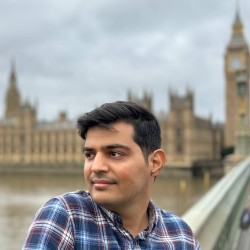
Amir Khosravian
Academic and research departments
Centre for Automotive Engineering, School of Mechanical Engineering Sciences, Faculty of Engineering and Physical Sciences.About
My research project
AI-Based Vehicle Dynamics Controllers and Observers for Off-road Autonomous DrivingDespite all the advancements in autonomous vehicles (AVs), their safe navigation in unstructured environments still remains a demanding challenge. In general, the vehicle dynamics are non-linear, and it is not easy to control the motion of the vehicle in off-road environments where road excitations may massively influence the load transfer and tire forces. To cope with the mentioned issue, in this project, novel algorithms to control the dynamics of the AV will be derived. Artificial intelligence (AI) algorithms will be utilized in the research, which by taking advantage of an optimized architecture, consider the non-linearity of the dynamic equations and provide the vehicle with real-time safe vehicle commands to navigate in unstructured environments safely.
Supervisors
Despite all the advancements in autonomous vehicles (AVs), their safe navigation in unstructured environments still remains a demanding challenge. In general, the vehicle dynamics are non-linear, and it is not easy to control the motion of the vehicle in off-road environments where road excitations may massively influence the load transfer and tire forces. To cope with the mentioned issue, in this project, novel algorithms to control the dynamics of the AV will be derived. Artificial intelligence (AI) algorithms will be utilized in the research, which by taking advantage of an optimized architecture, consider the non-linearity of the dynamic equations and provide the vehicle with real-time safe vehicle commands to navigate in unstructured environments safely.
My qualifications
Mechanical Engineering
Mechanical Engineering - Automotive Engineering
Design of Vehicle Dynamic System
ResearchResearch interests
Amir's research interest are heavily focused on autonomous systems such as autonomous vehicles (self-driving-cars) and robotics. He is also motivated to focus on machine learning and AI-based solutions especially in the field of computer vision, deep learning, and machine vision.
Research Activities:
- Computer Vision
- Deep Learning
- Autonomous Vehicles
- Robot and AV Navigation
- Vehicle Dynamics
- Machine Learning
- Optimal Control
- Motion Planning
- Path Planning & Following
Research interests
Amir's research interest are heavily focused on autonomous systems such as autonomous vehicles (self-driving-cars) and robotics. He is also motivated to focus on machine learning and AI-based solutions especially in the field of computer vision, deep learning, and machine vision.
Research Activities:
- Computer Vision
- Deep Learning
- Autonomous Vehicles
- Robot and AV Navigation
- Vehicle Dynamics
- Machine Learning
- Optimal Control
- Motion Planning
- Path Planning & Following
Teaching
Capable of Teaching:
- Deep learning and Computer Vision
- Autonomous Vehicle Simulation
- Path-tracking and Path-following control
- Various Autonomous Systems Simulators
- Advance Python Programming
- MATLAB/Simulink
- Embedded Coding and Optimizing Deep learning Performance Inference during the Implementation
Publications
In scene understanding for autonomous vehicles (AVs), models trained on the available datasets fail to generalize well to the complex, real-world scenarios with higher dynamics. In this work, we attempt to handle the distribution mismatch by employing the generative adversarial network (GAN) and weather modeling to strengthen the intra-domain data. We also alleviate the fragility of our trained models against natural distortions with state-of-the-art augmentation approaches. Finally, we assess our method for cross-domain object detection through CARLA simulation. Our experiments demonstrate that: (1) Augmenting training class with even limited intra-domain data captured from the adverse weather conditions boosts the generalization of the two kinds of object detectors; (2) Exploiting GANs and weather modeling to elaborately simulate the adverse, intra-domain weather conditions manages to surmount the adverse data scarcity issue for intra-domain object detection; (3) A combination of Augmix and style augmentations not only can promote the robustness of our trained models against different natural distortions but also can boost their performance in the cross-domain object detection; (4) Training GANs for unsupervised image-to-image translation by means of the existing, large-scale datasets outside of our training domain is found beneficial to alleviate image-based and instance-based domain shifts.
Recent studies have recently exploited knowledge distillation (KD) technique to address time-consuming annotation task in semantic segmentation, through which one teacher trained on a single dataset could be leveraged for annotating unlabeled data. However, in this context, knowledge capacity is restricted, and knowledge variety is rare in different conditions, such as cross-model KD, in which the single teacher KD prohibits the student model from distilling information using cross-domain context. In this study, we aim to train a robust, lightweight student under the supervision of several expert teachers, which provide better instructive guidance compared to a single student-teacher learning framework. To be more specific, we first train five distinct convolutional neural networks (CNNs) as teachers for semantic segmentation on several datasets. To this end, several state-of-the-art augmentation transformations have also been utilized in training phase of our teachers. The impacts of such training scenarios are then assessed in terms of student robustness and accuracy. As the main contribution of this paper, our proposed multi-teacher KD paradigm endows the student with the ability to amalgamate and capture a variety of knowledge illustrations from different sources. Results demonstrated that our method outperforms the existing studies on both clean and corrupted data in the semantic segmentation task while benefiting from our proposed score weight system. Experiments validate that our multi-teacher framework results in an improvement of 9% up to 32.18% compared to the single-teacher paradigm. Moreover, it is demonstrated that our paradigm surpasses previous supervised real-time studies in the semantic segmentation challenge.
The detection of traffic signs in clean and noise-free images has been investigated by numerous researchers; however, very few of these works have focused on noisy environments. While in the real world, for different reasons (e.g. the speed and acceleration of a vehicle and the roughness around it), the input images of the convolutional neural networks (CNNs) could be extremely noisy. Contrary to other research works, in this paper, we investigate the robustness of the deep learning models against the synthetically modeled noises in the detection of small objects. To this end, the state-of-the-art architectures of Faster-RCNN Resnet101, R-FCN Resnet101, and Faster-RCNN Inception Resnet V2 are trained by means of the Tsinghua-Tencent 100K database, and the performances of the trained models on noisy data are evaluated. After verifying the robustness of these models, different training scenarios (1 – Modeling various climatic conditions, 2 – Style randomization, and 3 – Augmix augmentation) are used to enhance the model robustness. The findings indicate that these scenarios result in up to 13.09%, 12%, and 13.61% gains in the mentioned three networks by means of the mPC metric. They also result in 11.74%, 8.89%, and 7.27% gains in the rPC metric, demonstrating that improvement in robustness does not lead to performance drop on the clean data.
In this paper, a large-scale dataset called the Iran Autonomous Driving Dataset (IADD) is presented, aiming to improve the generalization capability of the deep networks outside of their training domains. The IADD focuses on 2D object detection and contains more than 97,000 annotated images, covering six common object classes in the field of autonomous vehicles. To improve the generalization of the models, a wide variety of driving conditions and domains, including the city and suburban road settings, adverse weather conditions, and various traffic flows, are presented in the IADD images. The results of exhaustive evaluations conducted on several state-of-the-art convolutional neural networks reveal that not only the trained architectures have performed successfully on test data of the IADD, but also they have upheld high precision in the assessments of generalization capability. In order to challenge the models, broad range of simulations have been performed in the CARLA software environment; which due to the synthetic nature of the simulated images, severe domain shifts have been observed between the CARLA and the IADD. Also, the cross-domain evaluation results have confirmed the efficacy of the IADD in enhancing the generalization ability of the deep learning models. The dataset is available in: https://github.com/ahv1373/IADD.