation (OSDA) is perhaps the most challenging one, as it further assumes the presence of unknown classes in the target domain. In this paper, we study OSDA with a particular focus on enriching its ability to traverse across larger domain gaps, and we show that existing state-of-the-art methods suffer a considerable performance drop in the presence of larger domain gaps, especially on a new dataset (PACS) that we re-purposed for OSDA. Exploring this is pivotal for OSDA as with increasing domain shift, identifying unknown samples in the target domain becomes harder for the model, thus making negative transfer between source and target domains more challenging. Accordingly, we propose a Mutual-to-Separate (MTS) framework to address the larger domain gaps. Essentially we design two networks – (a) Sample Separation Network (SSN): which is trained to learn a hyperplane for separating unknown samples from known ones, and (b) Distribution Matching Network (DMN): which is trained to maximise domain confusion between source and target domains without unknown samples under the guidance of the SSN. The key insight lies in how we exploit the mutually beneficial information between these two networks. On closer observation, we see that SSN can reveal which samples in the target domain belong to the unknown class by instance weighting whereas, DMN pushes apart the samples that most likely belong to the unknown class in the target domain, which in turn reduces the difficulty of SSN in identifying unknown samples. It follows that (a) and (b) will mutually supervise each other and alternate until convergence, which can better align the source and target domains in the shared label space. Extensive experiments on five datasets (Office-31, Office-Home, PACS, VisDA, and mini DomainNet) demonstrate the efficiency of the proposed method. Detailed ablation experiments also validate the effectiveness of each component and the generality of the proposed framework. Codes are available at: https://github.com/PRIS-CV/Mutual-to-Separate.
Sketch semantic segmentation serves as an important part of sketch interpretation. Recently, some researchers have obtained significant results using graph neural networks (GNN) for this task. However, existing GNN-based methods usually neglect the drawing order of sketches thus missing out the sequence information inherent to sketches. Towards solving this problem to achieve better performance on sketch semantic segmentation, we propose an encoder-decoder GNN framework named ENDE-GNN. Working with an auxiliary decoder, our ENDE-GNN guides the GNN backbone network to not only extract the inter-stroke and intra-stroke features, but also pays attention to the drawing order of sketches. This decoder acts during training only, preventing any additional overhead during testing. The proposed ENDE-GNN obtains state-of-the-art performances on three public sketch semantic segmentation datasets, namely SPG, SketchSeg-150K, and CreativeSketch. We further evaluate the effectiveness of ENDE-GNN via ablation studies and visualizations. Codes are available at https://github.com/PRIS- CV/ENDE For SSS.
Sketches are distinctly different to photos. They are highly abstract and exhibit a severe lack of visual cues. Prior works have therefore explored additional traits unique to sketches to help recognition such as stroke ordering. In this paper, we pioneer in studying the role of structure in sketches, for the task of sketch recognition. In particular, we propose a novel graph representation specifically designed for sketches, which follows the inherent hierarchical relationship (segment-stroke-sketch") of sketching elements. By conforming to this hierarchy, we also introduce ajoint network that encapsulates both the structural and temporal traits of sketches for sketch recognition, termed S 3Net. S 3Net employs a recurrent neural network (RNN) to extract segmentlevel features, followed by a graph convolutional network (GCN) to aggregate them into sketch-level features. The RNN first encodes temporal cues in sketches while its outputs are used as node embedding to construct a hierarchical sketch-graph. The GCN module then takes in this sketchgraph to produce a structure-aware embedding for sketches. Extensive experiments on the QuickDraw dataset, exhibit superior performance over state-of-the-arts, surpassing them by over 4%. Ablative studies further demonstrate the effectiveness of the proposed structural graph for both inter-class, and intra-class feature discrimination. Code is available at: https://github.com/yanglan0225/s3net;.
We present the first competitive drawing agent Pixelor that exhibits human-level performance at a Pictionary-like sketching game, where the participant whose sketch is recognized first is a winner. Our AI agent can autonomously sketch a given visual concept, and achieve a recognizable rendition as quickly or faster than a human competitor. The key to victory for the agent’s goal is to learn the optimal stroke sequencing strategies that generate the most recognizable and distinguishable strokes first. Training Pixelor is done in two steps. First, we infer the stroke order that maximizes early recognizability of human training sketches. Second, this order is used to supervise the training of a sequence-to-sequence stroke generator. Our key technical contributions are a tractable search of the exponential space of orderings using neural sorting; and an improved Seq2Seq Wasserstein (S2S-WAE) generator that uses an optimal-transport loss to accommodate the multi-modal nature of the optimal stroke distribution. Our analysis shows that Pixelor is better than the human players of the Quick, Draw! game, under both AI and human judging of early recognition. To analyze the impact of human competitors’ strategies, we conducted a further human study with participants being given unlimited thinking time and training in early recognizability by feedback from an AI judge. The study shows that humans do gradually improve their strategies with training, but overall Pixelor still matches human performance. The code and the dataset are available at http://sketchx.ai/pixelor.
We present the first one-shot personalized sketch segmentation method. We aim to segment all sketches belonging to the same category provisioned with a single sketch with a given part annotation while (i) preserving the parts semantics embedded in the exemplar, and (ii) being robust to input style and abstraction. We refer to this scenario as personalized . With that, we importantly enable a much-desired personalization capability for downstream fine-grained sketch analysis tasks. To train a robust segmentation module, we deform the exemplar sketch to each of the available sketches of the same category. Our method generalizes to sketches not observed during training. Our central contribution is a sketch-specific hierarchical deformation network. Given a multi-level sketch-strokes encoding obtained via a graph convolutional network, our method estimates rigid-body transformation from the target to the exemplar, on the upper level. Finer deformation from the exemplar to the globally warped target sketch is further obtained through stroke-wise deformations, on the lower-level. Both levels of deformation are guided by mean squared distances between the keypoints learned without supervision, ensuring that the stroke semantics are preserved. We evaluate our method against the state-of-the-art segmentation and perceptual grouping baselines re-purposed for the one-shot setting and against two few-shot 3D shape segmentation methods. We show that our method outperforms all the alternatives by more than 10% on average. Ablation studies further demonstrate that our method is robust to personalization : changes in input part semantics and style differences.
In this paper, we focus on learning semantic representations for large-scale highly abstract sketches that were produced by the practical sketch-based application rather than the excessively well dawn sketches obtained by crowd-sourcing. We propose a dual-branch CNN-RNN network architecture to represent sketches, which simultaneously encodes both the static and temporal patterns of sketch strokes. Based on this architecture, we further explore learning the sketch-oriented semantic representations in two practical settings, i.e., hashing retrieval and zero-shot recognition on million-scale highly abstract sketches produced by practical online interactions. Specifically, we use our dual-branch architecture as a universal representation framework to design two sketch-specific deep models: (i) We propose a deep hashing model for sketch retrieval, where a novel hashing loss is specifically designed to further accommodate both the abstract and messy traits of sketches. (ii) We propose a deep embedding model for sketch zero-shot recognition, via collecting a large-scale edge-map dataset and proposing to extract a set of semantic vectors from edge-maps as the semantic knowledge for sketch zero-shot domain alignment. Both deep models are evaluated by comprehensive experiments on million-scale abstract sketches produced by a global online game QuickDraw and outperform state-of-the-art competitors.
In this paper, we tackle for the first time, the problem of self-supervised representation learning for free-hand sketches. This importantly addresses a common problem faced by the sketch community -- that annotated supervisory data are difficult to obtain. This problem is very challenging in that sketches are highly abstract and subject to different drawing styles, making existing solutions tailored for photos unsuitable. Key for the success of our self-supervised learning paradigm lies with our sketch-specific designs: (i) we propose a set of pretext tasks specifically designed for sketches that mimic different drawing styles, and (ii) we further exploit the use of a textual convolution network (TCN) in a dual-branch architecture for sketch feature learning, as means to accommodate the sequential stroke nature of sketches. We demonstrate the superiority of our sketch-specific designs through two sketch-related applications (retrieval and recognition) on a million-scale sketch dataset, and show that the proposed approach outperforms the state-of-the-art unsupervised representation learning methods, and significantly narrows the performance gap between with supervised representation learning.
Sketching enables many exciting applications, notably, image retrieval. The fear-to-sketch problem (i.e., "I can't sketch") has however proven to be fatal for its widespread adoption. This paper tackles this "fear" head on, and for the first time, proposes an auxiliary module for existing retrieval models that predominantly lets the users sketch without having to worry. We first conducted a pilot study that revealed the secret lies in the existence of noisy strokes, but not so much of the "I can't sketch". We consequently design a stroke subset selector that detects noisy strokes, leaving only those which make a positive contribution towards successful retrieval. Our Reinforcement Learning based formulation quantifies the importance of each stroke present in a given subset, based on the extent to which that stroke contributes to retrieval. When combined with pre-trained retrieval models as a pre-processing module, we achieve a significant gain of 8%40% over standard baselines and in turn report new state-of-the-art performance. Last but not least, we demonstrate the selector once trained, can also be used in a plug-and-play manner to empower various sketch applications in ways that were not previously possible.
Fine-grained sketch-based image retrieval (FG-SBIR) addresses the problem of retrieving a particular photo instance given a user’s query sketch. Its widespread applicability is however hindered by the fact that drawing a sketch takes time, and most people struggle to draw a complete and faithful sketch. In this paper, we reformulate the conventional FG-SBIR framework to tackle these challenges, with the ultimate goal of retrieving the target photo with the least number of strokes possible. We further propose an on-the-fly design that starts retrieving as soon as the user starts drawing. To accomplish this, we devise a reinforcement learning based cross-modal retrieval framework that directly optimizes rank of the ground-truth photo over a complete sketch drawing episode. Additionally, we introduce a novel reward scheme that circumvents the problems related to irrelevant sketch strokes, and thus provides us with a more consistent rank list during the retrieval. We achievesuperiorearly-retrievalefficiencyoverstate-of-theartmethodsandalternativebaselinesontwopubliclyavailable fine-grained sketch retrieval datasets.
We advance sketch research to scenes with the first dataset of freehand scene sketches, FS-COCO. With practical applications in mind, we collect sketches that convey scene content well but can be sketched within a few minutes by a person with any sketching skills. Our dataset comprises 10,000 freehand scene vector sketches with per point space-time information by 100 non-expert individuals, offering both object- and scene-level abstraction. Each sketch is augmented with its text description. Using our dataset, we study for the first time the problem of fine-grained image retrieval from freehand scene sketches and sketch captions. We draw insights on: (i) Scene salience encoded in sketches using the strokes temporal order; (ii) Performance comparison of image retrieval from a scene sketch and an image caption; (iii) Complementarity of information in sketches and image captions, as well as the potential benefit of combining the two modalities. In addition, we extend a popular vector sketch LSTM-based encoder to handle sketches with larger complexity than was supported by previous work. Namely, we propose a hierarchical sketch decoder, which we leverage at a sketch-specific ``pretext" task. Our dataset enables for the first time research on freehand scene sketch understanding and its practical applications. We release the dataset under CC BY-NC 4.0 license: https://fscoco.github.io
The main challenge for fine-grained few-shot image classification is to learn feature representations with higher inter-class and lower intra-class variations, with a mere few labelled samples. Conventional few-shot learning methods however cannot be naively adopted for this fine-grained setting -- a quick pilot study reveals that they in fact push for the opposite (i.e., lower inter-class variations and higher intra-class variations). To alleviate this problem, prior works predominately use a support set to reconstruct the query image and then utilize metric learning to determine its category. Upon careful inspection, we further reveal that such unidirectional reconstruction methods only help to increase inter-class variations and are not effective in tackling intra-class variations. In this paper, we for the first time introduce a bi-reconstruction mechanism that can simultaneously accommodate for inter-class and intra-class variations. In addition to using the support set to reconstruct the query set for increasing inter-class variations, we further use the query set to reconstruct the support set for reducing intra-class variations. This design effectively helps the model to explore more subtle and discriminative features which is key for the fine-grained problem in hand. Furthermore, we also construct a self-reconstruction module to work alongside the bi-directional module to make the features even more discriminative. Experimental results on three widely used fine-grained image classification datasets consistently show considerable improvements compared with other methods. Codes are available at: https://github.com/PRIS-CV/Bi-FRN.
In this paper, we leverage CLIP for zero-shot sketch based image retrieval (ZS-SBIR). We are largely inspired by recent advances on foundation models and the unparalleled generalisation ability they seem to offer, but for the first time tailor it to benefit the sketch community. We put forward novel designs on how best to achieve this synergy, for both the category setting and the fine-grained setting ("all"). At the very core of our solution is a prompt learning setup. First we show just via factoring in sketch-specific prompts, we already have a category-level ZS-SBIR system that overshoots all prior arts, by a large margin (24.8%) - a great testimony on studying the CLIP and ZS-SBIR synergy. Moving onto the fine-grained setup is however trickier, and requires a deeper dive into this synergy. For that, we come up with two specific designs to tackle the fine-grained matching nature of the problem: (i) an additional regularisation loss to ensure the relative separation between sketches and photos is uniform across categories, which is not the case for the gold standard standalone triplet loss, and (ii) a clever patch shuffling technique to help establishing instance-level structural correspondences between sketch-photo pairs. With these designs, we again observe significant performance gains in the region of 26.9% over previous state-of-the-art. The take-home message, if any, is the proposed CLIP and prompt learning paradigm carries great promise in tackling other sketch-related tasks (not limited to ZS-SBIR) where data scarcity remains a great challenge. Project page: https://aneeshan95.github.io/Sketch_LVM/
Despite great strides made on fine-grained visual classification (FGVC), current methods are still heavily reliant on fully-supervised paradigms where ample expert labels are called for. Semi-supervised learning (SSL) techniques, acquiring knowledge from unlabeled data, provide a considerable means forward and have shown great promise for coarse-grained problems. However, exiting SSL paradigms mostly assume in-distribution (i.e., category-aligned) unlabeled data, which hinders their effectiveness when re-proposed on FGVC. In this paper, we put forward a novel design specifically aimed at making out-of-distribution data work for semi-supervised FGVC, i.e., to "clue them in". We work off an important assumption that all fine-grained categories naturally follow a hierarchical structure (e.g., the phylogenetic tree of "Aves" that covers all bird species). It follows that, instead of operating on individual samples, we can instead predict sample relations within this tree structure as the optimization goal of SSL. Beyond this, we further introduced two strategies uniquely brought by these tree structures to achieve inter-sample consistency regularization and reliable pseudo-relation. Our experimental results reveal that (i) the proposed method yields good robustness against out-of-distribution data, and (ii) it can be equipped with prior arts, boosting their performance thus yielding state-of-the-art results. Code is available at https://github.com/PRIS-CV/RelMatch.
Rising concerns about privacy and anonymity preservation of deep learning models have facilitated research in data-free learning (DFL). For the first time, we identify that for data-scarce tasks like Sketch-Based Image Retrieval (SBIR), where the difficulty in acquiring paired photos and hand-drawn sketches limits data-dependent cross-modal learning algorithms, DFL can prove to be a much more practical paradigm. We thus propose Data-Free (DF)-SBIR, where, unlike existing DFL problems, pre-trained, single-modality classification models have to be leveraged to learn a cross-modal metric-space for retrieval without access to any training data. The widespread availability of pre-trained classification models, along with the difficulty in acquiring paired photo-sketch datasets for SBIR justify the practicality of this setting. We present a methodology for DF-SBIR, which can leverage knowledge from models independently trained to perform classification on photos and sketches. We evaluate our model on the Sketchy, TU-Berlin, and QuickDraw benchmarks, designing a variety of baselines based on state-of-the-art DFL literature, and observe that our method surpasses all of them by significant margins. Our method also achieves mAPs competitive with data-dependent approaches, all the while requiring no training data. Implementation is available at \url{https://github.com/abhrac/data-free-sbir}.
3D shape modeling is labor-intensive and time-consuming and requires years of expertise. Recently, 2D sketches and text inputs were considered as conditional modalities to 3D shape generation networks to facilitate 3D shape modeling. However, text does not contain enough fine-grained information and is more suitable to describe a category or appearance rather than geometry, while 2D sketches are ambiguous, and depicting complex 3D shapes in 2D again requires extensive practice. Instead, we explore virtual reality sketches that are drawn directly in 3D. We assume that the sketches are created by novices, without any art training, and aim to reconstruct physically-plausible 3D shapes. Since such sketches are potentially ambiguous, we tackle the problem of the generation of multiple 3D shapes that follow the input sketch structure. Limited in the size of the training data, we carefully design our method, training the model step-by-step and leveraging multi-modal 3D shape representation. To guarantee the plausibility of generated 3D shapes we leverage the normalizing flow that models the distribution of the latent space of 3D shapes. To encourage the fidelity of the generated 3D models to an input sketch, we propose a dedicated loss that we deploy at different stages of the training process. We plan to make our code publicly available.
In recent years, fine-grained visual classification (FGVC) algorithms have achieved excellent performance across a variety of datasets. However, it is still rare to see these algorithms applied in daily life. The main reasons for this are i) the algorithms are developed based on different design guidelines and cannot be deployed in the same environment; ii) there is not a simple and efficient platform to present the algorithm's results to the user - the accuracy is meaningless to the users. To address the above problem, we built a complex scenario-oriented fine-grained visual classification platform. The platform consists of a PyTorch-based fine-grained visual recognition algorithm library (FGL) and a WeChat applet-based user interaction module (WEM). We can quickly develop new algorithms or readily apply existing algorithms in the same environment through FGL. Driven by FGL, the WEM enables users to achieve fine-grained recognition of complex scenes interactively. In addition to showing the user the fine-grained labels of objects, we will also show how the model makes decisions to help the user master the ability to recognise the fine-grained object so that everyone can become a domain expert. A video demo shows an example of the proposed platform in a real-world scenario: https://reurl.cc/rRZE7O.
Deep image-based modeling received lots of attention in recent years, yet the parallel problem of sketch-based modeling has only been briefly studied, often as a potential application. In this work, for the first time, we identify the main differences between sketch and image inputs: (i) style variance, (ii) imprecise perspective, and (iii) sparsity. We discuss why each of these differences can pose a challenge, and even make a certain class of image-based methods inapplicable. We study alternative solutions to address each of the difference. By doing so, we drive out a few important insights: (i) sparsity commonly results in an incorrect prediction of foreground versus background, (ii) diversity of human styles, if not taken into account, can lead to very poor generalization properties, and finally (iii) unless a dedicated sketching interface is used, one can not expect sketches to match a perspective of a fixed viewpoint. Finally, we compare a set of representative deep single-image modeling solutions and show how their performance can be improved to tackle sketch input by taking into consideration the identified critical differences.
Visual text recognition is undoubtedly one of the most extensively researched topics in computer vision. Great progress have been made to date, with the latest models starting to focus on the more practical "in-the-wild" setting. However, a salient problem still hinders practical deployment - prior state-of-arts mostly struggle with recognising unseen (or rarely seen) character sequences. In this paper, we put forward a novel framework to specifically tackle this "unseen" problem. Our framework is iterative in nature, in that it utilises predicted knowledge of character sequences from a previous iteration, to augment the main network in improving the next prediction. Key to our success is a unique cross-modal variational autoencoder to act as a feedback module, which is trained with the presence of textual error distribution data. This module importantly translates a discrete predicted character space, to a continuous affine transformation parameter space used to condition the visual feature map at next iteration. Experiments on common datasets have shown competitive performance over state-of-the-arts under the conventional setting. Most importantly, under the new disjoint setup where train-test labels are mutually exclusive, ours offers the best performance thus showcasing the capability of generalising onto unseen words (Figure 1 offers a summary).
Text recognition remains a fundamental and extensively researched topic in computer vision, largely owing to its wide array of commercial applications. The challenging nature of the very problem however dictated a fragmentation of research efforts: Scene Text Recognition (STR) that deals with text in everyday scenes, and Handwriting Text Recognition (HTR) that tackles hand-written text. In this paper, for the first time, we argue for their unification - we aim for a single model that can compete favourably with two separate state-of-the-art STR and HTR models. We first show that cross-utilisation of STR and HTR models trigger significant performance drops due to differences in their inherent challenges. We then tackle their union by introducing a knowledge distillation (KD) based framework. This however is non-trivial, largely due to the variable-length and sequential nature of text sequences, which renders off-the-shelf KD techniques that mostly work with global fixed length data, inadequate. For that, we propose four distillation losses, all of which are specifically designed to cope with the aforementioned unique characteristics of text recognition. Empirical evidence suggests that our proposed unified model performs at par with individual models, even surpassing them in certain cases. Ablative studies demonstrate that naive baselines such as a two-stage framework, multi-task and domain adaption/generalisation alternatives do not work that well, further authenticating our design.
Zero-shot sketch-based image retrieval typically asks for a trained model to be applied as is to unseen categories. In this paper, we question to argue that this setup by definition is not compatible with the inherent abstract and subjective nature of sketches - the model might transfer well to new categories, but will not understand sketches existing in different test-time distribution as a result. We thus extend ZS-SBIR asking it to transfer to both categories and sketch distributions. Our key contribution is a test-time training paradigm that can adapt using just one sketch. Since there is no paired photo, we make use of a sketch raster-vector reconstruction module as a self-supervised auxiliary task. To maintain the fidelity of the trained cross-modal joint embedding during test-time update, we design a novel metal-earning based training paradigm to learn a separation between model updates incurred by this auxiliary task from those off the primary objective of discriminative learning. Extensive experiments show our model to outperform state-of-the-arts, thanks to the proposed test-time adaption that not only transfers to new categories but also accommodates to new sketching styles.
The recent focus on Fine-Grained Sketch-Based Image Retrieval (FG-SBIR) has shifted towards generalising a model to new categories without any training data from them. In real-world applications, however, a trained FG-SBIR model is often applied to both new categories and different human sketchers, i.e., different drawing styles. Although this complicates the generalisation problem, fortunately, a handful of examples are typically available, enabling the model to adapt to the new category/style. In this paper, we offer a novel perspective - instead of asking for a model that generalises, we advocate for one that quickly adapts, with just very few samples during testing (in a few-shot manner). To solve this new problem, we introduce a novel model-agnostic meta-learning (MAML) based framework with several key modifications: (1) As a retrieval task with a margin-based contrastive loss, we simplify the MAML training in the inner loop to make it more stable and tractable. (2) The margin in our contrastive loss is also meta-learned with the rest of the model. (3) Three additional regularisation losses are introduced in the outer loop, to make the meta-learned FG-SBIR model more effective for category/style adaptation. Extensive experiments on public datasets suggest a large gain over generalisation and zero-shot based approaches, and a few strong few-shot baselines.
Although text recognition has significantly evolved over the years, state-of the-art (SOTA) models still struggle in the wild scenarios due to complex backgrounds, varying fonts, uncontrolled illuminations, distortions and other artifacts. This is because such models solely depend on visual information for text recognition, thus lacking semantic reasoning capabilities. In this paper, we argue that semantic information offers a complementary role in addition to visual only. More specifically, we additionally utilize semantic information by proposing a multi-stage multi-scale attentional decoder that performs joint visual-semantic reasoning. Our novelty lies in the intuition that for text recognition, prediction should be refined in a stage-wise manner. Therefore our key contribution is in designing a stage-wise unrolling attentional decoder where non-differentiability, invoked by discretely predicted character labels, needs to be bypassed for end-to-end training. While the first stage predicts using visual features, subsequent stages refine on-top of it using joint visual-semantic information. Additionally, we introduce multi-scale 2D attention along with dense and residual connections between different stages to deal with varying scales of character sizes, for better performance and faster convergence during training. Experimental results show our approach to outperform existing SOTA methods by a considerable margin.
The key challenge in designing a sketch representation lies with handling the abstract and iconic nature of sketches. Existing work predominantly utilizes either, (i) a pixelative format that treats sketches as natural images employing off-the-shelf CNN-based networks, or (ii) an elaborately designed vector format that leverages the structural information of drawing orders using sequential RNN-based methods. While the pixelative format lacks intuitive exploitation of structural cues, sketches in vector format are absent in most cases limiting their practical usage. Hence, in this paper, we propose a lattice structured sketch representation that not only removes the bottleneck of requiring vector data but also preserves the structural cues that vector data provides. Essentially, sketch lattice is a set of points sampled from the pixelative format of the sketch using a lattice graph. We show that our lattice structure is particularly amenable to structural changes that largely benefits sketch abstraction modeling for generation tasks. Our lattice representation could be effectively encoded using a graph model, that uses significantly fewer model parameters (13.5 times lesser) than existing state-of-the-art. Extensive experiments demonstrate the effectiveness of sketch lattice for sketch manipulation, including sketch healing and image-to-sketch synthesis.
We scrutinise an important observation plaguing scene-level sketch research - that a significant portion of scene sketches are "partial". A quick pilot study reveals: (i) a scene sketch does not necessarily contain all objects in the corresponding photo, due to the subjective holistic interpretation of scenes, (ii) there exists significant empty (white) regions as a result of object-level abstraction, and as a result, (iii) existing scene-level fine-grained sketch-based image retrieval methods collapse as scene sketches become more partial. To solve this "partial" problem, we advocate for a simple set-based approach using optimal transport (OT) to model cross-modal region associativity in a partially-aware fashion. Importantly, we improve upon OT to further account for holistic partialness by comparing intra-modal adjacency matrices. Our proposed method is not only robust to partial scene-sketches but also yields state-of-the-art performance on existing datasets.
Sketches are highly expressive, inherently capturing subjective and fine-grained visual cues. The exploration of such innate properties of human sketches has, however, been limited to that of image retrieval. In this paper, for the first time, we cultivate the expressiveness of sketches but for the fundamental vision task of object detection. The end result is a sketch-enabled object detection framework that detects based on what you sketch - that "zebra" (e.g., one that is eating the grass) in a herd of zebras (instance-aware detection), and only the part (e.g., "head" of a "zebra") that you desire (part-aware detection). We further dictate that our model works without (i) knowing which category to expect at testing (zero-shot) and (ii) not requiring additional bounding boxes (as per fully supervised) and class labels (as per weakly supervised). Instead of devising a model from the ground up, we show an intuitive synergy between foundation models (e.g., CLIP) and existing sketch models build for sketch-based image retrieval (SBIR), which can already elegantly solve the task - CLIP to provide model generalisation, and SBIR to bridge the (sketch→photo) gap. In particular, we first perform independent prompting on both sketch and photo branches of an SBIR model to build highly generalisable sketch and photo encoders on the back of the generalisation ability of CLIP. We then devise a training paradigm to adapt the learned encoders for object detection, such that the region embeddings of detected boxes are aligned with the sketch and photo embeddings from SBIR. Evaluating our framework on standard object detection datasets like PASCAL-VOC and MS-COCO outperforms both supervised (SOD) and weakly-supervised object detectors (WSOD) on zero-shot setups. Project Page: https://pinakinathc.github.io/sketch-detect
This paper advances the fine-grained sketch-based image retrieval (FG-SBIR) literature by putting forward a strong baseline that overshoots prior state-of-the-arts by ≈11 %. This is not via complicated design though, but by addressing two critical issues facing the community (i) the gold standard triplet loss does not enforce holistic latent space geometry, and (ii) there are never enough sketches to train a high accuracy model. For the former, we propose a simple modification to the standard triplet loss, that explicitly enforces separation amongst photos/sketch instances. For the latter, we put forward a novel knowledge distillation module can leverage photo data for model training. Both modules are then plugged into a novel plug-n-playable training paradigm that allows for more stable training. More specifically, for (i) we employ an intra-modal triplet loss amongst sketches to bring sketches of the same instance closer from others, and one more amongst photos to push away different photo instances while bringing closer a structurally augmented version of the same photo (offering a gain of ≈4-6%). To tackle (ii), we first pre-train a teacher on the large set of unlabelled photos over the aforementioned intra-modal photo triplet loss. Then we distill the contextual similarity present amongst the instances in the teacher's embedding space to that in the student's embedding space, by matching the distribution over inter-feature distances of respective samples in both embedding spaces (delivering a further gain of ≈ 4-5%). Apart from outperforming prior arts significantly, our model also yields satisfactory results on generalising to new classes. Project page: https://aneeshan95.github.io/Sketch_PVT/
Human sketch has already proved its worth in various visual understanding tasks (e.g., retrieval, segmentation, image-captioning, etc). In this paper, we reveal a new trait of sketches - that they are also salient. This is intuitive as sketching is a natural attentive process at its core. More specifically, we aim to study how sketches can be used as a weak label to detect salient objects present in an image. To this end, we propose a novel method that emphasises on how "salient object" could be explained by hand-drawn sketches. To accomplish this, we introduce a photo-to-sketch generation model that aims to generate sequential sketch coordinates corresponding to a given visual photo through a 2D attention mechanism. Attention maps accumulated across the time steps give rise to salient regions in the process. Extensive quantitative and qualitative experiments prove our hypothesis and delineate how our sketch-based saliency detection model gives a competitive performance compared to the state-of-the-art.
Given an abstract, deformed, ordinary sketch from untrained amateurs like you and me, this paper turns it into a photorealistic image - just like those shown in Fig. 1(a), all non-cherry-picked. We differ significantly from prior art in that we do not dictate an edgemap-like sketch to start with, but aim to work with abstract free-hand human sketches. In doing so, we essentially democratise the sketch-to-photo pipeline, "picturing" a sketch regardless of how good you sketch. Our contribution at the outset is a decoupled encoder-decoder training paradigm, where the decoder is a StyleGAN trained on photos only. This importantly ensures that generated results are always photorealistic. The rest is then all centred around how best to deal with the abstraction gap between sketch and photo. For that, we propose an autoregressive sketch mapper trained on sketch-photo pairs that maps a sketch to the StyleGAN latent space. We further introduce specific designs to tackle the abstract nature of human sketches, including a fine-grained discriminative loss on the back of a trained sketch-photo retrieval model, and a partial-aware sketch augmentation strategy. Finally, we showcase a few downstream tasks our generation model enables, amongst them is showing how fine-grained sketch-based image retrieval, a well-studied problem in the sketch community, can be reduced to an image (generated) to image retrieval task, surpassing state-of-the-arts. We put forward generated results in the supplementary for everyone to scrutinise. Project page: https://subhadeepkoley.github.io/PictureThatSketch
In this paper, we leverage CLIP for zero-shot sketch based image retrieval (ZS-SBIR). We are largely inspired by recent advances on foundation models and the unparalleled generalisation ability they seem to offer, but for the first time tailor it to benefit the sketch community. We put forward novel designs on how best to achieve this synergy, for both the category setting and the fine-grained setting ('all"}. At the very core of our solution is a prompt learning setup. First we show just via factoring in sketch-specific prompts, we already have a category-level ZS-SBIR system that over-shoots all prior arts, by a large margin (24.8%) - a great testimony on studying the CLIP and ZS-SBIR synergy. Moving onto the fine-grained setup is however trickier, and re-quires a deeper dive into this synergy. For that, we come up with two specific designs to tackle the fine-grained matching nature of the problem: (i) an additional regularisation loss to ensure the relative separation between sketches and photos is uniform across categories, which is not the case for the gold standard standalone triplet loss, and (ii) a clever patch shuffling technique to help establishing instance-level structural correspondences between sketch-photo pairs. With these designs, we again observe signifi-cant performance gains in the region of 26.9% over previ-ous state-of-the-art. The take-home message, if any, is the proposed CLIP and prompt learning paradigm carries great promise in tackling other sketch-related tasks (not limited to ZS-SBIR) where data scarcity remains a great challenge. Project page: https://aneeshan95.github.ioISketchLVM/
In this paper, we extend scene understanding to include that of human sketch. The result is a complete trilogy of scene representation from three diverse and complementary modalities - sketch, photo, and text. Instead of learning a rigid three-way embedding and be done with it, wefocus on learning a flexible joint embedding that fully supports the "optionality" that this complementarity brings. Our embedding supports optionality on two axes: (i) optionality across modalities - use any combination of modalities as query for downstream tasks like retrieval, (ii) optionality across tasks - simultaneously utilising the embedding for either discriminative (e.g., retrieval) or generative tasks (e.g., captioning). This provides flexibility to end-users by exploiting the best of each modality, therefore serving the very purpose behind our proposal of a trilogy in the first place. First, a combination of information-bottleneck and conditional invertible neural networks disentangle the modality-specific component from modality-agnostic in sketch, photo, and text. Second, the modality-agnostic instances from sketch, photo, and text are synergised using a modified cross-attention. Once learned, we show our embedding can accommodate a multi-facet of scene-related tasks, including those enabled for the first time by the inclusion of sketch, all without any task-specific modifications. Project Page: https://pinakinathc.github.io/scenetrilogy
Generalized Few-shot Semantic Segmentation (GFSS) aims to segment each image pixel into either base classes with abundant training examples or novel classes with only a handful of (e.g., 1-5) training images per class. Compared to the widely studied Few-shot Semantic Segmentation (FSS), which is limited to segmenting novel classes only, GFSS is much under-studied despite being more practical. Existing approach to GFSS is based on classifier parameter fusion whereby a newly trained novel class classifier and a pre-trained base class classifier are combined to form a new classifier. As the training data is dominated by base classes, this approach is inevitably biased towards the base classes. In this work, we propose a novel Prediction Calibration Network (PCN) to address this problem. Instead of fusing the classifier parameters, we fuse the scores produced separately by the base and novel classifiers. To ensure that the fused scores are not biased to either the base or novel classes, a new Transformer-based calibration module is introduced. It is known that the lower-level features are useful of detecting edge information in an input image than higher level features. Thus, we build a cross-attention module that guides the classifier's final prediction using the fused multi-level features. However, transformers are computationally demanding. Crucially, to make the proposed cross-attention module training tractable at the pixel level, this module is designed based on feature-score cross-covariance and episodically trained to be generalizable at inference time. Extensive experiments on PASCAL-5(i) and COCO-20(i) show that our PCN outperforms the state-the-the-art alternatives by large margins.
A fundamental challenge faced by existing Fine-Grained Sketch-Based Image Retrieval (FG-SBIR) models is the data scarcity – model performances are largely bottlenecked by the lack of sketch-photo pairs. Whilst the number of photos can be easily scaled, each corresponding sketch still needs to be individually produced. In this paper, we aim to mitigate such an upper-bound on sketch data, and study whether unlabelled photos alone (of which they are many) can be cultivated for performance gain. In particular, we introduce a novel semi-supervised framework for cross-modal retrieval that can additionally leverage large-scale unla-belled photos to account for data scarcity. At the center of our semi-supervision design is a sequential photo-to-sketch generation model that aims to generate paired sketches for unlabelled photos. Importantly, we further introduce a discriminator-guided mechanism to guide against unfaithful generation, together with a distillation loss-based regu-larizer to provide tolerance against noisy training samples. Last but not least, we treat generation and retrieval as two conjugate problems, where a joint learning procedure is devised for each module to mutually benefit from each other. Extensive experiments show that our semi-supervised model yields a significant performance boost over the state-of-the-art supervised alternatives, as well as existing methods that can exploit unlabelled photos for FG-SBIR.
A common strategy adopted by existing state-of-the-art unsupervised domain adaptation (UDA) methods is to employ two classifiers to identify the misaligned local regions between source and target domain. Following the 'wisdom of the crowd' principle, one has to ask: why stop at two? Indeed, we find that using more classifiers leads to better performance, but also introduces more model parameters, therefore risking overfitting. In this paper, we introduce a novel method called STochastic clAssifieRs (STAR) for addressing this problem. Instead of representing one classifier as a weight vector, STAR models it as a Gaussian distribution with its variance representing the inter-classifier discrepancy. With STAR, we can now sample an arbitrary number of classifiers from the distribution, whilst keeping the model size the same as having two classifiers. Extensive experiments demonstrate that a variety of existing UDA methods can greatly benefit from STAR and achieve the state-of-the-art performance on both image classification and semantic segmentation tasks.
We present the first competitive drawing agent Pixelor that exhibits human-level performance at a Pictionary-like sketching game, where the participant whose sketch is recognized first is a winner. Our AI agent can autonomously sketch a given visual concept, and achieve a recognizable rendition as quickly or faster than a human competitor. The key to victory for the agent’s goal is to learn the optimal stroke sequencing strategies that generate the most recognizable and distinguishable strokes first. Training Pixelor is done in two steps. First, we infer the stroke order that maximizes early recognizability of human training sketches. Second, this order is used to supervise the training of a sequence-to-sequence stroke generator. Our key technical contributions are a tractable search of the exponential space of orderings using neural sorting; and an improved Seq2Seq Wasserstein (S2S-WAE) generator that uses an optimal-transport loss to accommodate the multi-modal nature of the optimal stroke distribution. Our analysis shows that Pixelor is better than the human players of the Quick, Draw! game, under both AI and human judging of early recognition. To analyze the impact of human competitors’ strategies, we conducted a further human study with participants being given unlimited thinking time and training in early recognizability by feedback from an AI judge. The study shows that humans do gradually improve their strategies with training, but overall Pixelor still matches human performance. The code and the dataset are available at http://sketchx.ai/pixelor.
Existing sketch-analysis work studies sketches depicting static objects or scenes. In this work, we propose a novel cross-modal retrieval problem of fine-grained instance-level sketch-based video retrieval (FG-SBVR), where a sketch sequence is used as a query to retrieve a specific target video instance. Compared with sketch-based still image retrieval, and coarse-grained category-level video retrieval, this is more challenging as both visual appearance and motion need to be simultaneously matched at a fine-grained level. We contribute the first FG-SBVR dataset with rich annotations. We then introduce a novel multi-stream multi-modality deep network to perform FG-SBVR under both strong and weakly supervised settings. The key component of the network is a relation module, designed to prevent model overfitting given scarce training data. We show that this model significantly outperforms a number of existing state-of-the-art models designed for video analysis.
Deep image-based modeling has received a lot of attention in recent years. Sketch-based modeling in particular has gained popularity given the ubiquitous nature of touchscreen devices. In this paper, we (i) study and compare diverse single-image reconstruction methods on sketch input, comparing the different 3D shape representations: multi-view, voxel- and point-cloud-based, mesh-based and implicit ones; and (ii) analyze the main challenges and requirements of sketch-based modeling systems. We introduce the regression loss and provide two variants of its formulation for the two most promising 3D shape representations: point clouds and signed distance functions. We show that this loss can increase general reconstruction accuracy, and the view- and style-robustness of the reconstruction methods. Moreover, we demonstrate that this loss can benefit the disentanglement of latent space to view invariant and view-specific information, resulting in further improved performance. To address the figure-ground ambiguity typical for sparse freehand sketches, we propose a two-branch architecture that exploits sparse user labeling. We hope that our work will inform future research on sketch-based modeling.
In this paper we study, for the first time, the problem of fine-grained sketch-based 3D shape retrieval. We advocate the use of sketches as a fine-grained input modality to retrieve 3D shapes at instance-level - e.g., given a sketch of a chair, we set out to retrieve a specific chair from a gallery of all chairs. Fine-grained sketch-based 3D shape retrieval (FG-SBSR) has not been possible till now due to a lack of datasets that exhibit one-to-one sketch-3D correspondences. The first key contribution of this paper is two new datasets, consisting a total of 4,680 sketch-3D pairings from two object categories. Even with the datasets, FG-SBSR is still highly challenging because (i) the inherent domain gap between 2D sketch and 3D shape is large, and (ii) retrieval needs to be conducted at the instance level instead of the coarse category level matching as in traditional SBSR. Thus, the second contribution of the paper is the first cross-modal deep embedding model for FG-SBSR, which specifically tackles the unique challenges presented by this new problem. Core to the deep embedding model is a novel cross-modal view attention module which automatically computes the optimal combination of 2D projections of a 3D shape given a query sketch.
3D shape modeling is labor-intensive, time-consuming, and requires years of expertise. To facilitate 3D shape modeling, we propose a 3D shape generation network that takes a 3D VR sketch as a condition. We assume that sketches are created by novices without art training and aim to reconstruct geometrically realistic 3D shapes of a given category. To handle potential sketch ambiguity, our method creates multiple 3D shapes that align with the original sketch’s structure. We carefully design our method, training the model step-by-step and leveraging multi-modal 3D shape representation to support training with limited training data. To guarantee the realism of generated 3D shapes we leverage the normalizing flow that models the distribution of the latent space of 3D shapes. To encourage the fidelity of the generated 3D shapes to an input sketch, we propose a dedicated loss that we deploy at different stages of the training process. The code is available at https://github.com/Rowl1ng/3Dsketch2shape.
We present a probabilistic approach for the automatic production of tree models with convincing 3D appearance and motion. The only input is a video of a moving tree that provides us an initial dynamic tree model, which is used to generate new individual trees of the same type. Our approach combines global and local constraints to construct a dynamic 3D tree model from a 2D skeleton. Our modeling takes into account factors such as the shape of branches, the overall shape of the tree, and physically plausible motion. Furthermore, we provide a generative model that creates multiple trees in 3D, given a single example model. This means that users no longer have to make each tree individually, or specify rules to make new trees. Results with different species are presented and compared to both reference input data and state of the art alternatives.
Abstract Free-hand sketch recognition has become increasingly popular due to the recent expansion of portable touchscreen devices. However, the problem is non-trivial due to the complexity of internal structures that leads to intra-class variations, coupled with the sparsity in visual cues that results in inter-class ambiguities. In order to address the structural complexity, a novel structured representation for sketches is proposed to capture the holistic structure of a sketch. Moreover, to overcome the visual cue sparsity problem and therefore achieve state-of-the-art recognition performance, we propose a Multiple Kernel Learning (MKL) framework for sketch recognition, fusing several features common to sketches. We evaluate the performance of all the proposed techniques on the most diverse sketch dataset to date (Mathias et al., 2012), and offer detailed and systematic analyses of the performance of different features and representations, including a breakdown by sketch-super-category. Finally, we investigate the use of attributes as a high-level feature for sketches and show how this complements low-level features for improving recognition performance under the MKL framework, and consequently explore novel applications such as attribute-based retrieval.
We introduce a simple but versatile camera model that we call the Rational Tensor Camera (RTcam). RTcams are well principled mathematically and provably subsume several important contemporary camera models in both computer graphics and vision; their generality Is one contribution. They can be used alone or compounded to produce more complicated visual effects. In this paper, we apply RTcams to generate synthetic artwork with novel perspective effects from real photographs. Existing Nonphotorealistic Rendering from Photographs (NPRP) is constrained to the projection inherent in the source photograph, which is most often linear. RTcams lift this restriction and so contribute to NPRP via multiperspective projection. This paper describes RTcams, compares them to contemporary alternatives, and discusses how to control them in practice. Illustrative examples are provided throughout.
Finding meaningful groupings of image primitives has been a long-standing problem in computer vision. This paper studies how salient groupings can be produced using established theories in the field of visual perception alone. The major contribution is a novel definition of the Gestalt principle of Prägnanz, based upon Koffka's definition that image descriptions should be both stable and simple. Our method is global in the sense that it operates over all primitives in an image at once. It works regardless of the type of image primitives and is generally independent of image properties such as intensity, color, and texture. A novel experiment is designed to quantitatively evaluate the groupings outputs by our method, which takes human disagreement into account and is generic to outputs of any grouper. We also demonstrate the value of our method in an image segmentation application and quantitatively show that segmentations deliver promising results when benchmarked using the Berkeley Segmentation Dataset (BSDS).
Visual tracking aims to match objects of interest in consecutive video frames. This paper proposes a novel and robust algorithm to address the problem of object tracking. To this end, we investigate the fusion of state-of-the-art image segmentation hierarchies and graph matching. More specifically, (i) we represent the object to be tracked using a hierarchy of regions, each of which is described with a combined feature set of SIFT descriptors and color histograms; (ii) we formulate the tracking process as a graph matching problem, which is solved by minimizing an energy function incorporating appearance and geometry contexts; and (iii) more importantly, an effective graph updating mechanism is proposed to adapt to the object changes over time for ensuring the tracking robustness. Experiments are carried out on several challenging sequences and results show that our method performs well in terms of object tracking, even in the presence of variations of scale and illumination, moving camera, occlusion, and background clutter.
The problem of fine-grained sketch-based image retrieval (FG-SBIR) is defined and investigated in this paper. In FG-SBIR, free-hand human sketch images are used as queries to retrieve photo images containing the same object instances. It is thus a cross-domain (sketch to photo) instance-level retrieval task. It is an extremely challenging problem because (i) visual comparisons and matching need to be executed under large domain gap, i.e., from black and white line drawing sketches to colour photos; (ii) it requires to capture the fine-grained (dis)similarities of sketches and photo images while free-hand sketches drawn by different people present different levels of deformation and expressive interpretation; and (iii) annotated cross-domain fine-grained SBIR datasets are scarce, challenging many state-of-the-art machine learning techniques, particularly those based on deep learning. In this paper, for the first time, we address all these challenges, providing a step towards the capabilities that would underpin a commercial sketch-based object instance retrieval application. Specifically, a new large-scale FG-SBIR database is introduced which is carefully designed to reflect the real-world application scenarios. A deep cross-domain matching model is then formulated to solve the intrinsic drawing style variability, large domain gap issues, and capture instance-level discriminative features. It distinguishes itself by a carefully designed attention module. Extensive experiments on the new dataset demonstrate the effectiveness of the proposed model and validate the need for a rigorous definition of the FG-SBIR problem and collecting suitable datasets.
With the increasing popularity of portable camera devices and embedded visual processing, text extraction from natural scene images has become a key problem that is deemed to change our everyday lives via novel applications such as augmented reality. Text extraction from natural scene images algorithms is generally composed of the following three stages: (i) detection and localization, (ii) text enhancement and segmentation and (iii) optical character recognition (OCR). The problem is challenging in nature due to variations in the font size and color, text alignment, illumination change and reflections. This paper aims to classify and assess the latest algorithms. More specifically, we draw attention to studies on the first two steps in the extraction process, since OCR is a well-studied area where powerful algorithms already exist. This paper offers to the researchers a link to public image database for the algorithm assessment of text extraction from natural scene images.
Effectively solving the problem of sketch generation, which aims to produce human-drawing-like sketches from real photographs, opens the door for many vision applications such as sketch-based image retrieval and non-photorealistic rendering. In this paper, we approach automatic sketch generation from a human visual perception perspective. Instead of gathering insights from photographs, for the first time, we extract information from a large pool of human sketches. In particular, we study how multiple Gestalt rules can be encapsulated into a unified perceptual grouping framework for sketch generation. We further show that by solving the problem of Gestalt confliction, i.e., encoding the relative importance of each rule, more similar to human-made sketches can be generated. For that, we release a manually labeled sketch dataset of 96 object categories and 7680 sketches. A novel evaluation framework is proposed to quantify human likeness of machine-generated sketches by examining how well they can be classified using models trained from human data. Finally, we demonstrate the superiority of our sketches under the practical application of sketch-based image retrieval. © 2015 Elsevier B.V.
Heterogeneous face recognition (HFR) refers to matching face imagery across different domains. It has received much interest from the research community as a result of its profound implications in law enforcement. A wide variety of new invariant features, cross-modality matching models and heterogeneous datasets are being established in recent years. This survey provides a comprehensive review of established techniques and recent developments in HFR. Moreover, we offer a detailed account of datasets and benchmarks commonly used for evaluation. We finish by assessing the state of the field and discussing promising directions for future research.
Wireless sensor networks are being increasingly accepted as an effective tool for structural health monitoring. The ability to deploy a wireless array of sensors efficiently and effectively is a key factor in structural health monitoring. Sensor installation and management can be difficult in practice for a variety of reasons: a hostile environment, high labour costs and bandwidth limitations. We present and evaluate a proof-of-concept application of virtual visual sensors to the well-known engineering problem of the cantilever beam, as a convenient physical sensor substitute for certain problems and environments. We demonstrate the effectiveness of virtual visual sensors as a means to achieve non-destructive evaluation. Major benefits of virtual visual sensors are its non-invasive nature, ease of installation and cost-effectiveness. The novelty of virtual visual sensors lies in the combination of marker extraction with visual tracking realised by modern computer vision algorithms. We demonstrate that by deploying a collection of virtual visual sensors on an oscillating structure, its modal shapes and frequencies can be readily extracted from a sequence of video images. Subsequently, we perform damage detection and localisation by means of a wavelet-based analysis. The contributions of this article are as follows: (1) use of a sub-pixel accuracy marker extraction algorithm to construct virtual sensors in the spatial domain, (2) embedding dynamic marker linking within a tracking-by-correspondence paradigm that offers benefits in computational efficiency and registration accuracy over traditional tracking-by-searching systems and (3) validation of virtual visual sensors in the context of a structural health monitoring application.
We present a generative model which can automatically summarize the stroke composition of free-hand sketches of a given category. When our model is fit to a collection of sketches with similar poses, it discovers and learns the structure and appearance of a set of coherent parts, with each part represented by a group of strokes. It represents both consistent (topology) as well as diverse aspects (structure and appearance variations) of each sketch category. Key to the success of our model are important insights learned from a comprehensive study performed on human stroke data. By fitting this model to images, we are able to synthesize visually similar and pleasant free-hand sketches. © 2016, The Author(s).
Facial expression is one of the most expressive ways to display human emotions. Facial expression analysis (FEA) has been broadly studied in the past decades. In our daily life, few of the facial expressions are exactly one of the predefined affective states but are blends of several basic expressions. Even though the concept of 'blended emotions' has been proposed years ago, most researchers did not deal with FEA as a multiple outputs problem yet. In this paper, multi-label learning algorithm for FEA is proposed to solve this problem. Firstly, to depict facial expressions more effectively, we model FEA as a multi-label problem, which depicts all facial expressions with multiple continuous values and labels of predefined affective states. Secondly, in order to model FEA jointly with multiple outputs, multi-label Group Lasso regularized maximum margin classifier (GLMM) and Group Lasso regularized regression (GLR) algorithms are proposed which can analyze all facial expressions at one time instead of modeling as a binary learning problem. Thirdly, to improve the effectiveness of our proposed model used in video sequences, GLR is further extended to be a Total Variation and Group Lasso based regression model (GLTV) which adds a prior term (Total Variation term) in the original model. JAFFE dataset and the extended Cohn Kanade (CK+) dataset have been used to verify the superior performance of our approaches with common used criterions in multi-label classification and regression realms.
This paper shows that classifying shapes is a tool useful in nonphotorealistic rendering (NPR) from photographs. Our classifier inputs regions from an image segmentation hierarchy and outputs the 'best” fitting simple shape such as a circle, square, or triangle. Other approaches to NPR have recognized the benefits of segmentation, but none have classified the shape of segments. By doing so, we can create artwork of a more abstract nature, emulating the style of modern artists such as Matisse and other artists who favored shape simplification in their artwork. The classifier chooses the shape that 'best” represents the region. Since the classifier is trained by a user, the 'best shape” has a subjective quality that can over-ride measurements such as minimum error and more importantly captures user preferences. Once trained, the system is fully automatic, although simple user interaction is also possible to allow for differences in individual tastes. A gallery of results shows how this classifier contributes to NPR from images by producing abstract artwork.
We propose a deep learning approach to free-hand sketch recognition that achieves state-of-the-art performance, significantly surpassing that of humans. Our superior performance is a result of modelling and exploiting the unique characteristics of free-hand sketches, i.e., consisting of an ordered set of strokes but lacking visual cues such as colour and texture, being highly iconic and abstract, and exhibiting extremely large appearance variations due to different levels of abstraction and deformation. Specifically, our deep neural network, termed Sketch-a-Net has the following novel components: (i) we propose a network architecture designed for sketch rather than natural photo statistics. (ii) Two novel data augmentation strategies are developed which exploit the unique sketch-domain properties to modify and synthesise sketch training data at multiple abstraction levels. Based on this idea we are able to both significantly increase the volume and diversity of sketches for training, and address the challenge of varying levels of sketching detail commonplace in free-hand sketches. (iii) We explore different network ensemble fusion strategies, including a re-purposed joint Bayesian scheme, to further improve recognition performance. We show that state-of-the-art deep networks specifically engineered for photos of natural objects fail to perform well on sketch recognition, regardless whether they are trained using photos or sketches. Furthermore, through visualising the learned filters, we offer useful insights in to where the superior performance of our network comes from. © 2016, Springer Science+Business Media New York.
We propose a deep learning approach to free-hand sketch recognition that achieves state-of-the-art performance, significantly surpassing that of humans. Our superior performance is a result of modelling and exploiting the unique characteristics of free-hand sketches, i.e., consisting of an ordered set of strokes but lacking visual cues such as colour and texture, being highly iconic and abstract, and exhibiting extremely large appearance variations due to different levels of abstraction and deformation. Specifically, our deep neural network, termed Sketch-a-Net has the following novel components: (i) we propose a network architecture designed for sketch rather than natural photo statistics. (ii) Two novel data augmentation strategies are developed which exploit the unique sketch-domain properties to modify and synthesise sketch training data at multiple abstraction levels. Based on this idea we are able to both significantly increase the volume and diversity of sketches for training, and address the challenge of varying levels of sketching detail commonplace in free-hand sketches. (iii) We explore different network ensemble fusion strategies, including a re-purposed joint Bayesian scheme, to further improve recognition performance. We show that state-of-the-art deep networks specifically engineered for photos of natural objects fail to perform well on sketch recognition, regardless whether they are trained using photos or sketches. Furthermore, through visualising the learned filters, we offer useful insights in to where the superior performance of our network comes from. © 2016, Springer Science+Business Media New York.
Computer vision is an interdisciplinary field that deals with how computers can be made to gain high-level understanding from digital images or videos. From the perspective of engineering, it seeks to automate tasks that the human visual system can do. As a scientific discipline, computer vision is about the theory behind artificial systems that extracts information from images. With the emergence of big vision data and the development of artificial intelligence, it is important to investigate new theories, methods, and applications in computer vision.
We study the problem of fine-grained sketch-based image retrieval. By performing instance-level (rather than category-level) retrieval, it embodies a timely and practical application, particularly with the ubiquitous availability of touchscreens. Three factors contribute to the challenging nature of the problem: 1) free-hand sketches are inherently abstract and iconic, making visual comparisons with photos difficult; 2) sketches and photos are in two different visual domains, i.e., black and white lines versus color pixels; and 3) fine-grained distinctions are especially challenging when executed across domain and abstraction-level. To address these challenges, we propose to bridge the image-sketch gap both at the high level via parts and attributes, as well as at the low level via introducing a new domain alignment method. More specifically, first, we contribute a data set with 304 photos and 912 sketches, where each sketch and image is annotated with its semantic parts and associated part-level attributes. With the help of this data set, second, we investigate how strongly supervised deformable part-based models can be learned that subsequently enable automatic detection of part-level attributes, and provide pose-aligned sketch-image comparisons. To reduce the sketch-image gap when comparing low-level features, third, we also propose a novel method for instance-level domain-alignment that exploits both subspace and instance-level cues to better align the domains. Finally, fourth, these are combined in a matching framework integrating aligned low-level features, mid-level geometric structure, and high-level semantic attributes. Extensive experiments conducted on our new data set demonstrate effectiveness of the proposed method.
Sketch-based image retrieval (SBIR) is challenging due to the inherent domain-gap between sketch and photo. Compared with pixel-perfect depictions of photos, sketches are iconic renderings of the real world with highly abstract. Therefore, matching sketch and photo directly using low-level visual clues are insufficient, since a common low-level subspace that traverses semantically across the two modalities is non-trivial to establish. Most existing SBIR studies do not directly tackle this cross-modal problem. This naturally motivates us to explore the effectiveness of cross-modal retrieval methods in SBIR, which have been applied in the image-text matching successfully. In this paper, we introduce and compare a series of state-of-the-art cross-modal subspace learning methods and benchmark them on two recently released fine-grained SBIR datasets. Through thorough examination of the experimental results, we have demonstrated that the subspace learning can effectively model the sketch-photo domain-gap. In addition we draw a few key insights to drive future research. © 2017 Elsevier B.V.
In this paper, we develop a novel variational Bayesian learning method for the Dirichlet process (DP) mixture of the inverted Dirichlet distributions, which has been shown to be very flexible for modeling vectors with positive elements. The recently proposed extended variational inference (EVI) framework is adopted to derive an analytically tractable solution. The convergency of the proposed algorithm is theoretically guaranteed by introducing single lower bound approximation to the original objective function in the EVI framework. In principle, the proposed model can be viewed as an infinite inverted Dirichlet mixture model that allows the automatic determination of the number of mixture components from data. Therefore, the problem of predetermining the optimal number of mixing components has been overcome. Moreover, the problems of overfitting and underfitting are avoided by the Bayesian estimation approach. Compared with several recently proposed DP-related methods and conventional applied methods, the good performance and effectiveness of the proposed method have been demonstrated with both synthesized data and real data evaluations.
Human free-hand sketches provide the useful data for studying human perceptual grouping, where the grouping principles such as the Gestalt laws of grouping are naturally in play during both the perception and sketching stages. In this paper, we make the first attempt to develop a universal sketch perceptual grouper. That is, a grouper that can be applied to sketches of any category created with any drawing style and ability, to group constituent strokes/segments into semantically meaningful object parts. The first obstacle to achieving this goal is the lack of large-scale datasets with grouping annotation. To overcome this, we contribute the largest sketch perceptual grouping dataset to date, consisting of 20 000 unique sketches evenly distributed over 25 object categories. Furthermore, we propose a novel deep perceptual grouping model learned with both generative and discriminative losses. The generative loss improves the generalization ability of the model, while the discriminative loss guarantees both local and global grouping consistency. Extensive experiments demonstrate that the proposed grouper significantly outperforms the state-of-the-art competitors. In addition, we show that our grouper is useful for a number of sketch analysis tasks, including sketch semantic segmentation, synthesis, and fine-grained sketch-based image retrieval. © 1992-2012 IEEE.
For structural health monitoring applications there is a need for simple and contact-less methods of Non-Destructive Evaluation (NDE). A number of damage detection techniques have been developed, such as frequency shift, generalised fractal dimension and wavelet transforms with the aim to identify, locate and determine the severity of damage in a material or structure. These techniques are often tailored for factors such as (i) type of material, (ii) damage pattern (crack, delamination), and (iii) the nature of any input signals (space and time). This paper describes and evaluates a wavelet-based damage detection framework that locates damage on cantilevered beams via NDE using computer vision technologies. The novelty of the approach is the use of computer vision algorithms for the contact-less acquisition of modal shapes. Using the proposed method, the modal shapes of cantilever beams are reconstructed by extracting markers using sub-pixel Hough Transforms from images captured using conventional slow motion cameras. The extracted modal shapes are then used as an input for wavelet transform damage detection, exploiting both discrete and continuous variants. The experimental results are verified and compared against finite element analysis. The methodology enables a non-invasive damage detection system that avoids the need for expensive equipment or the attachment of sensors to the structure. Two types of damage are investigated in our experiments: (i) defects induced by removing material to reduce the stiffness of a steel beam and (ii) delaminations in a (0/90/0/90/0)s composite laminate. Results show successful detection of notch depths of 5%, 28% and 50% for the steel beam and of 30 mm delaminations in central and outer layers for the composite laminate.
In this paper, we study the problem of multi-view sketch correspondence, where we take as input multiple freehand sketches with different views of the same object and predict as output the semantic correspondence among the sketches. This problem is challenging since the visual features of corresponding points at different views can be very different. To this end, we take a deep learning approach and learn a novel local sketch descriptor from data. We contribute a training dataset by generating the pixel-level correspondence for the multi-view line drawings synthesized from 3D shapes. To handle the sparsity and ambiguity of sketches, we design a novel multi-branch neural network that integrates a patch-based representation and a multiscale strategy to learn the pixel-level correspondence among multi-view sketches. We demonstrate the effectiveness of our proposed approach with extensive experiments on hand-drawn sketches and multi-view line drawings rendered from multiple 3D shape datasets.
With the widespread explosion of sensing and computing, an increasing number of industrial applications and an ever-growing amount of academic research generate massive multi-modal data from multiple sources. Gaussian distribution is the probability distribution ubiquitously used in statistics, signal processing, and pattern recognition. However, in reality data are neither always Gaussian nor can be safely assumed to be Gaussian disturbed. In many real-life applications, the distribution of data is, e.g., bounded, asymmetric, and, therefore, is not Gaussian distributed. It has been found in recent studies that explicitly utilizing the non-Gaussian characteristics of data (e.g., data with bounded support, data with semi-bounded support, and data with L1/L2-norm constraint) can significantly improve the performance of practical systems. Hence, it is of particular importance and interest to make thorough studies of the non-Gaussian data and the corresponding non-Gaussian statistical models (e.g., beta distribution for bounded support data, gamma distribution for semi-bounded support data, and Dirichlet/vMF distribution for data with L1/L2-norm constraint). In order to analyze and understand such kind of non-Gaussian distributed data, the developments of related learning theories, statistical models, and efficient algorithms become crucial. The scope of this special issue of the Elsevier's Journal on Neurocomputing is to provide theoretical foundations and ground-breaking models and algorithms to solve this challenge.
Recently, recognition of online handwritten mathe- matical expression has been greatly improved by employing encoder-decoder based methods. Existing encoder-decoder models use string decoders to generate LaTeX strings for mathematical expression recognition. However, in this paper, we importantly argue that string representations might not be the most natural for mathematical expressions - mathematical expressions are inherently tree structures other than flat strings. For this purpose, we propose a novel sequential relation decoder (SRD) that aims to decode expressions into tree structures for online handwritten mathematical expression recognition. At each step of tree construction, a sub-tree structure composed of a relation node and two symbol nodes is computed based on previous sub-tree structures. This is the first work that builds a tree structure based decoder for encoder-decoder based mathematical expression recognition. Compared with string decoders, a decoder that better understands tree structures is crucial for mathematical expression recognition as it brings a more reasonable learning objective and improves overall generalization ability. We demonstrate how the proposed SRD outperforms state-of-the-art string decoders through a set of experiments on CROHME database, which is currently the largest benchmark for online handwritten mathematical expression recognition.
Categorizing free-hand human sketches has profound implications in applications such as human computer interaction and image retrieval. The task is non-trivial due to the iconic nature of sketches, signified by large variances in both appearance and structure when compared with photographs. Despite recent advances made by deep learning methods, the requirement of a large training set is commonly imposed making them impractical for real-world applications where training sketches are cumbersome to obtain - sketches have to be hand-drawn one by one other than crawled freely on the Internet. In this work, we aim to delve further into the data scarcity problem of sketch-related research, by proposing a few-shot sketch classification framework. The model is based on a co-regularized embedding algorithm where common/shareable parts of learned human sketches are exploited, thereby can embed query sketch into a co-regularized sparse representation space for few-shot classification. A new dataset of 8,000 part-level annotated sketches of 100 categories is also proposed to facilitate future research. Experiment shows that our approach can achieve an 5-way one-shot classification accuracy of 85%, and 20-way one-shot at 51%.
Sketch as an image search query is an ideal alternative to text in capturing the finegrained visual details. Prior successes on fine-grained sketch-based image retrieval (FGSBIR) have demonstrated the importance of tackling the unique traits of sketches as opposed to photos, e.g., temporal vs. static, strokes vs. pixels, and abstract vs. pixelperfect. In this paper, we study a further trait of sketches that has been overlooked to date, that is, they are hierarchical in terms of the levels of detail – a person typically sketches up to various extents of detail to depict an object. This hierarchical structure is often visually distinct. In this paper, we design a novel network that is capable of cultivating sketch-specific hierarchies and exploiting them to match sketch with photo at corresponding hierarchical levels. In particular, features from a sketch and a photo are enriched using cross-modal co-attention, coupled with hierarchical node fusion at every level to form a better embedding space to conduct retrieval. Experiments on common benchmarks show our method to outperform state-of-the-arts by a significant margin.
Domain shift refers to the well known problem that a model trained in one source domain performs poorly when applied to a target domain with different statistics. Domain Generalization (DG) techniques attempt to alleviate this issue by producing models which by design generalize well to novel testing domains. We propose a novel meta-learning method for domain generalization. Rather than designing a specific model that is robust to domain shift as in most previous DG work, we propose a model agnostic training procedure for DG. Our algorithm simulates train/test domain shift during training by synthesizing virtual testing domains within each mini-batch. The meta-optimization objective requires that steps to improve training domain performance should also improve testing domain performance. This meta-learning procedure trains models with good generalization ability to novel domains. We evaluate our method and achieve state of the art results on a recent cross-domain image classification benchmark, as well demonstrating its potential on two classic reinforcement learning tasks.
Sketch-based image retrieval (SBIR) is a cross-modal matching problem which is typically solved by learning a joint embedding space where the semantic content shared between photo and sketch modalities are preserved. However, a fundamental challenge in SBIR has been largely ignored so far, that is, sketches are drawn by humans and considerable style variations exist amongst different users. An effective SBIR model needs to explicitly account for this style diversity, crucially, to generalise to unseen user styles. To this end, a novel style-agnostic SBIR model is proposed. Different from existing models, a cross-modal variational autoencoder (VAE) is employed to explicitly disentangle each sketch into a semantic content part shared with the corresponding photo, and a style part unique to the sketcher. Importantly, to make our model dynamically adaptable to any unseen user styles, we propose to metatrain our cross-modal VAE by adding two style-adaptive components: a set of feature transformation layers to its encoder and a regulariser to the disentangled semantic content latent code. With this meta-learning framework, our model can not only disentangle the cross-modal shared semantic content for SBIR, but can adapt the disentanglement to any unseen user style as well, making the SBIR model truly style-agnostic. Extensive experiments show that our style-agnostic model yields state-of-the-art performance for both category-level and instance-level SBIR.
Perceptual organization remains one of the very few established theories on the human visual system. It underpinned many pre-deep seminal works on segmentation and detection, yet research has seen a rapid decline since the preferential shift to learning deep models. Of the limited attempts, most aimed at interpreting complex visual scenes using perceptual organizational rules. This has however been proven to be sub-optimal, since models were unable to effectively capture the visual complexity in real-world imagery. In this paper, we rejuvenate the study of perceptual organization, by advocating two positional changes: (i) we examine purposefully generated synthetic data, instead of complex real imagery, and (ii) we ask machines to synthesize novel perceptually-valid patterns, instead of explaining existing data. Our overall answer lies with the introduction of a novel visual challenge – the challenge of perceptual question answering (PQA). Upon observing example perceptual question-answer pairs, the goal for PQA is to solve similar questions by generating answers entirely from scratch (see Figure 1). Our first contribution is therefore the first dataset of perceptual question-answer pairs, each generated specifically for a particular Gestalt principle. We then borrow insights from human psychology to design an agent that casts perceptual organization as a self-attention problem, where a proposed grid-to-grid mapping network directly generates answer patterns from scratch. Experiments show our agent to outperform a selection of naive and strong baselines. A human study however indicates that ours uses astronomically more data to learn when compared to an average human, necessitating future research (with or without our dataset).
We study the practical task of fine-grained 3D-VRsketch-based 3D shape retrieval. This task is of particular interest as 2D sketches were shown to be effective queries for 2D images. However, due to the domain gap, it remains hard to achieve strong performance in 3D shape retrieval from 2D sketches. Recent work demonstrated the advantage of 3D VR sketching on this task. In our work, we focus on the challenge caused by inherent inaccuracies in 3D VR sketches. We observe that retrieval results obtained with a triplet loss with a fixed margin value, commonly used for retrieval tasks, contain many irrelevant shapes and often just one or few with a similar structure to the query. To mitigate this problem, we for the first time draw a connection between adaptive margin values and shape similarities. In particular, we propose to use a triplet loss with an adaptive margin value driven by a ‘fitting gap’, which is the similarity of two shapes under structure-preserving deformations. We also conduct a user study which confirms that this fitting gap is indeed a suitable criterion to evaluate the structural similarity of shapes. Furthermore, we introduce a dataset of 202 VR sketches for 202 3D shapes drawn from memory rather than from observation. The code and data are available at https://github.com/Rowl1ng/Structure-Aware-VR-Sketch-Shape-Retrieval
Whether what you see in Figure 1 is a " flamingo " or a " bird " , is the question we ask in this paper. While fine-grained visual classification (FGVC) strives to arrive at the former, for the majority of us non-experts just " bird " would probably suffice. The real question is therefore – how can we tailor for different fine-grained definitions under divergent levels of expertise. For that, we re-envisage the traditional setting of FGVC, from single-label classification , to that of top-down traversal of a pre-defined coarse-to-fine label hierarchy – so that our answer becomes " bird " ⇒ " Phoenicopteriformes " ⇒ " Phoenicopteridae " ⇒ " flamingo ". To approach this new problem, we first conduct a comprehensive human study where we confirm that most participants prefer multi-granularity labels, regardless whether they consider themselves experts. We then discover the key intuition that: coarse-level label prediction exacerbates fine-grained feature learning, yet fine-level feature betters the learning of coarse-level classifier. This discovery enables us to design a very simple albeit surprisingly effective solution to our new problem, where we (i) leverage level-specific classification heads to disentangle coarse-level features with fine-grained ones, and (ii) allow finer-grained features to participate in coarser-grained label predictions, which in turn helps with better disentanglement. Experiments show that our method achieves superior performance in the new FGVC setting, and performs better than state-of-the-art on the traditional single-label FGVC problem as well. Thanks to its simplicity, our method can be easily implemented on top of any existing FGVC frameworks and is parameter-free.
Self-supervised learning has gained prominence due to its efficacy at learning powerful representations from un-labelled data that achieve excellent performance on many challenging downstream tasks. However, supervision-free pretext tasks are challenging to design and usually modality specific. Although there is a rich literature of self-supervised methods for either spatial (such as images) or temporal data (sound or text) modalities, a common pretext task that benefits both modalities is largely missing. In this paper, we are interested in defining a self-supervised pretext task for sketches and handwriting data. This data is uniquely characterised by its existence in dual modalities of rasterized images and vector coordinate sequences. We address and exploit this dual representation by proposing two novel cross-modal translation pretext tasks for self-supervised feature learning: Vectorization and Rasteriza-tion. Vectorization learns to map image space to vector coordinates and rasterization maps vector coordinates to image space. We show that our learned encoder modules benefit both raster-based and vector-based downstream approaches to analysing hand-drawn data. Empirical evidence shows that our novel pretext tasks surpass existing single and multi-modal self-supervision methods.
Domain generalization (DG) is the challenging and topical problem of learning models that generalize to novel testing domains with different statistics than a set of known training domains. The simple approach of aggregating data from all source domains and training a single deep neural network end-to-end on all the data provides a surprisingly strong baseline that surpasses many prior published methods. In this paper we build on this strong baseline by designing an episodic training procedure that trains a single deep network in a way that exposes it to the domain shift that characterises a novel domain at runtime. Specifically, we decompose a deep network into feature extractor and classifier components, and then train each component by simulating it interacting with a partner who is badly tuned for the current domain. This makes both components more robust, ultimately leading to our networks producing state-of-the-art performance on three DG benchmarks. Furthermore, we consider the pervasive workflow of using an ImageNet trained CNN as a fixed feature extractor for downstream recognition tasks. Using the Visual Decathlon benchmark, we demonstrate that our episodic-DG training improves the performance of such a general purpose feature extractor by explicitly training a feature for robustness to novel problems. This shows that DG training can benefit standard practice in computer vision.
Existing Temporal Action Detection (TAD) methods typ- ically take a pre-processing step in converting an input varying-length video into a fixed-length snippet represen- tation sequence, before temporal boundary estimation and action classification. This pre-processing step would tem- porally downsample the video, reducing the inference res- olution and hampering the detection performance in the original temporal resolution. In essence, this is due to a temporal quantization error introduced during the resolu- tion downsampling and recovery. This could negatively im- pact the TAD performance, but is largely ignored by existing methods. To address this problem, in this work we intro- duce a novel model-agnostic post-processing method with- out model redesign and retraining. Specifically, we model the start and end points of action instances with a Gaussian distribution for enabling temporal boundary inference at a sub-snippet level. We further introduce an efficient Taylor- expansion based approximation, dubbed as Gaussian Ap- proximated Post-processing (GAP). Extensive experiments demonstrate that our GAP can consistently improve a wide variety of pre-trained off-the-shelf TAD models on the chal- lenging ActivityNet (+0.2%∼0.7% in average mAP) and THUMOS (+0.2%∼0.5% in average mAP) benchmarks. Such performance gains are already significant and highly comparable to those achieved by novel model designs. Also, GAP can be integrated with model training for further performance gain. Importantly, GAP enables lower tem- poral resolutions for more efficient inference, facilitating low-resource applications. The code will be available in https://github.com/sauradip/GAP
A layout to image (L2I) generation model aims to generate a complicated image containing multiple objects (things) against natural background (stuff), conditioned on a given layout. Built upon the recent advances in gen-erative adversarial networks (GANs), existing L2I models have made great progress. However, a close inspection of their generated images reveals two major limitations: (1) the object-to-object as well as object-to-stuff relations are often broken and (2) each object's appearance is typically distorted lacking the key defining characteristics associated with the object class. We argue that these are caused by the lack of context-aware object and stuff feature encoding in their generators, and location-sensitive appearance representation in their discriminators. To address these limitations, two new modules are proposed in this work. First, a context-aware feature transformation module is introduced in the generator to ensure that the generated feature encoding of either object or stuff is aware of other co-existing objects/stuff in the scene. Second, instead of feeding location-insensitive image features to the discriminator, we use the Gram matrix computed from the feature maps of the generated object images to preserve location-sensitive information, resulting in much enhanced object appearance. Extensive experiments show that the proposed method achieves state-of-the-art performance on the COCO-Thing-Stuff and Visual Genome benchmarks. Code available at: https://github.com/wtliao/layout2img.
Handwritten Text Recognition (HTR) remains a challenging problem to date, largely due to the varying writing styles that exist amongst us. Prior works however generally operate with the assumption that there is a limited number of styles, most of which have already been captured by existing datasets. In this paper, we take a completely different perspective – we work on the assumption that there is always a new style that is drastically different, and that we will only have very limited data during testing to perform adaptation. This creates a commercially viable solution – being exposed to the new style, the model has the best shot at adaptation, and the few-sample nature makes it practical to implement. We achieve this via a novel meta-learning framework which exploits additional new-writer data via a support set, and outputs a writer-adapted model via single gradient step update, all during inference (see Figure 1). We discover and leverage on the important insight that there exists few key characters per writer that exhibit relatively larger style discrepancies. For that, we additionally propose to meta-learn instance specific weights for a character-wise cross-entropy loss, which is specifically designed to work with the sequential nature of text data. Our writer-adaptive MetaHTR framework can be easily implemented on the top of most state-of-the-art HTR models. Experiments show an average performance gain of 5-7% can be obtained by observing very few new style data (≤ 16).
This paper, for the very first time, introduces human sketches to the landscape of XAI (Explainable Artificial Intelligence). We argue that sketch as a "human-centered" data form, represents a natural interface to study explainability. We focus on cultivating sketch-specific explainability designs. This starts by identifying strokes as a unique building block that offers a degree of flexibility in object construction and manipulation impossible in photos. Following this, we design a simple explainability-friendly sketch encoder that accommodates the intrinsic properties of strokes: shape, location, and order. We then define the first ever XAI task for sketch, that of stroke location inversion (SLI). Just as we have heat maps for photos and correlation matrices for text, SLI offers an explainability angle to sketch by asking a network how well it can recover stroke locations of an unseen sketch. We provide qualitative results for readers to interpret as snapshots of the SLI process in the paper and as GIFs on the project page. A minor but exciting note is that thanks to its sketch-specific design, our sketch encoder also yields the best sketch recognition accuracy to date while having the smallest number of parameters. The code is available at https://sketchxai.github.io.
FAME-ViL: Multi-Tasking Vision-Language Model for Heterogeneous Fashion Tasks Xiao Han1,2 Xiatian Zhu1,3 Licheng Yu Li Zhang4 Yi-Zhe Song1,2 Tao Xiang1,2 1 CVSSP, University of Surrey 2 iFlyTek-Surrey Joint Research Centre on Artificial Intelligence 3 Surrey Institute for People-Centred Artificial Intelligence 4 Fudan University {xiao.han, xiatian.zhu, y.song, t.xiang}@surrey.ac.uk lichengyu24@gmail.com lizhangfd@fudan.edu.cn Abstract In the fashion domain, there exists a variety of vision- and-language (V+L) tasks, including cross-modal retrieval, text-guided image retrieval, multi-modal classification, and image captioning. They differ drastically in each individ- ual input/output format and dataset size. It has been com- mon to design a task-specific model and fine-tune it in- dependently from a pre-trained V+L model (e.g., CLIP). This results in parameter inefficiency and inability to ex- ploit inter-task relatedness. To address such issues, we pro- pose a novel FAshion-focused Multi-task Efficient learn- ing method for Vision-and-Language tasks (FAME-ViL) in this work. Compared with existing approaches, FAME-ViL applies a single model for multiple heterogeneous fashion tasks, therefore being much more parameter-efficient. It is enabled by two novel components: (1) a task-versatile architecture with cross-attention adapters and task-specific adapters integrated into a unified V+L model, and (2) a sta- ble and effective multi-task training strategy that supports learning from heterogeneous data and prevents negative transfer. Extensive experiments on four fashion tasks show that our FAME-ViL can save 61.5% of parameters over alternatives, while significantly outperforming the conven- tional independently trained single-task models. Code is available at https://github.com/BrandonHanx/FAME-ViL
Given pixel-level annotated data, traditional photo segmentation techniques have achieved promising results. However, these photo segmentation models can only identify objects in categories for which data annotation and training have been carried out. This limitation has inspired recent work on few-shot and zero-shot learning for image segmentation. In this paper, we show the value of sketch for photo segmentation, in particular as a transferable representation to describe a concept to be segmented. We show, for the first time, that it is possible to generate a photo-segmentation model of a novel category using just a single sketch and furthermore exploit the unique fine-grained characteristics of sketch to produce more detailed segmentation. More specifically, we propose a sketch-based photo segmentation method that takes sketch as input and synthesizes the weights required for a neural network to segment the corresponding region of a given photo. Our framework can be applied at both the category-level and the instance-level, and fine-grained input sketches provide more accurate segmentation in the latter. This framework generalizes across categories via sketch and thus provides an alternative to zero-shot learning when segmenting a photo from a category without annotated training data. To investigate the instance-level relationship across sketch and photo, we create the SketchySeg dataset which contains segmentation annotations for photos corresponding to paired sketches in the Sketchy Dataset.
In this paper, for the first time, we investigate the problem of generating 3D shapes from professional 2D sketches via deep learning. We target sketches done by professional artists, as these sketches are likely to contain more details than the ones produced by novices, and thus the reconstruction from such sketches poses a higher demand on the level of detail in the reconstructed models. This is importantly different to previous work, where the training and testing was conducted on either synthetic sketches or sketches done by novices. Novices sketches often depict shapes that are physically unrealistic, while models trained with synthetic sketches could not cope with the level of abstraction and style found in real sketches. To address this problem, we collected the first large-scale dataset of professional sketches, where each sketch is paired with a reference 3D shape, with a total of 1,500 professional sketches collected across 500 3D shapes. The dataset is available at http://sketchx.ai/downloads/. We introduce two bespoke designs within a deep adversarial network to tackle the imprecision of human sketches and the unique figure/ground ambiguity problem inherent to sketch-based reconstruction. We show that existing 3D shapes generation methods designed for images fail to be naively applied to our problem, and demonstrate the effectiveness of our method both qualitatively and quantitatively.
Deep image-based modeling received lots of attention in recent years, yet the parallel problem of sketch-based modeling has only been briefly studied, often as a potential application. In this work, for the first time, we identify the main differences between sketch and image inputs: (i) style variance, (ii) imprecise perspective, and (iii) sparsity. We discuss why each of these differences can pose a challenge, and even make a certain class of image-based methods inapplicable. We study alternative solutions to address each of the difference. By doing so, we drive out a few important insights: (i) sparsity commonly results in an incorrect prediction of foreground versus background, (ii) diversity of human styles, if not taken into account, can lead to very poor generalization properties, and finally (iii) unless a dedicated sketching interface is used, one can not expect sketches to match a perspective of a fixed viewpoint. Finally, we compare a set of representative deep single-image modeling solutions and show how their performance can be improved to tackle sketch input by taking into consideration the identified critical differences.
Designing real and virtual garments is becoming extremely demanding with rapidly changing fashion trends and the increasing need for synthesizing realistically dressed digital humans for various applications. However, traditionally designing real and virtual garments has been time-consuming. Sketch-based modeling aims to bring the ease and immediacy of drawing to the 3D world thereby motivating faster iterations. We propose a novel sketch-based garment modeling framework specifically targeted to synchronize with the iterative process of garment ideation, e.g., adding or removing details from different views in each iteration. At the core of our learning-based approach is a view-aware feature aggregation module that fuses the features from the latest sketch with the thus far aggregated features to effectively refine the generated 3D shape. We evaluate our approach on a wide variety of garment types and iterative refinement scenarios. We also provide comparisons to alternative feature aggregation methods and demonstrate favorable results. The code is available at https://github.com/pinakinathc/multiviewsketch-garment.
Growing free online 3D shapes collections dictated research on 3D retrieval. Active debate has however been had on (i) what the best input modality is to trigger retrieval, and (ii) the ultimate usage scenario for such retrieval. In this paper, we offer a different perspective towards answering these questions - we study the use of 3D sketches as an input modality and advocate a VR-scenario where retrieval is conducted. Thus, the ultimate vision is that users can freely retrieve a 3D model by air-doodling in a VR environment. As a first stab at this new 3D VR-sketch to 3D shape retrieval problem, we make four contributions. First, we code a VR utility to collect 3D VR-sketches and conduct retrieval. Second, we collect the first set of 167 3D VRsketches on two shape categories from ModelNet. Third, we propose a novel approach to generate a synthetic dataset of human-like 3D sketches of different abstract levels to train deep networks. At last, we compare the common multi-view and volumetric approaches: We show that, in contrast to 3D shape to 3D shape retrieval, volumetric point-based approaches exhibit superior performance on 3D sketch to 3D shape retrieval due to the sparse and abstract nature of 3D VR-sketches. We believe these contributions will collectively serve as enablers for future attempts at this problem. The VR interface, code and datasets are available at https://tinyurl.com/3DSketch3DV.
Automatic data abstraction is an important capability for both benchmarking machine intelligence and supporting summarization applications. In the former one asks whether a machine can ‘understand’ enough about the meaning of input data to produce a meaningful but more compact abstraction. In the latter this capability is exploited for saving space or human time by summarizing the essence of input data. In this paper we study a general reinforcement learning based framework for learning to abstract sequential data in a goal-driven way. The ability to define different abstraction goals uniquely allows different aspects of the input data to be preserved according to the ultimate purpose of the abstraction. Our reinforcement learning objective does not require human-defined examples of ideal abstraction. Importantly our model processes the input sequence holistically without being constrained by the original input order. Our framework is also domain agnostic – we demonstrate applications to sketch, video and text data and achieve promising results in all domains.
Facial Expression Recognition (FER) techniques have already been adopted in numerous multimedia systems. Plenty of previous research assumes that each facial picture should be linked to only one of the predefined affective labels. Nevertheless, in practical applications, few of the expressions are exactly one of the predefined affective states. Therefore, to depict the facial expressions more accurately, this paper proposes a multi-label classification approach for FER and each facial expression would be labeled with one or multiple affective states. Meanwhile, by modeling the relationship between labels via Group Lasso regularization term, a maximum margin multi-label classifier is presented and the convex optimization formulation guarantees a global optimal solution. To evaluate the performance of our classifier, the JAFFE dataset is extended into a multi-label facial expression dataset by setting threshold to its continuous labels marked in the original dataset and the labeling results have shown that multiple labels can output a far more accurate description of facial expression. At the same time, the classification results have verified the superior performance of our algorithm.
We investigate whether it is possible to improve the performance of automated facial forensic sketch matching by learning from examples of facial forgetting over time. Forensic facial sketch recognition is a key capability for law enforcement, but remains an unsolved problem. It is extremely challenging because there are three distinct contributors to the domain gap between forensic sketches and photos: The well-studied sketch-photo modality gap, and the less studied gaps due to (i) the forgetting process of the eye-witness and (ii) their inability to elucidate their memory. In this paper, we address the memory problem head on by introducing a database of 400 forensic sketches created at different time-delays. Based on this database we build a model to reverse the forgetting process. Surprisingly, we show that it is possible to systematically 'un-forget' facial details. Moreover, it is possible to apply this model to dramatically improve forensic sketch recognition in practice: we achieve the state of the art results when matching 195 benchmark forensic sketches against corresponding photos and a 10,030 mugshot database.
Contemporary deep learning techniques have made image recognition a reasonably reliable technology. However training effective photo classifiers typically takes numerous examples which limits image recognition's scalability and applicability to scenarios where images may not be available. This has motivated investigation into zero-shot learning, which addresses the issue via knowledge transfer from other modalities such as text. In this paper we investigate an alternative approach of synthesizing image classifiers: Almost directly from a user's imagination, via freehand sketch. This approach doesn't require the category to be nameable or describable via attributes as per zero-shot learning. We achieve this via training a model regression network to map from free-hand sketch space to the space of photo classifiers. It turns out that this mapping can be learned in a category-agnostic way, allowing photo classifiers for new categories to be synthesized by user with no need for annotated training photos. We also demonstrate that this modality of classifier generation can also be used to enhance the granularity of an existing photo classifier, or as a complement to name-based zero-shot learning.
This paper addresses the problem of grouping image primitives; its principal contribution is an explicit definition of the Gestalt principle of Pragnanz, which organizes primitives into descriptions of images that are both simple and stable. Our definition of Pragnanz assumes just two things: that a vector of free variables controls some general grouping algorithm, and a scalar function measures the information in a grouping. Stable descriptions exist where the gradient of the function is zero, and these can be ordered by information content (simplicity) to create a "grouping" or "Gestalt" scale description. We provide a simple measure for information in a grouping based on its structure alone, leaving our grouper free to exploit other Gestalt principles as we see fit. We demonstrate the value of our definition of Pragnanz on several real-world images.
Categorizing free-hand human sketches has profound implications in applications such as human computer interaction and image retrieval. The task is non-trivial due to the iconic nature of sketches, signified by large variances in both appearance and structure when compared with photographs. One of the most fundamental problems is how to effectively describe a sketch image. Many existing descriptors, such as histogram of oriented gradients (HOG) and shape context (SC), have achieved great success. Moreover, some works have attempted to design features specifically engineered for sketches, such as symmetric-aware flip invariant sketch histogram (SYM-FISH). We present a novel patch-based sparse representation (PSR) for describing sketch image and it is evaluated under a sketch recognition framework. Extensive experiments on a large scale human drawn sketch dataset demonstrate the effectiveness of the proposed method.
We present the first fine-grained dataset of 1,497 3D VR sketch and 3D shape pairs of a chair category with large shapes diversity. Our dataset supports the recent trend in the sketch community on fine-grained data analysis, and extends it to an actively developing 3D domain. We argue for the most convenient sketching scenario where the sketch consists of sparse lines and does not require any sketching skills, prior training or time-consuming accurate drawing. We then, for the first time, study the scenario of fine-grained 3D VR sketch to 3D shape retrieval, as a novel VR sketching application and a proving ground to drive out generic insights to inform future research. By experimenting with carefully selected combinations of design factors on this new problem, we draw important conclusions to help follow-on work. We hope our dataset will enable other novel applications, especially those that require a fine-grained angle such as fine-grained 3D shape reconstruction. The dataset is available at tinyurl.com/ VRSketch3DV21.
Fine-grained sketch-based image retrieval (FG-SBIR) addresses matching specific photo instance using free-hand sketch as a query modality. Existing models aim to learn an embedding space in which sketch and photo can be directly compared. While successful, they require instance-level pairing within each coarse-grained category as annotated training data. Since the learned embedding space is domain-specific, these models do not generalise well across categories. This limits the practical applicability of FGSBIR. In this paper, we identify cross-category generalisation for FG-SBIR as a domain generalisation problem, and propose the first solution. Our key contribution is a novel unsupervised learning approach to model a universal manifold of prototypical visual sketch traits. This manifold can then be used to paramaterise the learning of a sketch/photo representation. Model adaptation to novel categories then becomes automatic via embedding the novel sketch in the manifold and updating the representation and retrieval function accordingly. Experiments on the two largest FG-SBIR datasets, Sketchy and QMUL-Shoe-V2, demonstrate the efficacy of our approach in enabling crosscategory generalisation of FG-SBIR.
We investigate the problem of fine-grained sketch-based image retrieval (SBIR), where free-hand human sketches are used as queries to perform instance-level retrieval of images. This is an extremely challenging task because (i) visual comparisons not only need to be fine-grained but also executed cross-domain, (ii) free-hand (finger) sketches are highly abstract, making fine-grained matching harder, and most importantly (iii) annotated cross-domain sketch-photo datasets required for training are scarce, challenging many state-of-the-art machine learning techniques. In this paper, for the first time, we address all these challenges, providing a step towards the capabilities that would underpin a commercial sketch-based image retrieval application. We introduce a new database of 1,432 sketchphoto pairs from two categories with 32,000 fine-grained triplet ranking annotations. We then develop a deep tripletranking model for instance-level SBIR with a novel data augmentation and staged pre-training strategy to alleviate the issue of insufficient fine-grained training data. Extensive experiments are carried out to contribute a variety of insights into the challenges of data sufficiency and over-fitting avoidance when training deep networks for finegrained cross-domain ranking tasks.
This paper demonstrates a new approach towards object recognition founded on the development of Neural Network classifiers and Bayesian Networks. The mapping from segmented image region descriptors to semantically meaningful class membership terms is achieved using Neural Networks. Bayesian Networks are then employed to probabilistically detect objects within an image by means of relating region class labels and their surrounding environments. Furthermore, it makes use of an intermediate level of image representation and demonstrates how object recognition can be achieved in this way.
Fine-grained sketch-based image retrieval (FG-SBIR) is a newly emerged topic in computer vision. The problem is challenging because in addition to bridging the sketch-photo domain gap, it also asks for instance-level discrimination within object categories. Most prior approaches focused on feature engineering and fine-grained ranking, yet neglected an important and central problem: how to establish a finegrained cross-domain feature space to conduct retrieval. In this paper, for the first time we formulate a cross-domain framework specifically designed for the task of FG-SBIR that simultaneously conducts instancelevel retrieval and attribute prediction. Different to conventional phototext cross-domain frameworks that performs transfer on category-level data, our joint multi-view space uniquely learns from the instance-level pair-wise annotations of sketch and photo. More specifically, we propose a joint view selection and attribute subspace learning algorithm to learn domain projection matrices for photo and sketch, respectively. It follows that visual attributes can be extracted from such matrices through projection to build a coupled semantic space to conduct retrieval. Experimental results on two recently released fine-grained photo-sketch datasets show that the proposed method is able to perform at a level close to those of deep models, while removing the need for extensive manual annotations.
This paper proposes a novel omni-image Interpolation technique and a new method to evaluation its performance. Omni-images are taken by non-linear catadioptric camera and offer important scientific and engineering benefits but often at the expense of the reduced visual accuracy. Interpolation algorithms aiming at improving the resolution of omni-images are deemed as an effective approach to deal with such a lack of visual content. Main contribution of this paper is that we propose an interpolation algorithm which could not only enhance the visual quality but also maintain camera parameters. Camera properties of the interpolated images are preserved by utilizing the epipolar geometry constraint of non-linear images. In our experiment, good performance was found on four sets of omni-images using the proposed method compared with the standard bilinear algorithm and bicubic algorithm.
Human free-hand sketches have been studied in various contexts including sketch recognition, synthesis and fine-grained sketch-based image retrieval (FG-SBIR). A fundamental challenge for sketch analysis is to deal with drastically different human drawing styles, particularly in terms of abstraction level. In this work, we propose the first stroke-level sketch abstraction model based on the insight of sketch abstraction as a process of trading off between the recognizability of a sketch and the number of strokes used to draw it. Concretely, we train a model for abstract sketch generation through reinforcement learning of a stroke removal policy that learns to predict which strokes can be safely removed without affecting recognizability. We show that our abstraction model can be used for various sketch analysis tasks including: (1) modeling stroke saliency and understanding the decision of sketch recognition models, (2) synthesizing sketches of variable abstraction for a given category, or reference object instance in a photo, and (3) training a FG-SBIR model with photos only, bypassing the expensive photo-sketch pair collection step.
The development of a city gradually fosters different functional regions, and between these regions there exists different social information due to human activities. In this paper, a Region Activation Entropy Model (RAEM) is proposed to discover the social relations hidden between the regions. Specifically we segment a city into coherent regions according the base station (BS) position and detect the stay and passing regions in trajectories of mobile phone users. We regard one user's trajectory as a short document and take the stay regions in the trajectory as words, so that we can use Natural Language Processing (NLP) method to discover the relations between regions. Furthermore, the Region Activation Force (RAF) is defined to measure the intensity of relationship between regions. By measuring the Region Activation Entropy (RAE) based on RAF, we find an 88% potential predictability in regional mobility. The result generated by RAEM can benefit a variety of applications, including city planning, location choosing for a business and predicting the spread of human. We evaluated our method using a one-month-long record collected by mobile phone carriers. We believe our findings offer a new perspective on research of human mobility.
To perceive and create a whole from parts is a prime trait of the human visual system. In this paper, we teach machines to perform a similar task by recreating a vectorised human sketch from its incomplete parts. This is fundamentally different to prior work on image completion (i) sketches exhibit a severe lack of visual cue and are of a sequential nature, and more importantly (ii) we ask for an agent that does not just fill in a missing part, but to recreate a novel sketch that closely resembles the partial input from scratch. Central to our contribution is a graph model that encodes both the visual and structural features over multiple categories. A novel sketch graph construction module is proposed that leverages the sequential nature of sketches to associate key parts centred around stroke junctions. The intuition is then that message passing within the said graph will naturally provide the healing power when it comes to missing parts (nodes). Finally, an off-the-shelf LSTM-based decoder is employed to decode sketches in a vectorised fashion. Both qualitative and quantitative results show that the proposed model significantly outperforms state-of-the-art alternatives.
We present a graph matching refinement framework that improves the performance of a given graph matching algorithm. Our method synergistically uses the inherent structure information embedded globally in the active association graph, and locally on each individual graph. The combination of such information reveals how consistent each candidate match is with its global and local contexts. In doing so, the proposed method removes most false matches and improves precision. The validation on standard benchmark datasets demonstrates the effectiveness of our method.
Modelling human free-hand sketches has become topical recently, driven by practical applications such as fine-grained sketch based image retrieval (FG-SBIR). Sketches are clearly related to photo edge-maps, but a human free-hand sketch of a photo is not simply a clean rendering of that photo’s edge map. Instead there is a fundamental process of abstraction and iconic rendering, where overall geometry is warped and salient details are selectively included. In this paper we study this sketching process and attempt to invert it. We model this inversion by translating iconic free-hand sketches to contours that resemble more geometrically realistic projections of object boundaries, and separately factorise out the salient added details. This factorised re-representation makes it easier to match a free-hand sketch to a photo instance of an object. Specifically, we propose a novel unsupervised image style transfer model based on enforcing a cyclic embedding consistency constraint. A deep FG-SBIR model is then formulated to accommodate complementary discriminative detail from each factorised sketch for better matching with the corresponding photo. Our method is evaluated both qualitatively and quantitatively to demonstrate its superiority over a number of state-of-the-art alternatives for style transfer and FG-SBIR.
We describe a mobile augmented reality application that is based on 3D snapshotting using multiple photographs. Optical square markers provide the anchor for reconstructed virtual objects in the scene. A novel approach based on pixel flow highly improves tracking performance. This dual tracking approach also allows for a new single-button user interface metaphor for moving virtual objects in the scene. The development of the AR viewer was accompanied by user studies confirming the chosen approach.
Sketch-based image retrieval (SBIR) has become a prominent research topic in recent years due to the proliferation of touch screens. The problem is however very challenging for that photos and sketches are inherently modeled in different modalities. Photos are accurate (colored and textured) depictions of the real-world, whereas sketches are highly abstract (black and white) renderings often drawn from human memory. This naturally motivates us to study the effectiveness of various cross-modal retrieval methods in SBIR. However, to the best of our knowledge, all established cross-modal algorithms are designed to traverse the more conventional cross-modal gap of image and text, making their general applicableness to SBIR unclear. In this paper, we design a series of experiments to clearly illustrate circumstances under which cross-modal methods can be best utilized to solve the SBIR problem. More specifically, we choose six state-of-the-art cross-modal subspace learning approaches that were shown to work well on image-text and conduct extensive experiments on a recently released SBIR dataset. Finally, we present detailed comparative analysis of the experimental results and offer insights to benefit future research.
This paper is about shape fitting to regions that segment an image and some applications that rely on the abstraction that offers. The novelty lies in three areas: (1) we fit a shape drawn from a selection of shape families, not just one class of shape, using a supervised classifier; (2) We use results from the classifier to match photographs and artwork of particular objects using a few qualitative shapes, which overcomes the significant differences between photographs and paintings; (3) We further use the shape classifier to process photographs into abstract synthetic art which, so far as we know, is novel too. Thus we use our shape classier in both discriminative (matching) and generative (image synthesis) tasks. We conclude the level of abstraction offered by our shape classifier is novel and useful.
Many segmentation algorithms describe images in terms of a hierarchy of regions. Although such hierarchies can produce state of the art segmentations and have many applications, they often contain more data than is required for an efficient description. This paper shows Laplacian graph energy is a generic measure that can be used to identify semantic structures within hierarchies, independently of the algorithm that produces them. Quantitative experimental validation using hierarchies from two state of art algorithms show we can reduce the number of levels and regions in a hierarchy by an order of magnitude with little or no loss in performance when compared against human produced ground truth. We provide a tracking application that illustrates the value of reduced hierarchies.
Growing free online 3D shapes collections dictated research on 3D retrieval. Active debate has however been had on (i) what is the best input modality to trigger retrieval, and (ii) the ultimate usage scenario for such retrieval. In this paper, we offer a different perspective towards answering these questions – we study the use of 3D sketches as an input modality, and advocate a VR-scenario where retrieval is conducted. The ultimate vision is therefore users can freely retrieve 3D model by air-doodling in a VR environment. As a first stab at this new 3D VR-sketch to 3D shape retrieval problem, we make four contributions: first, we code a VR utility to collect 3D VR-Sketches and conduct retrieval; second, we collect the first set of 167 3D VR-sketches on two shape categories from ModelNet; third, we propose a novel approach to generate a synthetic dataset of human-like 3D sketches of different abstract levels to train deep networks; at last, we compare the common multi-view and volumetric approaches, and show that in contrast to 3D shape retrieval, due to sparse and abstract nature of 3D VR-sketch, volumetric point-based approach exhibits superior performance. We believe these contribution will collectively serve as enablers for future attempts at this problem, and will make the VR interface, code, datasets publicly available to facilitate such research.
Categorizing free-hand human sketches has profound implications in applications such as human computer interaction and image retrieval. The task is non-trivial due to the iconic nature of sketches, signified by large variances in both appearance and structure when compared with photographs. Prior works often utilize off-the-shelf low-level features and assume the availability of a large training set, rendering them sensitive towards abstraction and less scalable to new categories. To overcome this limitation, we propose a transfer learning framework which enables one-shot learning of sketch categories. The framework is based on a novel co-regularized sparse coding model which exploits common/ shareable parts among human sketches of seen categories and transfer them to unseen categories. We contribute a new dataset consisting of 7,760 human segmented sketches from 97 object categories. Extensive experiments reveal that the proposed method can classify unseen sketch categories given just one training sample with a 33.04% accuracy, offering a two-fold improvement over baselines.
The problem of domain generalization is to learn from multiple training domains, and extract a domain-agnostic model that can then be applied to an unseen domain. Domain generalization (DG) has a clear motivation in contexts where there are target domains with distinct characteristics, yet sparse data for training. For example recognition in sketch images, which are distinctly more abstract and rarer than photos. Nevertheless, DG methods have primarily been evaluated on photo-only benchmarks focusing on alleviating the dataset bias where both problems of domain distinctiveness and data sparsity can be minimal. We argue that these benchmarks are overly straightforward, and show that simple deep learning baselines perform surprisingly well on them. In this paper, we make two main contributions: Firstly, we build upon the favorable domain shift-robust properties of deep learning methods, and develop a low-rank parameterized CNN model for end-to-end DG learning. Secondly, we develop a DG benchmark dataset covering photo, sketch, cartoon and painting domains. This is both more practically relevant, and harder (bigger domain shift) than existing benchmarks. The results show that our method outperforms existing DG alternatives, and our dataset provides a more significant DG challenge to drive future research.
We contribute the first large-scale dataset of scene sketches, SketchyScene, with the goal of advancing research on sketch understanding at both the object and scene level. The dataset is created through a novel and carefully designed crowdsourcing pipeline, enabling users to efficiently generate large quantities of realistic and diverse scene sketches. SketchyScene contains more than 29,000 scene-level sketches, 7,000+ pairs of scene templates and photos, and 11,000+ object sketches. All objects in the scene sketches have ground-truth semantic and instance masks. The dataset is also highly scalable and extensible, easily allowing augmenting and/or changing scene composition. We demonstrate the potential impact of SketchyScene by training new computational models for semantic segmentation of scene sketches and showing how the new dataset enables several applications including image retrieval, sketch colorization, editing, and captioning, etc. The dataset and code can be found at https://github.com/SketchyScene/SketchyScene.
Sketch is used for rendering the visual world since prehistoric times, and has become ubiquitous nowadays with the increasing availability of touchscreens on portable devices. However, how to automatically map images to sketches, a problem that has profound implications on applications such as sketch-based image retrieval, still remains open. In this paper, we propose a novel method that draws a sketch automatically from a single natural image. Sketch extraction is posed within an unified contour grouping framework, where perceptual grouping is first used to form contour segment groups, followed by a group-based contour simplification method that generate the final sketches. In our experiment, for the first time we pose sketch evaluation as a sketch-based object recognition problem and the results validate the effectiveness of our system over the state-of-the-arts alternatives.
Simple and contactless methods for determining the health of metallic and composite structures are necessary to allow non-invasive Non-Destructive Evaluation (NDE) of damaged structures. Many recognized damage detection techniques, such as frequency shift, generalized fractal dimension and wavelet transform, have been described with the aim to identify, locate damage and determine the severity of damage. These techniques are often tailored for factors such as (i) type of material, (ii) damage patterns (crack, impact damage, delamination), and (iii) nature of input signals (space and time). In this paper, a wavelet-based damage detection framework that locates damage on cantilevered composite beams via NDE using computer vision technologies is presented. Two types of damage have been investigated in this research: (i) defects induced by removing material to reduce stiffness in a metallic beam and (ii) manufactured delaminations in a composite laminate. The novelty in the proposed approach is the use of bespoke computer vision algorithms for the contactless acquisition of modal shapes, a task that is commonly regarded as a barrier to practical damage detection. Using the proposed method, it is demonstrated that modal shapes of cantilever beams can be readily reconstructed by extracting markers using Hough Transform from images captured using conventional slow motion cameras. This avoids the need to use expensive equipment such as laser doppler vibrometers. The extracted modal shapes are then used as input for a wavelet transform damage detection, exploiting both discrete and continuous variants. The experimental results are verified using finite element models (FEM).
This paper shows that it is possible to semi-automatically process photographs into Simple Art. Simple Art is a term that we use to refer to a group of artistic styles such a child art cave art, and Fine Artists as exemplified by Joan Miro. None of these styles has been previously studied by the NPR community. Our contribution is to provide a process that makes them accessible. We describe a method that automatically constructs a hierarchical model of an input photograph, and asks a user to identify objects inside it. Each object is a sub-tree, which can be rendered under user control. The method is demonstrated using emulations of Simple Art. We include an assessment of our results against a set of norms recommended by a Cultural Historian. We conclude that producing Simple Art raises important technical questions, especially surrounding the interplay between computational modelling and human abstractions.
Sketch-based image retrieval (SBIR) is a challenging task due to the ambiguity inherent in sketches when compared with photos. In this paper, we propose a novel convolutional neural network based on Siamese network for SBIR. The main idea is to pull output feature vectors closer for input sketch-image pairs that are labeled as similar, and push them away if irrelevant. This is achieved by jointly tuning two convolutional neural networks which linked by one loss function. Experimental results on Flickr15K demonstrate that the proposed method offers a better performance when compared with several state-of-the-art approaches. © 2016 IEEE.
Matching face images across different modalities is a challenging open problem for various reasons, notably feature heterogeneity, and particularly in the case of sketch recognition – abstraction, exaggeration and distortion. Existing studies have attempted to address this task by engineering invariant features, or learning a common subspace between the modalities. In this paper, we take a different approach and explore learning a mid-level representation within each domain that allows faces in each modality to be compared in a domain invariant way. In particular, we investigate sketch-photo face matching and go beyond the well-studied viewed sketches to tackle forensic sketches and caricatures where representations are often symbolic. We approach this by learning a facial attribute model independently in each domain that represents faces in terms of semantic properties. This representation is thus more invariant to heterogeneity, distortions and robust to mis-alignment. Our intermediate level attribute representation is then integrated synergistically with the original low-level features using CCA. Our framework shows impressive results on cross-modal matching tasks using forensic sketches, and even more challenging caricature sketches. Furthermore, we create a new dataset with ≈ 59, 000 attribute annotations for evaluation and to facilitate future research.
In this work we aim to develop a universal sketch grouper. That is, a grouper that can be applied to sketches of any category in any domain to group constituent strokes/segments into semantically meaningful object parts. The first obstacle to this goal is the lack of large-scale datasets with grouping annotation. To overcome this, we contribute the largest sketch perceptual grouping (SPG) dataset to date, consisting of 20, 000 unique sketches evenly distributed over 25 object categories. Furthermore, we propose a novel deep universal perceptual grouping model. The model is learned with both generative and discriminative losses. The generative losses improve the generalisation ability of the model to unseen object categories and datasets. The discriminative losses include a local grouping loss and a novel global grouping loss to enforce global grouping consistency. We show that the proposed model significantly outperforms the state-of-the-art groupers. Further, we show that our grouper is useful for a number of sketch analysis tasks including sketch synthesis and fine-grained sketch-based image retrieval (FG-SBIR). © Springer Nature Switzerland AG 2018.
Under person re-identification (Re-ID), a query photo of the target person is often required for retrieval. However, one is not always guaranteed to have such a photo readily available under a practical forensic setting. In this paper, we define the problem of Sketch Re-ID, which instead of using a photo as input, it initiates the query process using a professional sketch of the target person. This is akin to the traditional problem of forensic facial sketch recognition, yet with the major difference that our sketches are whole-body other than just the face. This problem is challenging because sketches and photos are in two distinct domains. Specifically, a sketch is the abstract description of a person. Besides, person appearance in photos is variational due to camera viewpoint, human pose and occlusion. We address the Sketch Re-ID problem by proposing a cross-domain adversarial feature learning approach to jointly learn the identity features and domain-invariant features. We employ adversarial feature learning to filter low-level interfering features and remain high-level semantic information. We also contribute to the community the first Sketch Re-ID dataset with 200 persons, where each person has one sketch and two photos from different cameras associated. Extensive experiments have been performed on the proposed dataset and other common sketch datasets including CUFSF and QUML-shoe. Results show that the proposed method outperforms the state-of-the-arts.
We aim to learn a domain generalizable person reidentification (ReID) model. When such a model is trained on a set of source domains (ReID datasets collected from different camera networks), it can be directly applied to any new unseen dataset for effective ReID without any model updating. Despite its practical value in real-world deployments, generalizable ReID has seldom been studied. In this work, a novel deep ReID model termed Domain-Invariant Mapping Network(DIMN) is proposed. DIMN is designed to learn a mapping between a person image and its identity classifier, i.e., it produces a classifier using a single shot. To make the model domain-invariant, we follow a meta-learning pipeline and sample a subset of source domain training tasks during each training episode. However, the model is significantly different from conventional meta-learning methods in that: (1) no model updating is required for the target domain, (2) different training tasks share a memory bank for maintaining both scalability and discrimination ability, and (3) it can be used to match an arbitrary number of identities in a target domain. Extensive experiments on a newly proposed large-scale ReID domain generalization benchmark show that our DIMN significantly outperforms alternative domain generalization or meta-learning methods.
To see is to sketch - free-hand sketching naturally builds ties between human and machine vision. In this paper, we present a novel approach for translating an object photo to a sketch, mimicking the human sketching process. This is an extremely challenging task because the photo and sketch domains differ significantly. Furthermore, human sketches exhibit various levels of sophistication and abstraction even when depicting the same object instance in a reference photo. This means that even if photo-sketch pairs are available, they only provide weak supervision signal to learn a translation model. Compared with existing supervised approaches that solve the problem of D(E(photo)) → sketch), where E(·) and D(·) denote encoder and decoder respectively, we take advantage of the inverse problem (e.g., D(E(sketch) → photo), and combine with the unsupervised learning tasks of within-domain reconstruction, all within a multi-task learning framework. Compared with existing unsupervised approaches based on cycle consistency (i.e., D(E(D(E(photo)))) → photo), we introduce a shortcut consistency enforced at the encoder bottleneck (e.g., D(E(photo)) → photo) to exploit the additional self-supervision. Both qualitative and quantitative results show that the proposed model is superior to a number of state-of-the-art alternatives. We also show that the synthetic sketches can be used to train a better fine-grained sketch-based image retrieval (FG-SBIR) model, effectively alleviating the problem of sketch data scarcity.
The problem of learning the class identity of visual objects has received considerable attention recently. With rare exception, all of the work to date assumes low variation in appearance, which limits them to a single depictive style usually photographic. The same object depicted in other styles - as a drawing, perhaps - cannot be identified reliably. Yet humans are able to name the object no matter how it is depicted, and even recognise a real object having previously seen only a drawing. This paper describes a classifier which is unique in being able to learn class identity no matter how the class instances are depicted. The key to this is our proposition that topological structure is a class invariant. Practically, we depend on spectral graph analysis of a hierarchical description of an image to construct a feature vector of fixed dimension. Hence structure is transformed to a feature vector, which can be classified using standard methods. We demonstrate the classifier on several diverse classes.
We propose a novel Directional Element Histogram of Oriented Gradient (DE-HOG) feature to human free-hand sketch recognition task that achieves superior performance to traditional HOG feature, originally designed for photographic objects. As a result of modeling the unique characteristics of free-hand sketch, i.e. consisting only a set of strokes omitting visual information such as color and brightness, being highly iconic and abstract. Specifically, we encode sketching strokes as a form of regularized directional vectors from the skeleton of a sketch, whilst still leveraging the HOG feature to meet the local deformation-invariant demands. Such a representation combines the best of two features by encoding necessary and discriminative stroke-level information, but can still robustly deal with various levels of sketching variations. Extensive experiments conducted on two large benchmark sketch recognition datasets demonstrate the performance of our proposed method.
Human sketches are unique in being able to capture both the spatial topology of a visual object, as well as its subtle appearance details. Fine-grained sketch-based image retrieval (FG-SBIR) importantly leverages on such fine-grained characteristics of sketches to conduct instance-level retrieval of photos. Nevertheless, human sketches are often highly abstract and iconic, resulting in severe misalignments with candidate photos which in turn make subtle visual detail matching difficult. Existing FG-SBIR approaches focus only on coarse holistic matching via deep cross-domain representation learning, yet ignore explicitly accounting for fine-grained details and their spatial context. In this paper, a novel deep FG-SBIR model is proposed which differs significantly from the existing models in that: (1) It is spatially aware, achieved by introducing an attention module that is sensitive to the spatial position of visual details: (2) It combines coarse and fine semantic information via a shortcut connection fusion block: and (3) It models feature correlation and is robust to misalignments between the extracted features across the two domains by introducing a novel higher-order learnable energy function (HOLEF) based loss. Extensive experiments show that the proposed deep spatial-semantic attention model significantly outperforms the state-of-the-art.
Gestalt principles, a set of conjoining rules derived from human visual studies, have been known to play an important role in computer vision. Many applications such as image segmentation, contour grouping and scene understanding often rely on such rules to work. However, the problem of Gestalt confliction, i.e., the relative importance of each rule compared with another, remains unsolved. In this paper, we investigate the problem of perceptual grouping by quantifying the confliction among three commonly used rules: similarity, continuity and proximity. More specifically, we propose to quantify the importance of Gestalt rules by solving a learning to rank problem, and formulate a multi-label graph-cuts algorithm to group image primitives while taking into account the learned Gestalt confliction. Our experiment results confirm the existence of Gestalt confliction in perceptual grouping and demonstrate an improved performance when such a confliction is accounted for via the proposed grouping algorithm. Finally, a novel cross domain image classification method is proposed by exploiting perceptual grouping as representation.
The problem of identifying the class of an object from its visual appearance has received significant attention recently. Most of the work to date is premised on photometric measures, often building codebooks made from interest regions. All of it has been tested only on photographs, so far as we know. Our approach differs in two significant ways. First, we do not build a codebook of interest regions but instead make use of a hierarchical description of an image based on a watershed transform. Root nodes in the hierarchy are putative objects to be classified. Second, we classify these putative objects using a vector of fixed length that represents the structure of the hierarchy below the node. This allows us to classify not just photographs, but also paintings and drawings of visual objects.
Automatic data abstraction is an important capability for both benchmarking machine intelligence and supporting summarization applications. In the former one asks whether a machine can `understand' enough about the meaning of input data to produce a meaningful but more compact abstraction. In the latter this capability is exploited for saving space or human time by summarizing the essence of input data. In this paper we study a general reinforcement learning based framework for learning to abstract sequential data in a goal-driven way. The ability to define different abstraction goals uniquely allows different aspects of the input data to be preserved according to the ultimate purpose of the abstraction. Our reinforcement learning objective does not require human-defined examples of ideal abstraction. Importantly our model processes the input sequence holistically without being constrained by the original input order. Our framework is also domain agnostic -- we demonstrate applications to sketch, video and text data and achieve promising results in all domains.
Fine-grained sketch-based image retrieval (SBIR) aims to go beyond conventional SBIR to perform instance-level cross-domain retrieval: finding the specific photo that matches an input sketch. Existing methods focus on designing/learning good features for cross-domain matching and/or learning cross-domain matching functions. However, they neglect the semantic aspect of retrieval, i.e., what meaningful object properties does a user try encode in her/his sketch? We propose a fine-grained SBIR model that exploits semantic attributes and deep feature learning in a complementary way. Specifically, we perform multi-task deep learning with three objectives, including: retrieval by fine-grained ranking on a learned representation, attribute prediction, and attribute-level ranking. Simultaneously predicting semantic attributes and using such predictions in the ranking procedure help retrieval results to be more semantically relevant. Importantly, the introduction of semantic attribute learning in the model allows for the elimination of the otherwise prohibitive cost of human annotations required for training a fine-grained deep ranking model. Experimental results demonstrate that our method outperforms the state-of-the-art on challenging fine-grained SBIR benchmarks while requiring less annotation.
ImageNet pre-training has long been considered crucial by the fine-grained sketch-based image retrieval (FG-SBIR) community due to the lack of large sketch-photo paired datasets for FG-SBIR training. In this paper, we propose a self-supervised alternative for representation pre-training. Specifically, we consider the jigsaw puzzle game of recomposing images from shuffled parts. We identify two key facets of jigsaw task design that are required for effective FG-SBIR pre-training. The first is formulating the puzzle in a mixed-modality fashion. Second we show that framing the optimisation as permutation matrix inference via Sinkhorn iterations is more effective than the common classifier formulation of Jigsaw self-supervision. Experiments show that this self-supervised pre-training strategy significantly outperforms the standard ImageNet-based pipeline across all four product-level FG-SBIR benchmarks. Interestingly it also leads to improved cross-category generalisation across both pre-train/fine-tune and fine-tune/testing stages.
We propose a perceptual grouping framework that organizes image edges into meaningful structures and demonstrate its usefulness on various computer vision tasks. Our grouper formulates edge grouping as a graph partition problem, where a learning to rank method is developed to encode probabilities of candidate edge pairs. In particular, RankSVM is employed for the first time to combine multiple Gestalt principles as cue for edge grouping. Afterwards, an edge grouping based object proposal measure is introduced that yields proposals comparable to state-of-the-art alternatives. We further show how human-like sketches can be generated from edge groupings and consequently used to deliver state-of-the-art sketch-based image retrieval performance. Last but not least, we tackle the problem of freehand human sketch segmentation by utilizing the proposed grouper to cluster strokes into semantic object parts.
In this paper, we investigate the problem of zeroshot sketch-based image retrieval (ZS-SBIR), where human sketches are used as queries to conduct retrieval of photos from unseen categories. We importantly advance prior arts by proposing a novel ZS-SBIR scenario that represents a firm step forward in its practical application. The new setting uniquely recognizes two important yet often neglected challenges of practical ZS-SBIR, (i) the large domain gap between amateur sketch and photo, and (ii) the necessity for moving towards large-scale retrieval. We first contribute to the community a novel ZS-SBIR dataset, QuickDraw-Extended, that consists of 330; 000 sketches and 204; 000 photos spanning across 110 categories. Highly abstract amateur human sketches are purposefully sourced to maximize the domain gap, instead of ones included in existing datasets that can often be semi-photorealistic. We then formulate a ZS-SBIR framework to jointly model sketches and photos into a common embedding space. A novel strategy to mine the mutual information among domains is specifically engineered to alleviate the domain gap. External semantic knowledge is further embedded to aid semantic transfer. We show that, rather surprisingly, retrieval performance significantly outperforms that of state-of-the-art on existing datasets that can already be achieved using a reduced version of our model. We further demonstrate the superior performance of our full model by comparing with a number of alternatives on the newly proposed dataset. The new dataset, plus all training and testing code of our model, will be publicly released to facilitate future research.
In this paper, we introduce a new dataset for scene classification based on camera metadata. We classify the most common scenes that have been researched much recently. This dataset consists of 12 scene categories. Each category contains 500 to 2000 images. Most images are high resolution such as 2000×2000 pixels. The images in the dataset are original, namely, each image brings with a camera metadata (EXIF). Various types, metadata cues of photos, strict definitions among scenes are characteristic factors that make this dataset a very challenging testbed for photo classification. We supply the scene photos together with scene labeling, as well as the EXIF information extraction via methodology, and we apply the dataset into sementic scene classification up to now.
We propose a deep hashing framework for sketch retrieval that, for the first time, works on a multi-million scale human sketch dataset. Leveraging on this large dataset, we explore a few sketch-specific traits that were otherwise under-studied in prior literature. Instead of following the conventional sketch recognition task, we introduce the novel problem of sketch hashing retrieval which is not only more challenging, but also offers a better testbed for large-scale sketch analysis, since: (i) more fine-grained sketch feature learning is required to accommodate the large variations in style and Abstraction, and (ii) a compact binary code needs to be learned at the same time to enable efficient retrieval. Key to our network design is the embedding of unique characteristics of human sketch, where (i) a two-branch CNN-RNN architecture is adapted to explore the temporal ordering of strokes, and (ii) a novel hashing loss is specifically designed to accommodate both the temporal and Abstract traits of sketches. By working with a 3.8M sketch dataset, we show that state-of-the-art hashing models specifically engineered for static images fail to perform well on temporal sketch data. Our network on the other hand not only offers the best retrieval performance on various code sizes, but also yields the best generalization performance under a zero-shot setting and when re-purposed for sketch recognition. Such superior performances effectively demonstrate the benefit of our sketch-specific design. © 2018 IEEE.
We study the problem of fine-grained sketch-based image retrieval. By performing instance-level (rather than category-level) retrieval, it embodies a timely and practical application, particularly with the ubiquitous availability of touchscreens. Three factors contribute to the challenging nature of the problem: (i) free-hand sketches are inherently abstract and iconic, making visual comparisons with photos more difficult, (ii) sketches and photos are in two different visual domains, i.e. black and white lines vs. color pixels, and (iii) fine-grained distinctions are especially challenging when executed across domain and abstraction-level. To address this, we propose to detect visual attributes at part-level, in order to build a new representation that not only captures fine-grained characteristics but also traverses across visual domains. More specifically, (i) we propose a dataset with 304 photos and 912 sketches, where each sketch and photo is annotated with its semantic parts and associated part-level attributes, and with the help of this dataset, we investigate (ii) how strongly-supervised deformable part-based models can be learned that subsequently enable automatic detection of part-level attributes, and (iii) a novel matching framework that synergistically integrates low-level features, mid-level geometric structure and high-level semantic attributes to boost retrieval performance. Extensive experiments conducted on our new dataset demonstrate value of the proposed method.
Recent encoder-decoder approaches typically employ string decoders to convert images into serialized strings for image-to-markup. However, for tree-structured representational markup, string representations can hardly cope with the structural complexity. In this work, we first show via a set of toy problems that string decoders struggle to decode tree structures, especially as structural complexity increases, we then propose a tree-structured decoder that specifically aims at generating a tree-structured markup. Our decoders works sequentially, where at each step a child node and its parent node are simultaneously generated to form a sub-tree. This sub-tree is consequently used to construct the final tree structure in a recurrent manner. Key to the success of our tree decoder is twofold, (i) it strictly respects the parent-child relationship of trees, and (ii) it explicitly outputs trees as oppose to a linear string. Evaluated on both math formula recognition and chemical formula recognition, the proposed tree decoder is shown to greatly outperform strong string decoder baselines.
Sketch recognition aims to automatically classify human hand sketches of objects into known categories. This has become increasingly a desirable capability due to recent advances in human computer interaction on portable devices. The problem is nontrivial because of the sparse and abstract nature of hand drawings as compared to photographic images of objects, compounded by a highly variable degree of details in human sketches. To this end, we present a method for the representation and matching of sketches by exploiting not only local features but also global structures of sketches, through a star graph based ensemble matching strategy. Different local feature representations were evaluated using the star graph model to demonstrate the effectiveness of the ensemble matching of structured features. We further show that by encapsulating holistic structure matching and learned bag-of-features models into a single framework, notable recognition performance improvement over the state-of-the-art can be observed. Extensive comparative experiments were carried out using the currently largest sketch dataset released by Eitz et al. [15], with over 20,000 sketches of 250 object categories generated by AMT (Amazon Mechanical Turk) crowd-sourcing.
Analysis of human sketches in deep learning has advanced immensely through the use of waypoint-sequences rather than raster-graphic representations. We further aim to model sketches as a sequence of low-dimensional parametric curves. To this end, we propose an inverse graphics framework capable of approximating a raster or waypoint based stroke encoded as a point-cloud with a variable-degree Bezier curve. Building on this module, ´we present Cloud2Curve, a generative model for scalable high-resolution vector sketches that can be trained end-to-end using point-cloud data alone. As a consequence, our model is also capable of deterministic vectorization which can map novel raster or waypoint based sketches to their corresponding high-resolution scalable Bezier equivalent. ´We evaluate the generation and vectorization capabilities of our model on Quick, Draw! and K-MNIST datasets. The analysis of free-hand sketches using deep learning [40] has flourished over the past few years, with sketches now being well analysed from classification [43, 42] and retrieval [27, 12, 4] perspectives. Sketches for digital analysis have always been acquired in two primary modalities - raster (pixel grids) and vector (line segments). Raster sketches have mostly been the modality of choice for sketch recognition and retrieval [43, 27]. However, generative sketch models began to advance rapidly [16] after focusing on vector representations and generating sketches as sequences [7, 37] of waypoints/line segments, similarly to how humans sketch. As a happy byproduct, this paradigm leads to clean and blur-free image generation as opposed to direct raster-graphic generations [30]. Recent works have studied creativity in sketch generation [16], learning to sketch raster photo input images [36], learning efficient
Additional publications
For full publication list please refer to Google Scholar, and CSRankings.
2023
- Subhadeep Koley, Ayan Kumar Bhunia, Aneeshan Sain, Pinaki Nath Chowdhury, Tao Xiang, Yi-Zhe Song, Picture that Sketch: Photorealistic Image Generation from Abstract Sketches, CVPR 2023
- Ke Li, Kaiyue Pang, Yi-Zhe Song, Photo Pre-Training, But for Sketch, CVPR 2023
- Zhiyu Qu, Yulia Gryaditskaya, Ke Li, Kaiyue Pang, Tao Xiang, Yi-Zhe Song, SketchXAI: A First Look at Explainability for Human Sketches, CVPR 2023
- Ayan Kumar Bhunia, Subhadeep Koley, Amandeep Kumar, Aneeshan Sain, Pinaki Nath Chowdhury, Tao Xiang, Yi-Zhe Song, Sketch2Saliency: Learning to Detect Salient Objects from Human Drawings, CVPR 2023
- Pinaki Nath Chowdhury, Ayan Kumar Bhunia, Aneeshan Sain, Subhadeep Koley, Tao Xiang, Yi-Zhe Song, What Can Human Sketches Do for Object Detection?, CVPR 2023
- Fengyin Lin, Mingkang Li, Yonggang Qi, Da Li, Timothy Hospedales, Yi-Zhe Song, Zero-Shot Everything Sketch-Based Image Retrieval, and in Explainable Style, CVPR 2023
- Pinaki Nath Chowdhury, Ayan Kumar Bhunia, Aneeshan Sain, Subhadeep Koley, Tao Xiang, Yi-Zhe Song, SceneTrilogy: On Human Scene-Sketch and its Complementarity with Photo and Text, CVPR 2023
- Aneeshan Sain, Ayan Kumar Bhunia, Subhadeep Koley, Pinaki Nath Chowdhury, Soumitri Chattopadhyay, Tao Xiang, Yi-Zhe Song, Exploiting Unlabelled Photos for Stronger Fine-Grained SBIR, CVPR 2023
- Aneeshan Sain, Ayan Kumar Bhunia, Pinaki Nath Chowdhury, Subhadeep Koley, Tao Xiang, Yi-Zhe Song, CLIP for All Things Zero-Shot Sketch-Based Image Retrieval, Fine-Grained or Not, CVPR 2023
- Abhra Chaudhuri, Ayan Kumar Bhunia, Yi-Zhe Song, Anjan Dutta, Data-Free Sketch-Based Image Retrieval, CVPR 2023
- Dongliang Chang, Yujun Tong, Ruoyi Du, Timothy Hospedales, Yi-Zhe Song, Zhanyu Ma, An Erudite Fine-Grained Visual Classification Model, CVPR 2023
- Ruoyi Du, Dongliang Chang, Kongming Liang, Timothy Hospedales, Yi-Zhe Song, Zhanyu Ma, On-the-fly Category Discovery, CVPR 2023
- Xiao Han, Xiatian Zhu, Licheng Yu, Li Zhang, Yi-Zhe Song, Tao Xiang, FAME-ViL: Multi-Tasking Vision-Language Model for Heterogeneous Fashion Tasks, CVPR 2023
- Sauradip Nag, Xiatian Zhu, Yi-Zhe Song, Tao Xiang, Post-Processing Temporal Action Detection, CVPR 2023
- Ayan Das, Yongxin Yang, Timothy Hospedales, Tao Xiang, Yi-Zhe Song, ChiroDiff: Modelling chirographic data with Diffusion Models, ICLR 2023
- Qiang Wang, Haoge Deng, Yonggang Qi, Da Li, Yi-Zhe Song, SketchKnitter: Vectorized Sketch Generation with Diffusion Models, ICLR 2023
2022
- Peng Xu, Timothy M. Hospedales, Qiyue Yin, Yi-Zhe Song, Tao Xiang, Liang Wang, Deep Learning for Free-Hand Sketch: A Survey, IEEE TPAMI
- X.-S. Wei, Yi-Zhe. Song, O. Mac Aodha, J. Wu, Y. Peng, J. Tang, J. Yang, and S. Belongie, Fine-Grained Image Analysis with Deep Learning: A Survey, IEEE TPAMI
- Yonggang Qi, Guoyao Su, Qiang Wang, Jie Yang, Kaiyue Pang and Yi-Zhe Song, Generative Sketch Healing, IJCV
- Anran Qi, Yulia Gryaditskaya, Tao Xiang, Yi-Zhe Song, One Sketch for All: One-Shot Personalized Sketch Segmentation, IEEE TIP
- Ayan Das, Yongxin Yang, Timothy M. Hospedales, Tao Xiang, Yi-Zhe Song, SketchODE: Learning neural sketch representation in continuous time, ICLR 2022
- Lan Yang, Kaiyue Pang, Honggang Zhang, Yi-Zhe Song, Finding Badly Drawn Bunnies, CVPR 2022
- Pinaki Nath Chowdhury, Ayan Kumar Bhunia, Viswanatha Reddy Gajjala, Aneeshan Sain, Tao Xiang, Yi-Zhe Song, Partially Does It: Towards Scene-Level FG-SBIR with Partial Input, CVPR 2022
- Aneeshan Sain, Ayan Kumar Bhunia, Vaishnav Potlapalli, Pinaki Nath Chowdhury, Tao Xiang, Yi-Zhe Song, Sketch3T: Test-time Training for Zero-Shot SBIR, CVPR 2022
- Ayan Kumar Bhunia, Viswanatha Reddy Gajjala, Subhadeep Koley, Rohit Kundu, Aneeshan Sain, Tao Xiang, Yi-Zhe Song, Doodle It Yourself: Class Incremental Learning by Drawing a Few Sketches, CVPR 2022
- Ayan Kumar Bhunia, Subhadeep Koley, Abdullah Faiz Ur Rahman Khilji, Aneeshan Sain, Pinaki Nath Chowdhury, Tao Xiang, Yi-Zhe Song, Sketching without Worrying: Noise-Tolerant Sketch-Based Image Retrieval, CVPR 2022
- Sen He, Yi-Zhe Song, Tao Xiang, Style-Based Global Appearance Flow for Virtual Try-On, CVPR 2022
- Pinaki Nath Chowdhury, Aneeshan Sain, Yulia Gryaditskaya, Ayan Kumar Bhunia, Tao Xiang, Yi-Zhe Song, FS-COCO: Towards Understanding of Freehand Sketches of Common Objects in Context, ECCV 2022
- Ayan Kumar Bhunia, Aneeshan Sain, Parth Hiren Shah, Animesh Gupta, Pinaki Nath Chowdhury, Tao Xiang, Yi-Zhe Song, Adaptive Fine-Grained Sketch-Based Image Retrieval, ECCV 2022
- Chenjian Gao, Qian Yu, Lu Sheng, Yi-Zhe Song, Dong Xu, SketchSampler: Sketch-based 3D Reconstruction via View-dependent Depth Sampling, ECCV 2022
- Xiao Han, Licheng Yu, Xiatian Zhu, Li Zhang, Yi-Zhe Song, Tao Xiang, FashionViL: Fashion-Focused Vision-and-Language Representation Learning, ECCV 2022
- Sauradip Nag, Xiatian Zhu, Yi-Zhe Song, Tao Xiang, Temporal Action Localization with Global Segmentation Mask Learning, ECCV 2022
- Sauradip Nag, Xiatian Zhu, Yi-Zhe Song, Tao Xiang, Semi-Supervised Temporal Action Localization with Proposal-Free Temporal Mask Learning, ECCV 2022
- Sauradip Nag, Xiatian Zhu, Yi-Zhe Song, Tao Xiang, Prompting Vision-Language for Temporal Action Localization using fewer data, ECCV 2022
2021
- Jiun Tian Hoe*, Kam Woh Ng*, Tianyu Zhang, Chee Seng Chan, Yi-Zhe Song, Tao Xiang. One Loss for All: Deep Hashing with a Single Cosine Similarity based Learning Objective, NeurIPS 2021
- Ling Luo, Yulia Gryaditskaya, Yongxin Yang, Tao Xiang, Yi-Zhe Song, Fine-Grained VR Sketching: Dataset and Insights, 3DV 2021
- Lan Yang, Kaiyue Pang, Honggang Zhang, Yi-Zhe Song, SketchAA: Abstract Representation for Abstract Sketches, ICCV 2021
- Yonggang Qi, Guoyao Su, Pinaki Nath Chowdhury, Mingkang Li, Yi-Zhe Song, SketchLattice: Latticed Representation for Sketch Manipulation, ICCV 2021
- Ayan Kumar Bhunia, Aneeshan Sain, Pinaki Nath Chowdhury, Yi-Zhe Song, Text is Text, No Matter What: Unifying Text Recognition using Knowledge Distillation, ICCV 2021
- Ayan Kumar Bhunia, Pinaki Nath Chowdhury, Aneeshan Sain, Yi-Zhe Song, Towards the Unseen: Iterative Text Recognition by Distilling from Errors, ICCV 2021
- Ayan Kumar Bhunia, Aneeshan Sain, Amandeep Kumar, Shuvozit Ghose, Pinaki Nath Chowdhury, Yi-Zhe Song, Joint Visual Semantic Reasoning: Multi-Stage Decoder for Text Recognition, ICCV 2021
- Sen He, Wentong Liao, Michael Ying Yang, Yi-Zhe Song, Bodo Rosenhahn, Tao Xiang, Disentangled Lifespan Face Synthesis, ICCV 2021
- Zhihe Lu, Sen He, Xiatian Zhu, Li Zhang, Yi-Zhe Song, Tao Xiang, Simpler is Better: Few-shot Semantic Segmentation with Classifier Weight Transformer, ICCV 2021
- Dongliang Chang, Kaiyue Pang, Yixiao Zheng, Zhanyu Ma, Yi-Zhe Song, Jun Guo, Your “Flamingo” is My “Bird”: Fine-Grained, or Not, CVPR 2021 Oral
- Ayan Kumar Bhunia, Pinaki Nath Chowdhury, Yongxin Yang, Timothy Hospedales, Tao Xiang, Yi-Zhe Song, Vectorization and Rasterization: Self-Supervised Learning for Sketch and Handwriting, CVPR 2021
- Ayan Das, Yongxin Yang, Timothy Hospedales, Tao Xiang, Yi-Zhe Song, Cloud2Curve: Generation and Vectorization of Parametric Sketches, CVPR 2021
- Aneeshan Sain, Ayan Kumar Bhunia, Yongxin Yang, Tao Xiang, Yi-Zhe Song, StyleMeUp: Towards Style-Agnostic Sketch-Based Image Retrieval, CVPR 2021
- Yonggang Qi, Kai Zhang, Aneeshan Sain, Yi-Zhe Song, PQA: Perceptual Question Answering, CVPR 2021
- Sen He, Wentong Liao, Michael Ying Yang, Yongxin Yang, Yi-Zhe Song, Bodo Rosenhahn, Tao Xiang, Context-Aware Layout to Image Generation with Enhanced Object Appearance, CVPR 2021
- Ayan Kumar Bhunia, Pinaki Nath Chowdhury, Aneeshan Sain, Yongxin Yang, Tao Xiang, Yi-Zhe Song, More Photos are All You Need: Semi-Supervised Learning for Fine-Grained Sketch-Based Image Retrieval, CVPR 2021
- Ayan Kumar Bhunia, Shuvozit Ghose, Amandeep Kumar, Pinaki Nath Chowdhury, Aneeshan Sain, Yi-Zhe Song, MetaHTR: Towards Writer-Adaptive Handwritten Text Recognition, CVPR 2021
- Anran Qi, Yulia Gryaditskaya, Jifei Song, Yongxin Yang, Yonggang Qi, Timothy M. Hospedales, Tao Xiang, Yi-Zhe Song, Towards Fine-Grained Sketch-Based 3D Shape Retrieval, IEEE Transactions on Image Processing (IEEE TIP), 2021
2020
- Ling Luo, Yulia Gryaditskaya, Yongxin Yang, Tao Xiang, Yi-Zhe Song, Towards 3D VR-Sketch to 3D Shape Retrieval, 3DV 2020 (Oral)
- Yue Zhong, Yulia Gryaditskaya, Honggang Zhang, Yi-Zhe Song, Deep Sketch-Based Modelling: Tips and Tricks, 3DV 2020
- Guoyao Su, Yonggang Qi, Kaiyue Pang , Jie Yang, Yi-Zhe Song, SketchHealer: A Graph-to-Sequence Network for Recreating Partial Human Sketches, BMVC 2020 (Oral)
- Aneeshan Sain, Ayan Kumar Bhunia, Yongxin Yang, Tao Xiang, Yi-Zhe Song, Cross-Modal Hierarchical Modelling for Fine-Grained Sketch Based Image Retrieval, BMVC 2020 (Oral).
- Ayan Kumar Bhunia*, Ayan Das*, Umar Riaz Muhammad*, Yongxin Yang, Timothy M. Hospedalis, Tao Xiang, Yulia Gryaditskaya, Yi-Zhe Song . Pixelor: A Competitive Sketching AI Agent. So you think you can beat me? SIGGRAPH Asia 2020.
- Ayan Das, Yongxin Yang, Timothy Hospedales, Tao Xiang, Yi-Zhe Song, BézierSketch: A generative model for scalable vector sketches, ECCV 2020
- Jianshu Zhang, Jun Du, Yongxin Yang, Yi-Zhe Song, Si Wei, Lirong Dai, A Tree-Structured Decoder for Image-to-Markup Generation, ICML 2020
- Kaiyue Pang, Yongxin Yang, Timothy Hospedales, Tao Xiang, Yi-Zhe Song, Solving Mixed-modal Jigsaw Puzzle for Fine-Grained Sketch-Based Image Retrieval, CVPR 2020
- Ayan Kumar Bhunia, Yongxin Yang, Timothy Hospedales, Tao Xiang, Yi-Zhe Song, Sketch Less for More: On-the-Fly Fine-Grained Sketch Based Image Retrieval, CVPR 2020 (Oral)
- Z. Lu, Y. Yang, X. Zhu, C. Liu, Y. Song and T. Xiang, "Stochastic Classifiers for Unsupervised Domain Adaptation", CVPR 2020
- Yu, Qian, Song, Jifei, Song, Yi-Zhe, Xiang, Tao and Hospedales, Timothy M. (2020) Fine-Grained Instance-Level Sketch-Based Image Retrieval, International Journal of Computer Vision.
- Dongliang Chang, Yifeng Ding, Jiyang Xie, Ayan Kumar Bhunia, Xiaoxu Li, Zhanyu Ma, Ming Wu, Jun Guo, Yi-Zhe Song, The Devil is in the Channels: Mutual-Channel Loss for Fine-grained Image Classification, IEEE Transactions on Image Processing
- Conghui Hu, Da Li, Yongxin Yang, Timothy M. Hospedales, Yi-Zhe Song, Sketch-a-Segmenter: Sketch-Based Photo Segmenter Generation. IEEE Transactions on Image Processing
- Yue Zhong, Yonggang Qi, Yulia Gryaditskaya, Honggang Zhang and Yi-Zhe Song,Towards Practical Sketch-based 3D ShapeGeneration: The Role of Professional Sketches IEEE Transactions on Circuits and Systems for Video Technology.
- Yu, Deng, Li, Lei, Zheng, Youyi, Lau, Manfred, Song, Yi-Zhe, Tai, Chiew-Lan and Fu, Hongbo (2020) SketchDesc: Learning Local Sketch Descriptors for Multi-view Correspondence IEEE Transactions on Circuits and Systems for Video Technology.
- Xu, Peng, Liu, Kun, Xiang, Tao, Hospedales, Timothy M., Ma, Zhanyu, Guo, Jun and Song, Yi-Zhe (2020) Fine-Grained Instance-Level Sketch-Based Video Retrieval IEEE Transactions on Circuits and Systems for Video Technology.
- Xu, Peng, Song, Zeyu, Yin, Qiyue, Song, Yi-Zhe and Wang, Liang (2020) Deep Self-Supervised Representation Learning for Free-Hand Sketch IEEE Transactions on Circuits and Systems for Video Technology.
- Jianjun Lei, Yuxin Song, Bo Peng, Zhanyu Ma, Ling Shao, Yi-Zhe Song, Semi-Heterogeneous Three-Way Joint Embedding Network for Sketch-Based Image Retrieval, IEEE Transactions on Circuits and Systems for Video Technology
- Peng Xu, Yongye Huang, Tongtong Yuan, Tao Xiang, Timothy M. Hospedales, Yi-Zhe Song and Liang Wang, On Learning Semantic Representations for Large-Scale Abstract Sketches, IEEE Transactions on Circuits and Systems for Video Technology
- Jianshu Zhang, Jun Du, Yongxin Yang, Yi-Zhe Song, Lirong Dai, SRD: A Tree Structure Based Decoder for Online Handwritten Mathematical Expression Recognition, IEEE Transactions on Multimedia
2019
- Da Li, Jianshu Zhang, Yongxin Yang, Cong Liu, Yi-Zhe Song, Timothy Hospedales, Episodic Training for Domain Generalization, International Conference on Computer Vision (ICCV 2019, Oral)
- Umar Riaz Muhammad, Yongxin Yang, Timothy Hospedales, Tao Xiang, Yi-Zhe Song, Goal-Driven Sequential Data Abstraction, International Conference on Computer Vision (ICCV 2019)
- Kaiyue Pang, Ke Li, Yongxin Yang, Timothy Hospedales, Tao Xiang, Yi-Zhe Song, Generalising Fine-Grained Sketch-Based Image Retrieval, IEEE Conference on Computer Vision and Pattern Recognition (CVPR 2019)
- Jifei Song, Yongxin Yang, Yi-Zhe Song, Tao Xiang, Timothy Hospedales, Generalizable Person Re-identification by Domain-Invariant Mapping Network, IEEE Conference on Computer Vision and Pattern Recognition (CVPR 2019)
- Sounak Dey, Pau Riba, Anjan Dutta, Josep Llados, Yi-Zhe Song, Doodle to Search: Practical Zero-Shot Sketch-based Image Retrieval, IEEE Conference on Computer Vision and Pattern Recognition (CVPR 2019, Oral)
- K. Li, K. Pang, Y. Song, T. Xiang, T. M. Hospedales, H. Zhang, Towards A Deep Universal Sketch Perceptual Grouper, IEEE Transactions on Image Processing IEEE Transactions on Image Processing
- Zhanyu Ma, Yuping Lai, W. Bastiaan Kleijn, Yi-Zhe Song, Liang Wang, Jun Guo, Variational Bayesian Learning for Dirichlet Process Mixture of Inverted Dirichlet Distributions in Non-Gaussian Image Feature Modeling, IEEE Transactions on Neural Networks Learning Systems
- Yonggang Qi, Yi-Zhe Song, Sketch Fewer to Recognize More by Learning A Co-regularized Sparse Representation, IEEE Transactions on Circuits and Systems for Video Technology
2018
- Kaiyue Pang, Da Li, Jifei Song, Yi-Zhe Song, Tao Xiang, Timothy Hospedales, Deep Factorised Inverse-Sketching, European Conference on Computer Vision (ECCV 2018)
- Changqing Zou*, Qian Yu*, Ruofei Du, Haoran Mo, Yi-Zhe Song, Tao Xiang, Chengying Gao, Baoquan Chen, and Hao Zhang, SketchyScene: Richly-Annotated Scene Sketches, European Conference on Computer Vision (ECCV 2018)
- Ke Li, Kaiyue Pang, Jifei Song, Yi-Zhe Song, Tao Xiang, Timothy Hospedales, Universal Sketch Perceptual Grouping, European Conference on Computer Vision (ECCV 2018)
- Jifei Song, Kaiyue Pang, Yi-Zhe Song, Tao Xiang, Tim Hospedales, Learning to Sketch with Shortcut Cycle Consistency, IEEE Conference on Computer Vision and Pattern Recognition (CVPR 2018)
- Conghui Hu, Da Li, Yi-Zhe Song, Tao Xiang, Tim Hospedales, Sketch-a-Classifier: Sketch-based Photo Classifier Generation, IEEE Conference on Computer Vision and Pattern Recognition (CVPR 2018)
- Umar Muhammad, Yongxin Yang, Yi-Zhe Song, Tao Xiang, Tim Hospedales, Learning Deep Sketch Abstraction, IEEE Conference on Computer Vision and Pattern Recognition (CVPR 2018)
- Peng Xu, Yongye Huang, Tongtong Yuan, Kaiyue Pang, Yi-Zhe Song, Tao Xiang, Tim Hospedales, Zhanyu Ma, Jun Guo, SketchMate: Deep Hashing for Million-Scale Human Sketch Retrieval, IEEE Conference on Computer Vision and Pattern Recognition (CVPR 2018)
- Da Li, Yongxin Yang, Yi-Zhe Song and Timothy M. Hospedales, Learning to Generalize: Meta-Learning for Domain Generalization, AAAI Conference on Artificial Intelligence (AAAI 2018)
- L Pang, Y Wang, YZ Song, T Huang, Y Tian, Cross-Domain Adversarial Feature Learning for Sketch Re-identification, ACM Multimedia Conference on Multimedia (ACM MM 2018)
2017
- Da Li, Yongxin Yang, Yi-Zhe Song and Timothy M. Hospedales, Deeper, Broader and Artier Domain Generalization, International Conference on Computer Vision (ICCV 2017)
- Jifei Song∗, Qian Yu∗, Yi-Zhe Song, Tao Xiang and Timothy M. Hospedales, Deep Spatial-Semantic Attention for Fine-Grained Sketch-Based Image Retrieval, International Conference on Computer Vision (ICCV 2017) (* Equal Contribution)
- Kaiyue Pang, Yi-Zhe Song, Tao Xiang and Timothy M. Hospedales, Cross-domain Generative Learning for Fine-Grained Sketch-Based Image Retrieval, British Machine Vision Conference (BMVC 2017), Poster
- Conghui Hu, Da Li, Yi-Zhe Song and Timothy M. Hospedales, Now You See Me: Deep Face Hallucination for Unviewed Sketches, British Machine Vision Conference (BMVC 2017), Oral
- Jifei Song, Yi-Zhe Song, Tao Xiang and Timothy M. Hospedales, Fine-Grained Image Retrieval: the Text/Sketch Input Dilemma, British Machine Vision Conference (BMVC 2017), Poster
- Ke Li, Kaiyue Pang, Yi-Zhe Song, Timothy M. Hospedales, Tao Xiang, Honggang Zhang, Synergistic Instance-Level Subspace Alignment for Fine-Grained Sketch-based Image Retrieval, IEEE Transactions on Image Processing (IEEE TIP)
2016
- S Ouyang, T Hospedales, YZ Song, X Li, CC Loy, X Wang, A survey on heterogeneous face recognition: Sketch, infra-red, 3D and low-resolution, Image and Vision Computing
- Y. Li, Y-Z. Song, T. Hospedales and S. Gong, Free-hand sketch synthesis with deformable stroke models, International Journal of Computer Vision (IJCV)
- Qian Yu, Feng Liu, Yi-Zhe Song, Tao Xiang, Timothy M. Hospedales, Chen Change Loy, Sketch Me That Shoe, IEEE Conference on Computer Vision and Pattern Recognition (CVPR 2016, Oral)
- Shuxin Ouyang, Timothy M. Hospedales, Yi-Zhe Song and Xueming Li, ForgetMeNot: Memory-Aware Forensic Facial Sketch Matching, IEEE Conference on Computer Vision and Pattern Recognition (CVPR 2016, Spotlight)
- J. Song, Y-Z. Song, T. Xiang, T. Hospedales and X. Ruan, Deep Multi-task Attribute-driven Ranking for Fine-grained Sketch-based Image Retrieval, British Machine Vision Conference (BMVC 2016, Oral)
- Q. Yu, Y. Yang, F. Liu, Y-Z. Song, T. Xiang and T. Hospedales, Sketch-a-Net: a Deep Neural Network that Beats Humans, International Journal of Computer Vision (IJCV)
- Ke Li, Kaiyue Pang, Yi-Zhe Song, Timothy Hospedales, Honggang Zhang and Yichuan Hu, Fine-Grained Sketch-Based Image Retrieval: The Role of Part-Aware Attributes, IEEE Winter Conference on Applications of Computer Vision (WACV 2016)
- Yonggang Qi, Yi-Zhe Song, Honggang Zhang, Jun Liu, Sketch-Based Image Retrieval via Siamese Convolutional Neural Network, IEEE International Conference on Image Processing (ICIP 2016)
2015
- Q. Yu, Y. Yang, Y-Z. Song, T. Xiang and T. Hospedales, Sketch-a-Net that Beats Humans, British Machine Vision Conference (BMVC 2015, Best Paper Award)
- Yonggang Qi, Yi-Zhe Song, Tao Xiang, Honggang Zhang, Timothy Hospedales, Yi Li and Jun Guo, Making Better Use of Edges via Perceptual Grouping, IEEE Conference on Computer Vision and Pattern Recognition (CVPR 2015)
- Yi Li, Timothy M. Hospedales, Yi-Zhe Song and Shaogang Gong, Free-hand Sketch Recognition by Multi-Kernel Feature Learning, Computer Vision and Image Understanding (CVIU)
- Yonggang Qi, Jun Guo, Yi-Zhe Song, Tao Xiang, Honggang Zhang, Zheng-Hua Tan, Im2Sketch: Sketch generation by unconflicted perceptual grouping, Neurocomputing
2014
- S. Ouyang, T. Hospedales, Y.-Z. Song and X. Li, Cross-Modal Face Matching: Beyond Viewed Sketches, Asian Conference on Computer Vision (ACCV 2014)
- S. Ouyang, T. Hospedales, Y.-Z. Song and X. Li, A Survey on Heterogeneous Face Recognition: Sketch, Infra-red, 3D and Low-resolution, Image and Vision Computing (IVC)
- Y. Li, T. Hospedales, Y.-Z. Song and S. Gong, Fine-grained sketch-based image retrieval by matching deformable part models, British Machine Vision Conference (BMVC 2014)
2013
- Yonggang Qi, Jun Guo, Yi Li, Honggang Zhang, Tao Xiang, Yi-Zhe Song, Sketching by perceptual grouping, IEEE International Conference on Image Processing (ICIP 2013)
- Yi Li, T. Hospedales, Yi-Zhe Song, Shaogang Gong, Sketch Recognition by Ensemble Matching of Structured Features, British Machine Vision Conference (BMVC 2013)
- Yi-Zhe Song, David Pickup, Chuan Li, Paul Rosin, Peter S Hall, Abstract art by shape classification, IEEE Transactions on Visualization and Computer Graphics (IEEE TVCG)