Artificial Intelligence to enable omics integration - delivering enhanced data utilisation for biomarker discovery
The field of biomarker discovery is steadily moving towards the greater utilisation of large-scale ‘omic approaches. This PhD will develop novel methods in computational and mathematical sciences, using advanced machine-learning approaches applied to biomedical data, for multi-‘omic data integration. This will allow for the concurrent and truly integrated analysis of multiple ‘omic types, to establish combined biomarker signatures/algorithms with greater value, optimising disease prediction, diagnosis and intervention across a wide range of different conditions.
Start date
1 October 2022Duration
3.5 yearsApplication deadline
Funding source
EPSRC Doctoral Training PartnershipFunding information
Enhanced EPSRC stipend (£19,062 per annum for 2022-23, with annual increments based on inflation) and fees covered. Funding also includes a research training and support grant of £3,000 for the duration of the studentship.
About
This is a great opportunity for an enthusiastic student to be trained in the cutting-edge disciplines of artificial intelligence (AI) and Machine Learning (ML), intersected with medicine and biology, as well as biomarker technologies for characterisation of human disease and wellness. Such a training offers great advantage for a career in research or industry. Specifically, in this studentship we focus on the increasing need in medicine for molecular indicators (biomarkers) in prostate cancer. These can be identified in blood or other body fluids, and enable more precise patient diagnosis as well as prediction of disease progression and response to treatments. New biomarkers can help make better predictions for patients, improving how they are cared for and improving the health economy.
We have reached a point where we can generate huge amounts of data on the types of molecules found in blood or urine, using advanced ‘omics techniques that measure hundreds or thousands of different molecules such as proteins, lipids, or metabolites. While individual analyses that use genomic or other ‘omic data have shown some predictive abilities, there is growing evidence that multi-‘omic models, where biomarkers are identified across multiple ‘omic platforms, can enhance predictions for patients. However, combining these very complex data is not straightforward. In this PhD, we will directly address that issue.
AI gives us the ability to combine complex data from different sources, assessing molecular signatures that give us the ability to make diagnoses or determine who will respond well or badly to a drug. Using the huge amounts of data already available to us, we will develop and assess novel machine-learning approaches that can improve biomarker discovery through making better use of all the data collected from patients.
The University of Surrey and our collaborative partners provide a vibrant, interdisciplinary research environment, with access to state-of-the-art facilities. We see our postgraduate researchers as an integral part of our research community, collaborating and innovating together with academics at all levels. We want the most talented researchers from diverse backgrounds to join us, bringing new ideas and perspectives. We will help you make the most of your potential, removing barriers where we can and supporting you with dedicated career guidance. We offer generous funding packages, sector-leading researcher development training and mentoring, and dedicated employability support.
Whatever your aspirations, Surrey is where research careers are launched and nurtured.
References
- Muazzam, D. Chiasserini, J. Kelsall, N. Geifman, A. D. Whetton, & P. A. Townsend; A Prostate Cancer Proteomics Database for SWATH-MS based Protein Quantification. Cancers (2021)
- D. Whetton, G. Preston, S. Abubeker, & N. Geifman; Proteomics and informatics for understanding phases and identifying biomarkers in COVID-19 disease. Journal of Proteome Research (2020)
- A. Dagliati, N. Geifman, N. Peek, J. Holmes. L. Sacchi, R. Bellazzi, S. E. Sajjadi, & A.Tucker. Using Topological Data Analysis and Pseudo Time Series to Infer Temporal Phenotypes from Electronic Health Records. Artificial Intelligence in Medicine (2020)
- F. Vitali, G. Nicora, A. Dagliati, N. Geifman & R. Bellazzi; Integrated multi-omics analyses in oncology: a review of machine learning methods and tools. Frontiers in Oncology (2020)
- K. A. McGurk, A. Dagliati, D. Chiasserini, D. Lee, D. Plant, I. Baricevic-Jones, J. Kelsall, R. Eineman, R. Reed, B. Geary, R. D. Unwin, A. Nicolaou, B. D. Keavney, A. Barton, A. D. Whetton, & N. Geifman; The use of missing values in proteomic data-independent acquisition mass spectrometry to enable disease activity discrimination. Bioinformatics (2019)
Eligibility criteria
Applicants are expected to have a good honours degree (first or upper second) or a masters degree in an appropriate discipline; this includes, but not limited, to biological or health sciences, or informatics and computer sciences. Good quantitative skills are required; experience in coding would be considered favourably.
English Language requirements
IELTS Academic: 6.5 or above (or equivalent) with 6 in each individual category.
How to apply
Applications should be submitted via the Biosciences and Medicine PhD programme page on the "Apply" tab (select October 2022 start date). Please clearly state the studentship title and supervisor on your application. Once you have completed and submitted your application, please send an email to the primary supervisors confirming you have applied.
Studentship FAQs
Read our studentship FAQs to find out more about applying and funding.
Application deadline
Contact details
Nophar Geifman
Research
Health and Biomedical Informatics / Digital Health
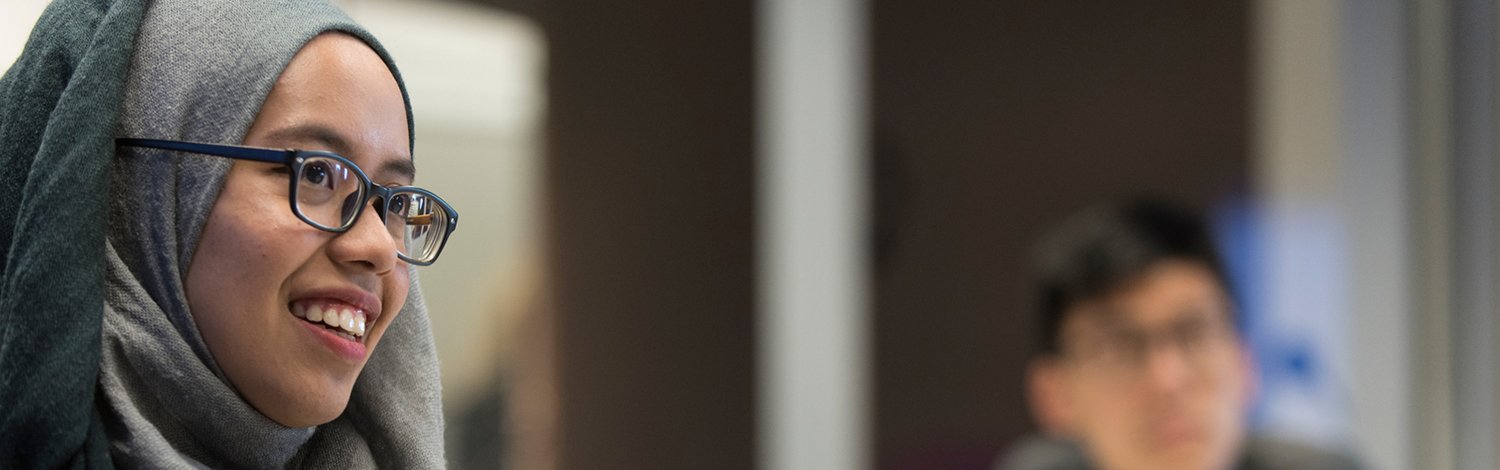
Studentships at Surrey
We have a wide range of studentship opportunities available.