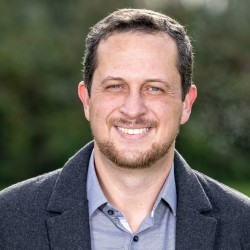
Dr Erick Giovani Sperandio Nascimento
About
Biography
Dr Erick Sperandio is Associate Professor (Reader) in Artificial Intelligence (AI) for Clean Air Systems at the Surrey Institute for People-Centred AI, member of the Global Centre for Clean Air Research (GCARE) and Sustainability Fellow at the Institute for Sustainability, both at the University of Surrey, United Kingdom. He is also Associate Professor at SENAI CIMATEC, Bahia, Brazil. Currently, he is the Programme Lead of AI and Sustainability, and has been teaching the Natural Language Processing, Practical Business Analytics and the forthcoming AI and Sustainability modules.
He holds a PhD in Environmental Engineering in the area of Atmospheric Computational Modeling, a Master in Informatics in the field of Computational Intelligence and Graduated in Computer Science from UFES. He currently coordinates, leads and participates in R&D projects in the areas of AI, computational modeling (CM) and supercomputing applied to different areas such as Sustainability, Atmospheric Sciences, Renewable Energies, Oil and Gas, Health, Advanced Manufacturing, Quantum Machine Learning, among others, supervising undergraduate and post-graduate students, also teaching and training students from the academia and industry to research and apply AI, CM and HPC to real-world problems.
He serves as one of the Principal Investigators at the Brazilian National Applied Research Center in Artificial Intelligence (CPA-IA) of SENAI CIMATEC, focusing on Industry, being one of the six CPA-IA in Brazil approved by the Brazilian Ministry of Science, Technology and Innovation (MCTI), São Paulo Research Foundation (FAPESP) and Brazilian Internet Steering Committee (CGI.br).
He is a Certified Instructor and University Ambassador of the NVIDIA Deep Learning Institute (DLI) in the areas of Deep Learning, Computer Vision, Natural Language Processing and Recommender Systems, and Principal Investigator of the NVIDIA/CIMATEC AI Joint Lab, the first in the whole American continent within the NVIDIA AI Technology Center (NVAITC) worldwide program.
Prior to his new position at Surrey, he acted as the Lead Researcher and co-founder of the SENAI CIMATEC Reference Center on Artificial Intelligence. He also was a member and vice-coordinator of the Basic Board of Scientific-Technological Advice and Evaluation, in the area of Innovation, of the Bahia Research Foundation (FAPESB), Brazil. He participated as one of the representatives of Brazil in the BRICS Innovation Collaboration Working Group on HPC, ICT and AI. He was the coordinator of the Work Group of the Axis 5 - Workforce and Training - of the Brazilian Strategy for Artificial Intelligence (EBIA), and member of the MCTI/EMBRAPII AI Innovation Network Training Committee, and was leading the working group of experts representing Brazil in the Global Partnership on Artificial Intelligence (GPAI), on the theme "AI and the Pandemic Response". He contributed to the creation, development and execution of undergraduate and post-graduate courses for teaching and training people in the fields of AI and data science.
Prior to his academic work, he was founder and CEO of a software company, where he developed multi-device software applications for areas like environmental data management, weather and air pollution forecasting, impact of rocket exhaust emissions on air quality, RFID access control, vehicle insurance management and control, among others, with funding from private and public sectors.
His research and innovation interests and activities are focused at developing, applying and teaching AI, CM and HPC techniques and approaches that are strongly linked to the domain areas of Clean Air Systems and their impact in the human life, such as:
- Sustainability
- Air pollution
- Renewable energies
- Weather forecasting
- Climate change
- Health
- Smart cities
- IoT
There are also other domain areas in AI in which he has been working that are of great interest of the academia, industry and society, such as:
- Fundamentals of AI and Deep Learning
- Computer vision
- Predictive and prescriptive maintenance
- Natural language processing
- Recommender systems
- Quantum machine learning
- Foundation models
- Bias and unfairness identification and mitigation in machine learning models
- Model interpretability and explainability
- Physics-informed neural networks
- Graph neural networks
The results of his research have been published in high-impact international peer-reviewed journals and conferences, and his teaching and training activities have helped students and professionals from the academia and industry to be more prepared and engaged in solving real-world problems using AI and data science.
You can follow him at:
Research Opportunities
We have a great fully-funded PhD opportunity on "Sustainable Foundation Models for Time Series Processing Applied to Sustainability". Deadline: 30/04/2024.
Publications
This article aims to calculate the COVID-19 Pandemic Vulnerability Index (COVID-19-PVI) across Brazilian municipalities, positing that vulnerability to the coronavirus is linked to socioeconomic disparities in this continental-sized country. From data collection on epidemiological, socioeconomic, demographic, and public health systems, it was possible to rank which features were most influential in the spread of COVID-19 using the artificial intelligence implicit in the boosting tree regression method. To ensure the robustness of the findings, this index is tested in Pearson correlations leading to conclusions about which regions were most vulnerable to the pandemic and its consequences, the importance of the spatial distribution of General Hospitals during the COVID-19 outbreak, and the influence of population density on the advancement of the coronavirus in the country.
The high demand for generation and development in wind electrical power competitiveness has gained significant popularity in wind energy and speed forecasting models. It is also an essential method for planning the wind power plant system. Several models were created in the past to forest the speed and energy of the Wind. However, results have very low prediction accuracy due to their nonlinear and irregular characteristics. Therefore, a novel Modular Red Deer Neural System (MRDNS) was developed in this research to forecast wind speed and energy effectively. Primarily, the system accepted the data from the wind turbine SCADA database and preprocessed it to remove the training flaws. Further, the relevant features are extracted. The complexity of the prediction process was reduced by processing the relevant features. By analysing these features, the wind speed and energy were predicted in accordance with the fitness function of the MRDNS. The model obtained higher prediction accuracy. The recommended strategy was checked in the Python platform and the robustness metrics including RMSE, MSE, and precision were computed. The model scored 99.99% prediction accuracy; also gained 0.0017 MSE value, 0.0422 RMSE value for wind power forecasting and 0.0003 MSE, 0.0174 RMSE for wind speed forecasting.
This chapter aims to present a classification model for categorizing textual clinical records of breast magnetic resonance imaging, based on lexical, syntactic and semantic analysis of clinical reports according to the Breast Imaging-Reporting and Data System (BI-RADS) classification, using Deep Learning and Natural Language Processing (NLP). The model was developed from transfer learning based on the pre-trained BERTimbau model, BERT model (Bidirectional Encoder Representations from Transformers) trained in Brazilian Portuguese. The dataset is composed of medical reports in Brazilian Portuguese classified into six categories: Inconclusive; Normal or Negative; Certainly Benign Findings; Probably Benign Findings; Suspicious Findings; High Risk of Cancer; Previously Known Malignant Injury. The following models were implemented and compared: Random Forest, SVM, Naïve Bayes, BERTimbau with and without finetuning. The BERTimbau model presented better results, with better performance after finetuning.
The objective of this work is to analyze the scaling behavior of wind speed in the region of the state of Bahia, northeastern Brazil, in search of long-range correlations and other information about the crossover phenomena. Thus, data from 41 automatic surface stations were used for a period of five years—between 2015 and 2020—for onshore reading. For offshore readings, data from a surface station located in the Abrolhos Archipelago were used. To achieve this goal, the DFA (detrended fluctuation analysis) technique was used in the analysis of measured data at the stations, along with numerical simulations using the WRF (weather research and forecasting) mesoscale model. The results of the analysis of hourly average wind speed from the measured and simulated data show the existence of scale behavior with the appearance, in most cases, of a double crossover—onshore and offshore. This suggests the phenomenon's dependence on the time period of the analyzed data, and also on the geographic location, showing a strong correlation with the Atlantic and Pacific oscillations (La Niña and El Niño), indicating the influence of local, mesoscale, and macroscale effects in the region of study. For the offshore case, the measured data and simulations presented a subdiffusive behavior (α≥1) before the first crossover, and persistence (0.5
Wind energy has achieved a leading position among renewable energies. The global installed capacity in 2022 was 906 GW of power, with a growth of 8.4% compared to the same period in the previous year. The forecast is that the barrier of 1,000,000 MW of installed wind capacity in the world will be exceeded in July 2023, according to data from the World Association of Wind Energy. In order to support the expected growth in the wind sector, maintenance strategies for wind turbines must provide the reliability and availability necessary to achieve these goals. The usual maintenance procedures may present difficulties in keeping up with the expansion of this energy source. The objective of this work was to carry out a systematic review of the literature focused on research on the predictive and prescriptive maintenance of wind turbines based on the implementation of data-oriented models with the use of artificial intelligence tools. Deep machine learning models involving the detection, diagnosis, and prognosis of failures in this equipment were addressed.
In recent years there has been an increase in wind generation, driven by environmental factors and the incentive offered for the development of clean and sustainable technologies for energy generation. However, due to the rapid growth of this technology, concerns about the safety and reliability of wind turbines are increasing, especially due to the associated risks and financial costs. Therefore, health monitoring and fault detection for wind turbines has become an important research focus. Thus, the objective of this work was to realize an exploratory study of real data from a wind turbine, using AI tools that help to group the different behaviors, according to the similarity of resources and characteristics of the data. For this, unsupervised learning methods were used to cluster the data and a model was proposed to train and test, using a multilayer perceptron network, to classify these clusters. The differential of this work is the use of real data from CHESF's wind turbines. Another important contribution is in relation to permanent magnet wind turbines, as there are not many studies in this field, therefore a great potential to be explored.
Greenhouse gas (GHG) emissions, especially CO2, represent a global concern. Among those responsible for CO2 emissions, buildings stand out due to the consumption of energy from fossil fuels. In this sense, initiatives for the decarbonization of buildings and construction tends to contribute to the achievement of the target defined in the Paris Agreement of limiting the increase in global temperature to 1.5 degrees Celsius above pre-industrial levels, as well as in achieving the Sustainable Development Goals (SDG) and of the Triple Bottom Line (TBL). This article aimed to identify renewable energy generation technologies that can be applied in urban vertical constructions, contributing to the reduction of carbon emissions in the atmosphere. To this end, the following methodology was adopted: a survey of the Conferences of the Parties on climate change; identification of European Union Legislative Directives for the decarbonization of buildings; and a literature review to identify research that deals with renewable energy generation technologies that can be adopted in buildings. The results indicated that there seems to be a correlation between the growth in the number of articles that deal with the topic of decarbonizing buildings and the increase in world concerns about global warming. A hybrid microgrid proposal, combining different sources of renewable energy such as solar photovoltaic, wind, biomass, micro-hydroelectric, and others for vertical buildings with more than five floors, is presented as viable to achieve zero emissions in these buildings, contributing to future research, that can carry out quantitative analyses and feasibility studies, as well as for experiments and applications in existing buildings and the projects of new vertical constructions.
This study estimates exposure and inhaled dose to air pollutants of children residing in a tropical coastal-urban area in Southeast Brazil. For that, twenty-one children filled their time-activities diaries and wore the passive samplers to monitor NO2. The personal exposure was also estimated using data provided by the combination of WRF-Urban/GEOS-Chem/CMAQ models, and the nearby monitoring station. Indoor/outdoor ratios were used to consider the amount of time spent indoors by children in homes and schools. The model's performance was assessed by comparing the modelled data with concentrations measured by urban monitoring stations. A sensitivity analyses was also performed to evaluate the impact of the model's height on the air pollutant concentrations. The results showed that the mean children's personal exposure to NO2 predicted by the model (22.3 μg/m3) was nearly twice to those measured by the passive samplers (12.3 μg/m3). In contrast, the nearest urban monitoring station did not represent the personal exposure to NO2 (9.3 μg/m3), suggesting a bias in the quantification of previous epidemiological studies. The building effect parameterisation (BEP) together with the lowering of the model height enhanced the air pollutant concentrations and the exposure of children to air pollutants. With the use of the CMAQ model, exposure to O3, PM10, PM2.5, and PM1 was also estimated and revealed that the daily children's personal exposure was 13.4, 38.9, 32.9, and 9.6 μg/m3, respectively. Meanwhile, the potential inhalation daily dose was 570-667 μg for PM2.5, 684-789 μg for PM10, and 163-194 μg for PM1, showing to be favourable to cause adverse health effects. The exposure of children to air pollutants estimated by the numerical model in this work was comparable to other studies found in the literature, showing one of the advantages of using the modelling approach since some air pollutants are poorly spatially represented and/or are not routinely monitored by environmental agencies in many regions.
This work presents a novel transformer-based deep neural network architecture integrated with wavelet transform for forecasting wind speed and wind energy (power) generation for the next 6 h ahead, using multiple meteorological variables as input for multivariate time series forecasting. To evaluate the performance of the proposed model, different case studies were investigated, using data collected from anemometers installed in three different regions in Bahia, Brazil. The performance of the proposed transformer-based model with wavelet transform was compared with an LSTM (Long Short Term Memory) model as a baseline, since it has been successfully used for time series processing in deep learning, as well as with previous state-of-the-art (SOTA) similar works. Results of the forecasting performance were evaluated using statistical metrics, along with the time for training and performing inferences, both using quantitative and qualitative analysis. They showed that the proposed method is effective for forecasting wind speed and power generation, with superior performance than the baseline model and comparable performance to previous similar SOTA works, presenting potential suitability for being extended for the general purpose of multivariate time series forecasting. Furthermore, results demonstrated that the integration of the transformer model with wavelet decomposition improved the forecast accuracy. Highlights:• A new transformer-based model for multivariate forecasting of wind speed and energy.•Integration with wavelet transform for feature augmentation to improve predictions.• Full assessment of the novel model comparing with LSTM, persistence and SOTA works.• Lower time to train than the fine-tuned LSTM, being more energy-efficient.• Evidenced superiority over LSTM and performance comparable to similar SOTA works.
This study aims to explain the role of local emission sources to PM2.5 mass concentration in a tropical coastal-urban area, highly influenced by industrial and urban emissions, located in the Southeast of Brazil. The Integrated Source Apportionment Method (ISAM) tool was coupled with the chemistry and transport Community Multiscale Air Quality (CMAQ) model (CMAQ-ISAM) to quantify the contribution of ten emission sectors of PM2.5. The simulations were performed over five months between spring 2019 and summer 2020 using a local inventory, which was processed by the Sparse Matrix Operator Kernel Emission (SMOKE). The meteorological fields were provided by the Weather Research and Forecasting (WRF-Urban) model. The boundary and initial conditions to the CMAQ-ISAM were performed by the GEOS-Chem model. The simulations results show that the road dust resuspension (36%) and point (17%) emissions sources were the major contributors to PM2.5 mass in the Metropolitan Region of Vitória (MRV). The boundary conditions (BCON), representing the transport contribution from sources outside the domain, were also a dominant contributor in the MRV (20% on average). Furthermore, the primary atmospheric pollutants emitted by the point (14%) and shipping (7%) sectors in the MRV also affected the cities located in the south region of the domain, strengthened by the wind fields that mostly come from the northeast direction.
The pandemic of the new coronavirus affected people’s lives by an unprecedented scale. Due to the need for isolation and the treatments, drugs, and vaccines, the pandemic amplified the digital health technologies, such as Artificial Intelligence (AI), Big Data Analytics (BDA), Blockchain, Telecommunication Technology (TT) as well as High-Performance Computing (HPC) and other technologies, to historic levels. These technologies are being used to mitigate, facilitate pandemic strategies, and find treatments and vaccines. This paper aims to reach articles about new technologies applied to COVID-19 published in the main database (PubMed/Medline, Elsevier Science Direct, Scopus, Isi Web of Science, Embase, Excerpta Medica, UptoDate, Lilacs, Novel Coronavirus Resource Directory from Elsevier), in the high-impact international scientific Journals (Scimago Journal and Country Rank - SJR - and Journal Citation Reports - JCR), such as The Lancet, Science, Nature, The New England Journal of Medicine, Physiological Reviews, Journal of the American Medical Association, Plos One, Journal of Clinical Investigation, and in the data from Center for Disease Control (CDC), National Institutes of Health (NIH), National Institute of Allergy and Infectious Diseases (NIAID) and World Health Organization (WHO). We prior selected meta-analysis, systematic reviews, article reviews, and original articles in this order. We reviewed 252 articles and used 140 from March to June 2020, using the terms coronavirus, SARS-CoV-2, novel coronavirus, Wuhan coronavirus, severe acute respiratory syndrome, 2019-nCoV, 2019 novel coronavirus, n-CoV-2, covid, n-SARS-2, COVID-19, corona virus, coronaviruses, New Technologies, Artificial Intelligence, Telemedicine, Telecommunication Technologies, AI, Big Data, BDA, TT, High-Performance Computing, Deep Learning, Neural Network, Blockchain, with the tools MeSH (Medical Subject Headings), AND, OR, and the characters [,“,; /., to ensure the best review topics. We concluded that this pandemic lastly consolidates the new technologies era and will change the whole way of the social life of human beings. Also, a big jump in medicine will happen on procedures, protocols, drug designs, attendances, encompassing all health areas, as well as in social and business behaviors.
The current scenario of a global pandemic caused by the virus SARS-CoV-2 (COVID19), highlights the importance of water studies in sewage systems. In Brazil, about 35 million Brazilians still do not have treated water and more than 100 million do not have basic sanitation. These people, already exposed to a range of diseases, are among the most vulnerable to COVID-19. According to studies, places that have poor sanitation allow the proliferation of the coronavirus, been observed a greater number of infected people being found in these regions. This social problem is strongly related to the lack of effective management of water resources, since they are the sources for the population's water supply and the recipients of effluents stemming from sanitation services (household effluents, urban drainage and solid waste). In this context, studies are needed to develop technologies and methodologies to improve the management of water resources. The application of tools such as artificial intelligence and hydrometeorological models are emerging as a promising alternative to meet the world's needs in water resources planning, assessment of environmental impacts on a region's hydrology, risk prediction and mitigation. The main model of this type, WRF-Hydro Weather Research and Forecasting Model), represents the state of the art regarding water resources, as well as being the object of study of small and medium-sized river basins that tend to have less water availability. hydrometeorological data and analysis. Thus, this article aims to analyze the feasibility of a web tool for greater software usability and computational cost use, making it possible to use the WRF-Hydro model integrated with Artificial Intelligence tools for short and medium term, optimizing the time of simulations with reduced computational cost, so that it is able to monitor and generate a predictive analysis of water bodies in the MATOPIBA region (Maranhão-Tocantins-Piauí-Bahia), constituting an instrument for water resources management. The results obtained show that the WRF-Hydro model proves to be an efficient computational tool in hydrometeorological simulation, with great potential for operational, research and technological development purposes, being considered viable to implement the web tool for analysis and management of water resources and consequently, assist in monitoring and mitigating the number of cases related to the current COVID-19 pandemic. This research are in development and represents a preliminary results with future perspectives.
An aborted rocket launch may occur because of explosions during pre-launch operations or launch performance, which generates a huge cloud near ground level comprising hot buoyant exhaust products. This action occur within a few minutes, and populated areas near the launch centre may be exposed to high levels of hazardous pollutant concentrations within a short time scale — from minutes to a couple of hours. Although aborted rocket launch events do not occur frequently, the occurrence rate has increased in the past few years, making it mandatory to perform short and long-range assessments to evaluate the impact of such operations on the air quality of a region. In this work, we use a modern approach based on the Model for Simulating the Rocket Exhaust Dispersion (MSRED) and its modelling system to report the simulated impact of a hydrogen chloride (HCl) exhaust cloud, formed during a hypothetical aborted rocket launch, on the atmosphere near the earth’s surface at the Alcantara Launch Center, Brazil’s space-port. The results show that when a launch occurs under stable atmospheric conditions, the HCl concentrations near the ground can reach levels that are extremely hazardous to human health.
The conventional sources of energy such as oil, natural gas, coal, or nuclear are finite and generate environmental pollution. Alternatively, renewable energy source like wind is clean and abundantly available in nature. Wind power has a huge potential of becoming a major source of renewable energy for this modern world. It is a clean, emission-free power generation technology. Wind energy has been experiencing very rapid growth in Brazil and in Uruguay; therefore, it’s a promising industry in these countries. Thus, this rapid expansion can bring several regional benefits and contribute to sustainable development, especially in places with low economic development. Therefore, the scope of this chapter is to estimate short-term wind speed forecasting applying computational intelligence, by recurrent neural networks (RNN), using anemometers data collected by an anemometric tower at a height of 100.0 m in Brazil (tropical region) and 101.8 m in Uruguay (subtropical region), both Latin American countries. The results of this study are compared with wind speed prediction results from the literature. In one of the cases investigated, this study proved to be more appropriate when analyzing evaluation metrics (error and regression) of the prediction results obtained by the proposed model.
The development of artificial intelligence (AI) algorithms for classification purpose of undesirable events has gained notoriety in the industrial world. Nevertheless, for AI algorithm training is necessary to have labeled data to identify the normal and anomalous operating conditions of the system. However, labeled data is scarce or nonexistent, as it requires a herculean effort to the specialists of labeling them. Thus, this chapter provides a comparison performance of six unsupervised Machine Learning (ML) algorithms to pattern recognition in multivariate time series data. The algorithms can identify patterns to assist in semiautomatic way the data annotating process for, subsequentially, leverage the training of AI supervised models. To verify the performance of the unsupervised ML algorithms to detect interest/anomaly pattern in real time series data, six algorithms were applied in following two identical cases (i) meteorological data from a hurricane season and (ii) monitoring data from dynamic machinery for predictive maintenance purposes. The performance evaluation was investigated with seven threshold indicators: accuracy, precision, recall, specificity, F1-Score, AUC-ROC and AUC-PRC. The results suggest that algorithms with multivariate approach can be successfully applied in the detection of anomalies in multivariate time series data.
Air quality improvement is directly associated with the Sustainable Development Goals (SDGs), established by the United Nations in 2015. To reduce potential impacts from air pollution, computational intelligence by supervised machine learning, using different artificial neural networks (ANNs) techniques, shows to be a promising tool. To enhance their abilities to predict air quality, ANNs have been combined with data preprocessing. The present work performs short-term forecasting of hourly ground-level ozone using long short-term memory (LSTM), a type of recurrent neural network, with the discrete wavelet transform. The study was performed using data from a tropical coastal-urban site in Southeast Brazil, highly influenced by intense convective weather with complex terrain. The models’ performance was carried out by comparing statistical indices of errors and agreement, namely: mean squared error (MSE), normalized mean squared error (NMSE), mean absolute error (MAE), Pearson’s r, R2 and mean absolute percentage error (MAPE). When comparing the statistical metrics values, it is shown that the combination of artificial neural networks with wavelet transform enhanced the model’s ability to forecast ozone levels compared to the baseline model, which did not use wavelets.
The Brazilian legal system postulates the expeditious resolution of judicial proceedings. However, legal courts are working under budgetary constraints and with reduced staff. As a way to face these restrictions, artificial intelligence (AI) has been tackling many complex problems in natural language processing (NLP). This work aims to detect the degree of similarity between judicial documents that can be achieved in the inference group using unsupervised learning, by applying three NLP techniques, namely term frequency-inverse document frequency (TF-IDF), Word2Vec CBoW, and Word2Vec Skip-gram, the last two being specialized with a Brazilian language corpus. We developed a template for grouping lawsuits, which is calculated based on the cosine distance between the elements of the group to its centroid. The Ordinary Appeal was chosen as a reference file since it triggers legal proceedings to follow to the higher court and because of the existence of a relevant contingent of lawsuits awaiting judgment. After the data-processing steps, documents had their content transformed into a vector representation, using the three NLP techniques. We notice that specialized word-embedding models—like Word2Vec—present better performance, making it possible to advance in the current state of the art in the area of NLP applied to the legal sector.
Este trabalho mostra a existência de correlações de longo alcance das séries históricas e temporais de velocidade do vento e radiação solar na cidade de Salvador (Bahia) provenientes de dados medidos em estações meteorológicas, além de simulações com o modelo de mesoescala WRF (Weather Research and Forecasting), através do método DFA (Detrended Fluctuation Analysis). Resultados preliminares indicam que as séries de dados locais são caracterizadas com persistência na velocidade do vento e radiação solar de forma satisfatória para a geração de energia, o que indica viabilidade da participação destas respectivas matrizes na matriz energética local. This work shows the positive correlation between the historical and temporal series of velocity and solar energy in the city of Salvador (Bahia). Research and Forecasting) through the DFA (Detrended Fluctuation Analysis) method. Preliminary results indicate that the data series are characterized with persistence in speed and solar energy in a satisfactory way for an energy generation, which indicates the feasibility of the learning of matrices of matrices in the local energy
Considering the fact that the exposure to polluted air has been associated with adverse health effects, it is important to look into the air pollution in urban areas. To evaluate the impact of emissions on the air quality in the Metropolitan Region of Salvador (MRS) in the Northeast region of Brazil, simulations using the Weather Research and Forecasting (WRF) and the Community Multiscale Air Quality (CMAQ) models were applied. The region’s choice was due to the fact that, although Salvador is the 3rd most populated city in Brazil and its metropolitan area is the 7th most populated one, there is a lack of scientific studies about regional air quality and air pollution dispersion, especially in terms of photochemical regional impact assessment of pollutants in this urban area. The aim of this work was to assess the impact of atmospheric pollutants (NOx and SO2) over the MRS from stacks held in a petrochemical complex that lies within this metropolitan site. The emissions rates were based on another study since there is no official emissions inventory available for the region. Moreover, as there were no pollutant measurement data to be compared, a qualitative analysis was conducted. The results showed the importance of the application of the state of the art in the computational atmospheric modeling field in order to assess the air quality of the MRS.
This work proposes the use of a fine-tuned Transformers-based Natural Language Processing (NLP) model called BERTimbau to generate the word embeddings from texts published in a Brazilian newspaper, to create a robust NLP model to classify news in Portuguese, a task that is costly for humans to perform for big amounts of data. To assess this approach, besides the generation of embeddings by the fine-tuned BERTimbau, a comparative analysis was conducted using the Word2Vec technique. The first step of the work was to rearrange news from nineteen to ten categories to reduce the existence of class imbalance in the corpus, using the K-means and TF-IDF techniques. In the Word2Vec step, the CBOW and Skip-gram architectures were applied. In BERTimbau and Word2Vec steps, the Doc2Vec method was used to represent each news as a unique embedding, generating a document embedding for each news. Metrics accuracy, weighted accuracy, precision, recall, F1-Score, AUC ROC and AUC PRC were applied to evaluate the results. It was noticed that the fine-tuned BERTimbau captured distinctions in the texts of the different categories, showing that the classification model based on this model has a superior performance than the other explored techniques.
This study details an evaluation of the onshore and offshore wind speed field in the state of Bahia, northeastern Brazil, using the WRF (Weather Research and Forecasting) mesoscale model, version 4.0. The simulations were run for a period of five years—between 2015 and 2020—with a horizontal resolution of 3 km, and were compared with data from 41 automatic surface stations for the onshore case. For the offshore case, data from a surface station located in the Abrolhos Archipelago were used. The winter period presents higher values of wind speed for the onshore region (9–14 m/s), and the northern and southwestern regions of the state stand out for the generation of wind energy. In the offshore case, spring presents the highest averages for wind speed (7–8 m/s), followed by the summer season, highlighting the maritime coast in the extreme south of the state (7–10 m/s). Furthermore, the nocturnal wind regime is more intense than the daytime one, indicating a great complementarity with solar energy. The year 2017 had the highest average values of wind speed in the region, being considered one of the warmest years without the influence of the El Nino phenomenon recorded globally since 1850. The hourly averages of onshore and offshore winds for the state of Bahia demonstrated the great wind potential of the region, with high and medium speeds at altitude, which were in accordance with the minimum attractiveness thresholds for investments in wind energy generation.
Application of deep-learning techniques has been increasing, which redefines state-of-the-art technology, especially in industrial applications such as fault diagnosis and classification. Therefore, implementing a system that can automatically detect faults at an early stage and recommend stopping of a machine to avoid unsafe conditions in the process and environment has become possible. This paper proposes the use of Predictive Maintenance model with Convolutional Neural Network (PdM-CNN), to classify automatically rotating equipment faults and advise when maintenance actions should be taken. This work uses data from only one vibration sensor installed on the motor-drive end bearing, which is the most common layout present in the industry. This work was developed under controlled ambient varying rotational speeds, load levels and severities, in order to verify whether it is possible to build a model capable of classifying such faults in rotating machinery using only one set of vibration sensors. The results showed that the accuracies of the PdM-CNN model were of 99.58% and 97.3%, when applied to two different publicly available databases. This demonstrates the model's ability to accurately detect and classify faults in industrial rotating machinery. With this model companies can improve the financial performance of their rotating machine monitoring through reducing sensor acquisition costs for fault identification and classification problems, easing their way towards the digital transformation required for the fourth industrial revolution.
In this study, the performance of the mesoscale Weather Research and Forecasting (WRF) model is evaluated using combinations of three planetary boundary layers (PBL) (YSU, ACM2, and MYJ) and three land surface model (LSM) schemes (RUC, Noah and Noah-MP) in order to identify the optimal parameters for the determination of wind speed in a tropical region. The state of Bahia in Brazil is selected as the location for the case study and simulations are performed over a period of eight months between 2015 and 2016 (dry and rainy seasons). The results of the simulations are compared with observational data obtained from three towers equipped with anemometers at heights of 80, 100, 120 and 150 m, strategically placed at each site and evaluated with statistical indices: MB, RMSE, MAGE, IOA, R, Fac2 and standard deviation. Overestimation of wind speed is observed in the simulations, despite similarities between the simulated and observed wind directions. In addition, the accuracies of simulations corresponding to sites that are closer to the ocean are observed to be lower. The most accurate wind speed estimates are obtained corresponding to Mucugê, which is located farthest from the ocean. Finally, analysis of the results obtained from each tower accounting for periods with higher and lower precipitation reveals that the combination of the PBL-YSU scheme with the LSM-RUC scheme yields the best results.
Reverse time migration (RTM) modeling is a computationally and data intensive component in the seismic processing workflow of oil and gas exploration. Therefore, the computational kernels of the RTM algorithms need to access a large range of memory locations. However, most of these accesses result in cache misses, degrading the overall system performance. GPU and FPGA are the two endpoints in the spectrum of acceleration platforms, since both can achieve better performance in comparison to CPU on several high-performance applications. Recent literature highlights FPGA better energy efficiency when compared to GPU. The present work proposes a FPGA accelerated platform prototype targeting the computation of the RTM algorithm on an HPC environment. Experimental results highlight that speedups of 112x can be achieved, when compared to a sequential execution on CPU. When compared to a GPU, the energy consumption has been reduced up to 55%.
The evolution of low-cost sensors (LCSs) has made the spatio-temporal mapping of indoor air quality (IAQ) possible in real-time but the availability of a diverse set of LCSs make their selection challenging. Converting individual sensors into a sensing network requires the knowledge of diverse research disciplines, which we aim to bring together by making IAQ an advanced feature of smart homes. The aim of this review is to discuss the advanced home automation technologies for the monitoring and control of IAQ through networked air pollution LCSs. The key steps that can allow transforming conventional homes into smart homes are sensor selection, deployment strategies, data processing, and development of predictive models. A detailed synthesis of air pollution LCSs allowed us to summarise their advantages and drawbacks for spatio-temporal mapping of IAQ. We concluded that the performance evaluation of LCSs under controlled laboratory conditions prior to deployment is recommended for quality assurance/control (QA/QC), however, routine calibration or implementing statistical techniques during operational times, especially during long-term monitoring, is required for a network of sensors. The deployment height of sensors could vary purposefully as per location and exposure height of the occupants inside home environments for a spatio-temporal mapping. Appropriate data processing tools are needed to handle a huge amount of multivariate data to automate pre-/post-processing tasks, leading to more scalable, reliable and adaptable solutions. The review also showed the potential of using machine learning technique for predicting spatio-temporal IAQ in LCS networked-systems.
This study simulates an unusual extreme rainfall event that occurred in Salvador City, Bahia, Brazil, on December 9, 2017, which was named subtropical storm Guará and had precipitation of approximately 24 mm within less than 1 h. Numerical simulations were conducted using the Weather Research and Forecasting (WRF) model over three domains with horizontal resolutions of 9, 3, and 1 km. Different combinations of seven microphysics, three cumulus, and three planetary boundary layer schemes were evaluated based on their ability to simulate the hourly precipitation during this rainfall event. Statistical indices (MB = -0.69; RMSE = 4.11; MAGE = 1.74; r = 0.55; IOA = 0.66, FAC2 = 0.58) and 47 time series plots showed that the most suitable configuration for this weather event were Mellor-Yamada-Janjić, Grell- Freitas, and Lin for the planetary boundary layer, cumulus, and microphysics schemes, respectively. The results were compared with the data measured at meteorological stations located in Salvador City. The WRF model simulated well the arrival and occurrence of this extreme weather event in a tropical and coastal region, considering that the region already has intense convective characteristics and is constantly influenced by sea breezes, which could interfere in the model results and compromise the performance of the simulations.
This work aims to compare deep learning models designed to predict daily number of cases and deaths caused by COVID-19 for 183 countries, using a daily basis time series, in addition to a feature augmentation strategy based on Discrete Wavelet Transform (DWT). The following deep learning architectures were compared using two different feature sets with and without DWT: (1) a homogeneous architecture containing multiple LSTM (Long-Short Term Memory) layers and (2) a hybrid architecture combining multiple CNN (Convolutional Neural Network) layers and multiple LSTM layers. Therefore, four deep learning models were evaluated: (1) LSTM, (2) CNN + LSTM, (3) DWT + LSTM and (4) DWT + CNN + LSTM. Their performances were quantitatively assessed using the metrics: Mean Absolute Error (MAE), Normalized Mean Squared Error (NMSE), Pearson R, and Factor of 2. The models were designed to predict the daily evolution of the two main epidemic variables up to 30 days ahead. After a fine-tuning procedure for hyperparameters optimization of each model, the results show a statistically significant difference between the models' performances both for the prediction of deaths and confirmed cases (p-value
Metropolitan areas may suffer with increase of air pollution due to the growth of urbanization, transportation, and industrial sectors. The Metropolitan Area of Vitoria (MAV) in Brazil is facing air pollution problems, especially because of the urbanization of past years and of having many industries inside the metropolitan area. Developing air quality system is crucial to understand the air pollution mechanism over these areas. However, having a good input dataset for applying on photochemical models is hard and requires quite of research. One input file for air quality modeling which can play a key role on results is the lateral boundary conditions (LBC). This study aimed to investigate the influence of LBC over CMAQ simulation for particulate matter and ozone over MAV by applying four different methods as LBC during August 2010. The first scenario (M1) is based on a fixed, time-independent boundary conditions with zero concentrations for all pollutants; the second scenario (M2) used a fixed, time-independent concentration values, with average values from local monitoring stations; the third CMAQ nesting scenario (M3) used the nested boundary conditions varying with time from a previous simulation with CMAQ over a larger modeling domain, centered on MAV; and finally, the fourth GEOS-Chem scenario (M4) used the boundary conditions varying with time from simulations of global model GEOS-Chem. All scenarios runs are based on the same meteorology conditions and pollutant emissions. The air quality simulations were made over a domain 61x79km centered on coordinates -20.25 degrees S, -40.28 degrees W with a resolution of 1km. The results were evaluated with the measured data from the local monitoring stations. Overall, significant differences on concentrations and number of chemical species between the LBC scenarios are shown across all LBC scenarios. The M3 and M4 dynamic LBC scenarios showed the best performances over ozone estimates while M1 and M2 had poor performance. Although no LBC scenarios do not seem to have a great influence on total PM10 and PM2.5 concentrations, individual PM2.5 species like Na, NO3-, and NH(4)(+)concentrations are influenced by the dynamic LBC approach, since those hourly individual PM2.5 species from CMAQ nesting approach (M3) and GEOS-Chem model (M4) were used as an input to LBC.
Brazilian legal system prescribes means of ensuring the prompt processing of court cases, such as the principle of reasonable process duration, the principle of celerity, procedural economy, and due legal process, with a view to optimizing procedural progress. In this context, one of the great challenges of the Brazilian judiciary is to predict the duration of legal cases based on information such as the judge, lawyers, parties involved, subject, monetary values of the case, starting date of the case, etc. Recently, there has been great interest in estimating the duration of various types of events using artificial intelligence algorithms to predict future behaviors based on time series. Thus, this study presents a proof-of-concept for creating and demonstrating a mechanism for predicting the amount of time, after the case is argued in court (time when a case is made available for the magistrate to make the decision), for the magistrate to issue a ruling. Cases from a Regional Labor Court were used as the database, with preparation data in two ways (original and discretization), to test seven machine learning techniques (i) Multilayer Perceptron (MLP); (ii) Gradient Boosting; (iii) Adaboost; (iv) Regressive Stacking; (v) Stacking Regressor with MLP; (vi) Regressive Stacking with Gradient Boosting; and (vii) Support Vector Regression (SVR), and determine which gives the best results. After executing the runs, it was identified that the adaboost technique excelled in the task of estimating the duration for issuing a ruling, as it had the best performance among the tested techniques. Thus, this study shows that it is possible to use machine learning techniques to perform this type of prediction, for the test data set, with an R2 of 0.819 and when transformed into levels, an accuracy of 84%.
Estimating heart rate is important for monitoring users in various situations. Estimates based on facial videos are increasingly being researched because they allow the monitoring of cardiac information in a non-invasive way and because the devices are simpler, as they require only cameras that capture the user’s face. From these videos of the user’s face, machine learning can estimate heart rate. This study investigates the benefits and challenges of using machine learning models to estimate heart rate from facial videos through patents, datasets, and article review. We have searched the Derwent Innovation, IEEE Xplore, Scopus, and Web of Science knowledge bases and identified seven patent filings, eleven datasets, and twenty articles on heart rate, photoplethysmography, or electrocardiogram data. In terms of patents, we note the advantages of inventions related to heart rate estimation, as described by the authors. In terms of datasets, we have discovered that most of them are for academic purposes and with different signs and annotations that allow coverage for subjects other than heartbeat estimation. In terms of articles, we have discovered techniques, such as extracting regions of interest for heart rate reading and using video magnification for small motion extraction, and models, such as EVM-CNN and VGG-16, that extract the observed individual’s heart rate, the best regions of interest for signal extraction, and ways to process them.
In this work we report numerical simulations of the contaminant dispersion and photochemical reactions of rocket exhaust clouds at the Centro de Lancamento de Alcantara (CLA) using the CMAQ modeling system. The simulations of carbon monoxide (CO), hydrogen chloride (HCl) and alumina (solid Al2O3) pollutants emission represent an effort in the construction of a computational tool in order to simulate normal and/or accidental events during rocket launches, making possible to predict the contaminant concentrations in accordance with emergency plans and pre and post-launchings for environmental management. The carbon monoxide and the alumina concentrations showed the formation of the ground and contrail cloud. The results also showed that hydrogen chloride concentrations would be harmful to human health, demonstrating the importance of assessing the impact of rocket launches in the environment and human health.
Atmospheric pollutants are strongly affected by transport processes and chemical transformations that alter their composition and the level of contamination in a region. In the last decade, several studies have employed numerical modeling to analyze atmospheric pollutants. The objective of this study is to evaluate the performance of the WRF-SMOKE-CMAQ modeling system to represent meteorological and air quality conditions over SAo Paulo, Brazil, where vehicular emissions are the primary contributors to air pollution. Meteorological fields were modeled using the Weather Research and Forecasting model (WRF), for a 12-day period during the winter of 2008 (Aug. 10th-Aug. 22nd), using three nested domains with 27-km, 9-km, and 3-km grid resolutions, which covered the most polluted cities in SAo Paulo state. The 3-km domain was aligned with the Sparse Matrix Operator Kernel Emissions (SMOKE), which processes the emission inventory for the Models-3 Community Multiscale Air Quality Modeling System (CMAQ). Data from an aerosol sampling campaign was used to evaluate the modeling. The PM10 and ozone average concentration of the entire period was well represented, with correlation coefficients for PM10, varying from 0.09 in Pinheiros to 0.69 in ICB/USP, while for ozone, the correlation coefficients varied from 0.56 in Pinheiros to 0.67 in IPEN. However, the model underestimated the concentrations of PM2.5 during the experiment, but with ammonium showing small differences between predicted and observed concentrations. As the meteorological model WRF underestimated the rainfall and overestimated the wind speed, the accuracy of the air quality model was expected to be below the desired value. However, in general, the CMAQ model reproduced the behavior of atmospheric aerosol and ozone in the urban area of SAo Paulo.
This Paper provides a qualitative analysis of the contaminant dispersion caused by the SpaceX Falcon 9 rocket accident at Cape Canaveral Air Force Station on 1 September 2016. To achieve this, the Model for Simulating Rocket Exhaust Dispersion and its modeling system were applied to simulate the dispersion of the contaminants emitted during the explosion of the Falcon 9 rocket. This modeling system is a modern tool for risk management and environmental analysis for the evaluation of normal and aborted rocket launch events, being also suitable for the assessment of explosion cases. It deals with the representation of the source term (formation, rising, expansion, and stabilization of the exhaust cloud), the simulation of the short-range dispersion (in the scale from minutes to a couple of hours), and the long-range and chemical transport modeling by integrating with the Community Multiscale Air Quality model and reading meteorological input data from the Weather Research and Forecast model. The results showed that the modeling system captured satisfactorily the phenomenon inside the planetary boundary layer.
The majority of current approaches for bias and fairness identification or mitigation in machine learning models are applications for a particular issue that fails to account for the connection between the application context and its associated sensitive attributes, which contributes to the recognition of consistent patterns in the application of bias and fairness metrics. This can be used to drive the development of future models, with the sensitive attribute acting as a connecting element to these metrics. Hence, this study aims to analyze patterns in several metrics for identifying bias and fairness, applying the gender-sensitive attribute as a case study, for three different areas of applications in machine learning models: computer vision, natural language processing, and recommendation systems. The gender attribute case study has been used in computer vision, natural language processing, and recommendation systems. The method entailed creating use cases for facial recognition in the FairFace dataset, message toxicity in the Jigsaw dataset, and movie recommendations in the MovieLens100K dataset, then developing models based on the VGG19, BERT, and Wide Deep architectures and evaluating them using the accuracy, precision, recall, and F1-score classification metrics, as well as assessing their outcomes using fourteen fairness metrics. Certain metrics disclosed bias and fairness, while others did not, revealing a consistent pattern for the same sensitive attribute across different application domains, and similarities for the statistical parity, PPR disparity, and error disparity metrics across domains, indicating fairness related to the studied sensitive attribute. Some attributes, on the other hand, did not follow this pattern. As a result, we conclude that the sensitive attribute may play a crucial role in defining the fairness metrics for a specific context.
The concern about air pollution in urban areas has substantially increased worldwide. One of its main components, particulate matter (PM) with aerodynamic diameter of ≤2.5 µm (PM2.5), can be inhaled and deposited in deeper regions of the respiratory system, causing adverse effects on human health, which are even more harmful to children. In this sense, the use of deterministic and stochastic models has become a key tool for predicting atmospheric behavior and, thus, providing information for decision makers to adopt preventive actions to mitigate air pollution impacts. However, stochastic models present their own strengths and weaknesses. To overcome some of disadvantages of deterministic models, there has been an increasing interest in the use of deep learning, due to its simpler implementation and its success on multiple tasks, including time series and air quality forecasting. Thus, the objective of the present study is to develop and evaluate the use of four different topologies of deep artificial neural networks (DNNs), analyzing the impact of feature augmentation in the prediction of PM2.5 concentrations by using five levels of discrete wavelet transform (DWT). The following types of deep neural networks were trained and tested on data collected from two living lab stations next to high-traffic roads in Guildford, UK: multi-layer perceptron (MLP), long short-term memory (LSTM), one-dimensional convolutional neural network (1D-CNN) and a hybrid neural network composed of LSTM and 1D-CNN. The performance of each model in making predictions up to twenty-four hours ahead was quantitatively assessed through statistical metrics. The results show that wavelets improved the forecasting results and that discrete wavelet transform is a relevant tool to enhance the performance of DNN topologies, with special emphasis on the hybrid topology that achieved the best results among the applied models.
Predictive maintenance is an invaluable tool to preserve the health of mission critical assets while minimizing the operational costs of scheduled intervention. Artificial intelligence techniques have been shown to be effective at treating large volumes of data, such as the ones collected by the sensors typically present in equipment. In this work, we aim to identify and summarize existing publications in the field of predictive maintenance that explore machine learning and deep learning algorithms to improve the performance of failure classification and detection. We show a significant upward trend in the use of deep learning methods of sensor data collected by mission critical assets for early failure detection to assist predictive maintenance schedules. We also identify aspects that require further investigation in future works, regarding exploration of life support systems for supercomputing assets and standardization of performance metrics.
Large variations in wind energy production over a period of minutes to hours is a challenge for electricity balancing authorities. The use of reliable tools for the prediction of wind power and wind power ramp events is essential for the operator of the electrical system. The main objective of this work is to analyze the wind power and wind power ramp forecasting at Brazil and Uruguay. To achieve this goal the wavelet decomposition applying 48 different mother wavelet functions and deep learning techniques are used. The recurrent neural network was trained to perform the forecasting of 1 h ahead, and then, using it, the trained network was applied to recursively infer the forecasting for the next hours of the wind speed. After this computational procedure, the wind power and the wind power ramp were predicted. The results showed good accuracy and can be used as a tool to help national grid operators for the energy supply. The wavelet discrete Meyer family (dmey) demonstrates greater precision in the decomposition of the wind speed signals. Therefore, it is proven that the wavelet dmey is the most accurate in the decomposition of temporal wind signals, whether using signals from tropical or subtropical regions. •Nowcasting wind prediction in tropical and subtropical sites using AI and Wavelet.•An ANN approach for the estimation of wind power ramp using deep learning.•Modeling of wind using atmospheric factors in tropical and subtropical sites.•Wind power and wind power ramp forecasting applying 48 mother Wavelet functions.
A large number of reports present artificial intelligence (AI) algorithms, which support pneumonia detection caused by COVID-19 from chest CT (computed tomography) scans. Only a few studies provided access to the source code, which limits the analysis of the out-of-distribution generalization ability. This study presents Cimatec-CovNet-19, a new light 3D convolutional neural network inspired by the VGG16 architecture that supports COVID-19 identification from chest CT scans. We trained the algorithm with a dataset of 3000 CT Scans (1500 COVID-19-positive) with images from different parts of the world, enhanced with 3000 images obtained with data augmentation techniques. We introduced a novel pre-processing approach to perform a slice-wise selection based solely on the lung CT masks and an empirically chosen threshold for the very first slice. It required only 16 slices from a CT examination to identify COVID-19. The model achieved a recall of 0.88, specificity of 0.88, ROC-AUC of 0.95, PR-AUC of 0.95, and F1-score of 0.88 on a test set with 414 samples (207 COVID-19). These results support Cimatec-CovNet-19 as a good and light screening tool for COVID-19 patients. The whole code is freely available for the scientific community.
Head-mounted displays are virtual reality devices that may be equipped with sensors and cameras to measure a patient's heart rate through facial regions. Heart rate is an essential body signal that can be used to remotely monitor users in a variety of situations. There is currently no study that predicts heart rate using only highlighted facial regions; thus, an adaptation is required for beats per minute predictions. Likewise, there are no datasets containing only the eye and lower face regions, necessitating the development of a simulation mechanism. This work aims to remotely estimate heart rate from facial regions that can be captured by the cameras of a head-mounted display using state-of-the-art EVM-CNN and Meta-rPPG techniques. We developed a region of interest extractor to simulate a dataset from a head-mounted display device using stabilizer and video magnification techniques. Then, we combined support vector machine and FaceMash to determine the regions of interest and adapted photoplethysmography and beats per minute signal predictions to work with the other techniques. We observed an improvement of 188.88% for the EVM and 55.93% for the Meta-rPPG. In addition, both models were able to predict heart rate using only facial regions as input. Moreover, the adapted technique Meta-rPPG outperformed the original work, whereas the EVM adaptation produced comparable results for the photoplethysmography signal.
Meteorological data collected using ocean buoys are very important for weather forecasting. In addition, they provide valuable information on ocean–atmosphere interaction processes that have not yet been explored. Accordingly, data collection using ocean buoys is well established around the world. In Brazil, ocean buoy data are obtained by the Brazilian Navy through a monitoring network on the Brazilian coast, which has high potential for wind power generation. In this context, the present study aimed to analyze the scaling behavior of wind speed on the Brazilian coast (continental shelf), South Atlantic Ocean and coast of Africa in order to determine long-range correlations and acquire more information on the crossover phenomenon at various scales. For this purpose, the detrended fluctuation analysis technique and numerical simulation with the Weather Research and Forecasting mesoscale model were used. The results from buoys show that wind speed exhibits a scaling behavior, but without the crossover phenomenon in the Brazilian coast, South Atlantic Ocean and coast of Africa, indicating the dependence of the phenomenon by the terrestrial surface, suggesting influence on the wind power generation. Buoy data from the South Atlantic Ocean and coast Africa showed a subdiffusive behavior (α≥1), whereas those from the Brazilian coast indicated persistence (0.5
Generally, the action recognition task requires a vast amount of labeled data, which represents a time-consuming human annotation effort. To mitigate the dependency on labeled data, this study proposes Semi-Supervised and Iterative Reinforcement Learning (RL-SSI), which adapts a supervised approach that uses 100% labeled data to a semi-supervised and iterative approach using reinforcement learning for human action recognition in videos. The JIGSAWS and Breakfast datasets were used to evaluate the RL-SSI model, because they are commonly used in the action segmentation task. The same applies to the performance metrics used in this work-F-Score (F1) and Edit Score-which are commonly applied for such tasks. In JIGSAWS tests, we observed that the RL-SSI outperformed previously developed state-of-the-art techniques in all quantitative measures, while using only 65% of the labeled data. When analysing the Breakfast tests, we compared the effectiveness of RL-SSI with the results of the self-supervised technique called SSTDA. We have found that RL-SSI outperformed SSTDA with an accuracy of 66.44% versus 65.8%, but RL-SSI was surpassed by the F1@10 segmentation measure, which presented an accuracy of 67.33% versus 69.3% for SSTDA. Despite this, our experiment only used 55.8% of the labeled data, while SSTDA used 65%. We conclude that our approach outperformed equivalent supervised learning methods and is comparable to SSTDA, when evaluated on multiple datasets of human action recognition, proving to be an important innovative method to successfully building solutions to reduce the amount of fully labeled data, leveraging the work of human specialists in the task of data labeling of videos, and their respectives frames, for human action recognition, thus reducing the required resources to accomplish it.
One of the difficulties of artificial intelligence is to ensure that model decisions are fair and free of bias. In research, datasets, metrics, techniques, and tools are applied to detect and mitigate algorithmic unfairness and bias. This study examines the current knowledge on bias and unfairness in machine learning models. The systematic review followed the PRISMA guidelines and is registered on OSF plataform. The search was carried out between 2021 and early 2022 in the Scopus, IEEE Xplore, Web of Science, and Google Scholar knowledge bases and found 128 articles published between 2017 and 2022, of which 45 were chosen based on search string optimization and inclusion and exclusion criteria. We discovered that the majority of retrieved works focus on bias and unfairness identification and mitigation techniques, offering tools, statistical approaches, important metrics, and datasets typically used for bias experiments. In terms of the primary forms of bias, data, algorithm, and user interaction were addressed in connection to the preprocessing, in-processing, and postprocessing mitigation methods. The use of Equalized Odds, Opportunity Equality, and Demographic Parity as primary fairness metrics emphasizes the crucial role of sensitive attributes in mitigating bias. The 25 datasets chosen span a wide range of areas, including criminal justice image enhancement, finance, education, product pricing, and health, with the majority including sensitive attributes. In terms of tools, Aequitas is the most often referenced, yet many of the tools were not employed in empirical experiments. A limitation of current research is the lack of multiclass and multimetric studies, which are found in just a few works and constrain the investigation to binary-focused method. Furthermore, the results indicate that different fairness metrics do not present uniform results for a given use case, and that more research with varied model architectures is necessary to standardize which ones are more appropriate for a given context. We also observed that all research addressed the transparency of the algorithm, or its capacity to explain how decisions are taken.
This work presents a novel investigation on the nowcasting prediction of wind speed for three sites in Bahia, Brazil. For this, it was applied the computational intelligence by supervised machine learning using different artificial neural network technique, which was trained, validated, and tested using time series are derived from measurements that are acquired in towers equipped with anemometers at heights of 100.0, 120.0 and 150.0 m. To define the most efficient ANN, different topologies were tested using MLP and RNN, applying Wavelet packet decomposition (bior, coif, db, dmey, rbior, sym). The best statistical analysis was RNN + discrete Meyer wavelet.
In atmospheric environments, traditional differential equations do not adequately describe the problem of turbulent diffusion because the usual derivatives are not well defined in the non-differentiable behaviour introduced by turbulence, where the fractional calculation has become a very useful tool for studying anomalous dispersion and other transportation processes. Considering a new direction, this paper presents an analytical series solution of a three-dimensional advection-diffusion equation of fractional order, in the Caputo sense, applied to the dispersion of atmospheric pollutants. The solution is obtained by applying the generalised integral transform technique (GITT), solving the transformed problem by the Laplace decomposition method (LDM), and considering the lateral and vertical turbulent diffusion dependence on the longitudinal distance from the source, as well as a fractional parameter. The fractional solution is more general than the traditional solution in the sense that consideration of the integer order of the fractional parameter yields the traditional solution. The solution considers the memory effect in eddy diffusivity and in the fractional derivative, and it is simple, easy to implement, and converges rapidly. Numerical simulations were conducted to compare the performance of the proposed fractional solution to the traditional solution using an experimental dataset and other models, which also made it possible to find a better parametrisation for use in Gaussian models. The best results are obtained with the fractional order of the derivative. (C) 2019 Elsevier Ltd. All rights reserved.
Given the growth and availability of computing power, artificial intelligence techniques have been applied to industrial equipment and computing devices in order to identify abnormalities in operation and predict the remaining useful life (RUL) of equipment with superior performance than traditional predictive maintenance. In this sense, this research aims to develop a neural network applied to predictive maintenance in mission critical supercomputing environments (MCSE) using deep learning techniques to predict the RUL of an equipment before the occurrence of failures, by using real historical unlabeled data, which were collected by sensors installed in a supercomputing environment. The method was developed using a hybrid approach based on a combination of Fully Convolutional Neural Network, Long Short-Term Memory and Multilayer Perceptron. The results presented a Pearson R of 0.87, R2 of 0.77, Factor of 2 of 0.89, and Normalized mean square error of 0.79, considering the predicted RUL value and the observed RUL value for the pre-failure behavior moments of the equipment. Thus, we can conclude that the developed approach had good performance to predict the RUL, increasing the ability to anticipate the failure situation of the MCSE, further increasing its availability and operating time.
This study presents the development of a new model named MSRED, which was designed to simulate the formation, rise, expansion, stabilisation and dispersion of rocket exhaust clouds for short-range assessment, using a three-dimensional semi-analytical solution of the advection–diffusion equation based on the ADMM method. For long-range modelling, the MSRED was built to generate a ready-to-use initial conditions file to be input to the CMAQ model, as it represents the state-of-the-art in regional and chemical transport air quality modelling. Simulations and analysis were carried out in order to evaluate the application of this integrated modelling system for different rocket launch cases and atmospheric conditions, for the Alcantara Launching Center (ALC, the Brazilian gate to the space) region. This hybrid, modern and multidisciplinary system is the basis of a modelling framework that will be employed at ALC for pre- and post-launching simulations of the environmental effects of rocket operations.
This work analyzes the time series of wind speeds in different regions of the state of Bahia and the Abrolhos Archipelago, Brazil, through the use of the DFA technique (Detrended Fluctuation Analysis) to verify the existence of long-range correlations and associated power laws. The time series of wind velocities are derived from measurements with hourly means that are acquired in three towers equipped with anemometers at heights of 80, 100, 120 and 150 m, and in the Abrolhos Archipelago with measurements taken at 10 m. These measurements are then compared with numerical simulations of the wind speed obtained with the WRF mesoscale model (Weather Research and Forecasting model). In the onshore case, the results of the application of the DFA technique in the measured and simulated datasets show correlations with power laws in two regions of distinct scales (subdiffusive and persistent) for both time series. It is suggested that this occurs due to the mesoscale effects and local circulations acting on the planetary boundary layer, where the turbulence in the daily cycle is generated by thermal (buoyancy) and mechanical (wind shear) forcing. However, in regions that are not subject to local-effect conditions, such as small islands far from the mainland, the synoptic effects are the most important and active in the maritime boundary layer, so the series of real and simulated datasets exhibit only subdiffusive behavior. (C) 2018 Elsevier Ltd. All rights reserved.
Due to the recent COVID-19 pandemic, a large number of reports present deep learning algorithms that support the detection of pneumonia caused by COVID-19 in chest radiographs. Few studies have provided the complete source code, limiting testing and reproducibility on different datasets. This work presents Cimatec_XCOV19, a novel deep learning system inspired by the Inception-V3 architecture that is able to (i) support the identification of abnormal chest radiographs and (ii) classify the abnormal radiographs as suggestive of COVID-19. The training dataset has 44,031 images with 2917 COVID-19 cases, one of the largest datasets in recent literature. We organized and published an external validation dataset of 1158 chest radiographs from a Brazilian hospital. Two experienced radiologists independently evaluated the radiographs. The Cimatec_XCOV19 algorithm obtained a sensitivity of 0.85, specificity of 0.82, and AUC ROC of 0.93. We compared the AUC ROC of our algorithm with a well-known public solution and did not find a statistically relevant difference between both performances. We provide full access to the code and the test dataset, enabling this work to be used as a tool for supporting the fast screening of COVID-19 on chest X-ray exams, serving as a reference for educators, and supporting further algorithm enhancements.
Great efforts have been made over the years to assess the effectiveness of air pollution controls in place in the metropolitan area of Sao Paulo (MASP), Brazil. In this work, the community multiscale air quality (CMAQ) model was used to evaluate the efficacy of emission control strategies in MASP, considering the spatial and temporal variability of fine particle concentration. Seven different emission scenarios were modeled to assess the relationship between the emission of precursors and ambient aerosol concentration, including a baseline emission inventory, and six sensitivity scenarios with emission reductions in relation to the baseline inventory: a 50% reduction in SO2 emissions; no SO2 emissions; a 50% reduction in SO2, NOx, and NH3 emissions; no sulfate (PSO4) particle emissions; no PSO4 and nitrate (PNO3) particle emissions; and no PNO3 emissions. Results show that ambient PM2.5 behavior is not linearly dependent on the emission of precursors. Variation levels in PM2.5 concentrations did not correspond to the reduction ratios applied to precursor emissions, mainly due to the contribution of organic and elemental carbon, and other secondary organic aerosol species. Reductions in SO2 emissions are less likely to be effective at reducing PM2.5 concentrations at the expected rate in many locations of the MASP. The largest reduction in ambient PM2.5 was obtained with the scenario that considered a reduction in 50% of SO2, NOx, and NH3 emissions (1 to 2 mu g/m(3) on average). It highlights the importance of considering the role of secondary organic aerosols and black carbon in the design of effective policies for ambient PM2.5 concentration control.
This work presents an analytical solution of the two-dimensional advection-diffusion equation of fractional order, in the sense of Caputo and applied it to the dispersion of atmospheric pollutants. The solution is obtained using Laplace decomposition and homotopy perturbation methods, and it considers the vertical eddy diffusivity dependency on the longitudinal distance of the source with fractional exponents of the same order of the fractional derivative (Kx). For validation of the model, simulations were compared with data from Copenhagen experiments considering moderately unstable conditions. The best results were obtained with =0.98, considering wind measured at 10m, and =0.94 with wind measured at a height of 115m.
Short-term wind speed forecasting for Colonia Eulacio, Soriano Department, Uruguay, is performed by applying an artificial neural network (ANN) technique to the hourly time series representative of the site. To train the ANN and validate the technique, data for one year are collected by one tower, with anemometers installed at heights of 101.8, 81.8, 25.7, and 10.0 m. Different ANN configurations are applied for each site and height; then, a quantitative analysis is conducted, and the statistical results are evaluated to select the configuration that best predicts the real data. This method has lower computational costs than other techniques, such as numerical modelling. For integrating wind power into existing grid systems, accurate short-term wind speed forecasting is fundamental. Therefore, the proposed short-term wind speed forecasting method is an important scientific contribution for reliable large-scale wind power forecasting and integration in Uruguay. The results of the short-term wind speed forecasting showed good accuracy at all the anemometer heights tested, suggesting that the method is a powerful tool that can help the Administración Nacional de Usinas y Transmissiones Eléctricas manage the national energy supply.
The purpose of this work is to build, train and evaluate a deep learning-based model to forecast tropospheric ozone levels hourly, up to twenty-four hours ahead, using data gathered from the automatic air quality monitoring system in the metropolitan region of Vitória city, Espírito Santo (ES), Brazil. Observational data of air pollutant concentrations and meteorological parameters were used as the input variables of the model once they represented the state of the atmospheric fluid in terms of its properties and chemical composition throughout the time. Several topologies of multilayer perceptron neural networks were tried and evaluated using statistics of the predictions over unseen data. The best architecture was compared with reference models and the results showed that deep learning models can be successfully applied to hourly forecasting of ozone concentrations for urban areas. Once such models are fitted to the data, the forecasting procedure has a very low computational cost, meaning that it can be used as an alternative approach in comparison with numerical modelling systems, which require much more computational power.
The community multiscale air quality (CMAQ) model was evaluated in the metropolitan region of Salvador (MRS), which is the capital of the state of Bahia, Brazil. Once located at the tropics, two episodes were selected (one in the dry season and another in the rainy season), in order to perform the assessment. The meteorological information required for air quality modeling was driven by the weather research and forecasting (WRF) model: its performance was evaluated against observational data collected by monitoring stations located in Salvador city, Brazil. For the emissions inventory, we applied the Emissions Database for Global Atmospheric Research (EDGAR), in conjunction with an estimated emissions inventory of local point sources located in the major industrial complexes, in addition with the biogenic emissions estimated by the model of emissions of gases and aerosols from nature (MEGAN). To compute these different emissions sources, we used the sparse matrix operator kernel emission (SMOKE). Following that, the CMAQ model was applied, in order to simulate the chemical transport and formation of air pollutants based on the meteorological and emissions input previously described. The required boundary conditions were determined by also applying the CMAQ model, which used data from the MEGAN and EDGAR inventories, to a larger domain that incorporates the domain in which the MRS is represented. The application of these modeling tools was shown to be a good practice in the assessment of air quality in urban areas; thus, this work aims to be the first great effort to study, simulate and validate the chemical transport of pollutants over the MRS, based on a hybrid emissions inventory and using the state of the art in the computational atmospheric modeling field.
This study focuses on atmospheric emissions from road vehicles in the Metropolitan Region of Salvador (RMS), northeaster Brazil. To investigate and analyse the contributions to air pollution, the methodology proposed and developed by the Ministry of the Environment (MMA) is used in the 1st National Inventory of Road Vehicle Emissions in 2011, the application of which has been published annually by the State Environmental Company of São Paulo (CETESB), from the 1st Vehicle Emissions Report, of which electric, hybrid and CNG vehicles are not considered. Usually, in the preparation of inventories, the emission rates are estimated according to the circulating fleet categorized by tonnage (light/heavy and passenger/cargo), type of fuel used, intensity of use and emission factors. Emissions are due to exhaust, fuel evaporation rate and tire, brakes, and track wear. Although, the emission inventories present uncertainties associated with the calculation of emissions according to the characteristics of the fuel, the circulating fleet and the technology on board, these inventories are powerful tools in this type of research. In this sense, the objective of this work was to elaborate an inventory of emissions by road vehicles in the RMS, referring to the period from 2013 to 2017, to subsidize the management of air quality in the RMS. The results show that RMS owns 36.48% of the circulating fleet in the State of Bahia, comprising approximately 65% of automobiles and 7.0% of heavy vehicles (trucks and buses) and heavy vehicles emit the highest levels of Nox and PM, with approximately 84% and 70%, respectively.
Short-range wind speed predictions for subtropical region is performed by applying Artificial Neural Network (ANN) technique to the hourly time series representative of the site. To train the ANN and validate the technique, data for one year are collected by one tower, with anemometers installed at heights of 101.8, 81.8, 25.7, and 10.0 m. Different ANN configurations to Multilayer Perceptron (MLP), Recurrent Neural Network (RNN), Gated Recurrent Unit (GRU), and Long Short-Term Memory (LSTM), a deep learning algorithm based method, are applied for each site and height. A quantitative analysis is conducted and the statistical results are evaluated to select the configuration that best predicts the real data. These methods have lower computational costs than other techniques, such as numerical modelling. The proposed method is an important scientific contribution for reliable large-scale wind power forecasting and integration into existing grid systems in Uruguay. The best results of the short-term wind speed forecasting was for MLP, which performed the forecasts using a hybrid method based on recursive inference, followed by LSTM, at all the anemometer heights tested, suggesting that this method is a powerful tool that can help the Administración Nacional de Usinas y Transmissiones Eléctricas manage the national energy supply.
Seismic modeling is the process of simulating wave propagations in a medium to represent underlying structures of a subsurface area of the earth. This modeling is based on a set of parameters that determine how the data is produced. Recent studies have demonstrated that deep learning methods can be trained with seismic data to estimate velocity models that give a representation of the subsurface where the seismic data was generated. Thus, an analysis is made on the impact that different sets of parameters have on the estimation of velocity models by a fully convolutional network (FCN). The experiments varied the number of sources among four options (1, 10, 25 or 50 shots) and used three different ranges of peak frequencies: 4, 8 and 16 Hz. The results demonstrated that, although the number of sources have more influence on the computational time needed to train the FCN than the peak frequency, both changes have significant impact on the quality of the estimation. The best estimations were obtained with the experiment of 25 sources with 4 Hz and increasing the peak frequency to 8 Hz improved even more the results, especially regarding the FCN's loss function.
In the industrial environment, the health status of critical machinery is constantly monitored, consequently generating a large amount of data that needs to be analyzed by experts. However, it becomes unfeasible to a human to verify and correlate all real time data, especially in annotating and classifying the interesting patterns present in the data - such as normal or abnormal/failure - which is valuable in researches that involve the development of predictive and classification models using Artificial Intelligence. This paper presents a comparative study between methods of detecting interesting patterns and anomalies based on unsupervised machine learning, aiming to automate the data annotation process between normal or abnormal classes (or failures), in order to further detect the failures in industrial machinery. Multivariate real data acquired from 21 sensors coupled to a gearbox of a turbo generator were used. The results revealed that unsupervised learning methods effectively detected normal and anomalous behaviors without the need of prior labeling or classification by experts, with emphasis on the C-AMDATS algorithm. In fact, the use of real data proves that the proposed approach is suitable for unsupervised anomaly detection. Therefore, it is possible to conclude that unsupervised machine learning algorithms are able to assist experts and managers in decision making and preparing labeled data for later use in supervised machine learning algorithms for prediction and classification purposes, providing greater reliability in maintenance.