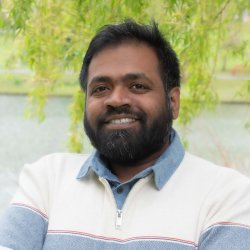
Dr Diptesh Kanojia
About
Biography
Researcher working on problems intersecting the areas of Natural Language Processing (NLP) and Machine Learning (ML) at the Institute for People-Centred AI (PAI) and School of CS and EE. As a research lead, I manage research within Human-Machine Interaction @ PAI, and also the NLP subgroup within the Nature Inspired Computing and Engineering group (NICE) research group @ Computer Science Research Centre. I also lead the teaching on NLP module jointly offered to undergraduate (COM3029) and postgraduate students (COMM061), at our school.
My research focuses on scalable approaches using foundation models for safe human-machine, and human-human interaction, within social and personal digital spaces. With People-Centred focus on Responsible and Inclusive AI, my work contributes to low-resource languages and addressing societal challenges like digital accessibility, online toxicity, and misinformation. Broadly, my interests lie in modelling information from linguistics, audio-visual signals (Multimodality), social phenomena (SocialNLP), and cognitive behaviour (CognitiveNLP), to improve machine understanding. Our research outcomes like data, code, and computational models are regularly shared publicly at SurreyNLP Github, and HuggingFace spaces.
Previously, I was a research fellow at the Centre for Translation Studies, and continue collaborating on relevant areas. I completed a Joint PhD from the IITB-Monash Research Academy [IIT Bombay, India & Monash University, Australia], and currently work with industry and academic partners, on areas from above. Before starting my PhD, I was a Research Engineer at the CFILT Lab, IIT Bombay, India, where my investigations led to publications in diverse sub-areas of NLP and AI.
Areas of specialism
University roles and responsibilities
- Academic Integrity Officer, 2024-present
- Health and Safety Incharge (Area 5), 2023-present
My qualifications
Thesis: "Investigations into Distributional Semantics for Cognate Detection and Phylogenetics"
News
In the media
ResearchResearch interests
Natural Language Processing, Machine Learning, Evaluation and Correction for Machine Translation, Cognitive NLP, Human-Machine Interaction with Multimodal Information modelling, Low-resource Languages.
Research projects
Lightweight Contextual Embeddings for Product SearchThis project in collaboration with eBay Inc. proposes the use of scalable approaches for product retrieval and ranking. We published our findings at CIKM Industry Day Talk, and as a publication at EMNLP Industry Track research paper.
Co-I: Prof Constantin Orăsan
Quality Estimation for Low-resource Indic LanguagesData Curation project for quality estimation of machine translation which led to our contributions to Findings of the QE and APE shared tasks in 2022, 2023, and 2024.
Research interests
Natural Language Processing, Machine Learning, Evaluation and Correction for Machine Translation, Cognitive NLP, Human-Machine Interaction with Multimodal Information modelling, Low-resource Languages.
Research projects
This project in collaboration with eBay Inc. proposes the use of scalable approaches for product retrieval and ranking. We published our findings at CIKM Industry Day Talk, and as a publication at EMNLP Industry Track research paper.
Co-I: Prof Constantin Orăsan
Data Curation project for quality estimation of machine translation which led to our contributions to Findings of the QE and APE shared tasks in 2022, 2023, and 2024.
Supervision
Postgraduate research supervision
Postdoc
Hadeel Saadany [2023-2024; Lightweight Contextual Embeddings for Product Search (w/ eBay Inc., USA)]
Primary Supervision (ongoing)
Archchana Sindhujan (PAI; Co-supervisor: Prof Constantin Orăsan)
Fatemeh Nazarieh (NICE, FEPS; Co-supervisor: Dr Muhammad Rana, Dr Zhenhua Feng)
Girish Koushik (NICE, FEPS; Co-supervisor: Prof Helen Treharne)
Xinran Liu (NICE, FEPS; Co-supervisor: Prof Wenwu Wang, Dr Zhenhua Feng)
Co-supervision (ongoing)
Swapnil Bhosale (PAI; Supervisor: Dr Xiatian Zhu)
Shenbin Qian (CTS, FASS; Supervisor: Prof Constantin Orăsan, Dr Félix do Carmo)
Aisha Saeid (NICE, FEPS; Supervisor: Prof Ferrante Neri)
Olutope Adebiyi (LLL, FASS; Supervisor: Dr Doris Dippold)
Teaching
I lead the teaching on Natural Language Processing (NLP) module which is an optional for final year undergraduate students on the BSc Computer Science programme (COM3029; FHEQ Level 6), and MSc Data Science and MSc Artificial Intelligence programmes (COMM061; FHEQ Level 7).
Within additional learning hours, every semester I introduce an aspect of skill development or learning in AI along with the module. In 2023/24, student were encouraged to read papers on multimodal information processing in AI, try out different coding implementations, helping them complete the coursework using innovative approaches.
Publications
Highlights
Google Scholar has a complete list of my publications. A select few publications are shown below.
Social media platforms enable the propagation of hateful content across different modalities such as textual, auditory, and visual, necessitating effective detection methods. While recent approaches have shown promise in handling individual modalities, their effectiveness across different modality combinations remains unexplored. Our paper presents a systematic analysis of fusion-based approaches for multimodal hate detection, focusing on performance across video and image-based content. Our comprehensive evaluation reveals significant modality-specific limitations: while simple embedding fusion achieves state-of-the-art performance on video content with a 9.9% points F1-score improvement, it struggles with complex image-text relationships in memes. Through detailed ablation studies and error analysis, we demonstrate how current fusion approaches fail to capture nuanced cross-modal interactions, particularly in cases involving benign confounders. Our findings provide crucial insights for developing more robust hate detection systems and highlight the need for modality-specific architectural considerations. The code is available at: https://github.com/surrey-nlp/Video-vs-Meme-Hate.
Evaluating machine translation (MT) of user-generated content (UGC) involves unique challenges such as checking whether the nuance of emotions from the source are preserved in the target text. Recent studies have proposed emotion-related datasets, frameworks and models to automatically evaluate MT quality of Chinese UGC, without relying on reference translations. However, whether these models are robust to the challenge of preserving emotional nuances has been left largely unexplored. To address this gap, we introduce a novel method inspired by information theory which generates challenging Chinese homophone words related to emotions, by leveraging the concept of self-information. Our approach generates homophones that were observed to cause translation errors in emotion preservation, and exposes vulnerabilities in MT systems and their evaluation methods when tackling emotional UGC. We evaluate the efficacy of our method using human evaluation for the quality of these generated homophones, and compare it with an existing one, showing that our method achieves higher correlation with human judgments. The generated Chinese homophones, along with their manual translations, are utilized to generate perturbations and to probe the robustness of existing quality evaluation models, including models trained using multi-task learning, fine-tuned variants of multilingual language models, as well as large language models (LLMs). Our results indicate that LLMs with larger size exhibit higher stability and robustness to such perturbations. We release our data and code for reproducibility and further research.
State-of-the-art natural language processing (NLP) models are trained on massive training corpora, and report a superlative performance on evaluation datasets. This survey delves into an important attribute of these datasets: the dialect of a language. Motivated by the performance degradation of NLP models for dialectal datasets and its implications for the equity of language technologies, we survey past research in NLP for dialects in terms of datasets, and approaches. We describe a wide range of NLP tasks in terms of two categories: natural language understanding (NLU) (for tasks such as dialect classification, sentiment analysis, parsing, and NLU benchmarks) and natural language generation (NLG) (for summarisation, machine translation, and dialogue systems). The survey is also broad in its coverage of languages which include English, Arabic, German, among others. We observe that past work in NLP concerning dialects goes deeper than mere dialect classification, and extends to several NLU and NLG tasks. For these tasks, we describe classical machine learning using statistical models, along with the recent deep learning-based approaches based on pre-trained language models. We expect that this survey will be useful to NLP researchers interested in building equitable language technologies by rethinking LLM benchmarks and model architectures.
This study explores the use of Google Translate (GT) for translating mental healthcare (MHealth) information and evaluates its accuracy, comprehensibility, and implications for multilingual healthcare communication through analysing GT output in the MHealth domain from English to Persian, Arabic, Turkish, Romanian, and Spanish. Two datasets comprising MHealth information from the UK National Health Service website and information leaflets from The Royal College of Psychiatrists were used. Native speakers of the target languages manually assessed the GT translations, focusing on medical terminology accuracy, comprehensibility, and critical syntactic/semantic errors. GT output analysis revealed challenges in accurately translating medical terminology, particularly in Arabic, Romanian, and Persian. Fluency issues were prevalent across various languages, affecting comprehension, mainly in Arabic and Spanish. Critical errors arose in specific contexts, such as bullet-point formatting, specifically in Persian, Turkish, and Romanian. Although improvements are seen in longer-text translations, there remains a need to enhance accuracy in medical and mental health terminology and fluency, whilst also addressing formatting issues for a more seamless user experience. The findings highlight the need to use customised translation engines for Mhealth translation and the challenges when relying solely on machine-translated medical content, emphasising the crucial role of human reviewers in multilingual healthcare communication.
Leveraging large language models (LLMs) for various natural language processing tasks has led to superlative claims about their performance. For the evaluation of machine translation (MT), existing research shows that LLMs are able to achieve results comparable to fine-tuned multilingual pre-trained language models. In this paper, we explore what translation information, such as the source, reference, translation errors and annotation guidelines, is needed for LLMs to evaluate MT quality. In addition, we investigate prompting techniques such as zero-shot, Chain of Thought (CoT) and few-shot prompting for eight language pairs covering high-, medium- and low-resource languages, leveraging varying LLM variants. Our findings indicate the importance of reference translations for an LLM-based evaluation. While larger models do not necessarily fare better, they tend to benefit more from CoT prompting, than smaller models. We also observe that LLMs do not always provide a numerical score when generating evaluations, which poses a question on their reliability for the task. Our work presents a comprehensive analysis for resource-constrained and training-less LLM-based evaluation of machine translation. We release the accrued prompt templates, code and data publicly for reproducibility.
This talk addresses the challenge of improving user experience on e-commerce platforms by enhancing product ranking relevant to user's search queries. Queries such as 'S2716DG' consist of alphanumeric characters where a letter or number can signify important detail for the product/model. Speaker describes recent research where we curate samples from existing datasets at eBay, manually annotated with buyer-centric relevance scores, and centrality scores which reflect how well the product title matches the user's intent. We introduce a User-intent Centrality Optimization (UCO) approach for existing models, which optimizes for the user intent in semantic product search. To that end, we propose a dual-loss based optimization to handle hard negatives, i.e., product titles that are semantically relevant but do not reflect the user's intent. Our contributions include curating a challenging evaluation set and implementing UCO, resulting in significant improvements in product ranking efficiency, observed for different evaluation metrics. Our work aims to ensure that the most buyer-centric titles for a query are ranked higher, thereby, enhancing the user experience on e-commerce platforms.
Leveraging large language models (LLMs) for various natural language processing tasks has led to superlative claims about their performance. For the evaluation of machine translation (MT), existing research shows that LLMs are able to achieve results comparable to fine-tuned multilingual pre-trained language models. In this paper, we explore what translation information, such as the source, reference, translation errors and annotation guidelines, is needed for LLMs to evaluate MT quality. In addition, we investigate prompting techniques such as zero-shot, Chain of Thought (CoT) and few-shot prompting for eight language pairs covering high-, medium-and low-resource languages, leveraging varying LLM variants. Our findings indicate the importance of reference translations for an LLM-based evaluation. While larger models do not necessarily fare better, they tend to benefit more from CoT prompting, than smaller models. We also observe that LLMs do not always provide a numerical score when generating evaluations, which poses a question on their reliability for the task. Our work presents a comprehensive analysis for resource-constrained and training-less LLM-based evaluation of machine translation. We release the accrued prompt templates, code and data publicly for reproducibility.
This paper investigates the reference-less evaluation of machine translation for low-resource language pairs, known as quality estimation (QE). Segment-level QE is a challenging cross-lingual language understanding task that provides a quality score (0 − 100) to the translated output. We comprehensively evaluate large language models (LLMs) in zero/few-shot scenarios and perform instruction fine-tuning using a novel prompt based on annotation guidelines. Our results indicate that prompt-based approaches are outperformed by the encoder-based fine-tuned QE models. Our error analysis reveals tokenization issues, along with errors due to transliteration and named entities, and argues for refinement in LLM pre-training for cross-lingual tasks. We release the data, and models trained publicly for further research.
Audio-to-talking face generation stands at the forefront of advancements in generative AI. It bridges the gap between audio and visual representations by generating synchronized and realistic talking faces. Despite recent progress, the lack of realism in animated faces, asynchronous audio-lip movements, and computational burden remain key barriers to practical applications. To address these challenges, we introduce a novel approach, StableTalk, leveraging the emerging capabilities of Stable diffusion models and vision Transformers for Talking face generation. We also integrate the Re-attention mechanism and adversarial loss to improve the consistency of facial animations and synchronization with a given audio input. More importantly, the computational efficiency of our method has been notably enhanced by optimizing operations within the latent space and dynamically adjusting the focus on different parts of the visual content based on the provided conditions. Our experimental results demonstrate the superiority of StableTalk over the existing approaches in image quality, audio-lip synchronization, and computational efficiency.
Sound Event Detection (SED) aims to predict the temporal boundaries of all the events of interest and their class labels, given an unconstrained audio sample. Taking either the splitand-classify (i.e., frame-level) strategy or the more principled event-level modeling approach, all existing methods consider the SED problem from the discriminative learning perspective. In this work, we reformulate the SED problem by taking a generative learning perspective. Specifically, we aim to generate sound temporal boundaries from noisy proposals in a denoising diffusion process, conditioned on a target audio sample. During training, our model learns to reverse the noising process by converting noisy latent queries to the groundtruth versions in the elegant Transformer decoder framework. Doing so enables the model generate accurate event boundaries from even noisy queries during inference. Extensive experiments on the Urban-SED and EPIC-Sounds datasets demonstrate that our model significantly outperforms existing alternatives, with 40+% faster convergence in training.
In this paper, we focus on how current Machine Translation (MT) tools perform on the translation of emotion-loaded texts by evaluating outputs from Google Translate according to a framework proposed in this paper. We propose this evaluation framework based on the Multidimensional Quality Metrics (MQM) and perform a detailed error analysis of the MT outputs. From our analysis, we observe that about 50% of the MT outputs fail to preserve the original emotion. After further analysis of the errors, we find that emotion carrying words and linguistic phenomena such as polysemous words, negation, abbreviation etc., are common causes for these translation errors.
Sound Event Detection (SED) aims to predict the temporal boundaries of all the events of interest and their class labels, given an unconstrained audio sample. Taking either the split-and-classify (i.e., frame-level) strategy or the more principled event-level modeling approach, all existing methods consider the SED problem from the discriminative learning perspective. In this work, we reformulate the SED problem by taking a generative learning perspective. Specifically, we aim to generate sound temporal boundaries from noisy proposals in a denoising diffusion process, conditioned on a target audio sample. During training, our model learns to reverse the noising process by converting noisy latent queries to the groundtruth versions in the elegant Transformer decoder framework. Doing so enables the model generate accurate event boundaries from even noisy queries during inference. Extensive experiments on the Urban-SED and EPIC-Sounds datasets demonstrate that our model significantly outperforms existing alternatives, with 40+% faster convergence in training. Code: https://github.com/Surrey-UPLab/DiffSED.
Novel view acoustic synthesis (NVAS) aims to render binaural audio at any target viewpoint, given a mono audio emitted by a sound source at a 3D scene. Existing methods have proposed NeRF-based implicit models to exploit visual cues as a condition for synthesizing binaural audio. However, in addition to low efficiency originating from heavy NeRF rendering, these methods all have a limited ability of characterizing the entire scene environment such as room geometry, material properties, and the spatial relation between the listener and sound source. To address these issues, we propose a novel Audio-Visual Gaussian Splatting (AV-GS) model. To obtain a material-aware and geometry-aware condition for audio synthesis, we learn an explicit point-based scene representation with an audio-guidance parameter on locally initialized Gaussian points, taking into account the space relation from the listener and sound source. To make the visual scene model audio adaptive, we propose a point densification and pruning strategy to optimally distribute the Gaussian points, with the per-point contribution in sound propagation (e.g., more points needed for texture-less wall surfaces as they affect sound path diversion). Extensive experiments validate the superiority of our AV-GS over existing alternatives on the real-world RWAS and simulation-based SoundSpaces datasets.
Quality Estimation (QE) systems are important in situations where it is necessary to assess the quality of translations, but there is no reference available. This paper describes the approach adopted by the SurreyAI team for addressing the Sentence-Level Direct Assessment shared task in WMT23. The proposed approach builds upon the TransQuest framework, exploring various autoencoder pre-trained language models within the MonoTransQuest architecture using single and ensemble settings. The autoencoder pre-trained language models employed in the proposed systems are XLMV, InfoXLM-large, and XLMR-large. The evaluation utilizes Spearman and Pearson correlation coefficients, assessing the relationship between machine-predicted quality scores and human judgments for 5 language pairs (English-Gujarati, English-Hindi, English-Marathi, English-Tamil and English-Telugu). The MonoTQ-InfoXLM-large approach emerges as a robust strategy, surpassing all other individual models proposed in this study by significantly improving over the baseline for the majority of the language pairs.
Cognates are present in multiple variants of the same text across different languages. Computational Phylogenetics uses algorithms and techniques to analyze these variants and infer phylogenetic trees for a hypothesized accurate representation based on the output of the computational algorithm used. In our work, we detect cognates among a few Indian languages namely Hindi, Marathi, Punjabi, and Sanskrit for helping build cognate sets for phylogenetic inference. Cognate detection helps phylogenetic inference by helping isolate diachronic sound changes and thus detect the words of a common origin. A cognate set manually annotated with the help of a lexicographer is generally used to automatically infer phylogenetic trees. Our work creates cognate sets of each language pair and infers phylogenetic trees based on a bayesian framework using the Maximum likelihood method. We also implement our work to an online interface and infer phylogenetic trees based on automatically detected cognate sets. The online interface helps create phylogenetic trees based on the textual data provided as an input. It helps a lexicographer provide manual input of data, edit the data based on their expert opinion and eventually create phylogenetic trees based on various algorithms including our work on automatically creating cognate sets. We go on to discuss the nuances in detection cognates with respect to these Indian languages and also discuss the categorization of Cognate words i.e., "Tatasama" and "Tadbhava" words.
Quality Estimation (QE) systems are important in situations where it is necessary to assess the quality of translations, but there is no reference available. This paper describes the approach adopted by the SurreyAI team for addressing the Sentence-Level Direct Assessment shared task in WMT23. The proposed approach builds upon the TransQuest framework, exploring various autoencoder pre-trained language models within the MonoTransQuest architecture using single and ensemble settings. The autoencoder pre-trained language models employed in the proposed systems are XLMV, InfoXLM-large, and XLMR-large. The evaluation utilizes Spearman and Pearson correlation coefficients, assessing the relationship between machine-predicted quality scores and human judgments for 5 language pairs (English-Gujarati, English-Hindi, English-Marathi, English-Tamil and English-Telugu). The MonoTQ-InfoXLM-large approach emerges as a robust strategy, surpassing all other individual models proposed in this study by significantly improving over the baseline for the majority of the language pairs.
Wordnets are rich lexico-semantic resources. Linked wordnets are extensions of wordnets, which link similar concepts in wordnets of different languages. Such resources are extremely useful in many Natural Language Processing (NLP) applications, primarily those based on knowledge-based approaches. In such approaches, these resources are considered as gold standard/oracle. Thus, it is crucial that these resources hold correct information. Thereby, they are created by human experts. However, manual maintenance of such resources is a tedious and costly affair. Thus techniques that can aid the experts are desirable. In this paper, we propose an approach to link wordnets. Given a synset of the source language, the approach returns a ranked list of potential candidate synsets in the target language from which the human expert can choose the correct one(s). Our technique is able to retrieve a winner synset in the top 10 ranked list for 60% of all synsets and 70% of noun synsets.
Gaze behaviour has been used as a way to gather cognitive information for a number of years. In this paper, we discuss the use of gaze behaviour in solving different tasks in natural language processing (NLP) without having to record it at test time. This is because the collection of gaze behaviour is a costly task, both in terms of time and money. Hence, in this paper, we focus on research done to alleviate the need for recording gaze behaviour at run time. We also mention different eye tracking corpora in multiple languages, which are currently available and can be used in natural language processing. We conclude our paper by discussing applications in a domain - education - and how learning gaze behaviour can help in solving the tasks of complex word identification and automatic essay grading.
This paper summarises the submissions our team, SURREY-CTS-NLP has made for the WASSA 2022 Shared Task for the prediction of empathy, distress and emotion. In this work, we tested different learning strategies, like ensemble learning and multi-task learning, as well as several large language models, but our primary focus was on analysing and extracting emotion-intensive features from both the essays in the training data and the news articles, to better predict empathy and distress scores from the perspective of discourse and sentiment analysis. We propose several text feature extraction schemes to compensate the small size of training examples for fine-tuning pretrained language models, including methods based on Rhetorical Structure Theory (RST) parsing, cosine similarity and sentiment score. Our best submissions achieve an average Pearson correlation score of 0.518 for the empathy prediction task and an F1 score of 0.571 for the emotion prediction task(1), indicating that using these schemes to extract emotion-intensive information can help improve model performance.
Automatic Cognate Detection (ACD) is a challenging task which has been utilized to help NLP applications like Machine Translation, Information Retrieval and Computational Phylogenetics. Unidentified cognate pairs can pose a challenge to these applications and result in a degradation of performance. In this paper, we detect cognate word pairs among ten Indian languages with Hindi and use deep learning methodologies to predict whether a word pair is cognate or not. We identify IndoWordnet as a potential resource to detect cognate word pairs based on orthographic similarity-based methods and train neural network models using the data obtained from it. We identify parallel corpora as another potential resource and perform the same experiments for them. We also validate the contribution of Wordnets through further experimentation and report improved performance of up to 26%. We discuss the nuances of cognate detection among closely related Indian languages and release the lists of detected cognates as a dataset. We also observe the behaviour of, to an extent, unrelated Indian language pairs and release the lists of detected cognates among them as well.
Current Machine Translation (MT) systems achieve very good results on a growing variety of language pairs and datasets. However, they are known to produce fluent translation outputs that can contain important meaning errors, thus undermining their reliability in practice. Quality Estimation (QE) is the task of automatically assessing the performance of MT systems at test time. Thus, in order to be useful, QE systems should be able to detect such errors. However, this ability is yet to be tested in the current evaluation practices, where QE systems are assessed only in terms of their correlation with human judgements. In this work, we bridge this gap by proposing a general methodology for adversarial testing of QE for MT. First, we show that despite a high correlation with human judgements achieved by the recent SOTA, certain types of meaning errors are still problematic for QE to detect. Second, we show that on average, the ability of a given model to discriminate between meaning-preserving and meaning-altering perturbations is predictive of its overall performance, thus potentially allowing for comparing QE systems without relying on manual quality annotation.
Quality Estimation (QE) is the task of evaluating machine translation output in the absence of reference translation. Conventional approaches to QE involve training separate models at different levels of granularity viz., word-level, sentence-level, and document-level, which sometimes lead to inconsistent predictions for the same input. To overcome this limitation, we focus on jointly training a single model for sentence-level and word-level QE tasks in a multi-task learning framework. Using two multi-task learning-based QE approaches , we show that multi-task learning improves the performance of both tasks. We evaluate these approaches by performing experiments in different settings, viz., single-pair, multi-pair, and zero-shot. We compare the multi-task learning-based approach with base-line QE models trained on single tasks and observe an improvement of up to 4.28% in Pearson's correlation (r) at sentence-level and 8.46% in F1-score at word-level, in the single-pair setting. In the multi-pair setting, we observe improvements of up to 3.04% at sentence-level and 13.74% at word-level; while in the zero-shot setting, we also observe improvements of up to 5.26% and 3.05%, respectively. We make the models proposed in this paper publicly available.
Cognates are present in multiple variants of the same text across different languages (e.g., "hund" in German and "hound" in English language mean "dog"). They pose a challenge to various Natural Language Processing (NLP) applications such as Machine Translation, Cross-lingual Sense Disambiguation, Computational Phylogenetics, and Information Retrieval. A possible solution to address this challenge is to identify cognates across language pairs. In this paper, we describe the creation of two cognate datasets for twelve Indian languages, namely Sanskrit, Hindi, Assamese, Oriya, Kannada, Gujarati, Tamil, Telugu, Punjabi, Bengali, Marathi, and Malayalam. We digitize the cognate data from an Indian language cognate dictionary and utilize linked Indian language Wordnets to generate cognate sets. Additionally, we use the Wordnet data to create a False Friends' dataset for eleven language pairs. We also evaluate the efficacy of our dataset using previously available baseline cognate detection approaches. We also perform a manual evaluation with the help of lexicographers and release the curated gold-standard dataset with this paper.
Dense word vectors or 'word embeddings' which encode semantic properties of words, have now become integral to NLP tasks like Machine Translation (MT), Question Answering (QA), Word Sense Disambiguation (WSD), and Information Retrieval (IR). In this paper, we use various existing approaches to create multiple word embeddings for 14 Indian languages. We place these embeddings for all these languages, viz., Assamese, Bengali, Gujarati, Hindi, Kannada, Konkani, Malayalam, Marathi, Nepali, Odiya, Punjabi, Sanskrit, Tamil, and Telugu in a single repository. Relatively newer approaches that emphasize catering to context (BERT, ELMo, etc.) have shown significant improvements, but require a large amount of resources to generate usable models. We release pre-trained embeddings generated using both contextual and non-contextual approaches. We also use MUSE and XLM to train cross-lingual embeddings for all pairs of the aforementioned languages. To show the efficacy of our embeddings, we evaluate our embedding models on XPOS, UPOS and NER tasks for all these languages. We release a total of 436 models using 8 different approaches. We hope they are useful for the resource-constrained Indian language NLP. The title of this paper refers to the famous novel 'A Passage to India' by E.M. Forster, published initially in 1924.
Automatic detection of cognates helps downstream NLP tasks of Machine Translation, Cross-lingual Information Retrieval, Computational Phylogenetics and Cross-lingual Named Entity Recognition. Previous approaches for the task of cognate detection use orthographic, phonetic and semantic similarity based features sets. In this paper, we propose a novel method for enriching the feature sets, with cognitive features extracted from human readers' gaze behaviour. We collect gaze behaviour data for a small sample of cognates and show that extracted cognitive features help the task of cognate detection. However, gaze data collection and annotation is a costly task. We use the collected gaze behaviour data to predict cognitive features for a larger sample and show that predicted cognitive features, also, significantly improve the task performance. We report improvements of 10% with the collected gaze features, and 12% using the predicted gaze features, over the previously proposed approaches. Furthermore, we release the collected gaze behaviour data along with our code and cross-lingual models.
The gaze behaviour of a reader is helpful in solving several NLP tasks such as automatic essay grading. However, collecting gaze behaviour from readers is costly in terms of time and money. In this paper, we propose a way to improve automatic essay grading using gaze behaviour, which is learnt at run time using a multi-task learning framework. To demonstrate the efficacy of this multi-task learning based approach to automatic essay grading, we collect gaze behaviour for 48 essays across 4 essay sets, and learn gaze behaviour for the rest of the essays, numbering over 7000 essays. Using the learnt gaze behaviour, we can achieve a statistically significant improvement in performance over the state-of-the-art system for the essay sets where we have gaze data. We also achieve a statistically significant improvement for 4 other essay sets, numbering about 6000 essays, where we have no gaze behaviour data available. Our approach establishes that learning gaze behaviour improves automatic essay grading.
Wordnets are rich lexico-semantic resources. Linked wordnets are extensions of wordnets, which link similar concepts in wordnets of different languages. Such resources are extremely useful in many Natural Language Processing (NLP) applications, primarily those based on knowledge-based approaches. In such approaches, these resources are considered as gold standard/oracle. Thus, it is crucial that these resources hold correct information. Thereby, they are created by human experts. However, human experts in multiple languages are hard to come by. Thus, the community would benefit from sharing of such manually created resources. In this paper, we release mappings of 18 Indian language wordnets linked with Princeton WordNet. We believe that availability of such resources will have a direct impact on the progress in NLP for these languages.
The detection and extraction of abbreviations from unstructured texts can help to improve the performance of Natural Language Processing tasks, such as machine translation and information retrieval. However, in terms of publicly available datasets, there is not enough data for training deep-neural-networks-based models to the point of generalising well over data. This paper presents PLOD, a large-scale dataset for abbreviation detection and extraction that contains 160k+ segments automatically annotated with abbreviations and their long forms. We performed manual validation over a set of instances and a complete automatic validation for this dataset. We then used it to generate several baseline models for detecting abbreviations and long forms. The best models achieved an F1-score of 0.92 for abbreviations and 0.89 for detecting their corresponding long forms. We release this dataset along with our code and all the models publicly in this Github Repository.
Cross-domain sentiment analysis (CDSA) helps to address the problem of data scarcity in scenarios where labelled data for a domain (known as the target domain) is unavailable or insufficient. However, the decision to choose a domain (known as the source domain) to leverage from is, at best, intuitive. In this paper, we investigate text similarity metrics to facilitate source domain selection for CDSA. We report results on 20 domains (all possible pairs) using 11 similarity metrics. Specifically, we compare CDSA performance with these metrics for different domain-pairs to enable the selection of a suitable source domain, given a target domain. These metrics include two novel metrics for evaluating domain adaptability to help source domain selection of labelled data and utilize word and sentence-based embeddings as metrics for unlabelled data. The goal of our experiments is a recommendation chart that gives the K best source domains for CDSA for a given target domain. We show that the best K source domains returned by our similarity metrics have a precision of over 50%, for varying values of K.
Creoles represent an under-explored and marginalized group of languages, with few available resources for NLP research. While the genealogical ties between Creoles and other highly-resourced languages imply a significant potential for transfer learning, this potential is hampered due to this lack of annotated data. In this work we present CreoleVal, a collection of benchmark datasets spanning 8 different NLP tasks, covering up to 28 Creole languages; it is an aggregate of brand new development datasets for machine comprehension, relation classification, and machine translation for Creoles, in addition to a practical gateway to a handful of preexisting benchmarks. For each benchmark, we conduct baseline experiments in a zero-shot setting in order to further ascertain the capabilities and limitations of transfer learning for Creoles. Ultimately, the goal of CreoleVal is to empower research on Creoles in NLP and computational linguistics. We hope this resource will contribute to technological inclusion for Creole language users around the globe.
Audio-Visual Segmentation (AVS) aims to precisely outline audible objects in a visual scene at the pixel level. Existing AVS methods require fine-grained annotations of audio-mask pairs in supervised learning fashion. This limits their scalability since it is time consuming and tedious to acquire such cross-modality pixel level labels. To overcome this obstacle, in this work we introduce unsupervised audio-visual segmentation with no need for task-specific data annotations and model training. For tackling this newly proposed problem, we formulate a novel Cross-Modality Semantic Filtering (CMSF) approach to accurately associate the underlying audio-mask pairs by leveraging the off-the-shelf multi-modal foundation models (e.g., detection [1], open-world segmentation [2] and multi-modal alignment [3]). Guiding the proposal generation by either audio or visual cues, we design two training-free variants: AT-GDINO-SAM and OWOD-BIND. Extensive experiments on the AVS-Bench dataset show that our unsupervised approach can perform well in comparison to prior art supervised counterparts across complex scenarios with multiple auditory objects. Particularly, in situations where existing supervised AVS methods struggle with overlapping foreground objects, our models still excel in accurately segmenting overlapped auditory objects. Our code will be publicly released.
We report the results of the WMT 2022 shared task on Quality Estimation, in which the challenge is to predict the quality of the output of neural machine translation systems at the word and sentence levels, without access to reference translations. This edition introduces a few novel aspects and extensions that aim to enable more fine-grained, and explainable quality estimation approaches. We introduce an updated quality annotation scheme using Multidimensional Quality Metrics to obtain sentence- and word-level quality scores for three language pairs. We also extend the Direct Assessments and post-edit data (MLQE-PE) to new language pairs: we present a novel and large dataset on English-Marathi, as well as a zero-shot test set on English-Yoruba. Further, we include an explainability sub-task for all language pairs and present a new format of a critical error detection task for two new language pairs. Participants from 11 different teams submitted altogether 991 systems to different task variants and language pairs.
The introduction of the MUStARD dataset, and its emotion recognition extension MUStARD++, have identified sarcasm to be a multi-modal phenomenon -- expressed not only in natural language text, but also through manners of speech (like tonality and intonation) and visual cues (facial expression). With this work, we aim to perform a rigorous benchmarking of the MUStARD++ dataset by considering state-of-the-art language, speech, and visual encoders, for fully utilizing the totality of the multi-modal richness that it has to offer, achieving a 2\% improvement in macro-F1 over the existing benchmark. Additionally, to cure the imbalance in the `sarcasm type' category in MUStARD++, we propose an extension, which we call \emph{MUStARD++ Balanced}, benchmarking the same with instances from the extension split across both train and test sets, achieving a further 2.4\% macro-F1 boost. The new clips were taken from a novel source -- the TV show, House MD, which adds to the diversity of the dataset, and were manually annotated by multiple annotators with substantial inter-annotator agreement in terms of Cohen's kappa and Krippendorf's alpha. Our code, extended data, and SOTA benchmark models are made public.
Cognates are variants of the same lexical form across different languages; for example 'fonema' in Spanish and 'phoneme' in English are cognates, both of which mean 'a unit of sound'. The task of automatic detection of cognates among any two languages can help downstream NLP tasks such as Cross-lingual Information Retrieval, Computational Phylogenetics, and Machine Translation. In this paper, we demonstrate the use of cross-lingual word embeddings for detecting cognates among fourteen Indian Languages. Our approach introduces the use of context from a knowledge graph to generate improved feature representations for cognate detection. We, then, evaluate the impact of our cognate detection mechanism on neural machine translation (NMT), as a downstream task. We evaluate our methods to detect cognates on a challenging dataset of twelve Indian languages, namely, Sanskrit, Hindi, Assamese, Oriya, Kannada, Gujarati, Tamil, Telugu, Punjabi, Bengali, Marathi, and Malayalam. Additionally, we create evaluation datasets for two more Indian languages, Konkani and Nepali. We observe an improvement of up to 18% points, in terms of F-score, for cognate detection. Furthermore, we observe that cognates extracted using our method help improve NMT quality by up to 2.76 BLEU. We also release our code, newly constructed datasets and cross-lingual models publicly.
Social media platforms have become new battlegrounds for anti-social elements, with misinformation being the weapon of choice. Fact-checking organizations try to debunk as many claims as possible while staying true to their journalistic processes but cannot cope with its rapid dissemination. We believe that the solution lies in partial automation of the fact-checking life cycle, saving human time for tasks which require high cognition. We propose a new workflow for efficiently detecting previously fact-checked claims that uses abstractive summarization to generate crisp queries. These queries can then be executed on a general-purpose retrieval system associated with a collection of previously fact-checked claims. We curate an abstractive text summarization dataset comprising noisy claims from Twitter and their gold summaries. It is shown that retrieval performance improves 2x by using popular out-of-the-box summarization models and 3x by fine-tuning them on the accompanying dataset compared to verbatim querying. Our approach achieves Recall@5 and MRR of 35% and 0.3, compared to baseline values of 10% and 0.1, respectively. Our dataset, code, and models are available publicly: https://github.com/varadhbhatnagar/FC-Claim-Det/
In today's digital world language technology has gained importance. Several softwares, have been developed and are available in the field of computational linguistics. Such tools play a crucial role in making classical language texts easily accessible. Some Indian philosophical schools have contributed towards various techniques of verbal cognition to analyze sentences correctly. These theories can be used to build computational tools for word sense disambiguation (WSD). In the absence of WSD, one cannot have proper verbal cognition. These theories considered the concept of 'Yogyat\=a' (congruity or compatibility) as the indispensable cause of verbal cognition. In this work, we come up with some insights on the basis of these theories to create a tool that will capture Yogyat\=a of words. We describe the problem of ambiguity in a text and present a method to resolve it computationally with the help of Yogyat\=a. Here, only two major schools i.e. Ny\=aya and Vy\=akarana are considered. Our paper attempts to show the implication of the creation of our tool in this area. Also, our tool involves the creation of an 'ontological tag-set' as well as strategies to mark up the lexicon. The introductory description of ablation is also covered in this paper. Such strategies and some case studies shall form the core of our paper.
Computational Humour (CH) has attracted the interest of Natural Language Processing and Computational Linguistics communities. Creating datasets for automatic measurement of humour quotient is difficult due to multiple possible interpretations of the content. In this work, we create a multi-modal humour-annotated dataset (similar to 40 hours) using stand-up comedy clips. We devise a novel scoring mechanism to annotate the training data with a humour quotient score using the audience's laughter. The normalized duration (laughter duration divided by the clip duration) of laughter in each clip is used to compute this humour coefficient score on a five-point scale (0-4). This method of scoring is validated by comparing with manually annotated scores, wherein a quadratic weighted kappa of 0.6 is obtained. We use this dataset to train a model that provides a "funniness" score, on a five-point scale, given the audio and its corresponding text. We compare various neural language models for the task of humour-rating and achieve an accuracy of 0:813 in terms of Quadratic Weighted Kappa (QWK). Our "Open Mic" dataset is released for further research along with the code.
Automatic essay grading (AEG) is a process in which machines assign a grade to an essay written in response to a topic, called the prompt. Zero-shot AEG is when we train a system to grade essays written to a new prompt which was not present in our training data. In this paper, we describe a solution to the problem of zero-shot automatic essay grading, using cognitive information, in the form of gaze behaviour. Our experiments show that using gaze behaviour helps in improving the performance of AEG systems, especially when we provide a new essay written in response to a new prompt for scoring, by an average of almost 5 percentage points of QWK.
Acronyms are abbreviated units of a phrase constructed by using initial components of the phrase in a text. Automatic extraction of acronyms from a text can help various Natural Language Processing tasks like machine translation, information retrieval, and text summarisation. This paper discusses an ensemble approach for the task of Acronym Extraction, which utilises two different methods to extract acronyms and their corresponding long forms. The first method utilises a multilingual contextual language model and fine-tunes the model to perform the task. The second method relies on a convolutional neural network architecture to extract acronyms and append them to the output of the previous method. We also augment the official training dataset with additional training samples extracted from several open-access journals to help improve the task performance. Our dataset analysis also highlights the noise within the current task dataset. Our approach achieves the following macro-F1 scores on test data released with the task: Danish (0.74), English-Legal (0.72), English-Scientific (0.73), French (0.63), Persian (0.57), Spanish (0.65), Vietnamese (0.65). We release our code and models publicly.
Establishing language relatedness by inferring phylogenetic trees has been a topic of interest in the area of diachronic linguistics. However, existing methods face meaning conflation deficiency due to the usage of lexical similarity-based measures. In this paper, we utilize fourteen linked Indian Wordnets to create inter-language distances using our novel approach to compute 'language distances'. Our pilot study uses deep cross-lingual word embeddings to compute inter-language distances and provide an effective distance matrix to infer phylogenetic trees. We also develop a baseline method using lexical similarity-based metrics for comparison and identify that our approach produces better phylogenetic trees which club related languages closer when compared to the baseline approach.
Fake news, misinformation, and unverifiable facts on social media platforms propagate disharmony and affect society, especially when dealing with an epidemic like COVID-19. The task of Fake News Detection aims to tackle the effects of such misinformation by classifying news items as fake or real. In this paper, we propose a novel approach that improves over the current automatic fake news detection approaches by automatically gathering evidence for each claim. Our approach extracts supporting evidence from the web articles and then selects appropriate text to be treated as evidence sets. We use a pre-trained summarizer on these evidence sets and then use the extracted summary as supporting evidence to aid the classification task. Our experiments, using both machine learning and deep learning-based methods, help perform an extensive evaluation of our approach. The results show that our approach outperforms the state-of-the-art methods in fake news detection to achieve an F1-score of 99.25 over the dataset provided for the CONSTRAINT-2021 Shared Task. We also release the augmented dataset, our code and models for any further research.
Cross-domain sentiment analysis (CDSA) helps to address the problem of data scarcity in scenarios where labelled data for a domain (known as the target domain) is unavailable or insufficient. However, the decision to choose a domain (known as the source domain) to leverage from is, at best, intuitive. In this paper, we investigate text similarity metrics to facilitate source domain selection for CDSA. We report results on 20 domains (all possible pairs) using 11 similarity metrics. Specifically, we compare CDSA performance with these metrics for different domain-pairs to enable the selection of a suitable source domain, given a target domain. These metrics include two novel metrics for evaluating domain adaptability to help source domain selection of labelled data and utilize word and sentence-based embeddings as metrics for unlabelled data. The goal of our experiments is a recommendation chart that gives the K best source domains for CDSA for a given target domain. We show that the best K source domains returned by our similarity metrics have a precision of over 50%, for varying values of K.
Named Entity Recognition (NER) is a foundational NLP task that aims to provide class labels like Person, Location, Organisation, Time, and Number to words in free text. Named Entities can also be multi-word expressions where the additional I-O-B annotation information helps label them during the NER annotation process. While English and European languages have considerable annotated data for the NER task, Indian languages lack on that front- both in terms of quantity and following annotation standards. This paper releases a significantly sized standard-abiding Hindi NER dataset containing 109,146 sentences and 2,220,856 tokens, annotated with 11 tags. We discuss the dataset statistics in all their essential detail and provide an in-depth analysis of the NER tag-set used with our data. The statistics of tag-set in our dataset show a healthy per-tag distribution, especially for prominent classes like Person, Location and Organisation. Since the proof of resource-effectiveness is in building models with the resource and testing the model on benchmark data and against the leader-board entries in shared tasks, we do the same with the aforesaid data. We use different language models to perform the sequence labelling task for NER and show the efficacy of our data by performing a comparative evaluation with models trained on another dataset available for the Hindi NER task. Our dataset helps achieve a weighted F1 score of 88.78 with all the tags and 92.22 when we collapse the tag-set, as discussed in the paper. To the best of our knowledge, no available dataset meets the standards of volume (amount) and variability (diversity), as far as Hindi NER is concerned. We fill this gap through this work, which we hope will significantly help NLP for Hindi. We release this dataset with our code and models for further research.
Automatic Cognate Detection helps NLP tasks of Machine Translation, Information Retrieval, and Phylogenetics. Cognate words are defined as word pairs across languages which exhibit partial or full lexical similarity and mean the same (e.g., hund-hound in German-English). In this paper, we use a Siamese Feed-forward neural network with word-embeddings to detect such word pairs. Our experiments with various embedding dimensions show larger embedding dimensions can only be used for large corpora sizes for this task. On a dataset built using linked Indian Wordnets, our approach beats the baseline approach with a significant margin (up to 71%) with the best F-score of 0.85% on the Hindi-Gujarati language pair.
Sentiment analysis has benefited from the availability of lexicons and benchmark datasets created over decades of research. However, its applications to the real world are a driving force for research in SA. This chapter describes some of these applications and related challenges in real-life scenarios. In this chapter, we focus on five applications of SA: health, social policy, e-commerce, digital humanities and other areas of NLP. This chapter is intended to equip an NLP researcher with the `what', `why' and `how' of applications of SA: what is the application about, why it is important and challenging and how current research in SA deals with the application. We note that, while the use of deep learning techniques is a popular paradigm that spans these applications, challenges around privacy and selection bias of datasets is a recurring theme across several applications.
Evaluation of machine translation (MT) is vital to determine the effectiveness of MT systems. This paper investigates quality estimation (QE) for machine translation (MT) for low-resource Indic languages. We analyse the influence of language relatedness within linguistic families and integrate various pre-trained encoders within the MonoTransQuest (MonoTQ) framework. This entails assessing models in single-language configurations before scaling up to multiple-language setups, focusing on languages within and across families, and using approaches grounded in transfer learning. Experimental outcomes and analyses indicate that language-relatedness significantly improves QE performance over baseline, sometimes even surpassing state-of-the-art approaches. Across monolingual and multilingual configurations, we discuss strategic encoder usage as a simple measure to exploit the language interactions within these models improving baseline QE efficiency for quality estimation. This investigation underscores the potential of tailored pre-trained encoders to improve QE performance and discusses the limitations of QE approaches for low-resource scenarios.
Additional publications
Significant Publications:-
Kanojia, D., Sharma, P., Ghodekar, S., Bhattacharyya, P., Haffari, G., & Kulkarni, M. (2021). Cognition-aware Cognate Detection. Proceedings of the 16th Conference of the European Chapter of the Association for Computational Linguistics (EACL). EACL 2021. [Best Paper Honourable Mention]
Kanojia, D., Dabre, R., Dewangan, S., Bhattacharyya, P., Haffari, G., & Kulkarni, M. (2020). Harnessing Cross-lingual Features to Improve Cognate Detection for Low-resource Languages. Proceedings of The 28th International Conference on Computational Linguistics (COLING). COLING 2020.
Mathias, S., Kanojia, D., Mishra, A., & Bhattacharyya, P. (2020). A survey on using gaze behaviour for natural language processing. IJCAI International Joint Conference on Artificial Intelligence, 2021-January, 4907–4913. https://doi.org/10.24963/ijcai.2020/683
Mathias, S., Murthy, R., Kanojia, D., Mishra, A., & Bhattacharyya, P. (2020). Happy Are Those Who Grade without Seeing: A Multi-Task Learning Approach to Grade Essays Using Gaze Behaviour. Proceedings of the 1st Conference of the Asia-Pacific Chapter of the Association for Computational Linguistics and the 10th International Joint Conference on Natural Language Processing (AACL-IJCNLP). AACL-IJCNLP 2020.
Kanojia, D., Kulkarni, M., Bhattacharyya, P., & Haffari, G. (2020). Challenge Dataset of Cognates and False Friend Pairs from Indian Languages. Proceedings of The 12th Language Resources and Evaluation Conference, 3096–3102.
Mathias, S., Murthy, R., Kanojia, D., & Bhattacharyya, P. (2021). Cognitively Aided Zero-Shot Automatic Essay Grading. Proceedings of the 17th International Conference on Natural Language Processing (ICON). ICON 2020.
Kumar, S., Kumar, S., Kanojia, D., & Bhattacharyya, P. (2020). "A Passage to India": Pre-trained Word Embeddings for {I}ndian Languages. Proceedings of the 1st Joint Workshop on Spoken Language Technologies for Under-Resourced Languages (SLTU) and Collaboration and Computing for Under-Resourced Languages (CCURL), 352–357. https://www.aclweb.org/anthology/2020.sltu-1.49
Kanojia, D., Dubey, A., Kulkarni, M., Bhattacharyya, P., & Haffari, G. (2019). Utilizing Word Embeddings based Features for Phylogenetic Tree Generation of Sanskrit Texts. Proceedings of the 6th International Sanskrit Computational Linguistics Symposium, 152–165.
Mathias, S., Kanojia, D., Patel, K., Agrawal, S., Mishra, A., & Bhattacharyya, P. (2018). Eyes are the Windows to the Soul: Predicting the Rating of Text Quality Using Gaze Behaviour. Proceedings of the 56th Annual Meeting of the Association for Computational Linguistics (Volume 1: Long Papers), 2352–2362. ACL 2018.
Mishra, A., Kanojia, D., Nagar, S., Dey, K., & Bhattacharyya, P. (2017). Scanpath Complexity: Modeling Reading Effort Using Gaze Information. Proceedings of the 31st Annual AAAI Conference on Artificial Intelligence, 4429–4436. AAAI 2017.
Mishra, A., Kanojia, D., Nagar, S., Dey, K., & Bhattacharyya, P. (2016, August). Harnessing Cognitive Features for Sarcasm Detection. In Proceedings of the 54th Annual Meeting of the Association for Computational Linguistics (Volume 1: Long Papers) (pp. 1095-1104). ACL 2016.
Mishra, A., Kanojia, D., Nagar, S., Dey, K., & Bhattacharyya, P. (2016, August). Leveraging cognitive features for sentiment analysis. Proceedings of The SIGNLL Conference on Computational Natural Language Learning (CoNLL). CoNLL 2016.
Mishra, A., Kanojia, D., & Bhattacharyya, P. (2016). Predicting Readers’ Sarcasm Understandability by Modeling Gaze Behavior. Proceedings of the 30th Annual AAAI Conference on Artificial Intelligence, 3747–3753. AAAI 2016.
Joshi, S., Kanojia, D., & Bhattacharyya, P. (2013). More than meets the eye: Study of Human Cognition in Sense Annotation. Proceedings of the 2013 Conference of the North American Chapter of the Association for Computational Linguistics: Human Language Technologies, 733–738.