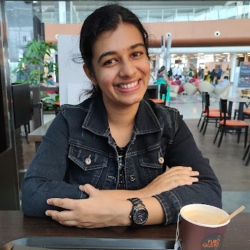
Silpa Vadakkeeveetil Sreelatha
Academic and research departments
Centre for Vision, Speech and Signal Processing (CVSSP), Surrey Institute for People-Centred Artificial Intelligence (PAI).About
My research project
Interpretable Representation Learning using Generative modelsGenerative models have witnessed significant performance improvements in image synthesis over the last decade with the introduction of the generative adversarial network (GAN), variational autoencoder (VAE), and Diffusion models. Extensive research has been carried out to demonstrate their utility in applications such as super-resolution image synthesis, text-conditioned image generation, and many others. However, it is necessary to identify the interpretable and disentangled representations concealed within the generative models to widen their ability to extrapolate, which is a critical component of human representation capabilities. For instance, a model that learns to generate digits should capture representations corresponding to digit identity, shape etc in separate units. Some of the advantages of learning such representations are ; 1. They aid in the controllable generation, which may be used in various applications such as zero-shot classification, image manipulation, etc. 2. They can be used to synthesize counterfactual images which could be used in explainable AI, fairness, and robustness. My project aims to learn interpretable representations in the generative models which could be used to improve the robustness, explainability and fairness of the classifiers.
Supervisors
Generative models have witnessed significant performance improvements in image synthesis over the last decade with the introduction of the generative adversarial network (GAN), variational autoencoder (VAE), and Diffusion models. Extensive research has been carried out to demonstrate their utility in applications such as super-resolution image synthesis, text-conditioned image generation, and many others. However, it is necessary to identify the interpretable and disentangled representations concealed within the generative models to widen their ability to extrapolate, which is a critical component of human representation capabilities. For instance, a model that learns to generate digits should capture representations corresponding to digit identity, shape etc in separate units. Some of the advantages of learning such representations are ; 1. They aid in the controllable generation, which may be used in various applications such as zero-shot classification, image manipulation, etc. 2. They can be used to synthesize counterfactual images which could be used in explainable AI, fairness, and robustness. My project aims to learn interpretable representations in the generative models which could be used to improve the robustness, explainability and fairness of the classifiers.
Publications
Neural networks trained on biased datasets tend to inadvertently learn spurious correlations, hindering generalization. We formally prove that (1) samples that exhibit spurious correlations lie on a lower rank manifold relative to the ones that do not; and (2) the depth of a network acts as an implicit regularizer on the rank of the attribute subspace that is encoded in its representations. Leveraging these insights, we present DeNetDM, a novel debiasing method that uses network depth modulation as a way of developing robustness to spurious correlations. Using a training paradigm derived from Product of Experts, we create both biased and debiased branches with deep and shallow architectures and then distill knowledge to produce the target debiased model. Our method requires no bias annotations or explicit data augmentation while performing on par with approaches that require either or both. We demonstrate that DeNetDM outperforms existing debiasing techniques on both synthetic and real-world datasets by 5%. Source code will be available upon acceptance.
Neural networks trained on biased datasets tend to inadvertently learn spurious correlations, hindering generalization. We formally prove that (1) samples that exhibit spurious correlations lie on a lower rank manifold relative to the ones that do not; and (2) the depth of a network acts as an implicit regularizer on the rank of the attribute subspace that is encoded in its representations. Leveraging these insights, we present DeNetDM, a novel debiasing method that uses network depth modulation as a way of developing robustness to spurious correlations. Using a training paradigm derived from Product of Experts, we create both biased and debiased branches with deep and shallow architectures and then distill knowledge to produce the target debiased model. Our method requires no bias annotations or explicit data augmentation while performing on par with approaches that require either or both. We demonstrate that DeNetDM outperforms existing debiasing techniques on both synthetic and real-world datasets by 5%.