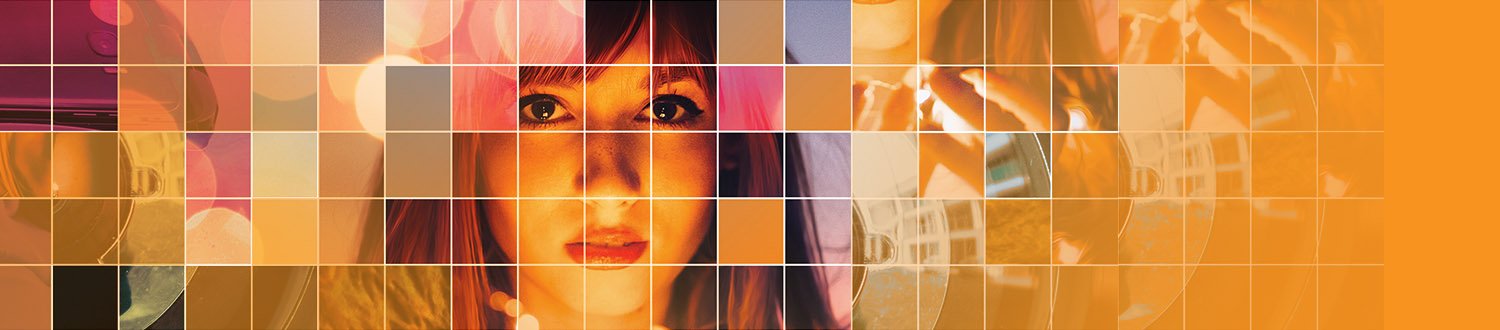
Security
World-leading face recognition technology
Security theme works on biometrics related technologies, specialising in facial recognition and natural language interfaces for human-AI collaboration.
Research lead
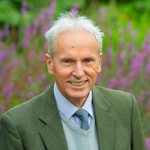
Professor Josef Kittler
Distinguished Professor
Overview
Since the mid-1990s, a major focus for our Centre has been the huge potential of machine perception to deliver applications that can keep society safe. Building on our expertise in face analysis techniques, we are developing biometric solutions that exploit information such as face shape and texture, lip dynamics and soft biometrics.
Biometrics: Making the world a safer place
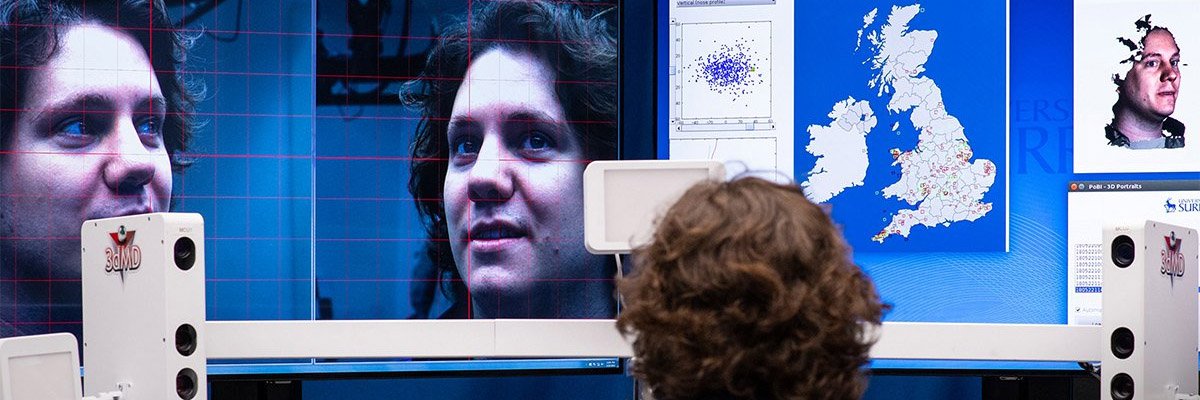
One of our key specialisms is pattern recognition, and our research in this sector is leading to exciting new applications in security, border control and access to facilities and services.
We are halfway through a five-year face recognition research project – FACER2VM – after being awarded a £6 million Programme Grant from the Engineering and Physical Sciences Research Council in 2016. This project, in collaboration with Imperial College and the University of Stirling, aims to develop ‘unconstrained’ facial recognition by addressing challenges posed by face appearance variations.
Face recognition can be very challenging, because the appearance of a subject in two images can be very different, due to different illumination, pose, expression, occlusion and noise. We have addressed these challenges in a variety of ways, including 3D reconstruction of 2D face images, face detection and face landmarking, face spoofing detection, linking face shape to DNA, person re-identification and tracking.
Professor Josef Kittler, who leads research on biometrics in CVSSP, explains: “We’re interested in the fusion of information derived from multiple biometric traits which together can produce a far more reliable solution. For example, if someone is sitting in front of a computer, you can capture their image, record their voice and detect unique features of the dynamics of their talking face. We’ve developed a fundamental methodology for fusing information, which is leading us in exciting new directions.”
Keeping track on the centre of attention
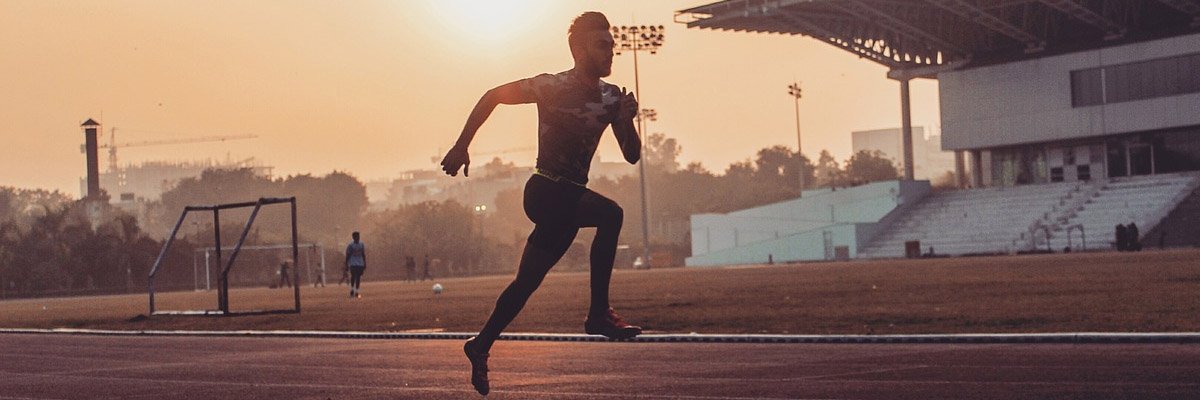
Tracking of visual targets is an important functionality of human and animal perception systems, often essential for their survival. It involves fusion of multiple cues, derived from motion, visual content matching and target recognition. We are developing algorithms that effectively harness different video cues to advance technologies in machine target detection and tracking.
As for biological systems, tracking objects in a video is important for many AI applications, including autonomous navigation, robotics, defence, camera control, and visual surveillance. A considerable research effort around the world, including at CVSSP, is devoted to developing high-performance tracking algorithms, which can keep an object of interest in the centre of attention.
The challenge of visual tracking is posed by the presence of multiple moving targets, image noise, low target resolution, complex dynamic background, illumination changes, occlusion, and frequently the changing appearance of a target due to its 3D and/or articulated nature. The early motion-based tracking algorithms have recently been superseded by learning algorithms which incorporate an increasing number of cues to improve performance and robustness. But is the growing dimensionality of target representation counterproductive?
Professor Josef Kittler, from CVSSP, explains: “Adding more cues contributes useful information on the one hand, but it often contaminates the target representation by irrelevant or redundant information. The secret of successful tracking is in eliminating this spurious information content.”
CVSSP and Jiangnan University win the Visual Object Tracking 2018 Competition
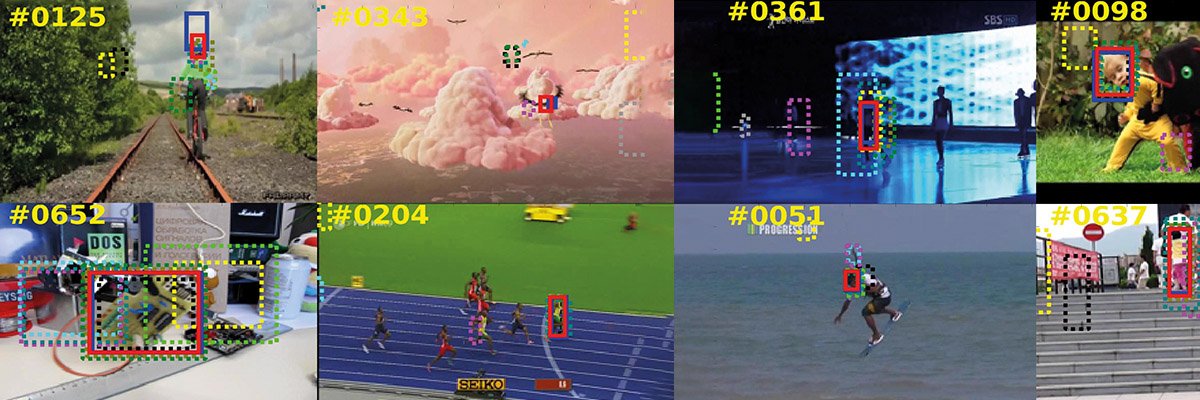
The progress in the field of visual object tracking is regularly assessed by international Visual Object Tracking (VOT) competitions. The solution developed by a team from University of Surrey and Jiangnan University in China ranked number one in the most recent visual object tracking competition, VOT 2018, on its public datasets.
The competition had 56 participants from the leading labs all over the world. The result was announced at the premier computer vision conference ECCV 2018. The winning algorithm was developed by Tianyang Xu under the supervision of Professor Xiaojun Wu (Jiangnan University), Dr Zhenhua Feng and Professor Josef Kittler (both CVSSP).
The effectiveness of the winning algorithm is shown on a number of challenging videos. The object to be tracked is defined in the first frame of the video. The subsequent frames show how successfully the object is tracked by various tracking algorithms. The Surrey/Jiangnan method is shown by the red bounding box. Other trackers are identified by different colours.
Human intelligence versus AI
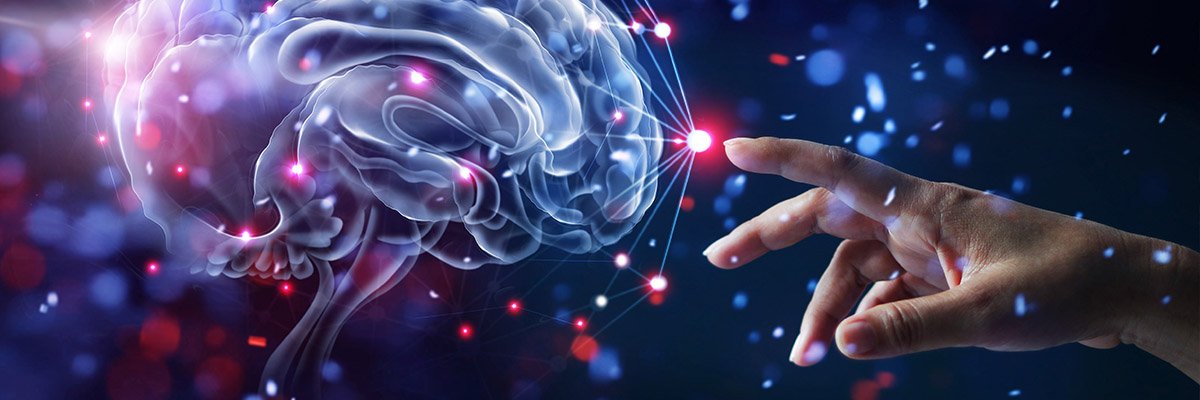
Will artificial intelligence scale up to emulate or even supersede human intelligence in all its respects, and what will it take? Our research tackles some of the world’s pressing challenges in AI, which could lead to improvements in security, and protection against fraud, crime and terrorism.
The last decade has witnessed unparalleled advances in AI. The step change in understanding how to design deep neural networks mimicking the human brain is manifest in unbelievable achievements, with a machine beating the world champion chess player, and Google AphaGo defeating the 18 times world champion Lee Sedol in the strategic board game Go. This raises a question: at what point, if ever, will the meteoric rise of AI surpass human intelligence?
CVSSP’s Professor Josef Kittler says: “AI now achieves unprecedented performance, often exceeding that of humans, in well-defined, routine data analysis tasks. What is still missing is the ability to cope with unexpected situations and anomalies, as invariably there is not enough data for training AI systems for such scenarios. Presently, AI also has no capability for making decisions in complex multi-criteria situations involving value-based judgement.”
CVSSP’s machine face recognition
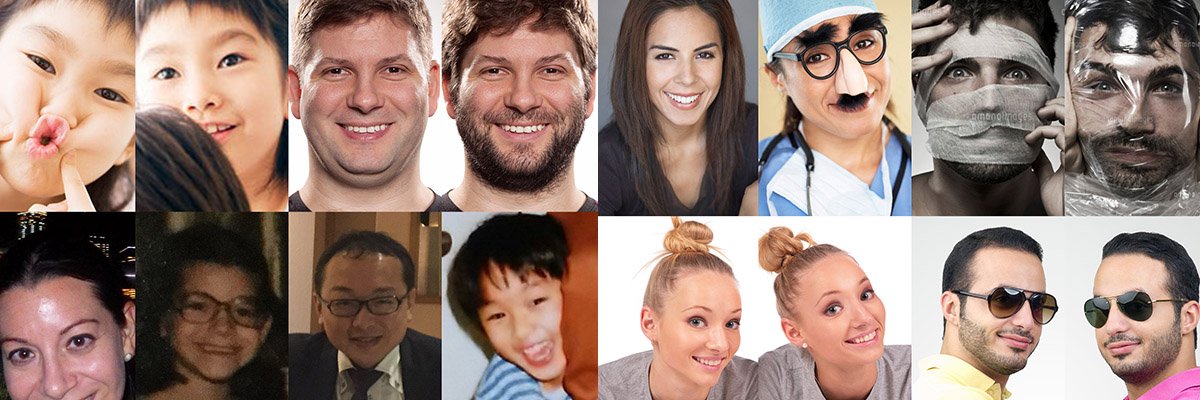
The core task of face recognition is face matching, which involves a comparison of two face images and deciding whether they belong to the same subject or not. The problem of face matching is challenging, because the appearance of a subject in two images can be very different, due to different illumination, pose, expression, age, resolution, occlusion and noise.
Humans have an extremely well-developed ability to recognise faces; and a small percentage of the population have an exceptional gift of remembering and matching faces. These people are known as super recognisers, and they have recently been the subject of a TV programme on the human brain, produced by the Japanese TV broadcaster, NHK. In this programme, NHK set to demonstrate the superiority of human face perception over the face recognition technology developed at Surrey.
In the NHK show, the test presented to a super recogniser, and to the CVSSP machine face recognition system, was devised by Dr Josh Davis, a psychologist from the University of Greenwich. Interestingly, in this standard test the face matching results produced by the machine were perfect, outperforming the human super recogniser by a margin of 20 per cent. However, in a supplementary test involving particularly challenging images, such as twins, heavy disguise, face distortion and occlusion the super recognisers performance was much better than that of the automatic system. The ability of humans to cope with unusual situations is still hard to emulate.
Running in the family – are hereditary facial features controlled by a single gene?
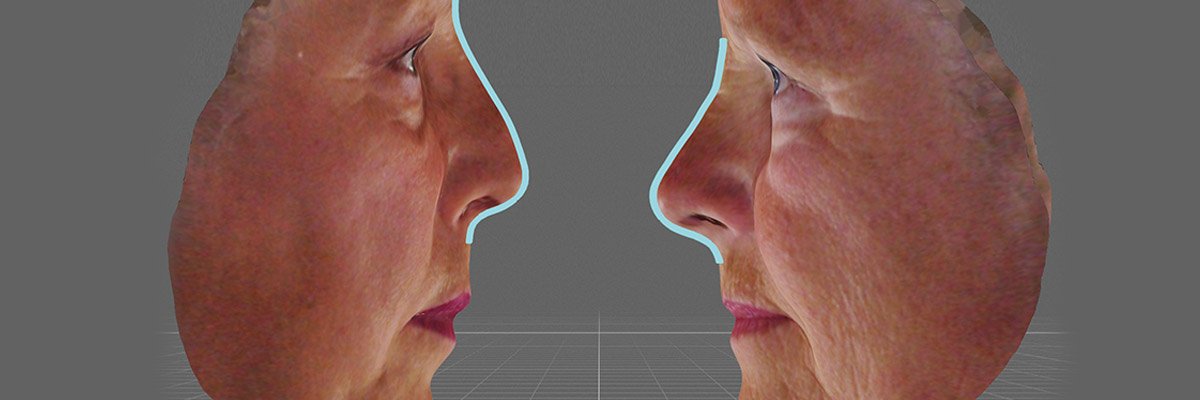
Do you have your grandmother’s eyes? Or your father’s nose? A new study by the Universities of Surrey and Oxford has uncovered variations to singular genes that have a large impact on human facial features, paving the way to understanding what determines the facial characteristics passed on from generation to generation.
Because human faces are so variable, we use them to recognise each other. Anecdotally, we see that similar facial features tend to occur in families, from one generation to the next. The extreme similarity of identical twins shows that most of this variability is genetically determined.
Researchers at CVSSP analysed over 3,000 faces of twins.The features of the face shape were analysed for heritability and those of high heritability index selected for further analysis.
The University of Oxford took the facial analysis and identified two genetic variants tied to facial profiles in females and one variant tied to shape features around the eyes in both males and females. Another variant was linked to a gene that is involved in regulating steroid biosynthesis. The study, which was published by Proceedings of the National Academy of Sciences in 2018, found that a single gene can have a large and specific effect on a person’s facial features.
Professor Josef Kittler said: “This is another example of how machine intelligence can have a positive impact and contribution to scientific discovery.”
Developing natural language interfaces for human–AI collaboration
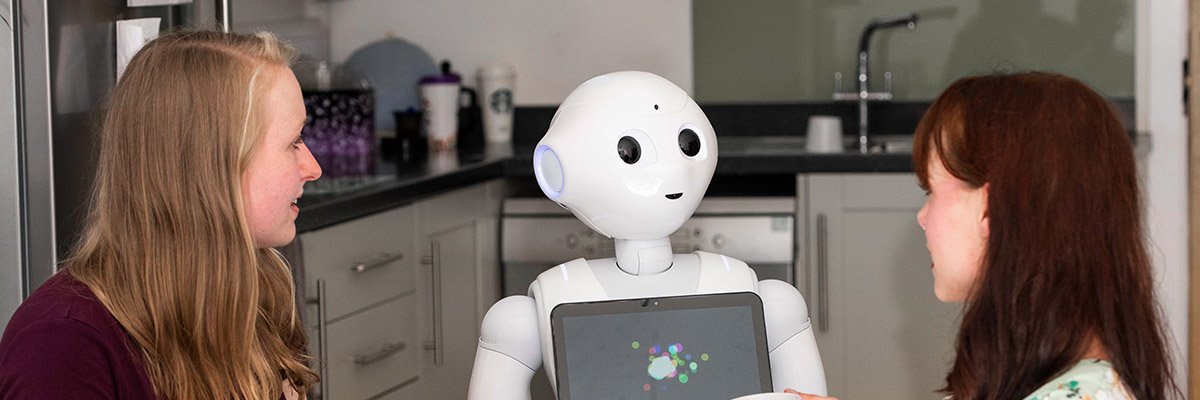
The ultimate goal of AI research is complete autonomy. However, the most effective deployment of AI systems in the foreseeable future is likely to be in an assistive and collaborative mode, with AI supporting humans at work and at home.
While AI systems are more efficient and often better than humans in performing routine tasks, human intelligence exhibits unsurpassed edge in complex decision making, involving multiple criteria and handling anomalous situations. Humans must also assume the ultimate responsibility for any action that an AI system takes.
For these reasons, the ideal mode of deployment of AI systems would be in an assistive capacity, supporting humans in their daily tasks. And for effective collaboration between machines and humans, natural communication is required – natural language.
We are developing AI technology for natural language processing. The key challenge is to build a bridge between the domain of the deployed AI system expertise – e.g. computer vision – and natural language. Interestingly, the natural language processing technology also opens multimodal processing, where different modalities (vision, language, audio) are jointly brought to bear on data analysis tasks, such as multimedia information retrieval.
Person re-identification in video with language and vision
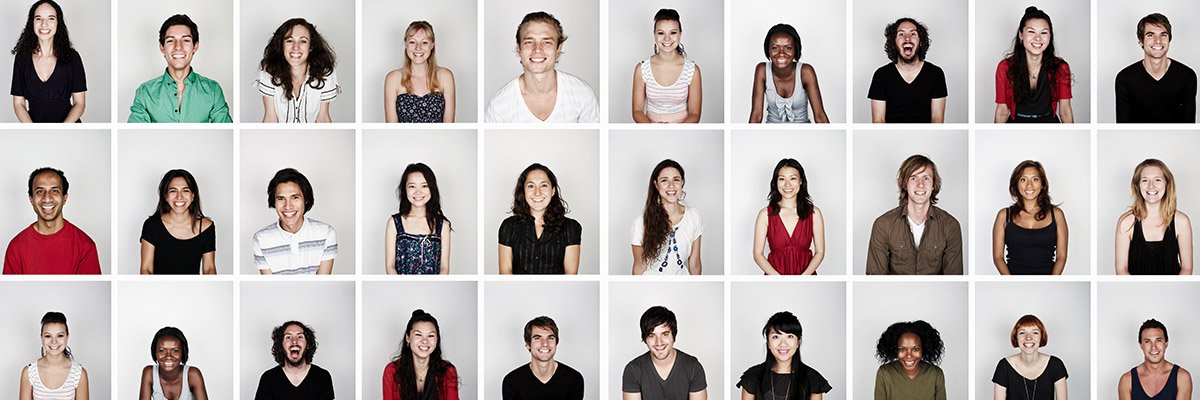
Forensic video analysis invariably involves the task of searching for suspects across the recordings captured by different cameras. The search is normally based on the subject appearance as specified by visual examples. However, in many scenarios a description of the crime scene is provided by a witness in the form of natural language. We are developing deep neural networks that enable cross-modal (language to vision) searches of video footage.
The system being developed learns the same concepts expressed in terms of natural language as well as in visual form. The representations learnt for the two modalities are then linked by another neural network to allow cross-modal matching.
The resulting solution supports searches of image/video databases defined by language queries, but, by the same token, it facilitates the retrieval of language description content from textual databases corresponding to a given visual query.
Professor Josef Kittler comments: “As an interesting by-product of our cross-modal matching research we have demonstrated that when both linguistic and visual descriptions of the search query are available, the retrieval performance can be improved significantly. This suggests that a major benefit can be gained from augmenting visual search query by a textual description.”
Latest research news
Research projects
- EPSRC Programme Grant: FACE2RVM (2016-2020) “Face matching for Automatic Identity Retrieval, Recognition, Verification and Management” Surrey-Imperial-Sterling (£6.1M, 2014-20)
- MURI/EPSRC project: (2018-2023) “Semantic information pursuit for multimodal data analysis.”
- EPSRC/dstl project: (2013-2018) “Signal processing solutions for the networked battlespace”