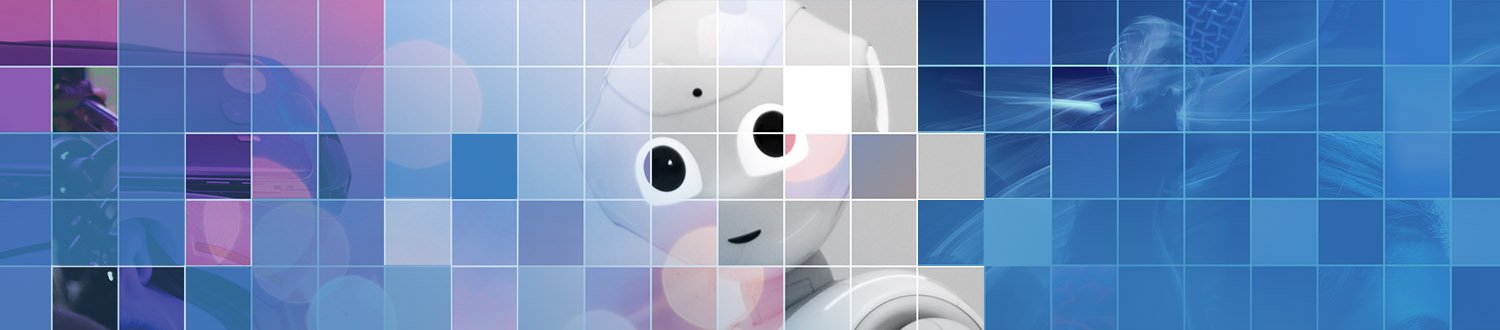
Robotics
Creating machines that can interact with the world
Robotics works on autonomous systems, covering a broad range of technologies related to visual human-machine interaction. These include sign language and autonomous vehicles.
The Future of Robotics and AI
Research leads
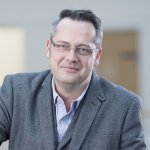
Professor Richard Bowden
Professor of Computer Vision and Machine Learning
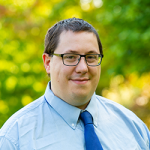
Dr Simon Hadfield
Associate Professor (Reader) in Robot Vision and Autonomous Systems
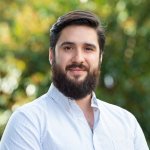
Dr Oscar Mendez Maldonado
Lecturer in Robotics and AI
Overview
Few areas of machine learning have moved faster than robotics over the past 20 years – and few will be more important in the future. In our Centre, robotics research has evolved from relatively simple experiments involving a specific task to the development of cognitive systems which can learn, adapt to new environments, and collaborate with each other.
Our consistent focus has always been building machines that can ‘learn by doing’ rather than being programmed with innate abilities. Over the years, our robotics lab has moved away from industrial robot arms to humanoid robots such as ‘Baxter’ and ‘Pepper’. Using Baxter, researchers are currently working with a major retailer to explore how the online shopping process could be optimised if robots were used in fulfilment centres to pick and bag products.
Our Centre’s robot lab also houses small mobile wheeled robots which are employed in the development of collaborative mapping strategies and the Surrey Autonomous Testbed, currently being used for our autonomous vehicles research.
Professor of Computer Vision and Machine Learning Richard Bowden explains: “As a lab we have always been interested in deploying computer vision and AI in the context of robotics as it is only when a machine can interact with the world that we have the ability to explore the relationships between perception and action. The things we perceive decide what actions we perform, and actions in the world change our perception. This is key to human learning - so why should it be any different for machines?”
Reinforcement learning helps robots adapt
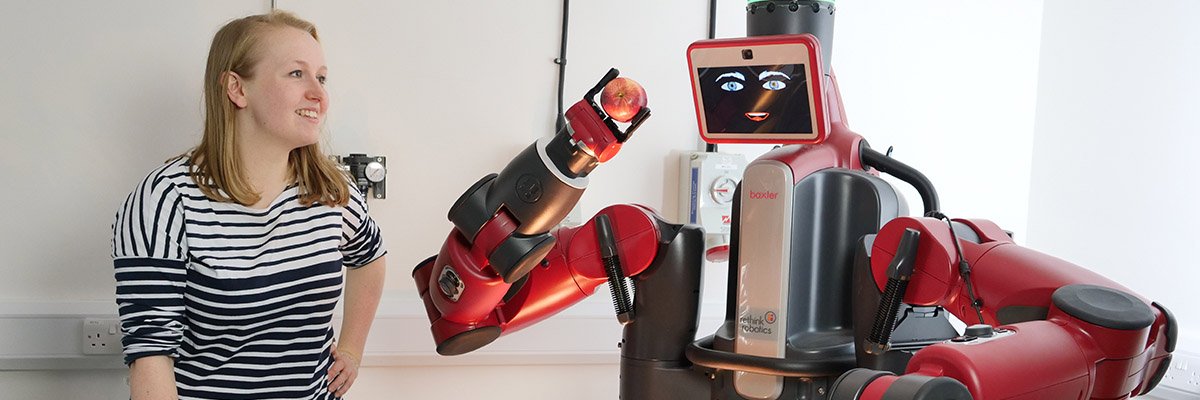
Studying for a PhD in robot vision within CVSSP, Rebecca Allday’s focus is on developing approaches using deep reinforcement learning. This allows robots to learn how to perform tasks without supervision through trial and error.
“While many industrial robots can reliably grasp and manipulate known items in predefined positions, they fail to complete the tasks when presented with anything that varies from this setup,” she explains. “Allowing robots to continuously learn through their new experiences, via reinforcement learning, makes them better at adapting to different situations.”
Since collecting training data in robotics is time consuming and tedious, Rebecca aims to create a system requiring minimal live data, which can combine computer vision and reinforcement learning to decide how to complete tasks.
Creating machines that can drive cars
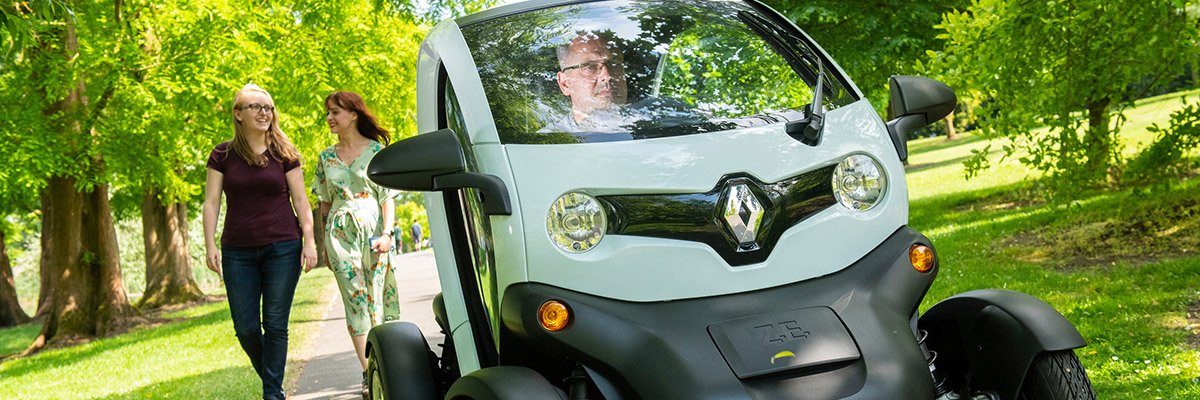
With over 25,000 people killed or seriously injured in UK road accidents in 2016, developing safety systems which could reduce fatalities is a key focus for the car industry. We are taking a novel approach to this challenge, using deep learning to develop machines that not only see but also understand.
At present autonomous vehicle research relies heavily on LIDAR, a surveying method that uses pulsed laser light to measure distances. Not only is this technology expensive, it also – while providing useful depth perception around a vehicle – fails to offer the higher level of understanding required for vehicles to act within an environment.
Our recent work in this field focuses on perception and understanding, and demonstrates that LIDAR is not needed. Our vision is to design and validate a novel intelligent control platform to enable the next generation of autonomous vehicles which not only drive but also think safely for themselves, thereby, making them capable of operating in complex and uncertain driving scenarios. To support this work, we are developing the Surrey Autonomous Testbed, based on a Renault Twizy: a road legal, fully sensor-enabled car with autonomous control and on-board processing. This technology is being made open source, enabling others to benefit.
Professor of Computer Vision and Machine Learning Richard Bowden explains: “I’m currently interested in what DARPA (Defense Advanced Research Projects Agency) calls ‘third wave AI’, something that can bring together the strengths of traditional AI (first wave) and modern deep learning (second wave).
“Visual perception is key to autonomy, but we need machines that can understand what they see and predict the actions of other agents that they interact with. Our autonomous testbed is an ideal platform for development of 3rd wave AI solutions.”
Localising using semantic vision
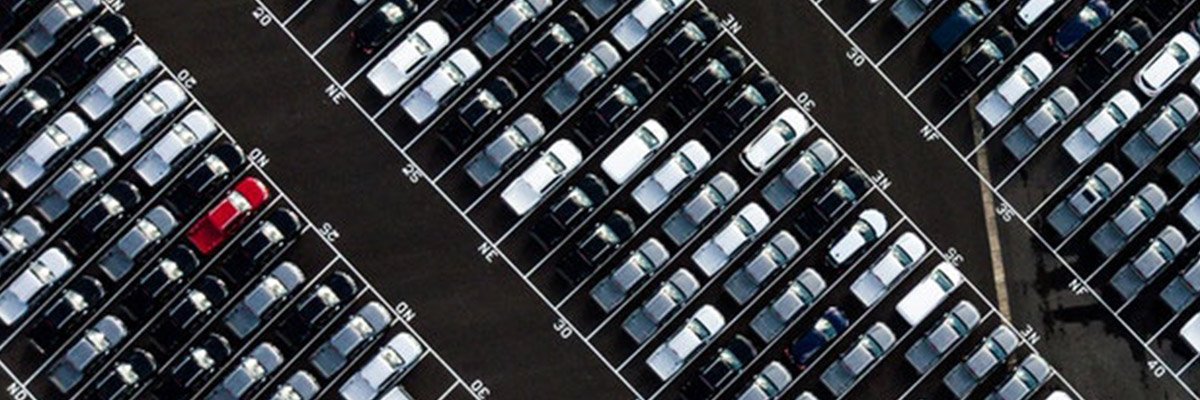
Autonomous Valet Parking (AVP) is a 30-month project funded by Innovate UK which will demonstrate fully autonomous parking in a multi-storey carpark without GPS, using computer vision alone. On the automatic vehicle control scale of 0-5, where level 5 means complete autonomy, this technology is level 4.
Lead Research Fellow Dr Oscar Mendez Maldonado explains: “Using LIDAR is akin to a human using a tape measure to measure distances to everything they can see. This is fundamentally not how humans do it: for localisation we use high level semantic cues or landmarks.”
The project builds on Dr Mendez’s PhD work on SeDAR (Semantic Detection and Ranging), which demonstrated that robotics can localise using semantic vision in the same way humans do, and can achieve the same accuracy as LIDAR using cameras alone.
Creating machines that can translate sign language
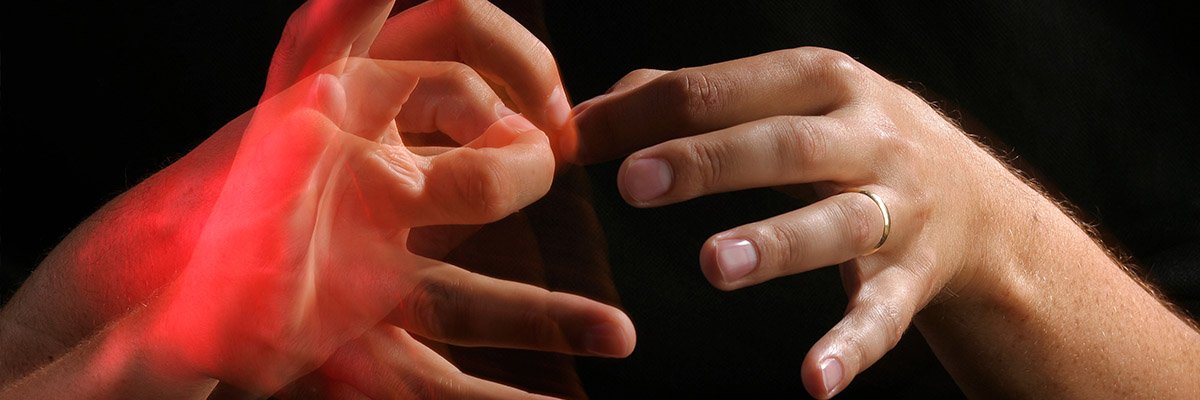
While we use visual (non-verbal) cues in everyday communication without pausing to think about it, there is also a world of visual communication that is as rich and expressive as any spoken language in the form of sign languages. Building machines that can understand, produce and translate sign to spoken language (and vice versa) would be a powerful tool for deaf-hearing communication.
Speech recognition has been an active area of research for many decades – long before devices such as Alexa and Siri entered our homes. Within CVSSP, we have focused on sign language recognition and associated technology such as gesture, facial expression and lipreading for the past 20 years. However it is only recently that technological leaps have allowed full translation of sign language to be tackled.
Building a machine that understands sign is a difficult task for many reasons. Each country has its own sign language and – as sign has no written form – datasets are sparse, with limited annotation. Understanding sign is not just about understanding a sequence of gestures: the grammar of sign uses space to position objects, direction to indicate verbs, and facial expressions to convey and modify meaning. It is this complex interplay of face, body, hands and grammar that makes automatic translation extremely challenging.
Professor of Computer Vision and Machine Learning Richard Bowden explains: “I feel we are unique in taking the trouble to ensure that everyone who works on sign, learns how to sign. After all, how can you hope to solve a problem that you don’t fully understand? Even so, we work closely with sign linguists and the deaf community.”
Developing learning tools
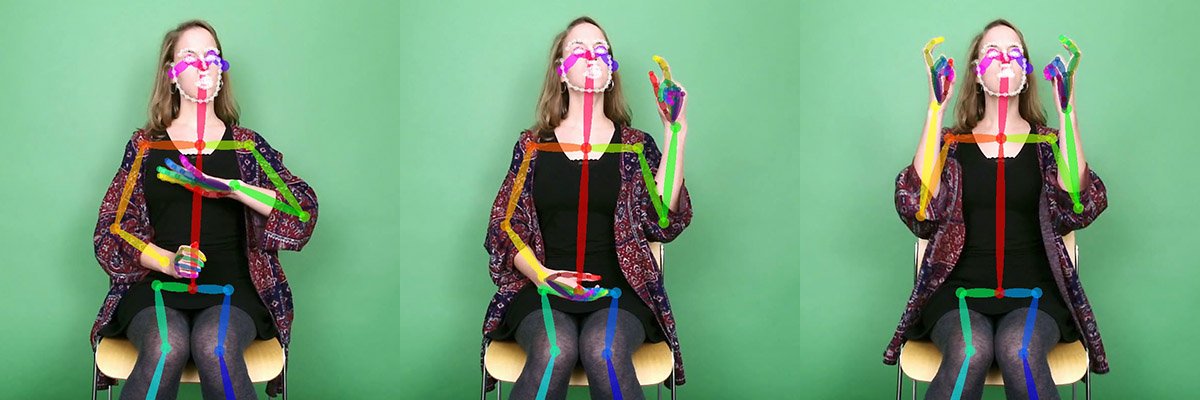
As a PhD student on the SMILE project (funded by the Swiss National Science Foundation), Necati Cihan Camgoz has helped to develop a tool, based around a game, which aims to assess students learning Swiss-German sign. The tool captures a student performing a sign, and provides feedback on how well it was performed and what could be improved in terms of hand shape, motion, location and timing.
As part of this project, Cihan published the world’s first end-to-end translation system for German sign to spoken German, and is now applying his expertise to the new EPSRC project ExTOL (End to End Translation of British Sign Language). The aim of ExTOL is to build an AI system capable of watching a human signing British Sign Language and turning this into written English, enabling linguists to increase their knowledge of the language and feed this knowledge back into the translation system.
Research projects
- SMILE - Scalable Multimodal sign language Technology for sIgn language Learning and assessment
- Context4ALL - Personalised Content Creation for the Deaf Community in a Connected Digital Single Market
- AVP - Autonomous Valet parking”
- ExTOL - End to End Translation of British Sign Language
- CogViSys
- COSPAL
- URUS
- DIPLECS
- Dicta-Sign.
- LILiR
- Making Sense
- Learning to Recognise Dynamic Visual Content.