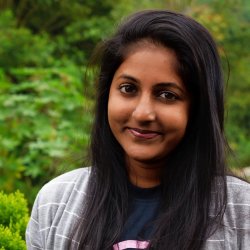
Archchana Sindhujan
About
My research project
Quality Estimation for Neural Machine TranslationEstimating the quality of the translation is considered a highly important task to identify the reliability of the translation and for the improvement of the translation system. Predicting the quality of the translation depends on certain features such as no critical errors, number of named entities, number of prepositional phrases or nouns in source and target etc. Considering these features Quality Estimation(QE) models to obtain the quality score can be implemented using machine learning.
Evaluating the translation is mainly categorized into two parts, automated evaluation and human evaluation. Qualified automated translation evaluation systems should consists the following state of affairs: excessive relationship with human evaluations quantified in the correlation of translation quality, reliability, consistency in the translation result, responsiveness to nuances in quality among systems, massive range of fields, usability and speed. Quality Estimation for translation can be approached in word-level, sentence-level, error detection etc. Since quality estimation is still a growing research area, this study mainly focusses on how we can use linguistically grounded methods to improve the predictions obtained from MT systems by estimating the quality of the translation.
Supervisors
Estimating the quality of the translation is considered a highly important task to identify the reliability of the translation and for the improvement of the translation system. Predicting the quality of the translation depends on certain features such as no critical errors, number of named entities, number of prepositional phrases or nouns in source and target etc. Considering these features Quality Estimation(QE) models to obtain the quality score can be implemented using machine learning.
Evaluating the translation is mainly categorized into two parts, automated evaluation and human evaluation. Qualified automated translation evaluation systems should consists the following state of affairs: excessive relationship with human evaluations quantified in the correlation of translation quality, reliability, consistency in the translation result, responsiveness to nuances in quality among systems, massive range of fields, usability and speed. Quality Estimation for translation can be approached in word-level, sentence-level, error detection etc. Since quality estimation is still a growing research area, this study mainly focusses on how we can use linguistically grounded methods to improve the predictions obtained from MT systems by estimating the quality of the translation.
Publications
Leveraging large language models (LLMs) for various natural language processing tasks has led to superlative claims about their performance. For the evaluation of machine translation (MT), existing research shows that LLMs are able to achieve results comparable to fine-tuned multilingual pre-trained language models. In this paper, we explore what translation information, such as the source, reference, translation errors and annotation guidelines, is needed for LLMs to evaluate MT quality. In addition, we investigate prompting techniques such as zero-shot, Chain of Thought (CoT) and few-shot prompting for eight language pairs covering high-, medium- and low-resource languages, leveraging varying LLM variants. Our findings indicate the importance of reference translations for an LLM-based evaluation. While larger models do not necessarily fare better, they tend to benefit more from CoT prompting, than smaller models. We also observe that LLMs do not always provide a numerical score when generating evaluations, which poses a question on their reliability for the task. Our work presents a comprehensive analysis for resource-constrained and training-less LLM-based evaluation of machine translation. We release the accrued prompt templates, code and data publicly for reproducibility.
Leveraging large language models (LLMs) for various natural language processing tasks has led to superlative claims about their performance. For the evaluation of machine translation (MT), existing research shows that LLMs are able to achieve results comparable to fine-tuned multilingual pre-trained language models. In this paper, we explore what translation information, such as the source, reference, translation errors and annotation guidelines, is needed for LLMs to evaluate MT quality. In addition, we investigate prompting techniques such as zero-shot, Chain of Thought (CoT) and few-shot prompting for eight language pairs covering high-, medium-and low-resource languages, leveraging varying LLM variants. Our findings indicate the importance of reference translations for an LLM-based evaluation. While larger models do not necessarily fare better, they tend to benefit more from CoT prompting, than smaller models. We also observe that LLMs do not always provide a numerical score when generating evaluations, which poses a question on their reliability for the task. Our work presents a comprehensive analysis for resource-constrained and training-less LLM-based evaluation of machine translation. We release the accrued prompt templates, code and data publicly for reproducibility.
This paper investigates the reference-less evaluation of machine translation for low-resource language pairs, known as quality estimation (QE). Segment-level QE is a challenging cross-lingual language understanding task that provides a quality score (0 − 100) to the translated output. We comprehensively evaluate large language models (LLMs) in zero/few-shot scenarios and perform instruction fine-tuning using a novel prompt based on annotation guidelines. Our results indicate that prompt-based approaches are outperformed by the encoder-based fine-tuned QE models. Our error analysis reveals tokenization issues, along with errors due to transliteration and named entities, and argues for refinement in LLM pre-training for cross-lingual tasks. We release the data, and models trained publicly for further research.
Quality Estimation (QE) systems are important in situations where it is necessary to assess the quality of translations, but there is no reference available. This paper describes the approach adopted by the SurreyAI team for addressing the Sentence-Level Direct Assessment shared task in WMT23. The proposed approach builds upon the TransQuest framework, exploring various autoencoder pre-trained language models within the MonoTransQuest architecture using single and ensemble settings. The autoencoder pre-trained language models employed in the proposed systems are XLMV, InfoXLM-large, and XLMR-large. The evaluation utilizes Spearman and Pearson correlation coefficients, assessing the relationship between machine-predicted quality scores and human judgments for 5 language pairs (English-Gujarati, English-Hindi, English-Marathi, English-Tamil and English-Telugu). The MonoTQ-InfoXLM-large approach emerges as a robust strategy, surpassing all other individual models proposed in this study by significantly improving over the baseline for the majority of the language pairs.
Multilingual societies use a mix of two or more languages when communicating. It has become a famous way of communication in social media in South Asian communities. Sinhala-English Code-Mixed Texts (SCMT) are known as the most popular text representation used in Sri Lanka in the informal context such as social media chats, comments, small talks etc. The challenges in utilizing the SCMT sentences are addressed in this paper. The main focus of this study is translating code-mixed sentences written in Sinhala-English to the standard Sinhala language. Since Sinhala is a low-resource language, we were able to collect only a limited number of SCMT-Sinhala parallel sentences. Creating the parallel corpus of SCMT-Sinhala was a time-consuming and costly task. The proposed architecture of Neural Machine Translation(NMT) to translate SCMT text to Sinhala, is built with a combination of normalization pipeline, Long Short Term Memory(LSTM) units, Sequence to Sequence(Seq2Seq) and Teachers Forcing mechanism. The proposed model is evaluated against the current state-of-the-art models using the same experimental setup, which proves the Teacher Forcing Algorithm combined with Seq2Seq and Normalization improves the quality of the translation. The predicted outputs from the model are compared using the BLEU (Bilingual Evaluation Understudy) metric and our proposed model achieved a better BLEU score of 33.89 in the evaluation.
Quality Estimation (QE) systems are important in situations where it is necessary to assess the quality of translations, but there is no reference available. This paper describes the approach adopted by the SurreyAI team for addressing the Sentence-Level Direct Assessment shared task in WMT23. The proposed approach builds upon the TransQuest framework, exploring various autoencoder pre-trained language models within the MonoTransQuest architecture using single and ensemble settings. The autoencoder pre-trained language models employed in the proposed systems are XLMV, InfoXLM-large, and XLMR-large. The evaluation utilizes Spearman and Pearson correlation coefficients, assessing the relationship between machine-predicted quality scores and human judgments for 5 language pairs (English-Gujarati, English-Hindi, English-Marathi, English-Tamil and English-Telugu). The MonoTQ-InfoXLM-large approach emerges as a robust strategy, surpassing all other individual models proposed in this study by significantly improving over the baseline for the majority of the language pairs.
Evaluation of machine translation (MT) is vital to determine the effectiveness of MT systems. This paper investigates quality estimation (QE) for machine translation (MT) for low-resource Indic languages. We analyse the influence of language relatedness within linguistic families and integrate various pre-trained encoders within the MonoTransQuest (MonoTQ) framework. This entails assessing models in single-language configurations before scaling up to multiple-language setups, focusing on languages within and across families, and using approaches grounded in transfer learning. Experimental outcomes and analyses indicate that language-relatedness significantly improves QE performance over baseline, sometimes even surpassing state-of-the-art approaches. Across monolingual and multilingual configurations, we discuss strategic encoder usage as a simple measure to exploit the language interactions within these models improving baseline QE efficiency for quality estimation. This investigation underscores the potential of tailored pre-trained encoders to improve QE performance and discusses the limitations of QE approaches for low-resource scenarios.
Conference Title: 2021 21st International Conference on Advances in ICT for Emerging Regions (ICter) Conference Start Date: 2021, Dec. 2 Conference End Date: 2021, Dec. 3 Conference Location: Colombo, Sri LankaProject Management System important in largescale projects. There are existing project management tools such as Redmine, Microsoft Project, Jira. Most of the existing project management systems only configured for general purposes such as Project Management, Task management, Time line management. None of them capable of generating project groups, track student progress or track client meetings. These functions are very helpful in tracking project progress as well as individual member progress. Hence, the purpose of this research is to introduce set of new features to a project management system with accurate and effective project management capabilities. This system is capable of generating project groups using student’s skills, Grade point average (GPA). Smart project tracking system where it uses project repositories and system generated timeline for the project. Using the project tracking system, Supervisors can manage groups remotely. Automatic peer review also added to identify each student’s contribution to the project and finally a client portal where clients can request solutions for their requirements and students can use them as their module project. Client meetings will be tracked using voice-to-text algorithm and also emotional recognition where it will identify client’s satisfaction. All These system modules will be used to calculate group performance.
Aspect-based sentiment analysis (ABSA) is used in different fields for analyzing customer reviews to project an overall customer opinion on certain products. With the expansion of the internet, people are provided with an inexpensive and time-saving method to express their opinion to a larger audience, while various industries are handed with the opportunity to gather free information from it to obtain market value. The implementation of machine learning methods for the evaluation of aspects related to movies and television series has not been commenced, and it could be a new development for the industry. This study focuses on conducting an ABSA on a movie or a television series based on genre, story as well as cast and crew aspects. The data collected from social media through web scraping is processed to produce adequate results to get a broad understanding on how the popularity of the movie or the television series related to above mentioned aspects. Then, each aspect is further analyzed to gather precise information belonging to each aspect. The accuracy of the results of the proposed system has been achieved over 79%. The results proved that the solution is highly successful than the former works with high business value.
"The Alter" eliminates software product inconsistency by adhering to SDLC best practices and ensuring that the final product complies with HCI principles with minimal supervisor guidance under the pandemic condition. This paper presents two surveys and an interview: A literature survey on current automatic UI generation tools and a user survey on why it is necessary to have a system that generates user interfaces automatically. The first survey reviews the underlying systems and software used to automate the user interface generating process. With the help of the SDLC process (Requirement gathering, UML diagrams generation, generating wireframes, web UI generation, evaluate the application of HCI concepts ) and HCI practices, the second survey attempts to bridge the gap between manual and automatic UI generation. The focus of the interview is on the significance of artifact consistency.
With the rise of online education more attention is being paid to the deficiencies in online learning platforms. Online Learning environments aim to deliver efficacious instructions, but rarely take providing a conventional classroom experience to the students into consideration. Efficient detection of students' learning situations can provide information to teachers to help them identify students having trouble in real-time. This idea has been exploited several times for Intelligent Tutoring Systems, but not yet in other types of learning environments that are less structured. "E-Learn Detector is a web application solution to these existing issues in online learning which consists of unique features such as verifying the user during logging procedure and throughout an examination, detecting suspicious behaviors and presence of multiple users during online examinations and detecting low engagement levels of students during online lectures. "E-Learn Detector" is developed with the aim to provide guidance to students to improve their academic performance and behavior during classroom activities and to induce the best out of the educational activities.
Personalization is one of the most sought out and popular methods for brand recognition and consumer attraction. The usage of deep reinforcement learning due to its' ability to learn actions the way humans learn from experience, if utilized and evaluated properly it can result in a revolutionary effect on personalization. The methodology proposed in this research utilizes deep reinforcement learning where an artificial agent may be trained by interacting with its environment. Utilizing the experience gathered, the agent is able optimize in the form of rewards. The approach explained, can be utilized across applications which can be personalized. Several scenarios ranging from changing the layout of webpages, to rearranging icons on mobile home screens are discussed. The main objective is to develop an API for the web developers and smartphone manufacturers to utilize so that depending on the application personalization can be achieved by enhancing saliency, minimizing selection time, increasing engagement, or an arrangement of these. The technique can manage a variety of adaptations, such as how graphical elements are shown and how they behave. An experiment was conducted which showcased improved user experience considering the position change of the user interface elements thereby personalizing the layout.
Code-mixing is one of the biggest challenges when processing social media text. This paper presents a thorough review on the state of the art code-mixed text processing and identified the main challenges in processing Sinhala code-mixed text. In this study we could identify how researchers conducted different kinds of tasks such as normalization of code-mixed data, word level language identification of the code-mixed text etc. The study lead to identify the challenges in Sinhala code-mixed text such as phonetic transliterations, borrowing of words, spelling errors, embedded languages, the use of numeric characters in words, discourse marker switching etc. Based on this challenges identified, it was necessary to standardize the Singlish text to Sinhala letters, since there are so many representations for the same word. So a dictionary is proposed where Sinhala letters are mapped to Singlish text which could be used as a standardization. Finally the paper discuss about the future work planed on using the proposed dictionary for Sinhala code-mixed data analysis.
Aspect based sentiment analysis (ABSA) is a methodology used in various fields for analyzing the reviews of customers to project an overall opinion on any different product or service. With the evolution of the internet, people are provided with a time saving cheap procedure like this, to express viewpoints to a mass audience. With the intension of gaining market value, numerous fields are bestowed the chance in collecting information from the internet, free of charge. The application of Machine Learning (ML) methods for the procedure of evaluation regarding aspects connected to TV series and movies, has not been initiated. This would be a novel progression for the film industry. Research under this study focuses on implementing ABSA on TV series or movies based on sub aspects of genre as well as sub aspects of cast and crew. As the initial step, web scraping can be used for data collection from social media. Next, data is processed to generate adequate results for anyone who needs a broader understanding on significance of a TV series or a movie related to the aspects mentioned earlier. To collect information belonging to each aspect is further analyzed. The accuracy of the results of the suggested system has been gained high as 79% and above. In conclusion, the accuracy of the solution is greatly successful compared to the previous tasks with high market value.
The agricultural world faces more difficulties due to crop pests that damage or infliction cultivated plants. The main challenges to those interested in cultivation are pest attack and disease. Pests spread the disease, and the yield is decreased. However, it is possible to control pest attacks and infections in the early attack stage to reduce pesticide use and keep the farm safe. Mobile applications can provide accurate identification rather than manual detection. Mobile applications and technologies are created when considering the solution. The importance of the proposed solution is to increase the rate of the plant product and achieve high revenue without any cost. One of the main components used in this system is the image processing technique. The pest images will be taken, and they will be subjected to various preprocessing for noise reduction and enhancement of the pictures. Using image processing, the user can determine the pest's life cycle stage. The user can identify the stage of the damaged plant by applying the classification algorithm. The content analysis is based on the machine learning process, especially using a Convolutional Neural Network. Hence, the proposed system will help to get knowledge of organic pest prevention methods. In the system, we determined the type of pest with 90% accuracy by submitting a damaged leaf and a pest image. The pest's lifecycle stage and stage of the affected plant also can be identified in our system with high accuracy. Moreover, it shows the organic prevention methods.
This paper proposes a recommendation system for the elderly that runs on a consumer smartphone as an interactive mobile application. This study looks at the relationship between depression and the factors contributing to depression in the elderly. Obesity and depression have a bidirectional negative relationship. Older people experience symptoms of depression that have gone undetected and untreated. Therefore, the proposed solution provides the elderly with overcoming depression and loneliness in everyday life and looking after themselves. An elder's life revolves around eating well-balanced meals and staying healthy. This system will include a food picture recognition capability for determining calories and nutritional content. The system will assess the area, size, and volume of food and provide advice such as activities to reduce food's calories. It will also propose alternative healthy food if there is any harmful food in the image. The use of diet to keep improving both health issues is a cornerstone strategy to manage depression in patients. Because chronic diseases and depression have similar physiology, equivalent dietary recommendations could be beneficial. Malnutrition is a significant issue among the elderly. On the other hand, adjusting food-related habits is complicated. Therefore, provide an intelligent meal planning application that recommends appropriate low-calorie food recipes based on the image or voice command of the ingredient.