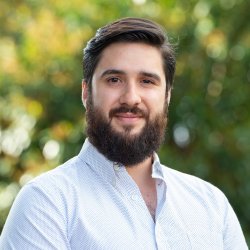
Dr Oscar Mendez Maldonado
Academic and research departments
Robotics, Centre for Vision, Speech and Signal Processing (CVSSP), School of Computer Science and Electronic Engineering.About
Biography
I am an award-winning, internationally recognised researcher, interested in the fields of Machine Learning, Robotics and Computer Vision. I’ve spent many years developing and building real-time robotic systems, creating autonomous agents and leading Machine Learning research. My work focuses developing Machine Learning research that leverages concepts and ideas from fields like Robotics and Computer Vision to create autonomous agents that have a better understanding of the world. I am interested in making autonomous agents not only understand the world around them, but to act upon this knowledge. Me and my research have featured in the media, including national newspaper articles, radio shows, web interviews and the University of Surrey News (SMILE, AVP).
Areas of specialism
University roles and responsibilities
- Lecturer for EEE1035 (Programming in C)
- Lecturer for EEE3043 (Robotics)
My qualifications
Affiliations and memberships
ResearchResearch interests
I am interested in the fields of Robotics, Computer Vision and Deep Learning. I’ve spent many years developing and building real-time robotic systems that can leverage the advances of Deep Learning to perform difficult computer vision tasks such as SLAM, 3D Reconstruction and Multi-View Geometry. I am also interested in collaboration and automation between robotic agents, specifically emergent behaviours that are not hard-coded into systems.
Research projects
As autonomous cars start to become a reality, one of the unanswered questions remains – where and how will those cars park?
This consortium’s “Autonomous Valet Parking” project seeks to develop Highly Autonomous Driving maps to support indoor navigation and localisation.
Autonomous Valet Parking (AVP) is functionality which allows a driver to be dropped off in a multi-storey car park or their final destination and the vehicle to then park itself autonomously.
Estimating the vehicle’s current position is more difficult in multi-storey carparks where GPS signals cannot be received, which means that the vehicle must rely on other sensors and localisation based on visual objects and features present in maps. This is an open problem in the automotive industry which must be solved to enable SAE Level 4 AVP deployment.
This consortium’s key objective is to identify obstacles to full deployment of AVP through the development of a technology demonstrator. It aims to achieve this goal by:
- Developing automotive-grade indoor parking maps required for autonomous vehicles to localise and navigate within a multi-storey car park.
- Developing the associated localisation algorithms – targeting a minimal sensor set of cameras, ultrasonic sensors and inertial measurement units – that make best use of these maps.
- Demonstrating this self-parking technology in a variety of car parks.
- Developing the safety case and prepare for in-car-park trials.
- Engaging with stakeholders to evaluate perceptions around AVP technology.
Scalable Multimodal sign language Technology for sIgn language Learning and assessmEnt (SMILE)The goal of the project SMILE is to pioneer an assessment system for Swiss German Sign Language (DSGS) using automatic sign language recognition technology. To achieve this goal, this project uses a multidisciplinary framework that follows two strands of research, one on sign language technology and one on sign assessment with a common link to sign language linguistics. The project consortium consists of three partner institutes with complementary expertise:
1. The Idiap Research Institute (Martigny, CH) will coordinate the project and will contribute to the project by developing a novel automatic sign language assessment and feedback approach taking inspirations from a speech recognition approach that was developed under SNSF project FlexASR.
2. The Hochschule für Heilpädagogik (HfH, Zurich, CH) will bring its expertise in sign language assessment and sign linguistics (through collaboration with Center for Sign Language Research, Basel). In addition, the HfH will play a central role in connecting the real world of L2 learners and the Deaf community in German Switzerland to the project.
3. The University of Surrey (UK) will bring to the project its longstanding expertise in sign language technology, visual data acquisition and computer vision, and in particular its wide experience in state-of-the-art sign language technology research through European-level projects such as DictaSign.
Indicators of esteem
Sullivan Thesis Prize Winner (2018)
Research interests
I am interested in the fields of Robotics, Computer Vision and Deep Learning. I’ve spent many years developing and building real-time robotic systems that can leverage the advances of Deep Learning to perform difficult computer vision tasks such as SLAM, 3D Reconstruction and Multi-View Geometry. I am also interested in collaboration and automation between robotic agents, specifically emergent behaviours that are not hard-coded into systems.
Research projects
As autonomous cars start to become a reality, one of the unanswered questions remains – where and how will those cars park?
This consortium’s “Autonomous Valet Parking” project seeks to develop Highly Autonomous Driving maps to support indoor navigation and localisation.
Autonomous Valet Parking (AVP) is functionality which allows a driver to be dropped off in a multi-storey car park or their final destination and the vehicle to then park itself autonomously.
Estimating the vehicle’s current position is more difficult in multi-storey carparks where GPS signals cannot be received, which means that the vehicle must rely on other sensors and localisation based on visual objects and features present in maps. This is an open problem in the automotive industry which must be solved to enable SAE Level 4 AVP deployment.
This consortium’s key objective is to identify obstacles to full deployment of AVP through the development of a technology demonstrator. It aims to achieve this goal by:
- Developing automotive-grade indoor parking maps required for autonomous vehicles to localise and navigate within a multi-storey car park.
- Developing the associated localisation algorithms – targeting a minimal sensor set of cameras, ultrasonic sensors and inertial measurement units – that make best use of these maps.
- Demonstrating this self-parking technology in a variety of car parks.
- Developing the safety case and prepare for in-car-park trials.
- Engaging with stakeholders to evaluate perceptions around AVP technology.
The goal of the project SMILE is to pioneer an assessment system for Swiss German Sign Language (DSGS) using automatic sign language recognition technology. To achieve this goal, this project uses a multidisciplinary framework that follows two strands of research, one on sign language technology and one on sign assessment with a common link to sign language linguistics. The project consortium consists of three partner institutes with complementary expertise:
1. The Idiap Research Institute (Martigny, CH) will coordinate the project and will contribute to the project by developing a novel automatic sign language assessment and feedback approach taking inspirations from a speech recognition approach that was developed under SNSF project FlexASR.
2. The Hochschule für Heilpädagogik (HfH, Zurich, CH) will bring its expertise in sign language assessment and sign linguistics (through collaboration with Center for Sign Language Research, Basel). In addition, the HfH will play a central role in connecting the real world of L2 learners and the Deaf community in German Switzerland to the project.
3. The University of Surrey (UK) will bring to the project its longstanding expertise in sign language technology, visual data acquisition and computer vision, and in particular its wide experience in state-of-the-art sign language technology research through European-level projects such as DictaSign.
Indicators of esteem
Sullivan Thesis Prize Winner (2018)
Supervision
Postgraduate research supervision
2020 James Ross, Co-Supervisor, University of Surrey. Autonomous Vehicles, Birds-Eye View Estimation, Semantic Segmentation.
2019 Xihan Bian, Co-Supervisor, University of Surrey. Reinforcement Learning, Artificial Intelligence, Robotics.
2019 Avishar Saha, Co-Supervisor, University of Surrey. Computer Vision and Autonomous Vehicles (Road Scene Prediction), Birds-Eye View Estimation.
2019 Nimet Kaygusuz, Co-Supervisor, University of Surrey. Robotics, Autonomous Vehicle Control and Stability (Vehicle State Estimation).
2017 Celyn Walters, Co-Supervisor, University of Surrey. Robotics, Autonomous Vehicles, Motion Planning.
Publications
Cross-view image matching for geo-localisation is a challenging problem due to the significant visual difference between aerial and ground-level viewpoints. The method provides localisation capabilities from geo-referenced images, eliminating the need for external devices or costly equipment. This enhances the capacity of agents to autonomously determine their position, navigate, and operate effectively in GNSS-denied environments. Current research employs a variety of techniques to reduce the domain gap such as applying polar transforms to aerial images or synthesising between perspectives. However, these approaches generally rely on having a 360 degree field of view, limiting real-world feasibility. We propose BEV-CV, an approach introducing two key novelties with a focus on improving the real-world viability of cross-view geo-localisation. Firstly bringing ground-level images into a semantic Birds-Eye-View before matching embeddings, allowing for direct comparison with aerial image representations. Secondly, we adapt datasets into application realistic format-limited-FOV images aligned to vehicle direction. BEV-CV achieves state-of-the-art recall accuracies, improving Top-1 rates of 70 degree crops of CVUSA and CVACT by 23% and 24% respectively. Also decreasing computational requirements by reducing floating point operations to below previous works, and decreasing embedding dimensionality by 33%-together allowing for faster localisation capabilities.
Cross-view Geo-localisation is typically performed at a coarse granularity, because densely sampled satellite image patches overlap heavily. This heavy overlap would make dis-ambiguating patches very challenging. However, by opting for sparsely sampled patches, prior work has placed an artificial upper bound on the localisation accuracy that is possible. Even a perfect oracle system cannot achieve accuracy greater than the average separation of the tiles. To solve this limitation, we propose combining cross-view geo-localisation and relative pose estimation to increase precision to a level practical for real-world application. We develop PEnG, a 2-stage system which first predicts the most likely edges from a city-scale graph representation upon which a query image lies. It then performs relative pose estimation within these edges to determine a precise position. PEnG presents the first technique to utilise both viewpoints available within cross-view geo-localisation datasets, referring to this as Multi-View Geo-Localisation (MVGL). This enhances accuracy to a sub-metre level, with some examples achieving centimetre level precision. Our proposed ensemble achieves state-of-the-art accuracy-with relative Top-5m retrieval improvements on previous works of 213%. Decreasing the median Euclidean distance error by 96.90% from the previous best of 734m down to 22.77m, when evaluating with 90° horizontal FOV images. Code is available here: github.com/tavisshore/peng.
Cross-View Geo-Localisation within urban regions is challenging in part due to the lack of geo-spatial struc-turing within current datasets and techniques. We propose utilising graph representations to model sequences of local observations and the connectivity of the target location. Modelling as a graph enables generating previously unseen sequences by sampling with new parameter configurations. To leverage this newly available information , we propose a GNN-based architecture, producing spatially strong embeddings and improving discriminabil-ity over isolated image embeddings. We outline SpaG-BOL, introducing three novel contributions. 1) The first graph-structured dataset for Cross-View Geo-Localisation, containing multiple streetview images per node to improve generalisation. 2) Introducing GNNs to the problem, we develop the first system that exploits the correlation between node proximity and feature similarity. 3) Lever-aging the unique properties of the graph representation-we demonstrate a novel retrieval filtering approach based on neighbourhood bearings. SpaGBOL achieves state-of-the-art accuracies on the unseen test graph-with relative Top-1 retrieval improvements on previous techniques of 11%, and 50% when filtering with Bearing Vector Matching on the SpaGBOL dataset. Code and dataset available: github.com/tavisshore/SpaGBOL.
Hand pose estimation from a single image has many applications. However, approaches to full 3D body pose estimation are typically trained on day-to-day activities or actions. As such, detailed hand-to-hand interactions are poorly represented, especially during motion. We see this in the failure cases of techniques such as OpenPose [6] or MediaPipe[30]. However, accurate hand pose estimation is crucial for many applications where the global body motion is less important than accurate hand pose estimation.This paper addresses the problem of 3D hand pose estimation from monocular images or sequences. We present a novel end-to-end framework for 3D hand regression that employs diffusion models that have shown excellent ability to capture the distribution of data for generative purposes. Moreover, we enforce kinematic constraints to ensure realistic poses are generated by incorporating an explicit forward kinematic layer as part of the network. The proposed model provides state-of-the-art performance when lifting a 2D single-hand image to 3D. However, when sequence data is available, we add a Transformer module over a temporal window of consecutive frames to refine the results, overcoming jittering and further increasing accuracy.The method is quantitatively and qualitatively evaluated showing state-of-the-art robustness, generalization, and accuracy on several different datasets.
Visual Odometry (VO) estimation is an important source of information for vehicle state estimation and autonomous driving. Recently, deep learning based approaches have begun to appear in the literature. However, in the context of driving, single sensor based approaches are often prone to failure because of degraded image quality due to environmental factors, camera placement, etc. To address this issue, we propose a deep sensor fusion framework which estimates vehicle motion using both pose and uncertainty estimations from multiple on-board cameras. We extract spatio-temporal feature representations from a set of consecutive images using a hybrid CNN-RNN model. We then utilise a Mixture Density Network (MDN) to estimate the 6-DoF pose as a mixture of distributions and a fusion module to estimate the final pose using MDN outputs from multi-cameras. We evaluate our approach on the publicly available, large scale autonomous vehicle dataset, nuScenes. The results show that the proposed fusion approach surpasses the state-of-the-art, and provides robust estimates and accurate trajectories compared to individual camera-based estimations.
Graph convolutional networks (GCNs) enable end-to-end learning on graph structured data. However, many works assume a given graph structure. When the input graph is noisy or unavailable, one approach is to construct or learn a latent graph structure. These methods typically fix the choice of node degree for the entire graph, which is suboptimal. Instead, we propose a novel end-to-end differentiable graph generator which builds graph topologies where each node selects both its neighborhood and its size. Our module can be readily integrated into existing pipelines involving graph convolution operations, replacing the predetermined or existing adjacency matrix with one that is learned, and optimized, as part of the general objective. As such it is applicable to any GCN. We integrate our module into trajectory prediction, point cloud classification and node classification pipelines resulting in improved accuracy over other structure-learning methods across a wide range of datasets and GCN backbones. We will release the code.
We approach instantaneous mapping, converting images to a top-down view of the world, as a translation problem. We show how a novel form of transformer network can be used to map from images and video directly to an overhead map or bird's-eye-view (BEV) of the world, in a single end-to-end network. We assume a 1-1 correspondence between a vertical scanline in the image, and rays passing through the camera location in an overhead map. This lets us formulate map generation from an image as a set of sequence-to-sequence translations. This constrained formulation , based upon a strong physical grounding of the problem, leads to a restricted transformer network that is convolutional in the horizontal direction only. The structure allows us to make efficient use of data when training, and obtains state-of-the-art results for instantaneous mapping of three large-scale datasets, including a 15% and 30% relative gain against existing best performing methods on the nuScenes and Argoverse datasets, respectively.
—The ability to produce large-scale maps for navigation , path planning and other tasks is a crucial step for autonomous agents, but has always been challenging. In this work, we introduce BEV-SLAM, a novel type of graph-based SLAM that aligns semantically-segmented Bird's Eye View (BEV) predictions from monocular cameras. We introduce a novel form of occlusion reasoning into BEV estimation and demonstrate its importance to aid spatial aggregation of BEV predictions. The result is a versatile SLAM system that can operate across arbitrary multi-camera configurations and can be seamlessly integrated with other sensors. We show that the use of multiple cameras significantly increases performance, and achieves lower relative error than high-performance GPS. The resulting system is able to create large, dense, globally-consistent world maps from monocular cameras mounted around an ego vehicle. The maps are metric and correctly-scaled, making them suitable for downstream navigation tasks.
How does a person work out their location using a floorplan? It is probably safe to say that we do not explicitly measure depths to every visible surface and try to match them against different pose estimates in the floorplan. And yet, this is exactly how most robotic scan-matching algorithms operate. Similarly, we do not extrude the 2D geometry present in the floorplan into 3D and try to align it to the real-world. And yet, this is how most vision-based approaches localise. Humans do the exact opposite. Instead of depth, we use high level semantic cues. Instead of extruding the floorplan up into the third dimension, we collapse the 3D world into a 2D representation. Evidence of this is that many of the floorplans we use in everyday life are not accurate, opting instead for high levels of discriminative landmarks. In this work, we use this insight to present a global localisation approach that relies solely on the semantic labels present in the floorplan and extracted from RGB images. While our approach is able to use range measurements if available, we demonstrate that they are unnecessary as we can achieve results comparable to state-of-the-art without them.
Hand pose estimation from a single image has many applications. However, approaches to full 3D body pose estimation are typically trained on day-to-day activities or actions. As such, detailed hand-to-hand interactions are poorly represented, especially during motion. We see this in the failure cases of techniques such as OpenPose [6] or MediaPipe[30]. However, accurate hand pose estimation is crucial for many applications where the global body motion is less important than accurate hand pose estimation. This paper addresses the problem of 3D hand pose estimation from monocular images or sequences. We present a novel end-to-end framework for 3D hand regression that employs diffusion models that have shown excellent ability to capture the distribution of data for generative purposes. Moreover, we enforce kinematic constraints to ensure realistic poses are generated by incorporating an explicit forward kinematic layer as part of the network. The proposed model provides state-of-the-art performance when lifting a 2D single-hand image to 3D. However, when sequence data is available, we add a Transformer module over a temporal window of consecutive frames to refine the results, overcoming jittering and further increasing accuracy. The method is quantitatively and qualitatively evaluated showing state-of-the-art robustness, generalization, and accuracy on several different datasets.
Visual Odometry (VO) is used in many applications including robotics and autonomous systems. However, traditional approaches based on feature matching are computationally expensive and do not directly address failure cases, instead relying on heuristic methods to detect failure. In this work, we propose a deep learning-based VO model to efficiently estimate 6-DoF poses, as well as a confidence model for these estimates. We utilise a CNN-RNN hybrid model to learn feature representations from image sequences. We then employ a Mixture Density Network (MDN) which estimates camera motion as a mixture of Gaussians, based on the extracted spatio-temporal representations. Our model uses pose labels as a source of supervision, but derives uncertainties in an unsupervised manner. We evaluate the proposed model on the KITTI and nuScenes datasets and report extensive quantitative and qualitative results to analyse the performance of both pose and uncertainty estimation. Our experiments show that the proposed model exceeds state-of-the-art performance in addition to detecting failure cases using the predicted pose uncertainty.
Hand pose estimation from a single image has many applications. However, approaches to full 3D body pose estimation are typically trained on day-to-day activities or actions. As such, detailed hand-to-hand interactions are poorly represented, especially during motion. We see this in the failure cases of techniques such as OpenPose or MediaPipe. However, accurate hand pose estimation is crucial for many applications where the global body motion is less important than accurate hand pose estimation. This paper addresses the problem of 3D hand pose estimation from monocular images or sequences. We present a novel end-to-end framework for 3D hand regression that employs diffusion models that have shown excellent ability to capture the distribution of data for generative purposes. Moreover, we enforce kinematic constraints to ensure realistic poses are generated by incorporating an explicit forward kinematic layer as part of the network. The proposed model provides state-of-the-art performance when lifting a 2D single-hand image to 3D. However, when sequence data is available, we add a Transformer module over a temporal window of consecutive frames to refine the results, overcoming jittering and further increasing accuracy. The method is quantitatively and qualitatively evaluated showing state-of-the-art robustness, generalization, and accuracy on several different datasets.
Attention is an important component of modern deep learning. However, less emphasis has been put on its inverse: ignoring distraction. Our daily lives require us to explicitly avoid giving attention to salient visual features that confound the task we are trying to accomplish. This visual prioritisation allows us to concentrate on important tasks while ignoring visual distractors.In this work, we introduce Neural Blindness, which gives an agent the ability to completely ignore objects or classes that are deemed distractors. More explicitly, we aim to render a neural network completely incapable of representing specific chosen classes in its latent space. In a very real sense, this makes the network "blind" to certain classes, allowing and agent to focus on what is important for a given task, and demonstrates how this can be used to improve localisation.
This work addresses 3D human pose reconstruction in single images. We present a method that combines Forward Kinematics (FK) with neural networks to ensure a fast and valid prediction of 3D pose. Pose is represented as a hierarchical tree/graph with nodes corresponding to human joints that model their physical limits. Given a 2D detection of keypoints in the image, we lift the skeleton to 3D using neural networks to predict both the joint rotations and bone lengths. These predictions are then combined with skeletal constraints using an FK layer implemented as a network layer in PyTorch. The result is a fast and accurate approach to the estimation of 3D skeletal pose. Through quantitative and qualitative evaluation, we demonstrate the method is significantly more accurate than MediaPipe in terms of both per joint positional error and visual appearance. Furthermore, we demonstrate generalization over different datasets. The implementation in PyTorch runs at between 100-200 milliseconds per image (including CNN detection) using CPU only.
This paper presents a new information source for supporting robot localisation: material composition. The proposed method complements the existing visual, structural, and semantic cues utilized in the literature. However, it has a distinct advantage in its ability to differentiate structurally [23], visually [25] or categorically [1] similar objects such as different doors, by using Raman spectrometers. Such devices can identify the material of objects it probes through the bonds between the material’s molecules. Unlike similar sensors, such as mass spectroscopy, it does so without damaging the material or environment. In addition to introducing the first material-based localisation algorithm, this paper supports the future growth of the field by presenting a gazebo plugin for Raman spectrometers, material sensing demonstrations, as well as the first-ever localisation data-set with benchmarks for material-based localisation. This benchmarking shows that the proposed technique results in a significant improvement over current state-of-the-art localisation techniques, achieving 16% more accurate localisation than the leading baseline. The code and dataset will be released at: https://github.com/ThirgoodC/RaSpectLoc
3D hand pose estimation from images has seen considerable interest from the literature, with new methods improving overall 3D accuracy. One current challenge is to address hand-to-hand interaction where self-occlusions and finger articulation pose a significant problem to estimation. Little work has applied physical constraints that minimize the hand intersections that occur as a result of noisy estimation. This work addresses the intersection of hands by exploiting an occupancy network that represents the hand’s volume as a continuous manifold. This allows us to model the probability distribution of points being inside a hand. We designed an intersection loss function to minimize the likelihood of hand-to-point intersections. Moreover, we propose a new hand mesh parameterization that is superior to the commonly used MANO model in many respects including lower mesh complexity, underlying 3D skeleton extraction, watertightness, etc. On the benchmark INTERHAND2.6M dataset, the models trained using our intersection loss achieve better results than the state-of-the-art by significantly decreasing the number of hand intersections while lowering the mean per-joint positional error. Additionally, we demonstrate superior performance for 3D hand uplift on RE:INTERHAND and SMILE datasets and show reduced hand-to-hand intersections for complex domains such as sign-language pose estimation.
How many times does a human have to drive through the same area to become familiar with it? To begin with, we might first build a mental model of our surroundings. Upon revisiting this area, we can use this model to extrapolate to new unseen locations and imagine their appearance. Based on this, we propose an approach where an agent is capable of modelling new environments after a single visitation. To this end, we introduce "Deep Imagination", a combination of classical Visual-based Monte Carlo Localisation and deep learning. By making use of a feature embedded 3D map, the system can "imagine" the view from any novel location. These "imagined" views are contrasted with the current observation in order to estimate the agent's current location. In order to build the embedded map, we train a deep Siamese Fully Convolutional U-Net to perform dense feature extraction. By training these features to be generic, no additional training or fine tuning is required to adapt to new environments. Our results demonstrate the generality and transfer capability of our learnt dense features by training and evaluating on multiple datasets. Additionally, we include several visualizations of the feature representations and resulting 3D maps, as well as their application to localisation.
This paper presents a new information source for supporting robot localisation: material composition. The proposed method complements the existing visual, structural, and semantic cues utilized in the literature. However, it has a distinct advantage in its ability to differentiate structurally [23], visually [25] or categorically [1] similar objects such as different doors, by using Raman spectrometers. Such devices can identify the material of objects it probes through the bonds between the material's molecules. Unlike similar sensors, such as mass spectroscopy, it does so without damaging the material or environment. In addition to introducing the first material-based localisation algorithm, this paper supports the future growth of the field by presenting a gazebo plugin for Raman spectrometers, material sensing demonstrations, as well as the first-ever localisation data-set with benchmarks for material-based localisation. This benchmarking shows that the proposed technique results in a significant improvement over current state-of-the-art localisation techniques, achieving 16 % more accurate localisation than the leading baseline. The code and dataset will be released at: https://github.com/ThirgoodC/RaSpectLoc
The use of human-level semantic information to aid robotic tasks has recently become an important area for both Computer Vision and Robotics. This has been enabled by advances in Deep Learning that allow consistent and robust semantic understanding. Leveraging this semantic vision of the world has allowed human-level understanding to naturally emerge from many different approaches. Particularly, the use of semantic information to aid in localisation and reconstruction has been at the forefront of both fields. Like robots, humans also require the ability to localise within a structure. To aid this, humans have designed highlevel semantic maps of our structures called floorplans. We are extremely good at localising in them, even with limited access to the depth information used by robots. This is because we focus on the distribution of semantic elements, rather than geometric ones. Evidence of this is that humans are normally able to localise in a floorplan that has not been scaled properly. In order to grant this ability to robots, it is necessary to use localisation approaches that leverage the same semantic information humans use. In this paper, we present a novel method for semantically enabled global localisation. Our approach relies on the semantic labels present in the floorplan. Deep Learning is leveraged to extract semantic labels from RGB images, which are compared to the floorplan for localisation. While our approach is able to use range measurements if available, we demonstrate that they are unnecessary as we can achieve results comparable to state-of-the-art without them.
Motion estimation approaches typically employ sensor fusion techniques, such as the Kalman Filter, to handle individual sensor failures. More recently, deep learning-based fusion approaches have been proposed, increasing the performance and requiring less model-specific implementations. However, current deep fusion approaches often assume that sensors are synchronised, which is not always practical, especially for low-cost hardware. To address this limitation, in this work, we propose AFT-VO, a novel transformer-based sensor fusion architecture to estimate VO from multiple sensors. Our framework combines predictions from asynchronous multi-view cameras and accounts for the time discrepancies of measurements coming from different sources. Our approach first employs a Mixture Density Network (MDN) to estimate the probability distributions of the 6-DoF poses for every camera in the system. Then a novel transformer-based fusion module, AFT-VO, is introduced, which combines these asynchronous pose estimations, along with their confidences. More specifically, we introduce Discretiser and Source Encoding techniques which enable the fusion of multi-source asynchronous signals. We evaluate our approach on the popular nuScenes and KITTI datasets. Our experiments demonstrate that multi-view fusion for VO estimation provides robust and accurate trajectories, outperforming the state of the art in both challenging weather and lighting conditions.
In the context of self-driving vehicles there is strong competition between approaches based on visual localisa-tion and Light Detection And Ranging (LiDAR). While LiDAR provides important depth information, it is sparse in resolution and expensive. On the other hand, cameras are low-cost and recent developments in deep learning mean they can provide high localisation performance. However, several fundamental problems remain, particularly in the domain of uncertainty, where learning based approaches can be notoriously over-confident. Markov, or grid-based, localisation was an early solution to the localisation problem but fell out of favour due to its computational complexity. Representing the likelihood field as a grid (or volume) means there is a trade off between accuracy and memory size. Furthermore, it is necessary to perform expensive convolutions across the entire likelihood volume. Despite the benefit of simultaneously maintaining a likelihood for all possible locations, grid based approaches were superseded by more efficient particle filters and Monte Carlo sampling (MCL). However, MCL introduces its own problems e.g. particle deprivation. Recent advances in deep learning hardware allow large likelihood volumes to be stored directly on the GPU, along with the hardware necessary to efficiently perform GPU-bound 3D convolutions and this obviates many of the disadvantages of grid based methods. In this work, we present a novel CNN-based localisation approach that can leverage modern deep learning hardware. By implementing a grid-based Markov localisation approach directly on the GPU, we create a hybrid Convolutional Neural Network (CNN) that can perform image-based localisation and odometry-based likelihood propagation within a single neural network. The resulting approach is capable of outperforming direct pose regression methods as well as state-of-the-art localisation systems.
Most 3D reconstruction approaches passively optimise over all data, exhaustively matching pairs, rather than actively selecting data to process. This is costly both in terms of time and computer resources, and quickly becomes intractable for large datasets. This work proposes an approach to intelligently filter large amounts of data for 3D reconstructions of unknown scenes using monocular cameras. Our contributions are twofold: First, we present a novel approach to efficiently optimise the Next-Best View ( NBV ) in terms of accuracy and coverage using partial scene geometry. Second, we extend this to intelligently selecting stereo pairs by jointly optimising the baseline and vergence to find the NBV ’s best stereo pair to perform reconstruction. Both contributions are extremely efficient, taking 0.8ms and 0.3ms per pose, respectively. Experimental evaluation shows that the proposed method allows efficient selection of stereo pairs for reconstruction, such that a dense model can be obtained with only a small number of images. Once a complete model has been obtained, the remaining computational budget is used to intelligently refine areas of uncertainty, achieving results comparable to state-of-the-art batch approaches on the Middlebury dataset, using as little as 3.8% of the views.
How many times does a human have to drive through the same area to become familiar with it? To begin with, we might first build a mental model of our surroundings. Upon revisiting this area, we can use this model to extrapolate to new unseen locations and imagine their appearance. Based on this, we propose an approach where an agent is capable of modelling new environments after a single visitation. To this end, we introduce “Deep Imagination”, a combination of classical Visual-based Monte Carlo Localisation and deep learning. By making use of a feature embedded 3D map, the system can “imagine” the view from any novel location. These “imagined” views are contrasted with the current observation in order to estimate the agent’s current location. In order to build the embedded map, we train a deep Siamese Fully Convolutional U-Net to perform dense feature extraction. By training these features to be generic, no additional training or fine tuning is required to adapt to new environments. Our results demonstrate the generality and transfer capability of our learnt dense features by training and evaluating on multiple datasets. Additionally, we include several visualizations of the feature representations and resulting 3D maps, as well as their application to localisation.
Sensor-based remote health monitoring is used in industrial, urban and healthcare settings to monitor ongoing operation of equipment and human health. An important aim is to intervene early if anomalous events or adverse health is detected. In the wild, these anomaly detection approaches are challenged by noise, label scarcity, high dimensionality, explainability and wide variability in operating environments. The Contextual Matrix Profile (CMP) is a configurable 2-dimensional version of the Matrix Profile (MP) that uses the distance matrix of all subsequences of a time series to discover patterns and anomalies. The CMP is shown to enhance the effectiveness of the MP and other SOTA methods at detecting, visualising and interpreting true anomalies in noisy real world data from different domains. It excels at zooming out and identifying temporal patterns at configurable time scales. However, the CMP does not address cross-sensor information, and cannot scale to high dimensional data. We propose a novel, self-supervised graph- based approach for temporal anomaly detection that works on context graphs generated from the CMP distance matrix. The learned graph embeddings encode the anomalous nature of a time context. In addition, we evaluate other graph outlier algorithms for the same task. Given our pipeline is modular, graph construction, generation of graph embeddings, and pattern recognition logic can all be chosen based on the specific pattern detection application.We verified the effectiveness of graph-based anomaly detection and compared it with the CMP and 3 state-of-the art methods on two real-world healthcare datasets with different anomalies. Our proposed method demonstrated better recall, alert rate and generalisability.
Robots need to be able to work in multiple different environments. Even when performing similar tasks, different behaviour should be deployed to best fit the current environment. In this paper, We propose a new approach to navigation, where it is treated as a multi-task learning problem. This enables the robot to learn to behave differently in visual navigation tasks for different environments while also learning shared expertise across environments. We evaluated our approach in both simulated environments as well as real-world data. Our method allows our system to converge with a 26% reduction in training time, while also increasing accuracy.
This work addresses 3D human pose reconstruction in single images. We present a method that combines Forward Kinematics (FK) with neural networks to ensure a fast and valid prediction of 3D pose. Pose is represented as a hierarchical tree/graph with nodes corresponding to human joints that model their physical limits. Given a 2D detection of keypoints in the image, we lift the skeleton to 3D using neural networks to predict both the joint rotations and bone lengths. These predictions are then combined with skeletal constraints using an FK layer implemented as a network layer in Py-Torch. The result is a fast and accurate approach to the estimation of 3D skeletal pose. Through quantitative and qualitative evaluation , we demonstrate the method is significantly more accurate than MediaPipe in terms of both per joint positional error and visual appearance. Furthermore, we demonstrate generalization over different datasets and sign languages. The implementation in PyTorch runs at between 100-200 milliseconds per image (including CNN detection) using CPU only. Index Terms— 3D pose estimation, hand and body reconstruction .
Accurate extrinsic sensor calibration is essential for both autonomous vehicles and robots. Traditionally this is an involved process requiring calibration targets, known fiducial markers and is generally performed in a lab. Moreover, even a small change in the sensor layout requires recalibration. With the anticipated arrival of consumer autonomous vehicles, there is demand for a system which can do this automatically, after deployment and without specialist human expertise. To solve these limitations, we propose a flexible framework which can estimate extrinsic parameters without an explicit calibration stage, even for sensors with unknown scale. Our first contribution builds upon standard hand-eye calibration by jointly recovering scale. Our second contribution is that our system is made robust to imperfect and degenerate sensor data, by collecting independent sets of poses and automatically selecting those which are most ideal. We show that our approach’s robustness is essential for the target scenario. Unlike previous approaches, ours runs in real time and constantly estimates the extrinsic transform. For both an ideal experimental setup and a real use case, comparison against these approaches shows that we outperform the state-of-the-art. Furthermore, we demonstrate that the recovered scale may be applied to the full trajectory, circumventing the need for scale estimation via sensor fusion.
Constructing Birds-Eye-View (BEV) maps from monocular images is typically a complex multi-stage process involving the separate vision tasks of ground plane estimation, road segmentation and 3D object detection. However, recent approaches have adopted end-to-end solutions which warp image-based features from the image-plane to BEV while implicitly taking account of camera geometry. In this work, we show how such instantaneous BEV estimation of a scene can be learnt, and a better state estimation of the world can be achieved by incorporating temporal information. Our model learns a representation from monocular video through factorised 3D convolutions and uses this to estimate a BEV occupancy grid of the final frame. We achieve state-of-the-art results for BEV estimation from monocular images, and establish a new benchmark for single-scene BEV estimation from monocular video.
In this work, we introduce a new perspective for learning transferable content in multi-task imitation learning. Humans are able to transfer skills and knowledge. If we can cycle to work and drive to the store, we can also cycle to the store and drive to work. We take inspiration from this and hypothesize the latent memory of a policy network can be disentangled into two partitions. These contain either the knowledge of the environmental context for the task or the generalizable skill needed to solve the task. This allows improved training efficiency and better generalization over previously unseen combinations of skills in the same environment, and the same task in unseen environments. We used the proposed approach to train a disentangled agent for two different multi-task IL environments. In both cases we out-performed the SOTA by 30% in task success rate. We also demonstrated this for navigation on a real robot.
Estimating a semantically segmented bird's-eye-view (BEV) map from a single image has become a popular technique for autonomous control and navigation. However, they show an increase in localization error with distance from the camera. While such an increase in error is entirely expected - localization is harder at distance - much of the drop in performance can be attributed to the cues used by current texture-based models, in particular, they make heavy use of object-ground intersections (such as shadows) [10]. which become increasingly sparse and uncertain for distant objects. In this work, we address these shortcomings in BEV-mapping by learning the spatial relationship between objects in a scene. We propose a graph neural network which predicts BEV objects from a monocular image by spatially reasoning about an object within the context of other objects. Our approach sets a new state-of-the-art in BEV estimation from monocular images across three large-scale datasets, including a 50% relative improvement for objects on nuScenes.
Reconstruction of 3D environments is a problem that has been widely addressed in the literature. While many approaches exist to perform reconstruction, few of them take an active role in deciding where the next observations should come from. Furthermore, the problem of travelling from the camera’s current position to the next, known as pathplanning, usually focuses on minimising path length. This approach is ill-suited for reconstruction applications, where learning about the environment is more valuable than speed of traversal. We present a novel Scenic Route Planner that selects paths which maximise information gain, both in terms of total map coverage and reconstruction accuracy. We also introduce a new type of collaborative behaviour into the planning stage called opportunistic collaboration, which allows sensors to switch between acting as independent Structure from Motion (SfM) agents or as a variable baseline stereo pair. We show that Scenic Planning enables similar performance to state-of-the-art batch approaches using less than 0.00027% of the possible stereo pairs (3% of the views). Comparison against length-based pathplanning approaches show that our approach produces more complete and more accurate maps with fewer frames. Finally, we demonstrate the Scenic Pathplanner’s ability to generalise to live scenarios by mounting cameras on autonomous ground-based sensor platforms and exploring an environment.
Estimating a semantically segmented bird's-eye-view (BEV) map from a single image has become a popular technique for autonomous control and navigation. However, they show an increase in localization error with distance from the camera. While such an increase in error is entirely expected – localization is harder at distance – much of the drop in performance can be attributed to the cues used by current texture-based models, in particular, they make heavy use of object-ground intersections (such as shadows) [9], which become increasingly sparse and uncertain for distant objects. In this work, we address these shortcomings in BEV-mapping by learning the spatial relationship between objects in a scene. We propose a graph neural network which predicts BEV objects from a monocular image by spatially reasoning about an object within the context of other objects. Our approach sets a new state-of-the-art in BEV estimation from monocular images across three large-scale datasets, including a 50% relative improvement for objects on nuScenes.
Background:Annual national diabetes audit data consistently shows most people with diabetes do not consistently achieve blood glucose targets for optimal health, despite the large range of treatment options available.Aim:To explore the efficacy of a novel clinical intervention to address physical and mental health needs within routine diabetes consultations across health care settings.Methods:A multicenter, parallel group, individually randomized trial comparing consultation duration in adults diagnosed with T1D or T2D for ≥6 months using the Spotlight-AQ platform versus usual care. Secondary outcomes were HbA1c, depression, diabetes distress, anxiety, functional health status, and healthcare professional burnout. Machine learning models were utilized to analyze the data collected from the Spotlight-AQ platform to validate the reliability of question-concern association; as well as to identify key features that distinguish people with type 1 and type 2 diabetes, as well as important features that distinguish different levels of HbA1c.Results:n = 98 adults with T1D or T2D; any HbA1c and receiving any diabetes treatment participated (n = 49 intervention). Consultation duration for intervention participants was reduced in intervention consultations by 0.5 to 4.1 minutes (3%-14%) versus no change in the control group (−0.9 to +1.28 minutes). HbA1c improved in the intervention group by 6 mmol/mol (range 0-30) versus control group 3 mmol/mol (range 0-8). Moderate improvements in psychosocial outcomes were seen in the intervention group for functional health status; reduced anxiety, depression, and diabetes distress and improved well-being. None were statistically significant. HCPs reported improved communication and greater focus on patient priorities in consultations. Artificial Intelligence examination highlighted therapy and psychological burden were most important in predicting HbA1c levels. The Natural Language Processing semantic analysis confirmed the mapping relationship between questions and their corresponding concerns. Machine learning model revealed type 1 and type 2 patients have different concerns regarding psychological burden and knowledge. Moreover, the machine learning model emphasized that individuals with varying levels of HbA1c exhibit diverse levels of psychological burden and therapy-related concerns.Conclusion:Spotlight-AQ was associated with shorter, more useful consultations; with improved HbA1c and moderate benefits on psychosocial outcomes. Results reflect the importance of a biopsychosocial approach to routine care visits. Spotlight-AQ is viable across health care settings for improved outcomes.
Additional publications
Mendez Maldonado, Oscar (2018). Collaborative strategies for autonomous localisation, 3D reconstruction and pathplanning. Doctoral thesis, University of Surrey. Sullivan Thesis Prize Winner (2018).