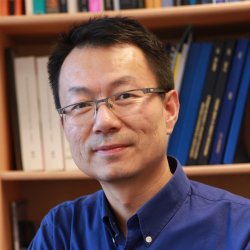
Professor Tao Xiang
Academic and research departments
Faculty of Engineering and Physical Sciences, Centre for Vision, Speech and Signal Processing (CVSSP).About
Biography
Tao Xiang is Professor of Computer Vision and Machine Learning and Distinguished Chair at the University of Surrey. He is also a Principal Researcher at Samsung AI Centre, Cambridge where he leads the Body Behaviour Group. Xiang’s research in computer vision has focused on video surveillance, daily activity analysis, and sketch analysis. He also has interests in large-scale machine learning problems including zero/few-shot learning and domain generalisation. He has published over 160 papers with 11K citations (h-index 60). Xiang serves as an area chair for computer vision conferences including ICCV and BMVC. He has secured research grants in excess of £3.9M.
Publications
Machine learning models typically suffer from the domain shift problem when trained on a source dataset and evaluated on a target dataset of different distribution. To overcome this problem, domain generalisation (DG) methods aim to leverage data from multiple source domains so that a trained model can generalise to unseen domains. In this paper, we propose a novel DG approach based on Deep Domain-Adversarial Image Generation (DDAIG). Specifically, DDAIG consists of three components, namely a label classifier, a domain classifier and a domain transformation network (DoTNet). The goal for DoTNet is to map the source training data to unseen domains. This is achieved by having a learning objective formulated to ensure that the generated data can be correctly classified by the label classifier while fooling the domain classifier. By augmenting the source training data with the generated unseen domain data, we can make the label classifier more robust to unknown domain changes. Extensive experiments on four DG datasets demonstrate the effectiveness of our approach.
The study of neural generative models of human sketches is a fascinating contemporary modeling problem due to the links between sketch image generation and the human drawing process. The landmark SketchRNN provided breakthrough by sequentially generating sketches as a sequence of waypoints. However this leads to low-resolution image generation, and failure to model long sketches. In this paper we present B´ezierSketch, a novel generative model for fully vector sketches that are automatically scalable and high-resolution. To this end, we first introduce a novel inverse graphics approach to stroke embedding that trains an encoder to embed each stroke to its best fit B´ezier curve. This enables us to treat sketches as short sequences of paramaterized strokes and thus train a recurrent sketch generator with greater capacity for longer sketches, while producing scalable high-resolution results. We report qualitative and quantitative results on the Quick, Draw! benchmark.
Sketches are highly expressive, inherently capturing subjective and fine-grained visual cues. The exploration of such innate properties of human sketches has, however, been limited to that of image retrieval. In this paper, for the first time, we cultivate the expressiveness of sketches but for the fundamental vision task of object detection. The end result is a sketch-enabled object detection framework that detects based on what \textit{you} sketch -- \textit{that} ``zebra'' (e.g., one that is eating the grass) in a herd of zebras (instance-aware detection), and only the \textit{part} (e.g., ``head" of a ``zebra") that you desire (part-aware detection). We further dictate that our model works without (i) knowing which category to expect at testing (zero-shot) and (ii) not requiring additional bounding boxes (as per fully supervised) and class labels (as per weakly supervised). Instead of devising a model from the ground up, we show an intuitive synergy between foundation models (e.g., CLIP) and existing sketch models build for sketch-based image retrieval (SBIR), which can already elegantly solve the task -- CLIP to provide model generalisation, and SBIR to bridge the (sketch$\rightarrow$photo) gap. In particular, we first perform independent prompting on both sketch and photo branches of an SBIR model to build highly generalisable sketch and photo encoders on the back of the generalisation ability of CLIP. We then devise a training paradigm to adapt the learned encoders for object detection, such that the region embeddings of detected boxes are aligned with the sketch and photo embeddings from SBIR. Evaluating our framework on standard object detection datasets like PASCAL-VOC and MS-COCO outperforms both supervised (SOD) and weakly-supervised object detectors (WSOD) on zero-shot setups. Project Page: \url{https://pinakinathc.github.io/sketch-detect}
Human sketch has already proved its worth in various visual understanding tasks (e.g., retrieval, segmentation, image-captioning, etc). In this paper, we reveal a new trait of sketches - that they are also salient. This is intuitive as sketching is a natural attentive process at its core. More specifically, we aim to study how sketches can be used as a weak label to detect salient objects present in an image. To this end, we propose a novel method that emphasises on how "salient object" could be explained by hand-drawn sketches. To accomplish this, we introduce a photo-to-sketch generation model that aims to generate sequential sketch coordinates corresponding to a given visual photo through a 2D attention mechanism. Attention maps accumulated across the time steps give rise to salient regions in the process. Extensive quantitative and qualitative experiments prove our hypothesis and delineate how our sketch-based saliency detection model gives a competitive performance compared to the state-of-the-art.
In this paper, we extend scene understanding to include that of human sketch. The result is a complete trilogy of scene representation from three diverse and complementary modalities -- sketch, photo, and text. Instead of learning a rigid three-way embedding and be done with it, we focus on learning a flexible joint embedding that fully supports the ``optionality" that this complementarity brings. Our embedding supports optionality on two axes: (i) optionality across modalities -- use any combination of modalities as query for downstream tasks like retrieval, (ii) optionality across tasks -- simultaneously utilising the embedding for either discriminative (e.g., retrieval) or generative tasks (e.g., captioning). This provides flexibility to end-users by exploiting the best of each modality, therefore serving the very purpose behind our proposal of a trilogy in the first place. First, a combination of information-bottleneck and conditional invertible neural networks disentangle the modality-specific component from modality-agnostic in sketch, photo, and text. Second, the modality-agnostic instances from sketch, photo, and text are synergised using a modified cross-attention. Once learned, we show our embedding can accommodate a multi-facet of scene-related tasks, including those enabled for the first time by the inclusion of sketch, all without any task-specific modifications. Project Page: \url{http://www.pinakinathc.me/scenetrilogy}
Given an abstract, deformed, ordinary sketch from untrained amateurs like you and me, this paper turns it into a photorealistic image - just like those shown in Fig. 1(a), all non-cherry-picked. We differ significantly from prior art in that we do not dictate an edgemap-like sketch to start with, but aim to work with abstract free-hand human sketches. In doing so, we essentially democratise the sketch-to-photo pipeline, "picturing" a sketch regardless of how good you sketch. Our contribution at the outset is a decoupled encoder-decoder training paradigm, where the decoder is a StyleGAN trained on photos only. This importantly ensures that generated results are always photorealistic. The rest is then all centred around how best to deal with the abstraction gap between sketch and photo. For that, we propose an autoregressive sketch mapper trained on sketch-photo pairs that maps a sketch to the StyleGAN latent space. We further introduce specific designs to tackle the abstract nature of human sketches, including a fine-grained discriminative loss on the back of a trained sketch-photo retrieval model, and a partial-aware sketch augmentation strategy. Finally, we showcase a few downstream tasks our generation model enables, amongst them is showing how fine-grained sketch-based image retrieval, a well-studied problem in the sketch community, can be reduced to an image (generated) to image retrieval task, surpassing state-of-the-arts. We put forward generated results in the supplementary for everyone to scrutinise.
This paper advances the fine-grained sketch-based image retrieval (FG-SBIR) literature by putting forward a strong baseline that overshoots prior state-of-the-arts by ~11%. This is not via complicated design though, but by addressing two critical issues facing the community (i) the gold standard triplet loss does not enforce holistic latent space geometry, and (ii) there are never enough sketches to train a high accuracy model. For the former, we propose a simple modification to the standard triplet loss, that explicitly enforces separation amongst photos/sketch instances. For the latter, we put forward a novel knowledge distillation module can leverage photo data for model training. Both modules are then plugged into a novel plug-n-playable training paradigm that allows for more stable training. More specifically, for (i) we employ an intra-modal triplet loss amongst sketches to bring sketches of the same instance closer from others, and one more amongst photos to push away different photo instances while bringing closer a structurally augmented version of the same photo (offering a gain of ~4-6%). To tackle (ii), we first pre-train a teacher on the large set of unlabelled photos over the aforementioned intra-modal photo triplet loss. Then we distill the contextual similarity present amongst the instances in the teacher's embedding space to that in the student's embedding space, by matching the distribution over inter-feature distances of respective samples in both embedding spaces (delivering a further gain of ~4-5%). Apart from outperforming prior arts significantly, our model also yields satisfactory results on generalising to new classes. Project page: https://aneeshan95.github.io/Sketch_PVT/
Vision transformers (ViTs) have pushed the state-of-the-art for various visual recognition tasks by patch-wise image tokenization followed by self-attention. However, the employment of self-attention modules results in a quadratic complexity in both computation and memory usage. Various attempts on approximating the self-attention computation with linear complexity have been made in Natural Language Processing. However, an in-depth analysis in this work shows that they are either theoretically flawed or empirically ineffective for visual recognition. We further identify that their limitations are rooted in keeping the softmax self-attention during approximations. Specifically, conventional self-attention is computed by normalizing the scaled dot-product between token feature vectors. Keeping this softmax operation challenges any subsequent linearization efforts. Based on this insight, for the first time, a softmax-free transformer or SOFT is proposed. To remove softmax in self-attention, Gaussian kernel function is used to replace the dot-product similarity without further normalization. This enables a full self-attention matrix to be approximated via a low-rank matrix decomposition. The robustness of the approximation is achieved by calculating its Moore-Penrose inverse using a Newton-Raphson method. Extensive experiments on ImageNet show that our SOFT significantly improves the computational efficiency of existing ViT variants. Crucially, with a linear complexity, much longer token sequences are permitted in SOFT, resulting in superior trade-off between accuracy and complexity.
We present the first competitive drawing agent Pixelor that exhibits human-level performance at a Pictionary-like sketching game, where the participant whose sketch is recognized first is a winner. Our AI agent can autonomously sketch a given visual concept, and achieve a recognizable rendition as quickly or faster than a human competitor. The key to victory for the agent’s goal is to learn the optimal stroke sequencing strategies that generate the most recognizable and distinguishable strokes first. Training Pixelor is done in two steps. First, we infer the stroke order that maximizes early recognizability of human training sketches. Second, this order is used to supervise the training of a sequence-to-sequence stroke generator. Our key technical contributions are a tractable search of the exponential space of orderings using neural sorting; and an improved Seq2Seq Wasserstein (S2S-WAE) generator that uses an optimal-transport loss to accommodate the multi-modal nature of the optimal stroke distribution. Our analysis shows that Pixelor is better than the human players of the Quick, Draw! game, under both AI and human judging of early recognition. To analyze the impact of human competitors’ strategies, we conducted a further human study with participants being given unlimited thinking time and training in early recognizability by feedback from an AI judge. The study shows that humans do gradually improve their strategies with training, but overall Pixelor still matches human performance. The code and the dataset are available at http://sketchx.ai/pixelor.
We present the first one-shot personalized sketch segmentation method. We aim to segment all sketches belonging to the same category provisioned with a single sketch with a given part annotation while (i) preserving the parts semantics embedded in the exemplar, and (ii) being robust to input style and abstraction. We refer to this scenario as personalized . With that, we importantly enable a much-desired personalization capability for downstream fine-grained sketch analysis tasks. To train a robust segmentation module, we deform the exemplar sketch to each of the available sketches of the same category. Our method generalizes to sketches not observed during training. Our central contribution is a sketch-specific hierarchical deformation network. Given a multi-level sketch-strokes encoding obtained via a graph convolutional network, our method estimates rigid-body transformation from the target to the exemplar, on the upper level. Finer deformation from the exemplar to the globally warped target sketch is further obtained through stroke-wise deformations, on the lower-level. Both levels of deformation are guided by mean squared distances between the keypoints learned without supervision, ensuring that the stroke semantics are preserved. We evaluate our method against the state-of-the-art segmentation and perceptual grouping baselines re-purposed for the one-shot setting and against two few-shot 3D shape segmentation methods. We show that our method outperforms all the alternatives by more than 10% on average. Ablation studies further demonstrate that our method is robust to personalization : changes in input part semantics and style differences.
In this paper, we focus on learning semantic representations for large-scale highly abstract sketches that were produced by the practical sketch-based application rather than the excessively well dawn sketches obtained by crowd-sourcing. We propose a dual-branch CNN-RNN network architecture to represent sketches, which simultaneously encodes both the static and temporal patterns of sketch strokes. Based on this architecture, we further explore learning the sketch-oriented semantic representations in two practical settings, i.e., hashing retrieval and zero-shot recognition on million-scale highly abstract sketches produced by practical online interactions. Specifically, we use our dual-branch architecture as a universal representation framework to design two sketch-specific deep models: (i) We propose a deep hashing model for sketch retrieval, where a novel hashing loss is specifically designed to further accommodate both the abstract and messy traits of sketches. (ii) We propose a deep embedding model for sketch zero-shot recognition, via collecting a large-scale edge-map dataset and proposing to extract a set of semantic vectors from edge-maps as the semantic knowledge for sketch zero-shot domain alignment. Both deep models are evaluated by comprehensive experiments on million-scale abstract sketches produced by a global online game QuickDraw and outperform state-of-the-art competitors.
Sketching enables many exciting applications, notably, image retrieval. The fear-to-sketch problem (i.e., "I can't sketch") has however proven to be fatal for its widespread adoption. This paper tackles this "fear" head on, and for the first time, proposes an auxiliary module for existing retrieval models that predominantly lets the users sketch without having to worry. We first conducted a pilot study that revealed the secret lies in the existence of noisy strokes, but not so much of the "I can't sketch". We consequently design a stroke subset selector that detects noisy strokes, leaving only those which make a positive contribution towards successful retrieval. Our Reinforcement Learning based formulation quantifies the importance of each stroke present in a given subset, based on the extent to which that stroke contributes to retrieval. When combined with pre-trained retrieval models as a pre-processing module, we achieve a significant gain of 8%40% over standard baselines and in turn report new state-of-the-art performance. Last but not least, we demonstrate the selector once trained, can also be used in a plug-and-play manner to empower various sketch applications in ways that were not previously possible.
Fine-grained sketch-based image retrieval (FG-SBIR) addresses the problem of retrieving a particular photo instance given a user’s query sketch. Its widespread applicability is however hindered by the fact that drawing a sketch takes time, and most people struggle to draw a complete and faithful sketch. In this paper, we reformulate the conventional FG-SBIR framework to tackle these challenges, with the ultimate goal of retrieving the target photo with the least number of strokes possible. We further propose an on-the-fly design that starts retrieving as soon as the user starts drawing. To accomplish this, we devise a reinforcement learning based cross-modal retrieval framework that directly optimizes rank of the ground-truth photo over a complete sketch drawing episode. Additionally, we introduce a novel reward scheme that circumvents the problems related to irrelevant sketch strokes, and thus provides us with a more consistent rank list during the retrieval. We achievesuperiorearly-retrievalefficiencyoverstate-of-theartmethodsandalternativebaselinesontwopubliclyavailable fine-grained sketch retrieval datasets.
We advance sketch research to scenes with the first dataset of freehand scene sketches, FS-COCO. With practical applications in mind, we collect sketches that convey scene content well but can be sketched within a few minutes by a person with any sketching skills. Our dataset comprises 10,000 freehand scene vector sketches with per point space-time information by 100 non-expert individuals, offering both object- and scene-level abstraction. Each sketch is augmented with its text description. Using our dataset, we study for the first time the problem of fine-grained image retrieval from freehand scene sketches and sketch captions. We draw insights on: (i) Scene salience encoded in sketches using the strokes temporal order; (ii) Performance comparison of image retrieval from a scene sketch and an image caption; (iii) Complementarity of information in sketches and image captions, as well as the potential benefit of combining the two modalities. In addition, we extend a popular vector sketch LSTM-based encoder to handle sketches with larger complexity than was supported by previous work. Namely, we propose a hierarchical sketch decoder, which we leverage at a sketch-specific ``pretext" task. Our dataset enables for the first time research on freehand scene sketch understanding and its practical applications. We release the dataset under CC BY-NC 4.0 license: https://fscoco.github.io
In this paper, we leverage CLIP for zero-shot sketch based image retrieval (ZS-SBIR). We are largely inspired by recent advances on foundation models and the unparalleled generalisation ability they seem to offer, but for the first time tailor it to benefit the sketch community. We put forward novel designs on how best to achieve this synergy, for both the category setting and the fine-grained setting ("all"). At the very core of our solution is a prompt learning setup. First we show just via factoring in sketch-specific prompts, we already have a category-level ZS-SBIR system that overshoots all prior arts, by a large margin (24.8%) - a great testimony on studying the CLIP and ZS-SBIR synergy. Moving onto the fine-grained setup is however trickier, and requires a deeper dive into this synergy. For that, we come up with two specific designs to tackle the fine-grained matching nature of the problem: (i) an additional regularisation loss to ensure the relative separation between sketches and photos is uniform across categories, which is not the case for the gold standard standalone triplet loss, and (ii) a clever patch shuffling technique to help establishing instance-level structural correspondences between sketch-photo pairs. With these designs, we again observe significant performance gains in the region of 26.9% over previous state-of-the-art. The take-home message, if any, is the proposed CLIP and prompt learning paradigm carries great promise in tackling other sketch-related tasks (not limited to ZS-SBIR) where data scarcity remains a great challenge. Project page: https://aneeshan95.github.io/Sketch_LVM/
3D shape modeling is labor-intensive and time-consuming and requires years of expertise. Recently, 2D sketches and text inputs were considered as conditional modalities to 3D shape generation networks to facilitate 3D shape modeling. However, text does not contain enough fine-grained information and is more suitable to describe a category or appearance rather than geometry, while 2D sketches are ambiguous, and depicting complex 3D shapes in 2D again requires extensive practice. Instead, we explore virtual reality sketches that are drawn directly in 3D. We assume that the sketches are created by novices, without any art training, and aim to reconstruct physically-plausible 3D shapes. Since such sketches are potentially ambiguous, we tackle the problem of the generation of multiple 3D shapes that follow the input sketch structure. Limited in the size of the training data, we carefully design our method, training the model step-by-step and leveraging multi-modal 3D shape representation. To guarantee the plausibility of generated 3D shapes we leverage the normalizing flow that models the distribution of the latent space of 3D shapes. To encourage the fidelity of the generated 3D models to an input sketch, we propose a dedicated loss that we deploy at different stages of the training process. We plan to make our code publicly available.
Zero-shot sketch-based image retrieval typically asks for a trained model to be applied as is to unseen categories. In this paper, we question to argue that this setup by definition is not compatible with the inherent abstract and subjective nature of sketches - the model might transfer well to new categories, but will not understand sketches existing in different test-time distribution as a result. We thus extend ZS-SBIR asking it to transfer to both categories and sketch distributions. Our key contribution is a test-time training paradigm that can adapt using just one sketch. Since there is no paired photo, we make use of a sketch raster-vector reconstruction module as a self-supervised auxiliary task. To maintain the fidelity of the trained cross-modal joint embedding during test-time update, we design a novel metal-earning based training paradigm to learn a separation between model updates incurred by this auxiliary task from those off the primary objective of discriminative learning. Extensive experiments show our model to outperform state-of-the-arts, thanks to the proposed test-time adaption that not only transfers to new categories but also accommodates to new sketching styles.
The recent focus on Fine-Grained Sketch-Based Image Retrieval (FG-SBIR) has shifted towards generalising a model to new categories without any training data from them. In real-world applications, however, a trained FG-SBIR model is often applied to both new categories and different human sketchers, i.e., different drawing styles. Although this complicates the generalisation problem, fortunately, a handful of examples are typically available, enabling the model to adapt to the new category/style. In this paper, we offer a novel perspective - instead of asking for a model that generalises, we advocate for one that quickly adapts, with just very few samples during testing (in a few-shot manner). To solve this new problem, we introduce a novel model-agnostic meta-learning (MAML) based framework with several key modifications: (1) As a retrieval task with a margin-based contrastive loss, we simplify the MAML training in the inner loop to make it more stable and tractable. (2) The margin in our contrastive loss is also meta-learned with the rest of the model. (3) Three additional regularisation losses are introduced in the outer loop, to make the meta-learned FG-SBIR model more effective for category/style adaptation. Extensive experiments on public datasets suggest a large gain over generalisation and zero-shot based approaches, and a few strong few-shot baselines.
We scrutinise an important observation plaguing scene-level sketch research - that a significant portion of scene sketches are "partial". A quick pilot study reveals: (i) a scene sketch does not necessarily contain all objects in the corresponding photo, due to the subjective holistic interpretation of scenes, (ii) there exists significant empty (white) regions as a result of object-level abstraction, and as a result, (iii) existing scene-level fine-grained sketch-based image retrieval methods collapse as scene sketches become more partial. To solve this "partial" problem, we advocate for a simple set-based approach using optimal transport (OT) to model cross-modal region associativity in a partially-aware fashion. Importantly, we improve upon OT to further account for holistic partialness by comparing intra-modal adjacency matrices. Our proposed method is not only robust to partial scene-sketches but also yields state-of-the-art performance on existing datasets.
Sketches are highly expressive, inherently capturing subjective and fine-grained visual cues. The exploration of such innate properties of human sketches has, however, been limited to that of image retrieval. In this paper, for the first time, we cultivate the expressiveness of sketches but for the fundamental vision task of object detection. The end result is a sketch-enabled object detection framework that detects based on what you sketch - that "zebra" (e.g., one that is eating the grass) in a herd of zebras (instance-aware detection), and only the part (e.g., "head" of a "zebra") that you desire (part-aware detection). We further dictate that our model works without (i) knowing which category to expect at testing (zero-shot) and (ii) not requiring additional bounding boxes (as per fully supervised) and class labels (as per weakly supervised). Instead of devising a model from the ground up, we show an intuitive synergy between foundation models (e.g., CLIP) and existing sketch models build for sketch-based image retrieval (SBIR), which can already elegantly solve the task - CLIP to provide model generalisation, and SBIR to bridge the (sketch→photo) gap. In particular, we first perform independent prompting on both sketch and photo branches of an SBIR model to build highly generalisable sketch and photo encoders on the back of the generalisation ability of CLIP. We then devise a training paradigm to adapt the learned encoders for object detection, such that the region embeddings of detected boxes are aligned with the sketch and photo embeddings from SBIR. Evaluating our framework on standard object detection datasets like PASCAL-VOC and MS-COCO outperforms both supervised (SOD) and weakly-supervised object detectors (WSOD) on zero-shot setups. Project Page: https://pinakinathc.github.io/sketch-detect
This paper advances the fine-grained sketch-based image retrieval (FG-SBIR) literature by putting forward a strong baseline that overshoots prior state-of-the-arts by ≈11 %. This is not via complicated design though, but by addressing two critical issues facing the community (i) the gold standard triplet loss does not enforce holistic latent space geometry, and (ii) there are never enough sketches to train a high accuracy model. For the former, we propose a simple modification to the standard triplet loss, that explicitly enforces separation amongst photos/sketch instances. For the latter, we put forward a novel knowledge distillation module can leverage photo data for model training. Both modules are then plugged into a novel plug-n-playable training paradigm that allows for more stable training. More specifically, for (i) we employ an intra-modal triplet loss amongst sketches to bring sketches of the same instance closer from others, and one more amongst photos to push away different photo instances while bringing closer a structurally augmented version of the same photo (offering a gain of ≈4-6%). To tackle (ii), we first pre-train a teacher on the large set of unlabelled photos over the aforementioned intra-modal photo triplet loss. Then we distill the contextual similarity present amongst the instances in the teacher's embedding space to that in the student's embedding space, by matching the distribution over inter-feature distances of respective samples in both embedding spaces (delivering a further gain of ≈ 4-5%). Apart from outperforming prior arts significantly, our model also yields satisfactory results on generalising to new classes. Project page: https://aneeshan95.github.io/Sketch_PVT/
Human sketch has already proved its worth in various visual understanding tasks (e.g., retrieval, segmentation, image-captioning, etc). In this paper, we reveal a new trait of sketches - that they are also salient. This is intuitive as sketching is a natural attentive process at its core. More specifically, we aim to study how sketches can be used as a weak label to detect salient objects present in an image. To this end, we propose a novel method that emphasises on how "salient object" could be explained by hand-drawn sketches. To accomplish this, we introduce a photo-to-sketch generation model that aims to generate sequential sketch coordinates corresponding to a given visual photo through a 2D attention mechanism. Attention maps accumulated across the time steps give rise to salient regions in the process. Extensive quantitative and qualitative experiments prove our hypothesis and delineate how our sketch-based saliency detection model gives a competitive performance compared to the state-of-the-art.
Given an abstract, deformed, ordinary sketch from untrained amateurs like you and me, this paper turns it into a photorealistic image - just like those shown in Fig. 1(a), all non-cherry-picked. We differ significantly from prior art in that we do not dictate an edgemap-like sketch to start with, but aim to work with abstract free-hand human sketches. In doing so, we essentially democratise the sketch-to-photo pipeline, "picturing" a sketch regardless of how good you sketch. Our contribution at the outset is a decoupled encoder-decoder training paradigm, where the decoder is a StyleGAN trained on photos only. This importantly ensures that generated results are always photorealistic. The rest is then all centred around how best to deal with the abstraction gap between sketch and photo. For that, we propose an autoregressive sketch mapper trained on sketch-photo pairs that maps a sketch to the StyleGAN latent space. We further introduce specific designs to tackle the abstract nature of human sketches, including a fine-grained discriminative loss on the back of a trained sketch-photo retrieval model, and a partial-aware sketch augmentation strategy. Finally, we showcase a few downstream tasks our generation model enables, amongst them is showing how fine-grained sketch-based image retrieval, a well-studied problem in the sketch community, can be reduced to an image (generated) to image retrieval task, surpassing state-of-the-arts. We put forward generated results in the supplementary for everyone to scrutinise. Project page: https://subhadeepkoley.github.io/PictureThatSketch
In this paper, we leverage CLIP for zero-shot sketch based image retrieval (ZS-SBIR). We are largely inspired by recent advances on foundation models and the unparalleled generalisation ability they seem to offer, but for the first time tailor it to benefit the sketch community. We put forward novel designs on how best to achieve this synergy, for both the category setting and the fine-grained setting ('all"}. At the very core of our solution is a prompt learning setup. First we show just via factoring in sketch-specific prompts, we already have a category-level ZS-SBIR system that over-shoots all prior arts, by a large margin (24.8%) - a great testimony on studying the CLIP and ZS-SBIR synergy. Moving onto the fine-grained setup is however trickier, and re-quires a deeper dive into this synergy. For that, we come up with two specific designs to tackle the fine-grained matching nature of the problem: (i) an additional regularisation loss to ensure the relative separation between sketches and photos is uniform across categories, which is not the case for the gold standard standalone triplet loss, and (ii) a clever patch shuffling technique to help establishing instance-level structural correspondences between sketch-photo pairs. With these designs, we again observe signifi-cant performance gains in the region of 26.9% over previ-ous state-of-the-art. The take-home message, if any, is the proposed CLIP and prompt learning paradigm carries great promise in tackling other sketch-related tasks (not limited to ZS-SBIR) where data scarcity remains a great challenge. Project page: https://aneeshan95.github.ioISketchLVM/
In this paper, we extend scene understanding to include that of human sketch. The result is a complete trilogy of scene representation from three diverse and complementary modalities - sketch, photo, and text. Instead of learning a rigid three-way embedding and be done with it, wefocus on learning a flexible joint embedding that fully supports the "optionality" that this complementarity brings. Our embedding supports optionality on two axes: (i) optionality across modalities - use any combination of modalities as query for downstream tasks like retrieval, (ii) optionality across tasks - simultaneously utilising the embedding for either discriminative (e.g., retrieval) or generative tasks (e.g., captioning). This provides flexibility to end-users by exploiting the best of each modality, therefore serving the very purpose behind our proposal of a trilogy in the first place. First, a combination of information-bottleneck and conditional invertible neural networks disentangle the modality-specific component from modality-agnostic in sketch, photo, and text. Second, the modality-agnostic instances from sketch, photo, and text are synergised using a modified cross-attention. Once learned, we show our embedding can accommodate a multi-facet of scene-related tasks, including those enabled for the first time by the inclusion of sketch, all without any task-specific modifications. Project Page: https://pinakinathc.github.io/scenetrilogy
Generalized Few-shot Semantic Segmentation (GFSS) aims to segment each image pixel into either base classes with abundant training examples or novel classes with only a handful of (e.g., 1-5) training images per class. Compared to the widely studied Few-shot Semantic Segmentation (FSS), which is limited to segmenting novel classes only, GFSS is much under-studied despite being more practical. Existing approach to GFSS is based on classifier parameter fusion whereby a newly trained novel class classifier and a pre-trained base class classifier are combined to form a new classifier. As the training data is dominated by base classes, this approach is inevitably biased towards the base classes. In this work, we propose a novel Prediction Calibration Network (PCN) to address this problem. Instead of fusing the classifier parameters, we fuse the scores produced separately by the base and novel classifiers. To ensure that the fused scores are not biased to either the base or novel classes, a new Transformer-based calibration module is introduced. It is known that the lower-level features are useful of detecting edge information in an input image than higher level features. Thus, we build a cross-attention module that guides the classifier's final prediction using the fused multi-level features. However, transformers are computationally demanding. Crucially, to make the proposed cross-attention module training tractable at the pixel level, this module is designed based on feature-score cross-covariance and episodically trained to be generalizable at inference time. Extensive experiments on PASCAL-5(i) and COCO-20(i) show that our PCN outperforms the state-the-the-art alternatives by large margins.
Precipitation clouds are visible aggregates of hydrometeor in the air that floating in the atmosphere after condensation, which can be divided into stratiform cloud and convective cloud. Different precipitation clouds often accompany different precipitation processes. Accurate identification of precipitation clouds is significant for the prediction of severe precipitation processes. Traditional identification methods mostly depend on the differences of radar reflectivity distribution morphology between stratiform and convective precipitation clouds in three-dimensional space. This paper proposes a new method for precipitation clouds identification based on deep learning algorithm. It mainly includes two parts, which are constant altitude plan position indicator data (CAPPI) inversion for radar reflectivity, and the precipitation clouds identification based on Faster-RCNN. The testing result shows that the method proposed in this paper performs better than typical existing algorithms in terms of accuracy rate. Moreover, this method boasts great advantages in running time and adaptive ability.
Most existing action recognition models are large convolutional neural networks that work only with raw RGB frames as input. However, practical applications require lightweight models that directly process compressed videos. In this work, for the first time, such a model is developed, which is lightweight enough to run in real-time on embedded AI devices without sacrifices in recognition accuracy. A new Aligned Temporal Trilinear Pooling (ATTP) module is formulated to fuse three modalities in a compressed video. To remedy the weaker motion vectors (compared to optical flow computed from raw RGB streams) for representing dynamic content, we introduce a temporal fusion method to explicitly induce the temporal context, as well as knowledge distillation from a model trained with optical flows via feature alignment. Compared to existing compressed video action recognition models, it is much more compact and faster thanks to adopting a lightweight CNN backbone.
Person re-identification (Re-ID) aims to match a target person across camera views at different locations and times. Existing Re-ID studies focus on the short-term cloth-consistent setting, under which a person re-appears in different camera views with the same outfit. A discriminative feature representation learned by existing deep Re-ID models is thus dominated by the visual appearance of clothing. In this work, we focus on a much more difficult yet practical setting where person matching is conducted over long-duration, e.g., over days and months and therefore inevitably under the new challenge of changing clothes. This problem, termed Long-Term Cloth-Changing (LTCC) Re-ID is much understudied due to the lack of large scale datasets. The first contribution of this work is a new LTCC dataset containing people captured over a long period of time with frequent clothing changes. As a second contribution, we propose a novel Re-ID method specifically designed to address the cloth-changing challenge. Specifically, we consider that under cloth-changes, soft-biometrics such as body shape would be more reliable. We, therefore, introduce a shape embedding module as well as a cloth-elimination shape-distillation module aiming to eliminate the now unreliable clothing appearance features and focus on the body shape information. Extensive experiments show that superior performance is achieved by the proposed model on the new LTCC dataset. The dataset is available on the project website: https://naiq.github.io/LTCC_Perosn_ReID.html.
This paper focuses on domain generalization (DG), the task of learning from multiple source domains a model that generalizes well to unseen domains. A main challenge for DG is that the available source domains often exhibit limited diversity, hampering the model’s ability to learn to generalize. We therefore employ a data generator to synthesize data from pseudo-novel domains to augment the source domains. This explicitly increases the diversity of available training domains and leads to a more generalizable model. To train the generator, we model the distribution divergence between source and synthesized pseudo-novel domains using optimal transport, and maximize the divergence. To ensure that semantics are preserved in the synthesized data, we further impose cycle-consistency and classification losses on the generator. Our method, L2A-OT (Learning to Augment by Optimal Transport) outperforms current state-of-the-art DG methods on four benchmark datasets.
A fundamental challenge faced by existing Fine-Grained Sketch-Based Image Retrieval (FG-SBIR) models is the data scarcity – model performances are largely bottlenecked by the lack of sketch-photo pairs. Whilst the number of photos can be easily scaled, each corresponding sketch still needs to be individually produced. In this paper, we aim to mitigate such an upper-bound on sketch data, and study whether unlabelled photos alone (of which they are many) can be cultivated for performance gain. In particular, we introduce a novel semi-supervised framework for cross-modal retrieval that can additionally leverage large-scale unla-belled photos to account for data scarcity. At the center of our semi-supervision design is a sequential photo-to-sketch generation model that aims to generate paired sketches for unlabelled photos. Importantly, we further introduce a discriminator-guided mechanism to guide against unfaithful generation, together with a distillation loss-based regu-larizer to provide tolerance against noisy training samples. Last but not least, we treat generation and retrieval as two conjugate problems, where a joint learning procedure is devised for each module to mutually benefit from each other. Extensive experiments show that our semi-supervised model yields a significant performance boost over the state-of-the-art supervised alternatives, as well as existing methods that can exploit unlabelled photos for FG-SBIR.
A common strategy adopted by existing state-of-the-art unsupervised domain adaptation (UDA) methods is to employ two classifiers to identify the misaligned local regions between source and target domain. Following the 'wisdom of the crowd' principle, one has to ask: why stop at two? Indeed, we find that using more classifiers leads to better performance, but also introduces more model parameters, therefore risking overfitting. In this paper, we introduce a novel method called STochastic clAssifieRs (STAR) for addressing this problem. Instead of representing one classifier as a weight vector, STAR models it as a Gaussian distribution with its variance representing the inter-classifier discrepancy. With STAR, we can now sample an arbitrary number of classifiers from the distribution, whilst keeping the model size the same as having two classifiers. Extensive experiments demonstrate that a variety of existing UDA methods can greatly benefit from STAR and achieve the state-of-the-art performance on both image classification and semantic segmentation tasks.
We present the first competitive drawing agent Pixelor that exhibits human-level performance at a Pictionary-like sketching game, where the participant whose sketch is recognized first is a winner. Our AI agent can autonomously sketch a given visual concept, and achieve a recognizable rendition as quickly or faster than a human competitor. The key to victory for the agent’s goal is to learn the optimal stroke sequencing strategies that generate the most recognizable and distinguishable strokes first. Training Pixelor is done in two steps. First, we infer the stroke order that maximizes early recognizability of human training sketches. Second, this order is used to supervise the training of a sequence-to-sequence stroke generator. Our key technical contributions are a tractable search of the exponential space of orderings using neural sorting; and an improved Seq2Seq Wasserstein (S2S-WAE) generator that uses an optimal-transport loss to accommodate the multi-modal nature of the optimal stroke distribution. Our analysis shows that Pixelor is better than the human players of the Quick, Draw! game, under both AI and human judging of early recognition. To analyze the impact of human competitors’ strategies, we conducted a further human study with participants being given unlimited thinking time and training in early recognizability by feedback from an AI judge. The study shows that humans do gradually improve their strategies with training, but overall Pixelor still matches human performance. The code and the dataset are available at http://sketchx.ai/pixelor.
Existing sketch-analysis work studies sketches depicting static objects or scenes. In this work, we propose a novel cross-modal retrieval problem of fine-grained instance-level sketch-based video retrieval (FG-SBVR), where a sketch sequence is used as a query to retrieve a specific target video instance. Compared with sketch-based still image retrieval, and coarse-grained category-level video retrieval, this is more challenging as both visual appearance and motion need to be simultaneously matched at a fine-grained level. We contribute the first FG-SBVR dataset with rich annotations. We then introduce a novel multi-stream multi-modality deep network to perform FG-SBVR under both strong and weakly supervised settings. The key component of the network is a relation module, designed to prevent model overfitting given scarce training data. We show that this model significantly outperforms a number of existing state-of-the-art models designed for video analysis.
In this paper we study, for the first time, the problem of fine-grained sketch-based 3D shape retrieval. We advocate the use of sketches as a fine-grained input modality to retrieve 3D shapes at instance-level - e.g., given a sketch of a chair, we set out to retrieve a specific chair from a gallery of all chairs. Fine-grained sketch-based 3D shape retrieval (FG-SBSR) has not been possible till now due to a lack of datasets that exhibit one-to-one sketch-3D correspondences. The first key contribution of this paper is two new datasets, consisting a total of 4,680 sketch-3D pairings from two object categories. Even with the datasets, FG-SBSR is still highly challenging because (i) the inherent domain gap between 2D sketch and 3D shape is large, and (ii) retrieval needs to be conducted at the instance level instead of the coarse category level matching as in traditional SBSR. Thus, the second contribution of the paper is the first cross-modal deep embedding model for FG-SBSR, which specifically tackles the unique challenges presented by this new problem. Core to the deep embedding model is a novel cross-modal view attention module which automatically computes the optimal combination of 2D projections of a 3D shape given a query sketch.
The goal of few-shot learning (FSL) is to recognize a set of novel classes with only few labeled samples by exploiting a large set of abundant base class samples. Adopting a meta-learning framework, most recent FSL methods meta-learn a deep feature embedding network, and during inference classify novel class samples using nearest neighbor in the learned high-dimensional embedding space. This means that these methods are prone to the hubness problem, that is, a certain class prototype becomes the nearest neighbor of many test instances regardless which classes they belong to. However, this problem is largely ignored in existing FSL studies. In this work, for the first time we show that many FSL methods indeed suffer from the hubness problem. To mitigate its negative effects, we further propose to employ z-score feature normalization, a simple yet effective trans-formation, during meta-training. A theoretical analysis is provided on why it helps. Extensive experiments are then conducted to show that with z-score normalization, the performance of many recent FSL methods can be boosted, resulting in new state-of-the-art on three benchmarks.
Existing few-shot learning (FSL) methods assume that there exist sufficient training samples from source classes for knowledge transfer to target classes with few training samples. However, this assumption is often invalid, especially when it comes to fine-grained recognition. In this work, we define a new FSL setting termed scarce-source few-shot learning (SSFSL), under which both the source and target classes have limited training samples. To overcome the source class data scarcity problem, a natural option is to crawl images from the web with class names as search keywords. However, the crawled images are inevitably corrupted by large amount of noise (irrelevant images) and thus may harm the performance. To address this problem, we propose a graph convolutional network (GCN)-based label denoising (LDN) method to remove the irrelevant images. Further, with the cleaned web images as well as the original clean training images, we propose a GCN-based FSL method. For both the LDN and FSL tasks, a novel adaptive aggregation GCN (AdarGCN) model is proposed, which differs from existing GCN models in that adaptive aggregation is performed based on a multi-head multi-level aggregation module. With AdarGCN, how much and how far information carried by each graph node is propagated in the graph structure can be determined automatically, therefore alleviating the effects of both noisy and outlying training samples. Extensive experiments demonstrate the superior performance of our AdarGCN under both the new SSFSL and the conventional FSL settings.
Existing few-shot learning (FSL) methods make the implicit assumption that the few target class samples are from the same domain as the source class samples. However, in practice, this assumption is often invalid - the target classes could come from a different domain. This poses an additional challenge of domain adaptation (DA) with few training samples. In this paper, the problem of domain-adaptive few-shot learning (DA-FSL) is tackled, which is expected to have wide use in real-world scenarios and requires solving FSL and DA in a unified framework. To this end, we propose a novel domain-adversarial prototypical network (DAPN) model. It is designed to address a specific challenge in DA-FSL: the DA objective means that the source and target data distributions need to be aligned, typically through a shared domain-adaptive feature embedding space; but the FSL objective dictates that the target domain per class distribution must be different from that of any source domain class, meaning aligning the distributions across domains may harm the FSL performance. How to achieve global domain distribution alignment whilst maintaining source/target per-class discriminativeness thus becomes the key. Our solution is to explicitly enhance the source/target per-class separation before domain-adaptive feature embedding learning, to alleviate the negative effect of domain alignment on FSL. Extensive experiments show that our DAPN outperforms the state-of-the-arts.
An effective person re-identification (re-ID) model should learn feature representations that are both discriminative, for distinguishing similar-looking people, and generalisable, for deployment across datasets without any adaptation. In this paper, we develop novel CNN architectures to address both challenges. First, we present a re-ID CNN termed omni-scale network (OSNet) to learn features that not only capture different spatial scales but also encapsulate a synergistic combination of multiple scales, namely omni-scale features. The basic building block consists of multiple convolutional streams, each detecting features at a certain scale. For omni-scale feature learning, a unified aggregation gate is introduced to dynamically fuse multi-scale features with channel-wise weights. OSNet is lightweight as its building blocks comprise factorised convolutions. Second, to improve generalisable feature learning, we introduce instance normalisation (IN) layers into OSNet to cope with cross-dataset discrepancies. Further, to determine the optimal placements of these IN layers in the architecture, we formulate an efficient differentiable architecture search algorithm. Extensive experiments show that, in the conventional same-dataset setting, OSNet achieves state-of-the-art performance, despite being much smaller than existing re-ID models. In the more challenging yet practical cross-dataset setting, OSNet beats most recent unsupervised domain adaptation methods without using any target data. Our code and models are released at https://github.com/KaiyangZhou/deep-person-reid .
The problem of generalizing deep neural networks from multiple source domains to a target one is studied under two settings: When unlabeled target data is available, it is a multi-source unsupervised domain adaptation (UDA) problem, otherwise a domain generalization (DG) problem. We propose a unified framework termed domain adaptive ensemble learning (DAEL) to address both problems. A DAEL model is composed of a CNN feature extractor shared across domains and multiple classifier heads each trained to specialize in a particular source domain. Each such classifier is an expert to its own domain but a non-expert to others. DAEL aims to learn these experts collaboratively so that when forming an ensemble, they can leverage complementary information from each other to be more effective for an unseen target domain. To this end, each source domain is used in turn as a pseudo-target-domain with its own expert providing supervisory signal to the ensemble of non-experts learned from the other sources. To deal with unlabeled target data under the UDA setting where real expert does not exist, DAEL uses pseudo labels to supervise the ensemble learning. Extensive experiments on three multi-source UDA datasets and two DG datasets show that DAEL improves the state of the art on both problems, often by significant margins.
Detecting subtle intention such as deception and subtext of a person in a long dialog video, or implicit intention detection (IID), is a challenging problem. The transcript (textual cues) often reveals little, so audio-visual cues including voice tone as well as facial and body behaviour are the main focuses for automated IID. Contextual cues are also crucial, since a person's implicit intentions are often correlated and context-dependent when the person moves from one question-answer pair to the next. However, no such dataset exists which contains fine-grained questionanswer pair (video segment) level annotation. The first contribution of this work is thus a new benchmark dataset, called Vid2Int-Deception to fill this gap. A novel multigrain representation model is also proposed to capture the subtle movement changes of eyes, face, and body (relevant for inferring intention) from a long dialog video. Moreover, to model the temporal correlation between the implicit intentions across video segments, we propose a Videoto-Intention network (Vid2Int) based on attentive recurrent neural network (RNN). Extensive experiments show that our model achieves state-of-the-art results.
The fundamental goal of artificial intelligence (AI) is to mimic the core cognitive activities of human. Despite tremendous success in the AI research, most of existing methods have only single-cognitive ability. To overcome this limitation and take a solid step towards artificial general intelligence (AGI), we develop a foundation model pre-trained with huge multimodal data, which can be quickly adapted for various downstream cognitive tasks. To achieve this goal, we propose to pre-train our foundation model by self-supervised learning with weak semantic correlation data crawled from the Internet and show that promising results can be obtained on a wide range of downstream tasks. Particularly, with the developed model-interpretability tools, we demonstrate that strong imagination ability is now possessed by our foundation model. We believe that our work makes a transformative stride towards AGI, from our common practice of "weak or narrow AI" to that of "strong or generalized AI". Artificial intelligence approaches inspired by human cognitive function have usually single learned ability. The authors propose a multimodal foundation model that demonstrates the cross-domain learning and adaptation for broad range of downstream cognitive tasks.
A deep facial attribute editing model strives to meet two requirements: (1) attribute correctness - the target attribute should correctly appear on the edited face image; (2) irrelevance preservation - any irrelevant information (e.g., identity) should not be changed after editing. Meeting both requirements challenges the state-of-the-art works which resort to either spatial attention or latent space factorization. Specifically, the former assume that each attribute has well-defined local support regions; they are often more effective for editing a local attribute than a global one. The latter factorize the latent space of a fixed pretrained GAN into different attribute-relevant parts, but they cannot be trained end-to-end with the GAN, leading to sub-optimal solutions. To overcome these limitations, we propose a novel latent space factorization model, called L2M-GAN, which is learned end-to-end and effective for editing both local and global attributes. The key novel components are: (1) A latent space vector of the GAN is factorized into an attribute-relevant and irrelevant codes with an orthogonality constraint imposed to ensure disentanglement. (2) An attribute-relevant code transformer is learned to manipulate the attribute value; crucially, the transformed code are subject to the same orthogonality constraint. By forcing both the original attribute-relevant latent code and the edited code to be disentangled from any attribute-irrelevant code, our model strikes the perfect balance between attribute correctness and irrelevance preservation. Extensive experiments on CelebA-HQ show that our L2M-GAN achieves significant improvements over the state-of-the-arts.
Stochastic neural networks (SNNs) are currently topical, with several paradigms being actively investigated including dropout, Bayesian neural networks, variational information bottleneck (VIB) and noise regularized learning. These neural network variants impact several major considerations, including generalization, network compression, robustness against adversarial attack and label noise, and model calibration. However, many existing networks are complicated and expensive to train, and/or only address one or two of these practical considerations. In this paper we propose a simple and effective stochastic neural network (SE-SNN) architecture for discriminative learning by directly modeling activation uncertainty and encouraging high activation variability. Compared to existing SNNs, our SE-SNN is simpler to implement and faster to train, and produces state of the art results on network compression by pruning, adversarial defense, learning with label noise, and model calibration.
Most recent few-shot learning (FSL) methods are based on meta-learning with episodic training. In each meta-training episode, a discriminative feature embedding and/or classifier are first constructed from a support set in an inner loop, and then evaluated in an outer loop using a query set for model updating. This query set sample centered learning objective is however intrinsically limited in addressing the lack of training data problem in the support set. In this paper, a novel contrastive prototype learning with augmented embeddings (CPLAE) model is proposed to overcome this limitation. First, data augmentations are introduced to both the support and query sets with each sample now being represented as an augmented embedding (AE) composed of concatenated embeddings of both the original and augmented versions. Second, a novel support set class prototype centered contrastive loss is proposed for contrastive prototype learning (CPL). With a class prototype as an anchor, CPL aims to pull the query samples of the same class closer and those of different classes further away. This support set sample centered loss is highly complementary to the existing query centered loss, fully exploiting the limited training data in each episode. Extensive experiments on several benchmarks demonstrate that our proposed CPLAE achieves new state-of-the-art.
Self-Supervised monocular visual odometry (VO) is often cast into a view synthesis problem based on depth and camera pose estimation. One of the key challenges is to accurately and robustly estimate depth with occlusions and moving objects in the scene. Existing methods simply detect and mask out regions of occlusions locally by several convolutional layers, and then perform only partial view synthesis in the rest of the image. However, occlusion and moving object detection is an unsolved problem itself which requires global layout information. Inaccurate detection inevitably results in incorrect depth as well as pose estimation. In this work, instead of locally detecting and masking out occlusions and moving objects, we propose to alleviate their negative effects on monocular VO implicitly but more effectively from two global perspectives. First, a multi-scale non-local attention module, consisting of both intra-stage augmented attention and cascaded across-stage attention, is proposed for robust depth estimation given occlusions, alleviating the impacts of occlusions via global attention modeling. Second, adversarial learning is introduced in view synthesis for monocular VO. Unlike existing methods that use pixel-level losses on the quality of synthesized views, we enforce the synthetic view to be indistinguishable from the real one at the scene-level. Such a global constraint again helps cope with occluded and moving regions. Extensive experiments on the KITTI dataset show that our approach achieves new state-of-the-art in both pose estimation and depth recovery.
Person re-identification (Re-ID) aims at retrieving a person of interest across multiple non-overlapping cameras. With the advancement of deep neural networks and increasing demand of intelligent video surveillance, it has gained significantly increased interest in the computer vision community. By dissecting the involved components in developing a person Re-ID system, we categorize it into the closed-world and open-world settings. The widely studied closed-world setting is usually applied under various research-oriented assumptions, and has achieved inspiring success using deep learning techniques on a number of datasets. We first conduct a comprehensive overview with in-depth analysis for closed-world person Re-ID from three different perspectives, including deep feature representation learning, deep metric learning and ranking optimization. With the performance saturation under closed-world setting, the research focus for person Re-ID has recently shifted to the open-world setting, facing more challenging issues. This setting is closer to practical applications under specific scenarios. We summarize the open-world Re-ID in terms of five different aspects. By analyzing the advantages of existing methods, we design a powerful AGW baseline, achieving state-of-the-art or at least comparable performance on twelve datasets for four different Re-ID tasks. Meanwhile, we introduce a new evaluation metric (mINP) for person Re-ID, indicating the cost for finding all the correct matches, which provides an additional criteria to evaluate the Re-ID system for real applications. Finally, some important yet under-investigated open issues are discussed.
3D shape modeling is labor-intensive, time-consuming, and requires years of expertise. To facilitate 3D shape modeling, we propose a 3D shape generation network that takes a 3D VR sketch as a condition. We assume that sketches are created by novices without art training and aim to reconstruct geometrically realistic 3D shapes of a given category. To handle potential sketch ambiguity, our method creates multiple 3D shapes that align with the original sketch’s structure. We carefully design our method, training the model step-by-step and leveraging multi-modal 3D shape representation to support training with limited training data. To guarantee the realism of generated 3D shapes we leverage the normalizing flow that models the distribution of the latent space of 3D shapes. To encourage the fidelity of the generated 3D shapes to an input sketch, we propose a dedicated loss that we deploy at different stages of the training process. The code is available at https://github.com/Rowl1ng/3Dsketch2shape.
The problem of fine-grained sketch-based image retrieval (FG-SBIR) is defined and investigated in this paper. In FG-SBIR, free-hand human sketch images are used as queries to retrieve photo images containing the same object instances. It is thus a cross-domain (sketch to photo) instance-level retrieval task. It is an extremely challenging problem because (i) visual comparisons and matching need to be executed under large domain gap, i.e., from black and white line drawing sketches to colour photos; (ii) it requires to capture the fine-grained (dis)similarities of sketches and photo images while free-hand sketches drawn by different people present different levels of deformation and expressive interpretation; and (iii) annotated cross-domain fine-grained SBIR datasets are scarce, challenging many state-of-the-art machine learning techniques, particularly those based on deep learning. In this paper, for the first time, we address all these challenges, providing a step towards the capabilities that would underpin a commercial sketch-based object instance retrieval application. Specifically, a new large-scale FG-SBIR database is introduced which is carefully designed to reflect the real-world application scenarios. A deep cross-domain matching model is then formulated to solve the intrinsic drawing style variability, large domain gap issues, and capture instance-level discriminative features. It distinguishes itself by a carefully designed attention module. Extensive experiments on the new dataset demonstrate the effectiveness of the proposed model and validate the need for a rigorous definition of the FG-SBIR problem and collecting suitable datasets.
Effectively solving the problem of sketch generation, which aims to produce human-drawing-like sketches from real photographs, opens the door for many vision applications such as sketch-based image retrieval and non-photorealistic rendering. In this paper, we approach automatic sketch generation from a human visual perception perspective. Instead of gathering insights from photographs, for the first time, we extract information from a large pool of human sketches. In particular, we study how multiple Gestalt rules can be encapsulated into a unified perceptual grouping framework for sketch generation. We further show that by solving the problem of Gestalt confliction, i.e., encoding the relative importance of each rule, more similar to human-made sketches can be generated. For that, we release a manually labeled sketch dataset of 96 object categories and 7680 sketches. A novel evaluation framework is proposed to quantify human likeness of machine-generated sketches by examining how well they can be classified using models trained from human data. Finally, we demonstrate the superiority of our sketches under the practical application of sketch-based image retrieval. © 2015 Elsevier B.V.
We propose a deep learning approach to free-hand sketch recognition that achieves state-of-the-art performance, significantly surpassing that of humans. Our superior performance is a result of modelling and exploiting the unique characteristics of free-hand sketches, i.e., consisting of an ordered set of strokes but lacking visual cues such as colour and texture, being highly iconic and abstract, and exhibiting extremely large appearance variations due to different levels of abstraction and deformation. Specifically, our deep neural network, termed Sketch-a-Net has the following novel components: (i) we propose a network architecture designed for sketch rather than natural photo statistics. (ii) Two novel data augmentation strategies are developed which exploit the unique sketch-domain properties to modify and synthesise sketch training data at multiple abstraction levels. Based on this idea we are able to both significantly increase the volume and diversity of sketches for training, and address the challenge of varying levels of sketching detail commonplace in free-hand sketches. (iii) We explore different network ensemble fusion strategies, including a re-purposed joint Bayesian scheme, to further improve recognition performance. We show that state-of-the-art deep networks specifically engineered for photos of natural objects fail to perform well on sketch recognition, regardless whether they are trained using photos or sketches. Furthermore, through visualising the learned filters, we offer useful insights in to where the superior performance of our network comes from. © 2016, Springer Science+Business Media New York.
We propose a deep learning approach to free-hand sketch recognition that achieves state-of-the-art performance, significantly surpassing that of humans. Our superior performance is a result of modelling and exploiting the unique characteristics of free-hand sketches, i.e., consisting of an ordered set of strokes but lacking visual cues such as colour and texture, being highly iconic and abstract, and exhibiting extremely large appearance variations due to different levels of abstraction and deformation. Specifically, our deep neural network, termed Sketch-a-Net has the following novel components: (i) we propose a network architecture designed for sketch rather than natural photo statistics. (ii) Two novel data augmentation strategies are developed which exploit the unique sketch-domain properties to modify and synthesise sketch training data at multiple abstraction levels. Based on this idea we are able to both significantly increase the volume and diversity of sketches for training, and address the challenge of varying levels of sketching detail commonplace in free-hand sketches. (iii) We explore different network ensemble fusion strategies, including a re-purposed joint Bayesian scheme, to further improve recognition performance. We show that state-of-the-art deep networks specifically engineered for photos of natural objects fail to perform well on sketch recognition, regardless whether they are trained using photos or sketches. Furthermore, through visualising the learned filters, we offer useful insights in to where the superior performance of our network comes from. © 2016, Springer Science+Business Media New York.
We study the problem of fine-grained sketch-based image retrieval. By performing instance-level (rather than category-level) retrieval, it embodies a timely and practical application, particularly with the ubiquitous availability of touchscreens. Three factors contribute to the challenging nature of the problem: 1) free-hand sketches are inherently abstract and iconic, making visual comparisons with photos difficult; 2) sketches and photos are in two different visual domains, i.e., black and white lines versus color pixels; and 3) fine-grained distinctions are especially challenging when executed across domain and abstraction-level. To address these challenges, we propose to bridge the image-sketch gap both at the high level via parts and attributes, as well as at the low level via introducing a new domain alignment method. More specifically, first, we contribute a data set with 304 photos and 912 sketches, where each sketch and image is annotated with its semantic parts and associated part-level attributes. With the help of this data set, second, we investigate how strongly supervised deformable part-based models can be learned that subsequently enable automatic detection of part-level attributes, and provide pose-aligned sketch-image comparisons. To reduce the sketch-image gap when comparing low-level features, third, we also propose a novel method for instance-level domain-alignment that exploits both subspace and instance-level cues to better align the domains. Finally, fourth, these are combined in a matching framework integrating aligned low-level features, mid-level geometric structure, and high-level semantic attributes. Extensive experiments conducted on our new data set demonstrate effectiveness of the proposed method.
Sketch-based image retrieval (SBIR) is challenging due to the inherent domain-gap between sketch and photo. Compared with pixel-perfect depictions of photos, sketches are iconic renderings of the real world with highly abstract. Therefore, matching sketch and photo directly using low-level visual clues are insufficient, since a common low-level subspace that traverses semantically across the two modalities is non-trivial to establish. Most existing SBIR studies do not directly tackle this cross-modal problem. This naturally motivates us to explore the effectiveness of cross-modal retrieval methods in SBIR, which have been applied in the image-text matching successfully. In this paper, we introduce and compare a series of state-of-the-art cross-modal subspace learning methods and benchmark them on two recently released fine-grained SBIR datasets. Through thorough examination of the experimental results, we have demonstrated that the subspace learning can effectively model the sketch-photo domain-gap. In addition we draw a few key insights to drive future research. © 2017 Elsevier B.V.
Person re-identification (re-id) aims to match people across non-overlapping camera views in a public space. This is a challenging problem because the people captured in surveillance videos often wear similar clothing. Consequently, the differences in their appearance are typically subtle and only detectable at particular locations and scales. In this paper, we propose a deep re-id network (MuDeep) that is composed of two novel types of layers – a multi-scale deep learning layer, and a leader-based attention learning layer. Specifically, the former learns deep discriminative feature representations at different scales, while the latter utilizes the information from multiple scales to lead and determine the optimal weightings for each scale. The importance of different spatial locations for extracting discriminative features is learned explicitly via our leader-based attention learning layer. Extensive experiments are carried out to demonstrate that the proposed MuDeep outperforms the state-of-the-art on a number of benchmarks and has a better generalization ability under a domain generalization setting.
Human free-hand sketches provide the useful data for studying human perceptual grouping, where the grouping principles such as the Gestalt laws of grouping are naturally in play during both the perception and sketching stages. In this paper, we make the first attempt to develop a universal sketch perceptual grouper. That is, a grouper that can be applied to sketches of any category created with any drawing style and ability, to group constituent strokes/segments into semantically meaningful object parts. The first obstacle to achieving this goal is the lack of large-scale datasets with grouping annotation. To overcome this, we contribute the largest sketch perceptual grouping dataset to date, consisting of 20 000 unique sketches evenly distributed over 25 object categories. Furthermore, we propose a novel deep perceptual grouping model learned with both generative and discriminative losses. The generative loss improves the generalization ability of the model, while the discriminative loss guarantees both local and global grouping consistency. Extensive experiments demonstrate that the proposed grouper significantly outperforms the state-of-the-art competitors. In addition, we show that our grouper is useful for a number of sketch analysis tasks, including sketch semantic segmentation, synthesis, and fine-grained sketch-based image retrieval. © 1992-2012 IEEE.
Sketch as an image search query is an ideal alternative to text in capturing the finegrained visual details. Prior successes on fine-grained sketch-based image retrieval (FGSBIR) have demonstrated the importance of tackling the unique traits of sketches as opposed to photos, e.g., temporal vs. static, strokes vs. pixels, and abstract vs. pixelperfect. In this paper, we study a further trait of sketches that has been overlooked to date, that is, they are hierarchical in terms of the levels of detail – a person typically sketches up to various extents of detail to depict an object. This hierarchical structure is often visually distinct. In this paper, we design a novel network that is capable of cultivating sketch-specific hierarchies and exploiting them to match sketch with photo at corresponding hierarchical levels. In particular, features from a sketch and a photo are enriched using cross-modal co-attention, coupled with hierarchical node fusion at every level to form a better embedding space to conduct retrieval. Experiments on common benchmarks show our method to outperform state-of-the-arts by a significant margin.
Sketch-based image retrieval (SBIR) is a cross-modal matching problem which is typically solved by learning a joint embedding space where the semantic content shared between photo and sketch modalities are preserved. However, a fundamental challenge in SBIR has been largely ignored so far, that is, sketches are drawn by humans and considerable style variations exist amongst different users. An effective SBIR model needs to explicitly account for this style diversity, crucially, to generalise to unseen user styles. To this end, a novel style-agnostic SBIR model is proposed. Different from existing models, a cross-modal variational autoencoder (VAE) is employed to explicitly disentangle each sketch into a semantic content part shared with the corresponding photo, and a style part unique to the sketcher. Importantly, to make our model dynamically adaptable to any unseen user styles, we propose to metatrain our cross-modal VAE by adding two style-adaptive components: a set of feature transformation layers to its encoder and a regulariser to the disentangled semantic content latent code. With this meta-learning framework, our model can not only disentangle the cross-modal shared semantic content for SBIR, but can adapt the disentanglement to any unseen user style as well, making the SBIR model truly style-agnostic. Extensive experiments show that our style-agnostic model yields state-of-the-art performance for both category-level and instance-level SBIR.
We study the practical task of fine-grained 3D-VRsketch-based 3D shape retrieval. This task is of particular interest as 2D sketches were shown to be effective queries for 2D images. However, due to the domain gap, it remains hard to achieve strong performance in 3D shape retrieval from 2D sketches. Recent work demonstrated the advantage of 3D VR sketching on this task. In our work, we focus on the challenge caused by inherent inaccuracies in 3D VR sketches. We observe that retrieval results obtained with a triplet loss with a fixed margin value, commonly used for retrieval tasks, contain many irrelevant shapes and often just one or few with a similar structure to the query. To mitigate this problem, we for the first time draw a connection between adaptive margin values and shape similarities. In particular, we propose to use a triplet loss with an adaptive margin value driven by a ‘fitting gap’, which is the similarity of two shapes under structure-preserving deformations. We also conduct a user study which confirms that this fitting gap is indeed a suitable criterion to evaluate the structural similarity of shapes. Furthermore, we introduce a dataset of 202 VR sketches for 202 3D shapes drawn from memory rather than from observation. The code and data are available at https://github.com/Rowl1ng/Structure-Aware-VR-Sketch-Shape-Retrieval
Self-supervised learning has gained prominence due to its efficacy at learning powerful representations from un-labelled data that achieve excellent performance on many challenging downstream tasks. However, supervision-free pretext tasks are challenging to design and usually modality specific. Although there is a rich literature of self-supervised methods for either spatial (such as images) or temporal data (sound or text) modalities, a common pretext task that benefits both modalities is largely missing. In this paper, we are interested in defining a self-supervised pretext task for sketches and handwriting data. This data is uniquely characterised by its existence in dual modalities of rasterized images and vector coordinate sequences. We address and exploit this dual representation by proposing two novel cross-modal translation pretext tasks for self-supervised feature learning: Vectorization and Rasteriza-tion. Vectorization learns to map image space to vector coordinates and rasterization maps vector coordinates to image space. We show that our learned encoder modules benefit both raster-based and vector-based downstream approaches to analysing hand-drawn data. Empirical evidence shows that our novel pretext tasks surpass existing single and multi-modal self-supervision methods.
Existing Temporal Action Detection (TAD) methods typ- ically take a pre-processing step in converting an input varying-length video into a fixed-length snippet represen- tation sequence, before temporal boundary estimation and action classification. This pre-processing step would tem- porally downsample the video, reducing the inference res- olution and hampering the detection performance in the original temporal resolution. In essence, this is due to a temporal quantization error introduced during the resolu- tion downsampling and recovery. This could negatively im- pact the TAD performance, but is largely ignored by existing methods. To address this problem, in this work we intro- duce a novel model-agnostic post-processing method with- out model redesign and retraining. Specifically, we model the start and end points of action instances with a Gaussian distribution for enabling temporal boundary inference at a sub-snippet level. We further introduce an efficient Taylor- expansion based approximation, dubbed as Gaussian Ap- proximated Post-processing (GAP). Extensive experiments demonstrate that our GAP can consistently improve a wide variety of pre-trained off-the-shelf TAD models on the chal- lenging ActivityNet (+0.2%∼0.7% in average mAP) and THUMOS (+0.2%∼0.5% in average mAP) benchmarks. Such performance gains are already significant and highly comparable to those achieved by novel model designs. Also, GAP can be integrated with model training for further performance gain. Importantly, GAP enables lower tem- poral resolutions for more efficient inference, facilitating low-resource applications. The code will be available in https://github.com/sauradip/GAP
A layout to image (L2I) generation model aims to generate a complicated image containing multiple objects (things) against natural background (stuff), conditioned on a given layout. Built upon the recent advances in gen-erative adversarial networks (GANs), existing L2I models have made great progress. However, a close inspection of their generated images reveals two major limitations: (1) the object-to-object as well as object-to-stuff relations are often broken and (2) each object's appearance is typically distorted lacking the key defining characteristics associated with the object class. We argue that these are caused by the lack of context-aware object and stuff feature encoding in their generators, and location-sensitive appearance representation in their discriminators. To address these limitations, two new modules are proposed in this work. First, a context-aware feature transformation module is introduced in the generator to ensure that the generated feature encoding of either object or stuff is aware of other co-existing objects/stuff in the scene. Second, instead of feeding location-insensitive image features to the discriminator, we use the Gram matrix computed from the feature maps of the generated object images to preserve location-sensitive information, resulting in much enhanced object appearance. Extensive experiments show that the proposed method achieves state-of-the-art performance on the COCO-Thing-Stuff and Visual Genome benchmarks. Code available at: https://github.com/wtliao/layout2img.
This paper, for the very first time, introduces human sketches to the landscape of XAI (Explainable Artificial Intelligence). We argue that sketch as a "human-centered" data form, represents a natural interface to study explainability. We focus on cultivating sketch-specific explainability designs. This starts by identifying strokes as a unique building block that offers a degree of flexibility in object construction and manipulation impossible in photos. Following this, we design a simple explainability-friendly sketch encoder that accommodates the intrinsic properties of strokes: shape, location, and order. We then define the first ever XAI task for sketch, that of stroke location inversion (SLI). Just as we have heat maps for photos and correlation matrices for text, SLI offers an explainability angle to sketch by asking a network how well it can recover stroke locations of an unseen sketch. We provide qualitative results for readers to interpret as snapshots of the SLI process in the paper and as GIFs on the project page. A minor but exciting note is that thanks to its sketch-specific design, our sketch encoder also yields the best sketch recognition accuracy to date while having the smallest number of parameters. The code is available at https://sketchxai.github.io.
FAME-ViL: Multi-Tasking Vision-Language Model for Heterogeneous Fashion Tasks Xiao Han1,2 Xiatian Zhu1,3 Licheng Yu Li Zhang4 Yi-Zhe Song1,2 Tao Xiang1,2 1 CVSSP, University of Surrey 2 iFlyTek-Surrey Joint Research Centre on Artificial Intelligence 3 Surrey Institute for People-Centred Artificial Intelligence 4 Fudan University {xiao.han, xiatian.zhu, y.song, t.xiang}@surrey.ac.uk lichengyu24@gmail.com lizhangfd@fudan.edu.cn Abstract In the fashion domain, there exists a variety of vision- and-language (V+L) tasks, including cross-modal retrieval, text-guided image retrieval, multi-modal classification, and image captioning. They differ drastically in each individ- ual input/output format and dataset size. It has been com- mon to design a task-specific model and fine-tune it in- dependently from a pre-trained V+L model (e.g., CLIP). This results in parameter inefficiency and inability to ex- ploit inter-task relatedness. To address such issues, we pro- pose a novel FAshion-focused Multi-task Efficient learn- ing method for Vision-and-Language tasks (FAME-ViL) in this work. Compared with existing approaches, FAME-ViL applies a single model for multiple heterogeneous fashion tasks, therefore being much more parameter-efficient. It is enabled by two novel components: (1) a task-versatile architecture with cross-attention adapters and task-specific adapters integrated into a unified V+L model, and (2) a sta- ble and effective multi-task training strategy that supports learning from heterogeneous data and prevents negative transfer. Extensive experiments on four fashion tasks show that our FAME-ViL can save 61.5% of parameters over alternatives, while significantly outperforming the conven- tional independently trained single-task models. Code is available at https://github.com/BrandonHanx/FAME-ViL
During the past decade, both multi-label learning and zero-shot learning have attracted huge research attention, and significant progress has been made. Multi-label learning algorithms aim to predict multiple labels given one instance, while most existing zero-shot learning approaches target at predicting a single testing label for each unseen class via transferring knowledge from auxiliary seen classes to target unseen classes. However, relatively less effort has been made on predicting multiple labels in the zero-shot setting, which is nevertheless a quite challenging task. In this work, we investigate and formalize a flexible framework consisting of two components, i.e., visual-semantic embedding and zero-shot multi-label prediction. First, we present a deep regression model to project the visual features into the semantic space, which explicitly exploits the correlations in the intermediate semantic layer of word vectors and makes label prediction possible. Then, we formulate the label prediction problem as a pairwise one and employ Ranking SVM to seek the unique multi-label correlations in the embedding space. Furthermore, we provide a transductive multi-label zero-shot prediction approach that exploits the testing data manifold structure. We demonstrate the effectiveness of the proposed approach on three popular multi-label datasets with state-of-the-art performance obtained on both conventional and generalized ZSL settings.
Zero-shot learning (ZSL) is made possible by learning a projection function between a feature space and a semantic space (e.g., an attribute space). Key to ZSL is thus to learn a projection that is robust against the often large domain gap between the seen and unseen class domains. In this work, this is achieved by unseen class data synthesis and robust projection function learning. Specifically, a novel semantic data synthesis strategy is proposed, by which semantic class prototypes (e.g., attribute vectors) are used to simply perturb seen class data for generating unseen class ones. As in any data synthesis/hallucination approach, there are ambiguities and uncertainties on how well the synthesised data can capture the targeted unseen class data distribution. To cope with this, the second contribution of this work is a novel projection learning model termed competitive bidirectional projection learning (BPL) designed to best utilise the ambiguous synthesised data. As a third contribution, we show that the proposed ZSL model can be easily extended to few-shot learning (FSL) by again exploiting semantic (class prototype guided) feature synthesis and competitive BPL. Extensive experiments show that our model achieves the state-of-the-art results on both problems.
As an instance-level recognition problem, person re-identification (ReID) relies on discriminative features, which not only capture different spatial scales but also encapsulate an arbitrary combination of multiple scales. We callse features of both homogeneous and heterogeneous scales omni-scale features. In this paper, a novel deep ReID CNN is designed, termed Omni-Scale Network (OSNet), for omni-scale feature learning. This is achieved by designing a residual block composed of multiple convolutional feature streams, each detecting features at a certain scale. Importantly, a novel unified aggregation gate is introduced to dynamically fuse multi-scale features with input-dependent channel-wise weights. To efficiently learn spatial-channel correlations and avoid overfitting, the building block uses both pointwise and depthwise convolutions. By stacking such blocks layer-by-layer, our OSNet is extremely lightweight and can be trained from scratch on existing ReID benchmarks. Despite its small model size, our OSNet achieves state-of-the-art performance on six person-ReID datasets. Code and models are available at: https://github.com/KaiyangZhou/deep-person-reid.
In recent years, visual question answering (VQA) has become topical. The premise of VQA's significance as a benchmark in AI, is that both the image and textual question need to be well understood and mutually grounded in order to infer the correct answer. However, current VQA models perhaps `understand' less than initially hoped, and instead master the easier task of exploiting cues given away in the question and biases in the answer distribution [1] . In this paper we propose the inverse problem of VQA (iVQA). The iVQA task is to generate a question that corresponds to a given image and answer pair. We propose a variational iVQA model that can generate diverse, grammatically correct and content correlated questions that match the given answer. Based on this model, we show that iVQA is an interesting benchmark for visuo-linguistic understanding, and a more challenging alternative to VQA because an iVQA model needs to understand the image better to be successful. As a second contribution, we show how to use iVQA in a novel reinforcement learning framework to diagnose any existing VQA model by way of exposing its belief set: the set of question-answer pairs that the VQA model would predict true for a given image. This provides a completely new window into what VQA models `believe' about images. We show that existing VQA models have more erroneous beliefs than previously thought, revealing their intrinsic weaknesses. Suggestions are then made on how to address these weaknesses going forward.
Zero-shot learning (ZSL) aims to transfer knowledge from seen classes to unseen ones so that the latter can be recognised without any training samples. This is made possible by learning a projection function between a feature space and a semantic space (e.g. attribute space). Considering the seen and unseen classes as two domains, a big domain gap often exists which challenges ZSL. In this work, we propose a novel inductive ZSL model that leverages superclasses as the bridge between seen and unseen classes to narrow the domain gap. Specifically, we first build a class hierarchy of multiple superclass layers and a single class layer, where the superclasses are automatically generated by data-driven clustering over the semantic representations of all seen and unseen class names. We then exploit the superclasses from the class hierarchy to tackle the domain gap challenge in two aspects: deep feature learning and projection function learning. First, to narrow the domain gap in the feature space, we define a recurrent neural network over superclasses and then plug it into a convolutional neural network for enforcing the superclass hierarchy. Second, to further learn a transferrable projection function for ZSL, a novel projection function learning method is proposed by exploiting the superclasses to align the two domains. Importantly, our transferrable feature and projection learning methods can be easily extended to a closely related task—few-shot learning (FSL). Extensive experiments show that the proposed model outperforms the state-of-the-art alternatives in both ZSL and FSL tasks.
Image annotation aims to annotate a given image with a variable number of class labels corresponding to diverse visual concepts. In this paper, we address two main issues in large-scale image annotation: 1) how to learn a rich feature representation suitable for predicting a diverse set of visual concepts ranging from object, scene to abstract concept and 2) how to annotate an image with the optimal number of class labels. To address the first issue, we propose a novel multi-scale deep model for extracting rich and discriminative features capable of representing a wide range of visual concepts. Specifically, a novel two-branch deep neural network architecture is proposed, which comprises a very deep main network branch and a companion feature fusion network branch designed for fusing the multi-scale features computed from the main branch. The deep model is also made multi-modal by taking noisy user-provided tags as model input to complement the image input. For tackling the second issue, we introduce a label quantity prediction auxiliary task to the main label prediction task to explicitly estimate the optimal label number for a given image. Extensive experiments are carried out on two large-scale image annotation benchmark datasets, and the results show that our method significantly outperforms the state of the art.
Modelling long-range contextual relationships is critical for pixel-wise prediction tasks such as semantic segmentation. However, convolutional neural networks (CNNs) are inherently limited to model such dependencies due to the naive structure in its building modules (e.g., local convolution kernel). While recent global aggregation methods are beneficial for long-range structure information modelling, they would oversmooth and bring noise to the regions contain fine details (e.g., boundaries and small objects), which are very much cared in the semantic segmentation task. To alleviate this problem, we propose to explore the local context for making the aggregated long-range relationship being distributed more accurately in local regions. In particular, we design a novel local distribution module which models the affinity map between global and local relationship for each pixel adaptively. Integrating existing global aggregation modules, we show that our approach can be modularized as an end-to-end trainable block and easily plugged into existing semantic segmentation networks, giving rise to the GALD networks. Despite its simplicity and versatility, our approach allows us to build new state of the art on major semantic segmentation benchmarks including Cityscapes, ADE20K, Pascal Context, Camvid and COCO-stuff. Code and trained models are released at https://github.com/lxtGH/GALD-DGCNet to foster further research.
Growing free online 3D shapes collections dictated research on 3D retrieval. Active debate has however been had on (i) what the best input modality is to trigger retrieval, and (ii) the ultimate usage scenario for such retrieval. In this paper, we offer a different perspective towards answering these questions - we study the use of 3D sketches as an input modality and advocate a VR-scenario where retrieval is conducted. Thus, the ultimate vision is that users can freely retrieve a 3D model by air-doodling in a VR environment. As a first stab at this new 3D VR-sketch to 3D shape retrieval problem, we make four contributions. First, we code a VR utility to collect 3D VR-sketches and conduct retrieval. Second, we collect the first set of 167 3D VRsketches on two shape categories from ModelNet. Third, we propose a novel approach to generate a synthetic dataset of human-like 3D sketches of different abstract levels to train deep networks. At last, we compare the common multi-view and volumetric approaches: We show that, in contrast to 3D shape to 3D shape retrieval, volumetric point-based approaches exhibit superior performance on 3D sketch to 3D shape retrieval due to the sparse and abstract nature of 3D VR-sketches. We believe these contributions will collectively serve as enablers for future attempts at this problem. The VR interface, code and datasets are available at https://tinyurl.com/3DSketch3DV.
Most recent semantic segmentation methods adopt a fully-convolutional network (FCN) with an encoder-decoder architecture. The encoder progressively reduces the spatial resolution and learns more abstract/semantic visual concepts with larger receptive fields. Since context modeling is critical for segmentation, the latest efforts have been focused on increasing the receptive field, through either dilated/atrous convolutions or inserting attention modules. However, the encoder-decoder based FCN architecture remains unchanged. In this paper, we aim to provide an alternative perspective by treating semantic segmentation as a sequence-to-sequence prediction task. Specifically, we deploy a pure transformer (i.e., without convolution and resolution reduction) to encode an image as a sequence of patches. With the global context modeled in every layer of the transformer, this encoder can be combined with a simple decoder to provide a powerful segmentation model, termed SEgmentation TRansformer (SETR). Extensive experiments show that SETR achieves new state of the art on ADE20K (50.28% mIoU), Pascal Context (55.83% mIoU) and competitive results on Cityscapes. Particularly, we achieve the first position in the highly competitive ADE20K test server leaderboard on the day of submission.
Contemporary deep learning techniques have made image recognition a reasonably reliable technology. However training effective photo classifiers typically takes numerous examples which limits image recognition's scalability and applicability to scenarios where images may not be available. This has motivated investigation into zero-shot learning, which addresses the issue via knowledge transfer from other modalities such as text. In this paper we investigate an alternative approach of synthesizing image classifiers: Almost directly from a user's imagination, via freehand sketch. This approach doesn't require the category to be nameable or describable via attributes as per zero-shot learning. We achieve this via training a model regression network to map from free-hand sketch space to the space of photo classifiers. It turns out that this mapping can be learned in a category-agnostic way, allowing photo classifiers for new categories to be synthesized by user with no need for annotated training photos. We also demonstrate that this modality of classifier generation can also be used to enhance the granularity of an existing photo classifier, or as a complement to name-based zero-shot learning.
We present the first fine-grained dataset of 1,497 3D VR sketch and 3D shape pairs of a chair category with large shapes diversity. Our dataset supports the recent trend in the sketch community on fine-grained data analysis, and extends it to an actively developing 3D domain. We argue for the most convenient sketching scenario where the sketch consists of sparse lines and does not require any sketching skills, prior training or time-consuming accurate drawing. We then, for the first time, study the scenario of fine-grained 3D VR sketch to 3D shape retrieval, as a novel VR sketching application and a proving ground to drive out generic insights to inform future research. By experimenting with carefully selected combinations of design factors on this new problem, we draw important conclusions to help follow-on work. We hope our dataset will enable other novel applications, especially those that require a fine-grained angle such as fine-grained 3D shape reconstruction. The dataset is available at tinyurl.com/ VRSketch3DV21.
Fine-grained sketch-based image retrieval (FG-SBIR) addresses matching specific photo instance using free-hand sketch as a query modality. Existing models aim to learn an embedding space in which sketch and photo can be directly compared. While successful, they require instance-level pairing within each coarse-grained category as annotated training data. Since the learned embedding space is domain-specific, these models do not generalise well across categories. This limits the practical applicability of FGSBIR. In this paper, we identify cross-category generalisation for FG-SBIR as a domain generalisation problem, and propose the first solution. Our key contribution is a novel unsupervised learning approach to model a universal manifold of prototypical visual sketch traits. This manifold can then be used to paramaterise the learning of a sketch/photo representation. Model adaptation to novel categories then becomes automatic via embedding the novel sketch in the manifold and updating the representation and retrieval function accordingly. Experiments on the two largest FG-SBIR datasets, Sketchy and QMUL-Shoe-V2, demonstrate the efficacy of our approach in enabling crosscategory generalisation of FG-SBIR.
We investigate the problem of fine-grained sketch-based image retrieval (SBIR), where free-hand human sketches are used as queries to perform instance-level retrieval of images. This is an extremely challenging task because (i) visual comparisons not only need to be fine-grained but also executed cross-domain, (ii) free-hand (finger) sketches are highly abstract, making fine-grained matching harder, and most importantly (iii) annotated cross-domain sketch-photo datasets required for training are scarce, challenging many state-of-the-art machine learning techniques. In this paper, for the first time, we address all these challenges, providing a step towards the capabilities that would underpin a commercial sketch-based image retrieval application. We introduce a new database of 1,432 sketchphoto pairs from two categories with 32,000 fine-grained triplet ranking annotations. We then develop a deep tripletranking model for instance-level SBIR with a novel data augmentation and staged pre-training strategy to alleviate the issue of insufficient fine-grained training data. Extensive experiments are carried out to contribute a variety of insights into the challenges of data sufficiency and over-fitting avoidance when training deep networks for finegrained cross-domain ranking tasks.
When humans describe images they tend to use combinations of nouns and adjectives, corresponding to objects and their associated attributes respectively. To generate such a description automatically, one needs to model objects, attributes and their associations. Conventional methods require strong annotation of object and attribute locations, making them less scalable. In this paper, we model object-attribute associations from weakly labelled images, such as those widely available on media sharing sites (e.g. Flickr), where only image-level labels (either object or attributes) are given, without their locations and associations. This is achieved by introducing a novel weakly supervised non-parametric Bayesian model. Once learned, given a new image, our model can describe the image, including objects, attributes and their associations, as well as their locations and segmentation. Extensive experiments on benchmark datasets demonstrate that our weakly supervised model performs at par with strongly supervised models on tasks such as image description and retrieval based on object-attribute associations. © 2014 Springer International Publishing.
Human free-hand sketches have been studied in various contexts including sketch recognition, synthesis and fine-grained sketch-based image retrieval (FG-SBIR). A fundamental challenge for sketch analysis is to deal with drastically different human drawing styles, particularly in terms of abstraction level. In this work, we propose the first stroke-level sketch abstraction model based on the insight of sketch abstraction as a process of trading off between the recognizability of a sketch and the number of strokes used to draw it. Concretely, we train a model for abstract sketch generation through reinforcement learning of a stroke removal policy that learns to predict which strokes can be safely removed without affecting recognizability. We show that our abstraction model can be used for various sketch analysis tasks including: (1) modeling stroke saliency and understanding the decision of sketch recognition models, (2) synthesizing sketches of variable abstraction for a given category, or reference object instance in a photo, and (3) training a FG-SBIR model with photos only, bypassing the expensive photo-sketch pair collection step.
Modelling human free-hand sketches has become topical recently, driven by practical applications such as fine-grained sketch based image retrieval (FG-SBIR). Sketches are clearly related to photo edge-maps, but a human free-hand sketch of a photo is not simply a clean rendering of that photo’s edge map. Instead there is a fundamental process of abstraction and iconic rendering, where overall geometry is warped and salient details are selectively included. In this paper we study this sketching process and attempt to invert it. We model this inversion by translating iconic free-hand sketches to contours that resemble more geometrically realistic projections of object boundaries, and separately factorise out the salient added details. This factorised re-representation makes it easier to match a free-hand sketch to a photo instance of an object. Specifically, we propose a novel unsupervised image style transfer model based on enforcing a cyclic embedding consistency constraint. A deep FG-SBIR model is then formulated to accommodate complementary discriminative detail from each factorised sketch for better matching with the corresponding photo. Our method is evaluated both qualitatively and quantitatively to demonstrate its superiority over a number of state-of-the-art alternatives for style transfer and FG-SBIR.
Categorizing free-hand human sketches has profound implications in applications such as human computer interaction and image retrieval. The task is non-trivial due to the iconic nature of sketches, signified by large variances in both appearance and structure when compared with photographs. Prior works often utilize off-the-shelf low-level features and assume the availability of a large training set, rendering them sensitive towards abstraction and less scalable to new categories. To overcome this limitation, we propose a transfer learning framework which enables one-shot learning of sketch categories. The framework is based on a novel co-regularized sparse coding model which exploits common/ shareable parts among human sketches of seen categories and transfer them to unseen categories. We contribute a new dataset consisting of 7,760 human segmented sketches from 97 object categories. Extensive experiments reveal that the proposed method can classify unseen sketch categories given just one training sample with a 33.04% accuracy, offering a two-fold improvement over baselines.
Existing object detection methods typically rely on the availability of abundant labelled training samples per class and offline model training in a batch mode. These requirements substantially limit their scalability to open-ended accommodation of novel classes with limited labelled training data, both in terms of model accuracy and training efficiency during deployment. We present the first study aiming to go beyond these limitations by considering the Incremental Few-Shot Detection (iFSD) problem setting, where new classes must be registered incrementally (without revisiting base classes) and with few examples. To this end we propose OpeN-ended Centre nEt (ONCE), a detector designed for incrementally learning to detect novel class objects with few examples. This is achieved by an elegant adaptation of the efficient CentreNet detector to the few-shot learning scenario, and meta-learning a class-wise code generator model for registering novel classes. ONCE fully respects the incremental learning paradigm, with novel class registration requiring only a single forward pass of few-shot training samples, and no access to base classes - thus making it suitable for deployment on embedded devices, etc. Extensive experiments conducted on both the standard object detection (COCO, PASCAL VOC) and fashion landmark detection (DeepFashion2) tasks show the feasibility of iFSD for the first time, opening an interesting and very important line of research.
We contribute the first large-scale dataset of scene sketches, SketchyScene, with the goal of advancing research on sketch understanding at both the object and scene level. The dataset is created through a novel and carefully designed crowdsourcing pipeline, enabling users to efficiently generate large quantities of realistic and diverse scene sketches. SketchyScene contains more than 29,000 scene-level sketches, 7,000+ pairs of scene templates and photos, and 11,000+ object sketches. All objects in the scene sketches have ground-truth semantic and instance masks. The dataset is also highly scalable and extensible, easily allowing augmenting and/or changing scene composition. We demonstrate the potential impact of SketchyScene by training new computational models for semantic segmentation of scene sketches and showing how the new dataset enables several applications including image retrieval, sketch colorization, editing, and captioning, etc. The dataset and code can be found at https://github.com/SketchyScene/SketchyScene.
Recently, large-scale few-shot learning (FSL) becomes topical. It is discovered that, for a large-scale FSL problem with 1,000 classes in the source domain, a strong baseline emerges, that is, simply training a deep feature embedding model using the aggregated source classes and performing nearest neighbor (NN) search using the learned features on the target classes. The state-of-the-art large-scale FSL methods struggle to beat this baseline, indicating intrinsic limitations on scalability. To overcome the challenge, we propose a novel large-scale FSL model by learning transferable visual features with the class hierarchy which encodes the semantic relations between source and target classes. Extensive experiments show that the proposed model significantly outperforms not only the NN baseline but also the state-of-the-art alternatives. Furthermore, we show that the proposed model can be easily extended to the large-scale zero-shot learning (ZSL) problem and also achieves the state-of-the-art results.
Sketch is used for rendering the visual world since prehistoric times, and has become ubiquitous nowadays with the increasing availability of touchscreens on portable devices. However, how to automatically map images to sketches, a problem that has profound implications on applications such as sketch-based image retrieval, still remains open. In this paper, we propose a novel method that draws a sketch automatically from a single natural image. Sketch extraction is posed within an unified contour grouping framework, where perceptual grouping is first used to form contour segment groups, followed by a group-based contour simplification method that generate the final sketches. In our experiment, for the first time we pose sketch evaluation as a sketch-based object recognition problem and the results validate the effectiveness of our system over the state-of-the-arts alternatives.
In this work we aim to develop a universal sketch grouper. That is, a grouper that can be applied to sketches of any category in any domain to group constituent strokes/segments into semantically meaningful object parts. The first obstacle to this goal is the lack of large-scale datasets with grouping annotation. To overcome this, we contribute the largest sketch perceptual grouping (SPG) dataset to date, consisting of 20, 000 unique sketches evenly distributed over 25 object categories. Furthermore, we propose a novel deep universal perceptual grouping model. The model is learned with both generative and discriminative losses. The generative losses improve the generalisation ability of the model to unseen object categories and datasets. The discriminative losses include a local grouping loss and a novel global grouping loss to enforce global grouping consistency. We show that the proposed model significantly outperforms the state-of-the-art groupers. Further, we show that our grouper is useful for a number of sketch analysis tasks including sketch synthesis and fine-grained sketch-based image retrieval (FG-SBIR). © Springer Nature Switzerland AG 2018.
We aim to learn a domain generalizable person reidentification (ReID) model. When such a model is trained on a set of source domains (ReID datasets collected from different camera networks), it can be directly applied to any new unseen dataset for effective ReID without any model updating. Despite its practical value in real-world deployments, generalizable ReID has seldom been studied. In this work, a novel deep ReID model termed Domain-Invariant Mapping Network(DIMN) is proposed. DIMN is designed to learn a mapping between a person image and its identity classifier, i.e., it produces a classifier using a single shot. To make the model domain-invariant, we follow a meta-learning pipeline and sample a subset of source domain training tasks during each training episode. However, the model is significantly different from conventional meta-learning methods in that: (1) no model updating is required for the target domain, (2) different training tasks share a memory bank for maintaining both scalability and discrimination ability, and (3) it can be used to match an arbitrary number of identities in a target domain. Extensive experiments on a newly proposed large-scale ReID domain generalization benchmark show that our DIMN significantly outperforms alternative domain generalization or meta-learning methods.
To see is to sketch - free-hand sketching naturally builds ties between human and machine vision. In this paper, we present a novel approach for translating an object photo to a sketch, mimicking the human sketching process. This is an extremely challenging task because the photo and sketch domains differ significantly. Furthermore, human sketches exhibit various levels of sophistication and abstraction even when depicting the same object instance in a reference photo. This means that even if photo-sketch pairs are available, they only provide weak supervision signal to learn a translation model. Compared with existing supervised approaches that solve the problem of D(E(photo)) → sketch), where E(·) and D(·) denote encoder and decoder respectively, we take advantage of the inverse problem (e.g., D(E(sketch) → photo), and combine with the unsupervised learning tasks of within-domain reconstruction, all within a multi-task learning framework. Compared with existing unsupervised approaches based on cycle consistency (i.e., D(E(D(E(photo)))) → photo), we introduce a shortcut consistency enforced at the encoder bottleneck (e.g., D(E(photo)) → photo) to exploit the additional self-supervision. Both qualitative and quantitative results show that the proposed model is superior to a number of state-of-the-art alternatives. We also show that the synthetic sketches can be used to train a better fine-grained sketch-based image retrieval (FG-SBIR) model, effectively alleviating the problem of sketch data scarcity.
Human sketches are unique in being able to capture both the spatial topology of a visual object, as well as its subtle appearance details. Fine-grained sketch-based image retrieval (FG-SBIR) importantly leverages on such fine-grained characteristics of sketches to conduct instance-level retrieval of photos. Nevertheless, human sketches are often highly abstract and iconic, resulting in severe misalignments with candidate photos which in turn make subtle visual detail matching difficult. Existing FG-SBIR approaches focus only on coarse holistic matching via deep cross-domain representation learning, yet ignore explicitly accounting for fine-grained details and their spatial context. In this paper, a novel deep FG-SBIR model is proposed which differs significantly from the existing models in that: (1) It is spatially aware, achieved by introducing an attention module that is sensitive to the spatial position of visual details: (2) It combines coarse and fine semantic information via a shortcut connection fusion block: and (3) It models feature correlation and is robust to misalignments between the extracted features across the two domains by introducing a novel higher-order learnable energy function (HOLEF) based loss. Extensive experiments show that the proposed deep spatial-semantic attention model significantly outperforms the state-of-the-art.
Zero-shot learning has received increasing interest as a means to alleviate the often prohibitive expense of annotating training data for large scale recognition problems. These methods have achieved great success via learning intermediate semantic representations in the form of attributes and more recently, semantic word vectors. However, they have thus far been constrained to the single-label case, in contrast to the growing popularity and importance of more realistic multi-label data. In this paper, for the first time, we investigate and formalise a general framework for multi-label zero-shot learning, addressing the unique challenge therein: how to exploit multi-label correlation at test time with no training data for those classes? In particular, we propose (1) a multi-output deep regression model to project an image into a semantic word space, which explicitly exploits the correlations in the intermediate semantic layer of word vectors; (2) a novel zero-shot learning algorithm for multi-label data that exploits the unique compositionality property of semantic word vector representations; and (3) a transductive learning strategy to enable the regression model learned from seen classes to generalise well to unseen classes. Our zero-shot learning experiments on a number of standard multi-label datasets demonstrate that our method outperforms a variety of baselines. © 2014. The copyright of this document resides with its authors.
Gestalt principles, a set of conjoining rules derived from human visual studies, have been known to play an important role in computer vision. Many applications such as image segmentation, contour grouping and scene understanding often rely on such rules to work. However, the problem of Gestalt confliction, i.e., the relative importance of each rule compared with another, remains unsolved. In this paper, we investigate the problem of perceptual grouping by quantifying the confliction among three commonly used rules: similarity, continuity and proximity. More specifically, we propose to quantify the importance of Gestalt rules by solving a learning to rank problem, and formulate a multi-label graph-cuts algorithm to group image primitives while taking into account the learned Gestalt confliction. Our experiment results confirm the existence of Gestalt confliction in perceptual grouping and demonstrate an improved performance when such a confliction is accounted for via the proposed grouping algorithm. Finally, a novel cross domain image classification method is proposed by exploiting perceptual grouping as representation.
We aim to learn deep person re-identification (ReID) models that are robust against noisy training data. Two types of noise are prevalent in practice: (1) label noise caused by human annotator errors and (2) data outliers caused by person detector errors or occlusion. Both types of noise pose serious problems for training ReID models, yet have been largely ignored so far. In this paper, we propose a novel deep network termed DistributionNet for robust ReID. Instead of representing each person image as a feature vector, DistributionNet models it as a Gaussian distribution with its variance representing the uncertainty of the extracted features. A carefully designed loss is formulated in DistributionNet to unevenly allocate uncertainty across training samples. Consequently, noisy samples are assigned large variance/uncertainty, which effectively alleviates their negative impacts on model fitting. Extensive experiments demonstrate that our model is more effective than alternative noise-robust deep models. The source code is available at: https://github.com/TianyuanYu/DistributionNet.
Automatic data abstraction is an important capability for both benchmarking machine intelligence and supporting summarization applications. In the former one asks whether a machine can `understand' enough about the meaning of input data to produce a meaningful but more compact abstraction. In the latter this capability is exploited for saving space or human time by summarizing the essence of input data. In this paper we study a general reinforcement learning based framework for learning to abstract sequential data in a goal-driven way. The ability to define different abstraction goals uniquely allows different aspects of the input data to be preserved according to the ultimate purpose of the abstraction. Our reinforcement learning objective does not require human-defined examples of ideal abstraction. Importantly our model processes the input sequence holistically without being constrained by the original input order. Our framework is also domain agnostic -- we demonstrate applications to sketch, video and text data and achieve promising results in all domains.
In this paper, we propose to tackle the challenging few-shot learning (FSL) problem by learning global class representations using both base and novel class training samples. In each training episode, an episodic class mean computed from a support set is registered with the global representation via a registration module. This produces a registered global class representation for computing the classification loss using a query set. Though following a similar episodic training pipeline as existing meta learning based approaches, our method differs significantly in that novel class training samples are involved in the training from the beginning. To compensate for the lack of novel class training samples, an effective sample synthesis strategy is developed to avoid overfitting. Importantly, by joint base-novel class training, our approach can be easily extended to a more practical yet challenging FSL setting, i.e., generalized FSL, where the label space of test data is extended to both base and novel classes. Extensive experiments show that our approach is effective for both of the two FSL settings.
Fine-grained sketch-based image retrieval (SBIR) aims to go beyond conventional SBIR to perform instance-level cross-domain retrieval: finding the specific photo that matches an input sketch. Existing methods focus on designing/learning good features for cross-domain matching and/or learning cross-domain matching functions. However, they neglect the semantic aspect of retrieval, i.e., what meaningful object properties does a user try encode in her/his sketch? We propose a fine-grained SBIR model that exploits semantic attributes and deep feature learning in a complementary way. Specifically, we perform multi-task deep learning with three objectives, including: retrieval by fine-grained ranking on a learned representation, attribute prediction, and attribute-level ranking. Simultaneously predicting semantic attributes and using such predictions in the ranking procedure help retrieval results to be more semantically relevant. Importantly, the introduction of semantic attribute learning in the model allows for the elimination of the otherwise prohibitive cost of human annotations required for training a fine-grained deep ranking model. Experimental results demonstrate that our method outperforms the state-of-the-art on challenging fine-grained SBIR benchmarks while requiring less annotation.
ImageNet pre-training has long been considered crucial by the fine-grained sketch-based image retrieval (FG-SBIR) community due to the lack of large sketch-photo paired datasets for FG-SBIR training. In this paper, we propose a self-supervised alternative for representation pre-training. Specifically, we consider the jigsaw puzzle game of recomposing images from shuffled parts. We identify two key facets of jigsaw task design that are required for effective FG-SBIR pre-training. The first is formulating the puzzle in a mixed-modality fashion. Second we show that framing the optimisation as permutation matrix inference via Sinkhorn iterations is more effective than the common classifier formulation of Jigsaw self-supervision. Experiments show that this self-supervised pre-training strategy significantly outperforms the standard ImageNet-based pipeline across all four product-level FG-SBIR benchmarks. Interestingly it also leads to improved cross-category generalisation across both pre-train/fine-tune and fine-tune/testing stages.
Automated deception detection (ADD) from real-life videos is a challenging task. It specifically needs to address two problems: (1) Both face and body contain useful cues regarding whether a subject is deceptive. How to effectively fuse the two is thus key to the effectiveness of an ADD model. (2) Real-life deceptive samples are hard to collect; learning with limited training data thus challenges most deep learning based ADD models. In this work, both problems are addressed. Specifically, for face-body multimodal learning, a novel face-focused cross-stream network (FFCSN) is proposed. It differs significantly from the popular two-stream networks in that: (a) face detection is added into the spatial stream to capture the facial expressions explicitly, and (b) correlation learning is performed across the spatial and temporal streams for joint deep feature learning across both face and body. To address the training data scarcity problem, our FFCSN model is trained with both meta learning and adversarial learning. Extensive experiments show that our FFCSN model achieves state-of-the-art results. Further, the proposed FFCSN model as well as its robust training strategy are shown to be generally applicable to other human-centric video analysis tasks such as emotion recognition from user-generated videos.
We propose a perceptual grouping framework that organizes image edges into meaningful structures and demonstrate its usefulness on various computer vision tasks. Our grouper formulates edge grouping as a graph partition problem, where a learning to rank method is developed to encode probabilities of candidate edge pairs. In particular, RankSVM is employed for the first time to combine multiple Gestalt principles as cue for edge grouping. Afterwards, an edge grouping based object proposal measure is introduced that yields proposals comparable to state-of-the-art alternatives. We further show how human-like sketches can be generated from edge groupings and consequently used to deliver state-of-the-art sketch-based image retrieval performance. Last but not least, we tackle the problem of freehand human sketch segmentation by utilizing the proposed grouper to cluster strokes into semantic object parts.
We propose a deep hashing framework for sketch retrieval that, for the first time, works on a multi-million scale human sketch dataset. Leveraging on this large dataset, we explore a few sketch-specific traits that were otherwise under-studied in prior literature. Instead of following the conventional sketch recognition task, we introduce the novel problem of sketch hashing retrieval which is not only more challenging, but also offers a better testbed for large-scale sketch analysis, since: (i) more fine-grained sketch feature learning is required to accommodate the large variations in style and Abstraction, and (ii) a compact binary code needs to be learned at the same time to enable efficient retrieval. Key to our network design is the embedding of unique characteristics of human sketch, where (i) a two-branch CNN-RNN architecture is adapted to explore the temporal ordering of strokes, and (ii) a novel hashing loss is specifically designed to accommodate both the temporal and Abstract traits of sketches. By working with a 3.8M sketch dataset, we show that state-of-the-art hashing models specifically engineered for static images fail to perform well on temporal sketch data. Our network on the other hand not only offers the best retrieval performance on various code sizes, but also yields the best generalization performance under a zero-shot setting and when re-purposed for sketch recognition. Such superior performances effectively demonstrate the benefit of our sketch-specific design. © 2018 IEEE.
Analysis of human sketches in deep learning has advanced immensely through the use of waypoint-sequences rather than raster-graphic representations. We further aim to model sketches as a sequence of low-dimensional parametric curves. To this end, we propose an inverse graphics framework capable of approximating a raster or waypoint based stroke encoded as a point-cloud with a variable-degree Bezier curve. Building on this module, ´we present Cloud2Curve, a generative model for scalable high-resolution vector sketches that can be trained end-to-end using point-cloud data alone. As a consequence, our model is also capable of deterministic vectorization which can map novel raster or waypoint based sketches to their corresponding high-resolution scalable Bezier equivalent. ´We evaluate the generation and vectorization capabilities of our model on Quick, Draw! and K-MNIST datasets. The analysis of free-hand sketches using deep learning [40] has flourished over the past few years, with sketches now being well analysed from classification [43, 42] and retrieval [27, 12, 4] perspectives. Sketches for digital analysis have always been acquired in two primary modalities - raster (pixel grids) and vector (line segments). Raster sketches have mostly been the modality of choice for sketch recognition and retrieval [43, 27]. However, generative sketch models began to advance rapidly [16] after focusing on vector representations and generating sketches as sequences [7, 37] of waypoints/line segments, similarly to how humans sketch. As a happy byproduct, this paradigm leads to clean and blur-free image generation as opposed to direct raster-graphic generations [30]. Recent works have studied creativity in sketch generation [16], learning to sketch raster photo input images [36], learning efficient